How to Group Interview Responses by Motivation or Goal
-
Bella Williams
- 10 min read
Motivation-Based Grouping forms an essential framework for analyzing interview responses effectively. In the fast-paced environment of qualitative research, capturing the driving forces behind participant answers can significantly enhance the insights gathered. By focusing on motivations and goals, researchers can unveil deeper meanings within the data, encouraging more tailored and effective decision-making strategies.
This method allows for the systematic categorization of responses, streamlining the analysis process and reducing subjective bias. As teams sift through diverse perspectives, Motivation-Based Grouping serves as a compass, guiding them to align their findings with strategic objectives. Emphasizing motivations not only enriches the analysis but also fosters a more empathetic understanding of interviewees, ultimately leading to improved solutions and outcomes.
Analyze & Evaluate Calls. At Scale.
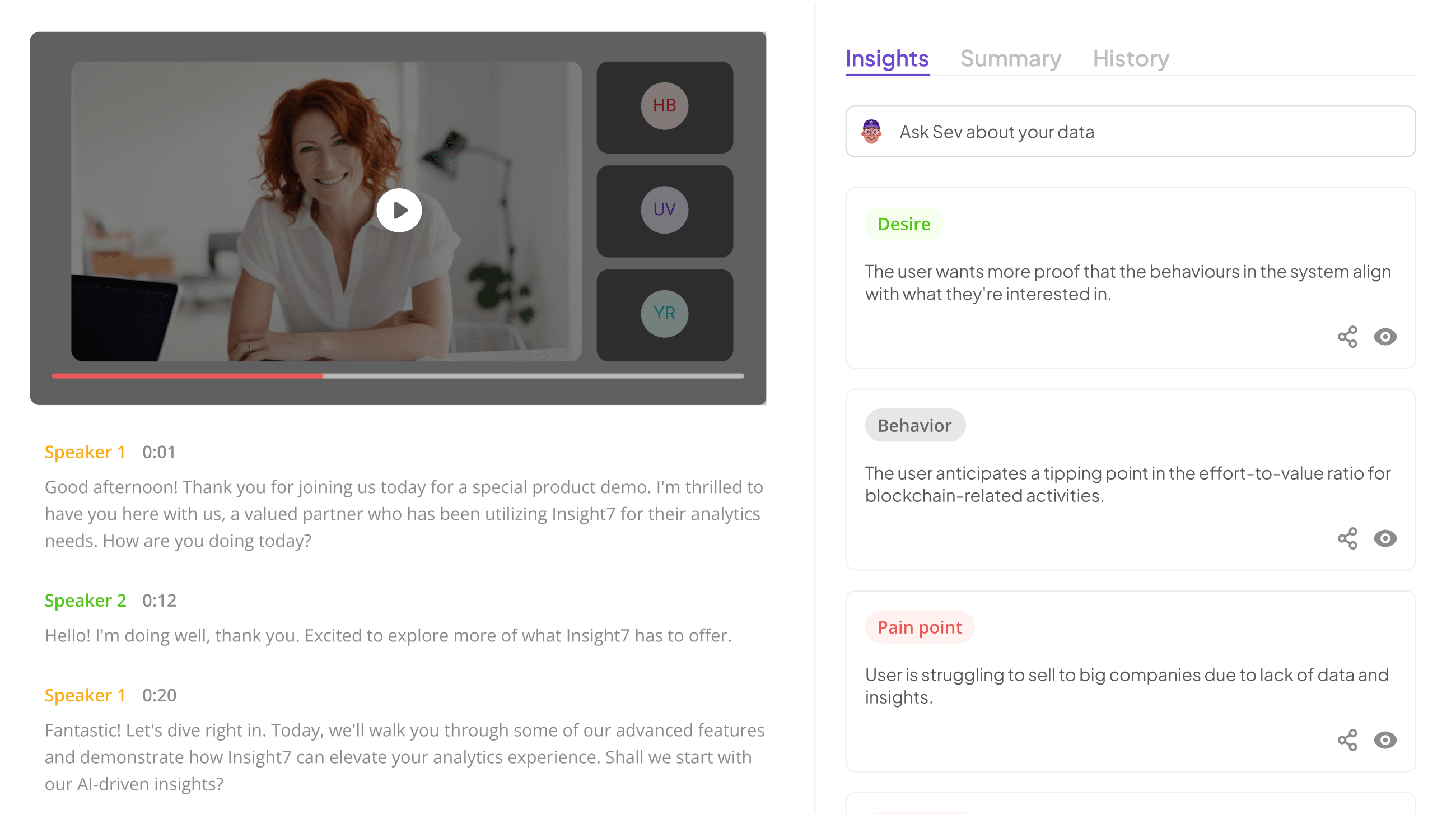
Understanding Motivation-Based Grouping
Understanding Motivation-Based Grouping is essential for effectively analyzing interview responses. By recognizing the motivations and goals of respondents, interviewers can gain deeper insights into their thoughts and preferences. This grouping process allows for a more organized analysis, helping identify patterns related to specific motivations. For example, individuals motivated by career advancement may express concerns that differ from those seeking a better work-life balance.
Motivation-Based Grouping can significantly enhance the quality of the insights extracted from interviews. By categorizing responses based on identified motivations, researchers can make data-driven decisions that align with user needs. Additionally, this approach improves overall analysis efficiency, saving valuable time during the synthesis phase. As this methodology unfolds, continuous exploration of themes and insights will contribute to a richer understanding of target audience dynamics, ultimately leading to more impactful outcomes.
Defining Motivation and Goal in Interviews
In interviews, understanding motivation and goals is essential for gaining deep insights into responses. Motivation drives individuals to express their thoughts and feelings, revealing their true intentions. By identifying the motivations behind answers, interviewers can comprehend not just what is said but why it is said. This understanding forms a foundation for meaningful analysis and informed decision-making.
To effectively utilize motivation-based grouping, interviewers should employ strategies that uncover these motivations. Open-ended questions encourage interviewees to share their genuine thoughts, leading to richer data collection. Additionally, categorizing responses based on identified motivations helps streamline the analysis process. This systematic approach enhances clarity and allows for better synthesis of insights, ultimately equipping decision-makers with the knowledge to address key objectives and challenges. Understanding and categorizing motivations transforms qualitative data into actionable strategies.
- Discuss the importance of identifying motivations and goals in interviews.
Understanding motivations and goals in interviews is crucial for deriving meaningful insights. Identifying what drives candidates can reveal their deeper intentions and priorities, which significantly influences their responses. This understanding helps interviewers discern patterns in behavior and thought processes, leading to more effective analyses. By focusing on these motivations, interviewers can tailor their questions to elicit richer and more relevant information.
The benefits of motivation-based grouping extend to improving analysis efficiency and enhancing decision-making. When responses are organized based on motivations, it streamlines the evaluation process for researchers and practitioners alike. Clear identification of goals allows for a more refined analysis of the data collected, helping stakeholders make better-informed decisions. In essence, recognizing motivations not only enriches the interview process but also enhances the overall effectiveness of qualitative research.
- Explanation of how motivations influence responses.
Understanding how motivations influence responses is key to effective interviews. When individuals express their thoughts, their motivations are often the driving force behind their answers. This connection shapes the narrative, leading to insights that align with their goals and desires. By recognizing these influences, interviewers can gain a deeper understanding of the underlying factors that shape responses.
Moreover, utilizing Motivation-Based Grouping enables the analysis of these motivations in a structured manner. For instance, categorizing responses by themes such as pain points, desires, and behaviors allows for clearer insights. This clustering not only simplifies data interpretation but also reveals patterns that may not be apparent at first glance. Ultimately, understanding how motivations shape responses can significantly enhance the quality of insights gathered, paving the way for more informed decisions in any research or development process.
Benefits of Motivation-Based Grouping
Motivation-Based Grouping provides significant advantages for analyzing interview responses effectively. This approach streamlines the analysis process by categorizing responses based on underlying motivations, enabling a clear understanding of participant goals. When responses are grouped by motivation, common themes emerge more quickly, facilitating faster insights and decision-making.
One key benefit of Motivation-Based Grouping is improved analysis efficiency. By clustering responses according to motivations, practitioners can quickly identify trends and patterns in the data, reducing the time spent on analysis. Additionally, this grouping enables more accurate decision-making. Insights derived from responses that align with specific motivations allow decision-makers to tailor strategies and solutions effectively, ensuring that they meet the needs and expectations of their audience. These benefits collectively enhance the quality and relevance of insights gained from interviews.
- Improving analysis efficiency.
To improve analysis efficiency, leveraging a systematic approach to Motivation-Based Grouping is essential. Identifying core motivations helps streamline the process of extracting relevant insights from interview responses. By categorizing responses based on these motivations, analysts can quickly access targeted data that informs decision-making. This clustering can significantly reduce the time spent sifting through unrelated responses, allowing for a more focused analysis.
A powerful way to enhance analysis efficiency is to utilize thematic analysis tools. These tools can automatically collate insights into distinct categories, facilitating a clear overview of participant sentiments. For instance, sorting responses by themes like challenges, desires, or specific goals ensures that the insights gathered are actionable and directly relevant to the intended objectives. By adopting these practices, researchers can not only save time but also enhance the quality and depth of their analyses.
- Enhancing decision-making accuracy.
To enhance decision-making accuracy, it is essential to employ Motivation-Based Grouping in the analysis of interview responses. This methodology not only aids in understanding candidates' underlying motivations but also streamlines the process of extracting relevant insights. By categorizing responses based on shared goals or motivations, decision-makers can focus on the factors that drive individuals' behaviors and opinions.
First, accurately identifying core motivations through effective questioning techniques can significantly improve the precision of the analysis. Open-ended questions encourage more comprehensive responses and reveal deeper motivators that influence candidates. Next, categorizing these responses into distinct groups enables focused analysis, reducing ambiguity and potential bias. When equipped with this structured understanding, decision-makers can confidently formulate strategies and action plans that align with the identified motivations, ultimately enhancing both the effectiveness of the decision-making process and the overall quality of insights derived.
Steps to Implement Motivation-Based Grouping
Implementing Motivation-Based Grouping begins with a clear understanding of the core motivations of your interview subjects. Start by conducting thorough interviews with open-ended questions that encourage participants to express their experiences and goals. This technique ensures you gather rich insights that reveal underlying motivations.
Next, categorize the collected responses based on the identified motivations. Group similar responses to help visualize patterns and common themes. For instance, if multiple candidates voice a desire for collaboration, create a distinct category for it. This categorization process aids in effective analysis, allowing you to quickly identify trends and formulate actionable insights. By following these steps, you can create a structured approach that enhances decision-making and streamlines your analysis process significantly.
Extract insights from interviews, calls, surveys and reviews for insights in minutes
Step 1: Identifying Core Motivations
To accomplish effective Motivation-Based Grouping, the first step is to identify core motivations among interview responses. Start by crafting open-ended questions that allow participants to express their thoughts and feelings freely. This approach invites detailed responses, providing essential insights into what drives the candidates' goals. Through these questions, you can discover underlying motivations that may otherwise go unnoticed.
After gathering responses, analyze the data for recurring themes. Pay attention to key phrases and sentiments expressed by participants. Creating a thematic map can help visualize the connections between different motivations and related goals. Interpreting these patterns will guide you in understanding the motivations behind their responses, enabling a structured approach to categorizing insights later in the process. Identifying core motivations is crucial for successful analysis, setting the foundation for greater understanding and informed decision-making.
- Techniques to determine candidate motivations.
To effectively determine candidate motivations, it's vital to employ various techniques that delve into their responses. Open-ended questions serve as a powerful tool, encouraging candidates to express their thoughts freely. This method allows for deeper insights into their underlying motivations and aspirations. Additionally, active listening plays a crucial role in identifying subtle cues in tone and body language, which can reveal unspoken desires and concerns.
Grouping interview responses by motivation demands a systematic approach. One can categorize responses based on common themes, such as personal growth, job satisfaction, or work-life balance. Analyzing the emotional intelligence displayed by candidates can further enhance understanding. Overall, utilizing these techniques will facilitate a more structured analysis, ensuring that motivations are not just heard but are methodically mapped to inform decision-making.
- Importance of open-ended questions.
Open-ended questions play a pivotal role in Motivation-Based Grouping during interviews. These questions provide candidates the space to express their thoughts and feelings in their own words, facilitating a deeper understanding of their underlying motivations. Unlike closed-ended questions that yield limited responses, open-ended inquiries elicit richer information, allowing interviewers to capture nuanced insights.
Moreover, open-ended questions encourage candidates to elaborate on their experiences and aspirations, fostering a more engaging dialogue. This dialogue reveals not only what candidates seek in their roles but also how their personal goals align with the organization's values. By effectively utilizing open-ended questions, interviewers can cultivate a comprehensive view of responses, making it easier to group insights based on motivation or goal. Ultimately, these questions enrich the analysis process, leading to more informed decision-making and enhanced recruitment strategies.
💬 Questions about How to Group Interview Responses by Motivation or Goal?
Our team typically responds within minutes
Step 2: Categorizing Responses
Categorizing responses is a crucial step in the interview process that allows you to distill complex feedback into understandable themes. By focusing on the motivations behind each response, you not only simplify data analysis but also reveal underlying patterns that might otherwise go unnoticed. This method enables you to group similar viewpoints, which can enhance your understanding of the key motivations driving interviewees.
To effectively categorize responses, start by employing a systematic approach. First, identify common themes or keywords across responses that signal specific motivations or goals. Next, create distinct categories for these themes, ensuring that each response is placed in the correct group based on its underlying motivation. This process can help you uncover significant insights that guide your strategic decisions and inform future actions. By categorizing responses effectively, you ensure that every voice contributes meaningfully to the overall analysis, ultimately leading to enhanced understanding and actionable insights.
- Methods for classifying responses based on identified motivations.
Classifying responses based on identified motivations is essential for deriving meaningful insights from interview data. To implement effective motivation-based grouping, begin by identifying the core motivations of respondents. Techniques such as open-ended questions allow participants to express their thoughts freely, offering deeper insights into their motivations. Notably, understanding these motivations influences how responses are interpreted and categorized.
Once motivations are identified, categorize responses into distinct groups reflecting those motivations. This can involve clustering responses by shared themes or sentiments. For instance, responses can be sorted into categories such as "pain points," "desires," or "behaviors." Each category can then be analyzed further to extract actionable insights. This structured method enhances both clarity and depth in data analysis, ultimately leading to more informed decision-making. By thoughtfully classifying responses, organizations can gain a comprehensive understanding of their audience’s motivations, guiding strategies and initiatives effectively.
- Examples to illustrate effective categorization.
Effective categorization can significantly enhance the analysis of interview responses, especially when focused on motivations or goals. Consider three key examples: first, grouping responses by participants’ expressed desires for leadership opportunities. This categorization not only highlights a common theme but also enables targeted strategies for talent development. Second, responses that reveal interest in work-life balance can be clustered to create initiatives addressing employee well-being, showcasing a company’s commitment to its workforce.
Lastly, statements emphasizing teamwork can be categorized to foster collaboration programs. By effectively organizing these insights, decision-makers can align strategies with employee values, ultimately creating a more engaged workforce. Adopting such motivation-based grouping enhances clarity in interpreting interview data, leading to actionable insights that resonate with organizational goals.
Tools for Effective Motivation-Based Grouping
To facilitate effective motivation-based grouping, utilizing the right tools is essential. Various applications and software can streamline the process, enabling deeper analysis and clearer insights. One notable tool is Insight7, which offers features tailored for analyzing interview responses based on participant motivations. Its ability to categorize themes and sentiments assists in pinpointing specific goals, making it easier to connect data with actionable insights.
Additionally, tools like NVivo, Dedoose, and MAXQDA are invaluable for qualitative analysis. NVivo provides robust coding options for identifying trends and motivations in responses, while Dedoose assists in mixed-methods research, offering a comprehensive view of qualitative and quantitative data. MAXQDA excels in text analytics, allowing for efficient grouping by themes. Lastly, Atlas.ti is particularly effective for visual data mapping, which can enhance understanding of complex motivations. Together, these tools empower researchers to conduct thorough motivation-based grouping, optimizing the analysis of interview responses.
Insight7
In the realm of interview analysis, understanding the underlying motivations that drive responses is essential. Insight7 delves into Motivation-Based Grouping, a method that allows analysts to uncover patterns by identifying these motivations. By categorizing responses according to shared goals, organizations can better grasp the emotional and rational factors influencing interviewees. This approach fosters a deeper connection to the heart of the feedback, leading to more relevant insights that aid decision-making.
To implement Motivation-Based Grouping effectively, it is crucial to first identify the core motivations of participants. Employing open-ended questions encourages interviewees to express their thoughts freely, providing richer data for analysis. Once motivations are detected, responses can be categorized systematically based on these insights. This direct alignment between motivations and responses enables a more streamlined analysis process, ultimately improving the overall efficiency in extracting actionable insights from interviews.
- Features and benefits for motivation-based grouping.
Motivation-Based Grouping offers several features and benefits that significantly enhance the interview analysis process. By categorizing responses based on the motivations that drive them, you can streamline your analysis and gain deeper insights into participants' perspectives. This method allows for a more structured approach to data interpretation, where themes naturally emerge, highlighting key areas of interest or concern. Consequently, insights derived from grouped responses are often more relevant and actionable.
One of the primary benefits of this grouping approach is improved efficiency. By clustering responses according to motivation, you reduce the time spent sifting through data and can focus on analyzing themes and sentiments. Additionally, enhanced accuracy in decision-making follows because you better understand participants’ underlying desires and challenges. This clarity empowers you to address specific needs effectively and tailor your strategies accordingly. Ultimately, Motivation-Based Grouping fosters a more informed and focused dialogue in response analysis.
Other Helpful Tools
Incorporating various tools can significantly enhance the process of motivation-based grouping in interview responses. Firstly, NVivo stands out as a robust qualitative analysis tool that assists in organizing data, identifying patterns, and deriving meaningful insights. By using coding features, researchers can easily tag responses based on participant motivations, facilitating a deeper understanding of the data.
Dedoose complements this approach by catering to mixed-methods research. Its capability to integrate qualitative and quantitative data allows researchers to view responses through multiple lenses. In a similar vein, MAXQDA supports text analytics, enriching the grouping process by applying thematic analysis to uncover underlying goals. Lastly, Atlas.ti provides visual data mapping, which not only enhances the exploration of responses but also aids in communicating findings effectively. These tools together empower researchers to group interview responses by motivation or goal efficiently and accurately.
- NVivo for qualitative analysis.
NVivo serves as a powerful tool for qualitative analysis, especially when it comes to motivation-based grouping in interview responses. By facilitating the organization and categorization of qualitative data, NVivo allows researchers to efficiently analyze complex information. Users can easily tag and code responses based on identified motivations, making it simpler to draw meaningful insights from the data. This structured approach can lead to richer interpretations and a more systematic understanding of participant perspectives.
To effectively utilize NVivo for motivation-based grouping, familiarize yourself with its core features. Start by importing interview transcripts into the software, followed by coding segments that reflect specific motivations. Next, use NVivo's visualization tools to create maps and models that illustrate connections between different responses. This dynamic method enhances the ability to observe patterns, driving informed conclusions about the motivations and goals that shaped the participants’ answers.
- Dedoose for mixed-methods research.
Dedoose serves as a valuable platform for mixed-methods research, particularly when it comes to analyzing interview responses through a motivational lens. This tool enables researchers to upload both qualitative and quantitative data, allowing for a comprehensive view of participants’ motivations and goals. By grouping interview responses based on these motivations, analysts can uncover deeper insights that may influence decision-making processes.
The application supports features such as transcription and categorization, ensuring that themes and patterns emerge clearly. Dedoose facilitates collaboration among team members, streamlining the analysis process. Not only does it assist in extracting meaningful quotes and visuals, but it also allows for real-time questioning of the data to pinpoint specific insights. Ultimately, using Dedoose for motivation-based grouping enhances the overall efficiency of research efforts, leading to more informed conclusions and actionable strategies.
- MAXQDA for text analytics.
MAXQDA serves as a powerful tool for conducting text analytics, particularly when grouping interview responses by motivation or goal. Utilizing this software can streamline the process of categorizing and analyzing qualitative data. When you upload transcripts into MAXQDA, the platform enables easy coding of specific responses based on underlying motivations. This feature is essential for analyzing large volumes of data efficiently.
By employing MAXQDA, you can visualize connections between different motivations through its user-friendly interface. Its variety of analysis tools helps identify trends and patterns, ultimately leading to deeper insights. Moreover, MAXQDA’s functionality allows for collaboration, making it easier to share findings with team members and refine your analysis collaboratively. Overall, this software can significantly enhance the effectiveness of motivation-based grouping in your research efforts.
- Atlas.ti for visual data mapping.
Using Atlas.ti for visual data mapping significantly enhances the process of Motivation-Based Grouping in interview responses. This powerful tool allows researchers to create visual representations of qualitative data, making it easier to identify themes and patterns related to motivations and goals. By employing various techniques such as mind maps and analysis kits, users can quickly organize responses, which ultimately saves valuable time.
The visual nature of Atlas.ti facilitates a deeper understanding of the insights collected during interviews. With features that allow for customized coding, researchers can define specific tags for various motivations, aligning the analysis with predetermined goals. As data is clustered under relevant themes, sentiment analysis becomes accessible, enabling a more nuanced understanding of participant feedback. The ability to visualize connections, such as between collaboration desires and retention goals, empowers researchers to make informed decisions based on comprehensive evidence derived from the interview data.
Conclusion: Maximizing Insights with Motivation-Based Grouping
In conclusion, motivation-based grouping serves as a powerful method for extracting meaningful insights from interview responses. By categorizing data according to underlying motivations, we not only streamline our analysis but also align our findings with specific goals. This approach aids in identifying trends and patterns that might otherwise be overlooked, enhancing the overall quality of our insights.
To maximize the benefits of motivation-based grouping, it’s essential to remain flexible and open-minded. A commitment to continuous improvement and adaptation will allow you to refine your categorization techniques over time. Ultimately, embracing this methodology can lead to more informed decisions and a deeper understanding of your audience's needs and desires.
💬 Questions about How to Group Interview Responses by Motivation or Goal?
Our team typically responds within minutes