How to Leverage AI to Score Qualitative Feedback at Scale
-
Bella Williams
- 10 min read
In a world flooded with qualitative feedback, the ability to distill meaningful insights is more important than ever. AI-Enhanced Feedback Analysis offers organizations a robust solution, enabling them to sift through vast amounts of feedback and uncover actionable insights efficiently. By employing advanced technologies, businesses can transform subjective opinions into objective data that drives decision-making processes.
This analysis not only helps in scoring feedback at scale but also empowers organizations to identify trends, sentiment nuances, and areas for improvement. As we delve deeper into the strategies and techniques behind AI-Enhanced Feedback Analysis, it becomes clear that understanding and implementing these tools can lead to significant advancements in customer engagement and satisfaction.
Analyze & Evaluate Calls. At Scale.
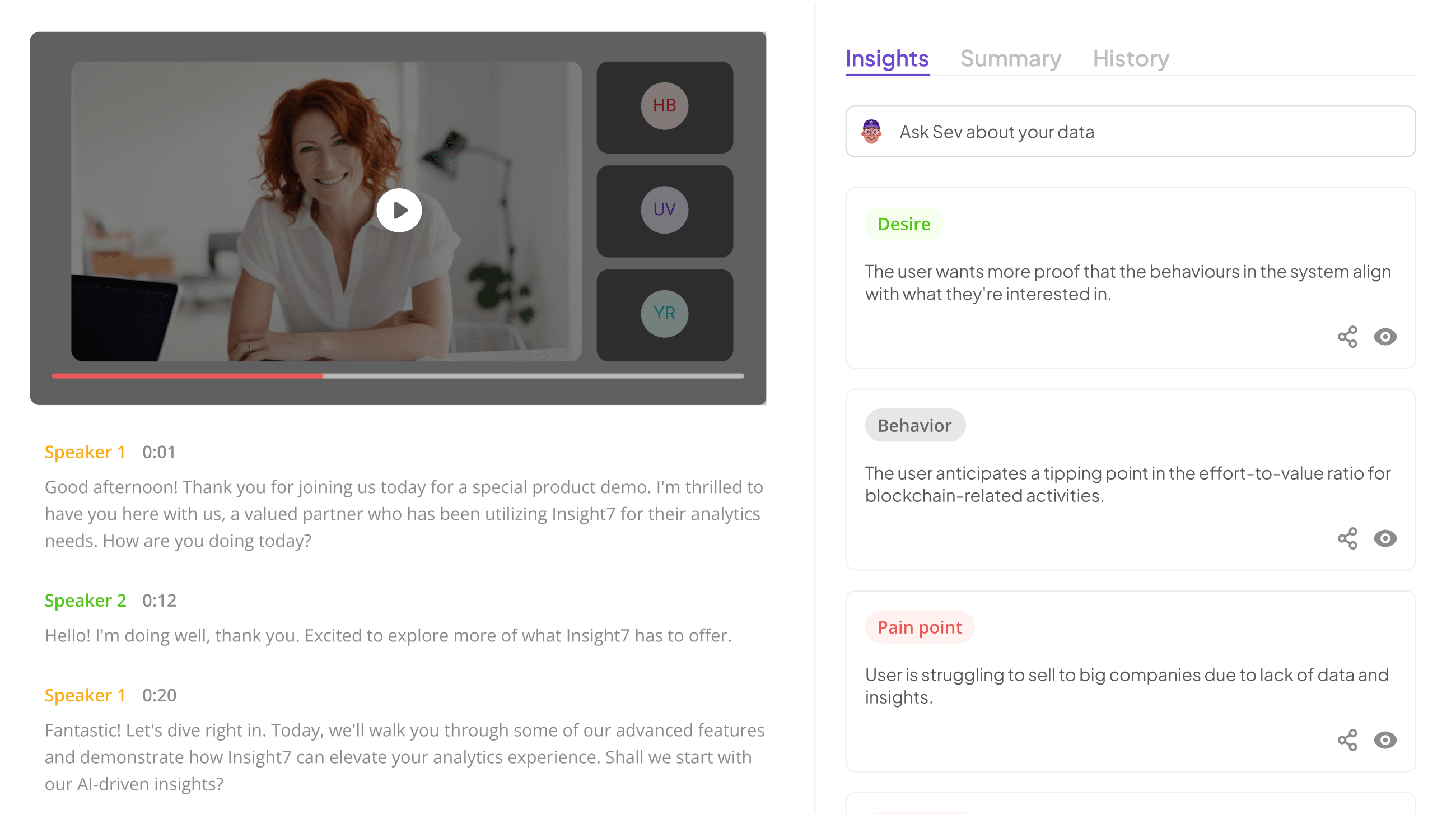
In todays data-driven world, businesses are inundated with qualitative feedback. Its vital to leverage cutting-edge technologies like AI to efficiently analyze and score this feedback at scale. This article will explore the concepts and strategies of AI-Enhanced Feedback Analysis, helping organizations unlock valuable insights from qualitative data.
In today's data-driven environment, the sheer volume of qualitative feedback can overwhelm businesses. This wealth of information, however, holds immense potential if processed effectively. Utilizing cutting-edge technologies, particularly AI, can transform these qualitative inputs into structured insights that facilitate decision-making. By embracing AI-Enhanced Feedback Analysis, organizations can not only manage feedback at scale but also derive actionable conclusions that inform product development and strategy.
Understanding the dynamics of AI-Enhanced Feedback Analysis begins with effective data management. Businesses must harness advanced tools like Natural Language Processing (NLP) and Machine Learning to automate the scoring of sentiments and identify patterns within the feedback. Additionally, these technologies enable organizations to sift through vast datasets efficiently, enabling quicker and more informed responses to customer needs. By adopting these innovative strategies, any business can unlock a treasure trove of insights that drive growth and improvement.
Understanding AI-Enhanced Feedback Analysis Techniques
AI-Enhanced Feedback Analysis techniques are essential for businesses looking to derive meaningful insights from qualitative feedback. By utilizing advanced technologies, organizations can process large volumes of feedback efficiently and effectively. These techniques enable businesses to go beyond surface-level data, tapping into deeper patterns that inform product development and customer satisfaction.
One crucial technique in AI-Enhanced Feedback Analysis is Natural Language Processing (NLP). NLP allows for the extraction of sentiment and themes from text, transforming subjective opinions into quantifiable metrics. Additionally, Machine Learning algorithms automate sentiment scoring, identifying trends and shifts in consumer sentiment over time. By employing these techniques, companies can uncover unique insights, allowing them to align their strategies with customer needs and preferences. Understanding and implementing these approaches ensures that organizations remain agile and responsive in a rapidly changing market landscape.
Before diving into actionable steps, its essential to understand how AI can be harnessed for qualitative analysis.
Before exploring actionable strategies, it's critical to grasp the potential of AI-Enhanced Feedback Analysis in transforming qualitative insights. AI technologies, particularly Natural Language Processing (NLP), enable businesses to convert vast amounts of text feedback into actionable data. By analyzing customer sentiments expressed in their comments, organizations can uncover underlying trends and patterns often missed with traditional analytical approaches.
Understanding how AI can process qualitative feedback allows companies to allocate resources more effectively. For instance, AI can efficiently analyze extensive datasets from customer interviews or surveys, identifying themes that inform product development and marketing strategies. As we delve deeper into specific techniques and tools, recognizing AI's capability to enhance qualitative analysis will empower businesses to make informed decisions and drive growth.
The Role of Natural Language Processing
Natural Language Processing (NLP) plays a critical role in revolutionizing how businesses translate qualitative feedback into actionable insights. By employing NLP techniques, organizations can automate the analysis of large volumes of qualitative data, which was previously a manual and time-consuming process. This transformation leads to faster scoring and interpretation of customer sentiment, providing firms with a clearer understanding of their audience's needs and preferences.
NLP's core functions include text classification, sentiment analysis, and topic modeling, each contributing to the wider goal of AI-Enhanced Feedback Analysis. Through effective text classification, businesses can categorize feedback accurately, allowing for a more structured approach to analysis. Sentiment analysis helps to gauge customer emotions, while topic modeling identifies recurring themes in feedback data. Together, these processes enhance qualitative feedback at scale, ensuring companies can respond to customer insights more efficiently and strategically.
Natural Language Processing (NLP) is at the core of transforming qualitative data into quantifiable insights.
Natural Language Processing (NLP) plays a pivotal role in transforming qualitative data into quantifiable insights. By effectively analyzing language patterns and sentiment within textual feedback, NLP enables businesses to process vast amounts of customer input efficiently. This technology allows organizations to gain valuable insights quickly, helping them stay competitive in today’s fast-paced environment.
The journey of AI-Enhanced Feedback Analysis begins with understanding the nuances of customer language. NLP systems are designed to interpret emotions, intentions, and context, turning subjective responses into objective data points. This transformation is crucial as it paves the way for actionable insights that inform strategic decisions. As businesses continue to generate more qualitative feedback, harnessing the power of NLP will be essential to distilling this information into meaningful metrics that drive improvements and innovation. By embracing AI-enhanced analysis, companies can streamline operations and foster deeper customer engagement.
Machine Learning for Sentiment Scoring
Machine Learning plays a crucial role in automating sentiment scoring, transforming qualitative feedback into actionable insights. By employing advanced algorithms, businesses can analyze vast datasets and identify trends in customer sentiments. This automation not only saves time but enhances accuracy, allowing organizations to make data-driven decisions quickly.
To implement AI-Enhanced Feedback Analysis effectively, the process typically consists of several key steps. First, businesses must gather and preprocess feedback data to ensure it is fit for analysis. Next, selecting the appropriate machine learning tools is vital for effective sentiment scoring. Popular options range from customizable platforms to advanced analytics solutions. Finally, interpreting the AI-driven results is crucial in understanding sentiments, helping companies refine their strategies and improve customer experiences. By following these steps, organizations can harness the power of AI for qualitative feedback analysis, unlocking valuable insights at scale.
Machine Learning algorithms are pivotal in automating sentiment analysis and uncovering trends within feedback datasets.
Machine learning algorithms serve as the backbone of AI-Enhanced Feedback Analysis, driving the automation of sentiment analysis and revealing crucial trends in customer feedback datasets. These algorithms can process vast amounts of data, identifying patterns and sentiments that would otherwise go unnoticed. For instance, positive and negative feedback can be systematically categorized, allowing organizations to get a clearer picture of customer perceptions and preferences.
The ability of machine learning to automate sentiment scoring transforms qualitative data into actionable insights. Instead of manually sifting through comments and reviews, businesses can quickly analyze sentiment at scale. This not only enhances efficiency but also contributes to more effective decision-making. By leveraging these algorithms, organizations can uncover underlying trends, ensuring they remain attuned to customer needs and expectations.
Extract insights from interviews, calls, surveys and reviews for insights in minutes
Implementing AI-Enhanced Feedback Analysis: A Step-by-Step Guide
Implementing AI-Enhanced Feedback Analysis is a transformative journey for organizations seeking to derive insights from qualitative data. The process begins with data collection and preprocessing, ensuring that your datasets are clean, structured, and ready for analysis. Utilize various methodologies, such as surveys or interviews, to gather feedback systematically. This groundwork sets the stage for the integration of AI tools.
The next step involves selecting the right AI tools tailored to your organization's needs. Options like Insight7 and MonkeyLearn offer capabilities that enhance your ability to extract insights. With the tools in place, you can analyze and interpret results. Focus on understanding trends and sentiment within the feedback, enabling data-informed decisions that align with your strategic objectives. Each stage of this process plays a crucial role in harnessing the full potential of AI-Enhanced Feedback Analysis, guiding organizations toward effective decision-making in a fast-paced environment.
Moving from theory to practice, let’s examine the steps involved in implementing AI for scoring feedback.
Implementing AI-Enhanced Feedback Analysis requires following a structured approach that transforms theoretical concepts into actionable strategies. The first step in this journey is data collection and preprocessing. You must gather relevant qualitative feedback and ensure the data is clean and organized for analysis. This foundational step sets the stage for successful AI application.
Next, selecting the right AI tools is critical for effective scoring. Popular options include Insight7, MonkeyLearn, and RapidMiner. Each tool brings unique features that cater to varying organizational needs. Incorporating these platforms allows businesses to automate sentiment scoring efficiently. Lastly, it's essential to analyze and interpret the results garnered from these tools. Understanding insights and transforming them into actionable strategies can significantly influence decision-making and drive improvements across various departments. By following these steps, organizations can fully leverage AI to enhance feedback analysis, unlocking significant value from qualitative data.
Step 1: Data Collection and Preprocessing
Collecting and preprocessing data is the foundational step in AI-Enhanced Feedback Analysis. Initially, businesses should gather qualitative feedback from diverse sources such as customer surveys, social media, and product reviews. This helps ensure a rich dataset that truly reflects customer sentiments. Once collected, the next phase is cleaning the data, which involves removing duplicates, correcting errors, and standardizing formats to make it suitable for analysis.
In this phase, it's essential to consider the context of the feedback. Is it positive or negative? Does it express a specific concern or suggestion? Categorizing feedback accurately will enhance the overall effectiveness of AI tooling in the subsequent analysis stages. Ultimately, this meticulous collection and preprocessing stage lays the groundwork for robust AI models, enabling a deeper understanding of customer insights and sentiments, which can dramatically impact business strategies.
Before engaging AI tools, its crucial to collect and prepare clean datasets ready for analysis.
Collecting and preparing clean datasets is the foundation of successful AI-Enhanced Feedback Analysis. Before you integrate any AI tools, ensure that your data is structured, accurate, and free from inconsistencies. This step is vital because the quality of input data directly influences the insights generated by the AI. Effective data preparation not only streamlines the analysis process but also ensures that the resulting insights are meaningful and actionable.
Begin by identifying relevant qualitative feedback sources, which may include surveys, customer interviews, or social media comments. Once you've gathered this information, dedicate time to clean and preprocess the data. This includes removing duplicates, correcting errors, and standardizing formats. A thorough dataset allows AI tools to perform sentiment analysis and trend identification with high accuracy, thus closing the gap between raw data and actionable insights. By prioritizing clean datasets, you empower AI technologies to deliver robust and insightful analyses that drive impactful business decisions.
Step 2: Selecting the Right AI Tools
Choosing the right AI tools is a pivotal step in executing an effective AI-enhanced feedback analysis strategy. The breadth of tools available can be overwhelming, yet selecting the right ones can dramatically enhance your ability to analyze qualitative feedback. Each tool boasts unique features tailored to specific needs and understanding these distinctions can guide you toward a successful implementation.
Consider factors like customization, ease of use, and the specific requirements of your feedback data. For example, Insight7 streamlines scoring and generates actionable insights effectively. Conversely, MonkeyLearn allows customization for unique data needs, while RapidMiner supports more detailed analytics. Lexalytics excels at translating complex feedback into actionable data. Prioritizing these aspects will help you select tools that not only align with your objectives but also amplify the efficacy of your AI efforts in scoring qualitative feedback at scale.
Choosing the correct set of tools can significantly influence the success of your AI-enhanced feedback strategy. Below are some top tools:
Choosing the correct set of tools can significantly influence the success of your AI-enhanced feedback strategy. The landscape of qualitative feedback analysis can be complex, and having the right tools at your disposal is crucial. By selecting effective AI solutions, you can automate the scoring of qualitative feedback, allowing for quicker insights and more informed decision-making processes. Integrating tools that are tailored for your specific feedback needs ensures that your overall strategy remains efficient and impactful.
Several top tools stand out in this field. Insight7 provides robust features for scoring qualitative feedback and generating actionable insights. MonkeyLearn allows you to customize models, catering to specific data requirements, making it an adaptable choice. RapidMiner is an advanced option, focusing on detailed text analytics and machine learning, while Lexalytics excels in transforming complex feedback into clear, understandable data. Lastly, Feedbackify offers an intuitive interface for real-time feedback collection and analysis, enhancing your workflow. By carefully assessing and utilizing these tools, you can achieve a successful AI-enhanced feedback analysis strategy.
insight7
To harness the power of AI-Enhanced Feedback Analysis, organizations must understand its multifaceted approach to interpreting qualitative data effectively. This method combines advanced technologies to automate the long-standing challenge of manual feedback evaluation. By deploying AI algorithms, businesses can turn unstructured feedback into actionable insights. This transformation enables quicker decision-making, driving sustained improvement across various functions.
A critical aspect of implementing AI-Enhanced Feedback Analysis lies in the integration of Natural Language Processing (NLP) and Machine Learning. These technologies work together to interpret sentiments and extract themes from qualitative responses. For instance, NLP categorizes feedback content, while Machine Learning algorithms score this data, identifying trends over time. Consequently, organizations gain a comprehensive view of customer sentiment and behavior, allowing for targeted improvements in products and services. This thorough understanding positions companies to respond adeptly to market demands, fostering deeper customer engagement and brand loyalty.
Insight7 offers comprehensive features to efficiently score qualitative feedback and generate actionable insights.
Insight7 provides a robust framework for efficiently scoring qualitative feedback and generating actionable insights. With cutting-edge technology, it empowers businesses to analyze diverse customer conversations at scale. This platform utilizes advanced Natural Language Processing and Machine Learning techniques to automate the analysis process, making it faster and more accurate than traditional methods.
One of the standout features includes user-friendly dashboards that allow teams to visualize and interpret feedback quickly. Additionally, AI-driven insights not only highlight key trends but also facilitate collaboration among team members, ensuring that critical information does not remain siloed. The ease of accessing data ensures that timely decisions can be made, which is crucial in staying ahead of the competition. Ultimately, utilizing this type of AI-enhanced feedback analysis transforms rich qualitative data into meaningful actions that drive business growth and customer satisfaction.
MonkeyLearn
AI-Enhanced Feedback Analysis is profoundly transforming how businesses interpret qualitative data. One tool that exemplifies this capability is structured to democratize insights, making them accessible to all team members, regardless of technical expertise. By facilitating straightforward workflows, users can effortlessly transcribe conversations, generate reports, and examine customer experiences to pinpoint friction points effectively.
In practice, the platform empowers users by organizing various inputs into a centralized library. Within this space, each call can be analyzed to extract vital insights related to pain points, desires, and behaviors. This analysis presents evidence-based feedback, allowing teams to make informed decisions efficiently. By focusing on customer voice and sentiments, organizations can harness the full potential of AI-Enhanced Feedback Analysis, allowing for quicker and more effective responses to client needs. As this technology evolves, its role in shaping strategic direction and enhancing customer satisfaction will only increase.
A highly customizable tool that allows businesses to train models specific to their feedback data needs.
A customizable tool like MonkeyLearn empowers businesses by enabling them to create specific models tailored to their unique feedback needs. This adaptability is essential in today’s complex landscape, where generic solutions often fall short in grasping the nuances of qualitative data. By fine-tuning models based on organizational requirements, businesses can extract finer insights, ensuring that the analysis directly aligns with their goals.
The process begins with collecting diverse feedback data from various sources, which the tool can then categorize and analyze against custom parameters. This allows businesses to prioritize sentiments and identify trends that best reflect their customers' sentiments. Through ongoing adjustments and training, these models evolve, continuously improving their ability to deliver actionable insights. Ultimately, this level of customization not only enhances the understanding of qualitative feedback but also facilitates strategic decision-making that drives business success.
RapidMiner
RapidMiner is a robust platform that facilitates advanced machine learning and text analytics, making it ideal for scoring qualitative feedback efficiently. With its intuitive interface, users can engage in comprehensive data analysis without requiring extensive coding skills. The platform supports multiple data sources, making it versatile for organizations of various sizes and needs.
Using AI-enhanced feedback analysis techniques within RapidMiner can streamline the process of interpreting qualitative data. For instance, users can automate sentiment analysis and uncover trends in feedback, enabling them to inform strategic decisions swiftly. Additionally, its powerful visualization tools allow businesses to see patterns and insights clearly. This functionality not only enhances data comprehension but also empowers organizations to react promptly to customer sentiments and experiences. Ultimately, RapidMiner stands out by offering the tools necessary to harness qualitative feedback at scale effectively.
An advanced platform for machine learning and text analytics thats suitable for detailed feedback analysis.
An advanced platform for machine learning and text analytics is essential for performing detailed feedback analysis. Such platforms empower organizations to efficiently process vast amounts of qualitative data, transforming raw insights into meaningful narratives. They combine Natural Language Processing (NLP) and advanced machine learning techniques, making it easier for users to extract valuable information without requiring extensive technical expertise.
These platforms facilitate a thorough analysis of multiple feedback sources by organizing data into accessible formats. Users can visualize key insights, identify pain points, and understand customer sentiment effectively. With features like real-time transcription and automated summarization, businesses can quickly adapt their strategies based on direct customer feedback. This holistic approach to AI-Enhanced Feedback Analysis enables organizations to harness the power of data, ensuring insights are actionable and relevant across all levels of a business.
Lexalytics
With AI-Enhanced Feedback Analysis, organizations can distill insights from qualitative data effortlessly. The approach prioritizes transforming complex feedback into clear, understandable information, enabling teams to access and act on customer sentiments swiftly. This system is designed to be user-friendly, allowing any member of the organization to participate in the analysis process without needing specialized training or technical expertise.
One of the platform's key features is its ability to categorize feedback into actionable insights. It pulls out pain points, desires, and compliments directly from customer conversations, presented visually to enhance understanding. This empowers decision-makers to pinpoint areas for improvement and strategize effectively. Additionally, group analysis capabilities allow businesses to explore multiple data sets simultaneously, further amplifying their insights while saving precious time and resources. Utilizing such technology positions organizations to better respond to customer needs and adapt quickly in a changing market.
Specializes in translating complex feedback into understandable data, perfect for business intelligence.
AI has revolutionized the ability to translate complex feedback into understandable data, essential for effective business intelligence. By utilizing advanced algorithms, businesses can convert intricate qualitative insights into clear, actionable information that drives decision-making. This process ensures that valuable feedback is not lost within vast datasets but is instead structured and easily accessible to teams.
One of the key benefits of AI-enhanced feedback analysis lies in its efficiency. Automated tools can quickly process large volumes of feedback, identifying trends, sentiments, and key themes that would be challenging to detect manually. As a result, organizations can respond faster to customer needs and market dynamics. This systematic approach not only aids in understanding customer perspectives but also aligns products and services with real-world demands, facilitating strategic growth and enhanced customer satisfaction. Through this transformation, businesses can harness the power of feedback to cultivate a more informed and adaptive operational strategy.
Feedbackify
Feedbackify is an innovative platform designed to streamline the process of collecting and analyzing customer feedback in real-time. With the surge of qualitative data generated by customer interactions, businesses often struggle to keep up with traditional analysis methods. This is where AI-Enhanced Feedback Analysis comes into play. By utilizing advanced algorithms, Feedbackify transforms raw data into actionable insights, providing teams with the tools needed to enhance customer engagement.
The key benefits of using Feedbackify include its user-friendly interface and its ability to capture sentiments efficiently. Organizations can leverage AI to identify trends and patterns that might be overlooked in manual processes. Moreover, capturing real-time feedback allows companies to respond to customer needs promptly, driving retention and satisfaction. In essence, Feedbackify exemplifies how technology can elevate the qualitative feedback landscape, enabling businesses to stay ahead of the curve and make informed, data-driven decisions.
An intuitive solution for collecting and analyzing customer feedback in real-time.
An intuitive solution for collecting and analyzing customer feedback in real-time empowers businesses to react promptly to customer insights. By integrating AI-Enhanced Feedback Analysis tools, organizations can seamlessly gather and interpret feedback as it flows in. This immediacy allows teams to address concerns swiftly, turning customer signals into actionable strategies without delay.
To streamline this process, businesses should consider the following steps:
- Automated Collection: Deploy tools that automatically gather feedback across various channels, ensuring no voice goes unheard.
- Real-Time Analysis: Utilize AI algorithms to analyze customer sentiments instantaneously, facilitating timely reactions.
- Centralized Insight Management: Centralize all feedback within a single platform, making it easier for teams to interpret and act on insights without confusion.
These strategies not only enhance operational efficiency but also cultivate a customer-centric culture, leading to improved satisfaction and loyalty. Embracing AI tools for feedback analysis enables businesses to stay ahead of their competition in today’s fast-paced environment.
Step 3: Analyzing and Interpreting Results
Once AI tools are deployed, analyzing and interpreting results becomes paramount. This stage involves meticulously reviewing the data generated by AI, focusing on consistent trends and significant insights. The use of AI-Enhanced Feedback Analysis facilitates this process, enabling businesses to uncover key themes and recurring patterns within qualitative feedback efficiently. For instance, summary statistics can reveal which pain points or suggestions are most prevalent across customer interactions.
To effectively interpret these results, you should consider several critical aspects: identify themes that align with your organizational goals, examine contextual factors influencing feedback, and visualize trends for easier understanding. This structured approach aids in converting raw insights into actionable strategies, empowering teams to make informed decisions based on customer sentiments. Emphasizing collaboration during this phase can also enhance understanding and ensure that all stakeholders have a stake in the findings, ultimately leading to better alignment in decision-making.
Once the tools are in place, interpreting AI-driven insights is crucial for driving data-informed decisions.
Once the tools for AI-Enhanced Feedback Analysis are established, the real challenge begins—interpreting the insights generated. The efficacy of AI in scoring qualitative feedback is contingent upon human understanding of the context and nuances. At this stage, it's essential to delve deep into the data to uncover trends and actionable insights that can drive strategic decisions. This process requires collaboration between data scientists and decision-makers to ensure the findings align with business objectives.
Interpreting AI-driven insights involves several key steps. First, identifying key themes, such as common pain points and customer preferences, allows organizations to prioritize issues that matter most to their audience. Second, employing evidence-based arguments from the analyzed feedback helps substantiate decision-making processes. Lastly, continuous evaluation of insights is necessary to adapt strategies and improve customer satisfaction over time. By effectively interpreting these insights, businesses can transform raw data into powerful narratives that influence present and future initiatives.
Conclusion: The Future of AI-Enhanced Feedback Analysis
AI-Enhanced Feedback Analysis is poised to shape the future of qualitative feedback management. As organizations increasingly adopt these advanced systems, they can expect to streamline workflows and accelerate decision-making. By utilizing AI to analyze feedback, businesses will automate processes that traditionally required extensive human effort, making scoring more efficient and accurate.
Looking ahead, the integration of AI technologies will enable deeper insights into customer sentiments and preferences. This will empower organizations to respond proactively to emerging trends, enhancing both customer satisfaction and loyalty. Ultimately, embracing AI-enhanced feedback analysis creates a pathway for continuous improvement, driving innovation and better outcomes across various sectors.
In conclusion, AI presents an unprecedented opportunity to revolutionize the way businesses handle qualitative feedback. By adopting AI-enhanced feedback analysis, organizations can streamline their processes, reduce manual labor, and ultimately gain a competitive edge in their market.
In conclusion, AI presents an unprecedented opportunity to revolutionize how qualitative feedback is managed. Employing AI-enhanced feedback analysis allows businesses to process vast amounts of customer insights swiftly. By automating the analysis of qualitative data, organizations can not only minimize manual efforts but also elevate the accuracy of their feedback scoring methodologies. This technological advancement empowers businesses to extract deeper insights more effectively.
Moreover, companies that incorporate AI-driven solutions can stay ahead of market trends and consumer preferences. By utilizing AI-enhanced feedback analysis, organizations can make strategic decisions based on real-time data, leading to improved products and services. This shift towards data-driven decision-making enables a more agile response to customer needs, ultimately solidifying their position in competitive markets.