AI Call Tagging has emerged as a game-changer in the realm of customer support. Imagine a scenario where every support call is automatically categorized by issue type, allowing teams to address customer concerns more effectively. This technological advancement not only streamlines processes but also enhances the overall support experience for both agents and customers.
In this section, we will explore the fundamental concepts of AI Call Tagging and its transformative potential. By employing advanced algorithms, AI systems can analyze conversations at scale, enabling support teams to focus on what matters most—providing exceptional service. Understanding how to implement AI Call Tagging will empower support organizations to better manage their resources and respond efficiently to diverse customer needs.
Analyze qualitative data. At Scale.
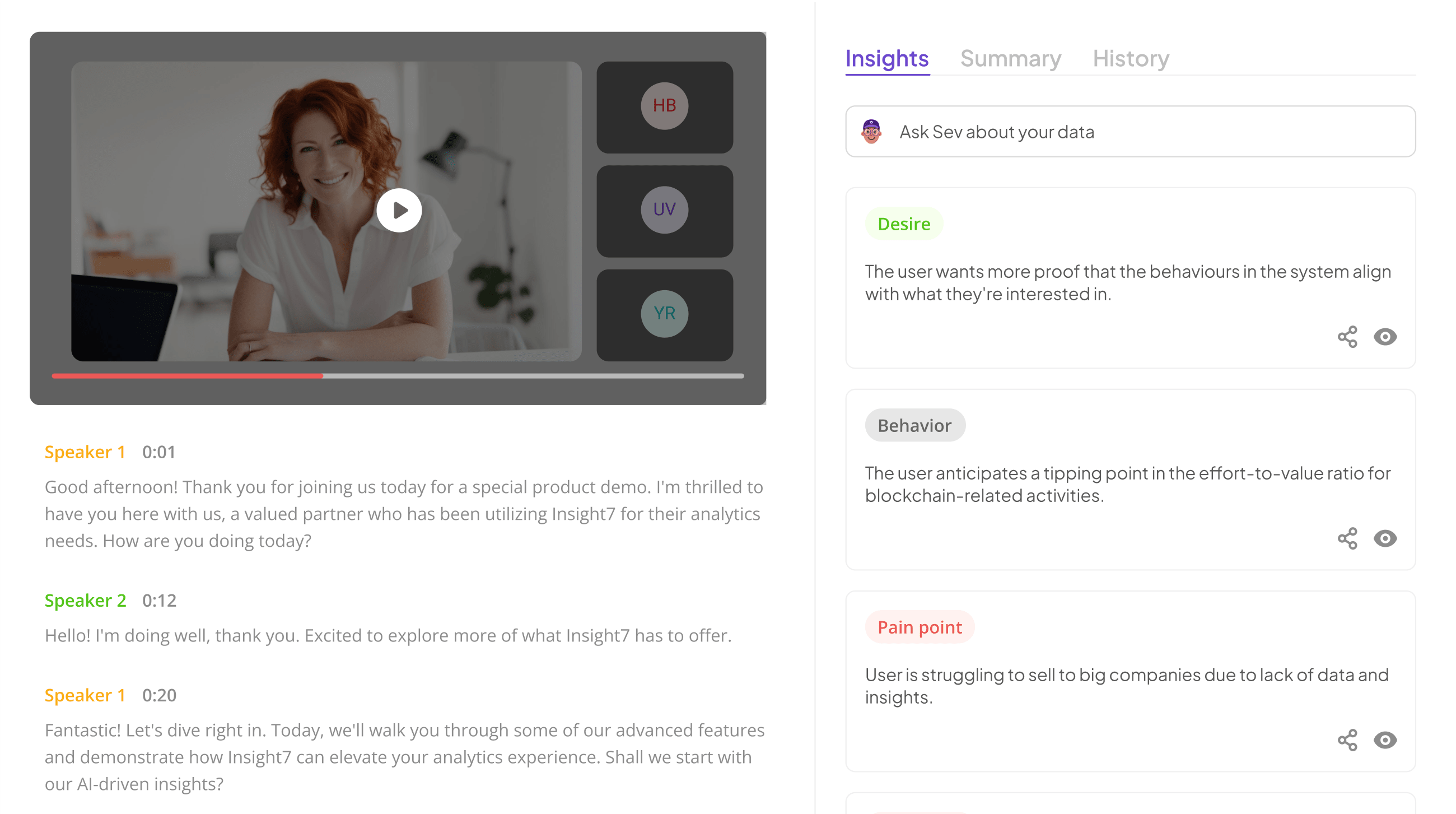
Benefits of AI Call Tagging in Customer Support
AI Call Tagging offers significant benefits for enhancing customer support operations. By automatically categorizing calls based on issue type, support teams can streamline the analysis process, enabling quicker resolutions. This efficiency reduces the time spent manually reviewing calls, allowing representatives to focus on customer engagement instead of administrative tasks. Additionally, with insights gathered from tagged data, teams can easily identify trends and recurring issues, leading to proactive improvements in service delivery.
Another advantage of AI Call Tagging is the enhancement of customer experience. By understanding the nature of customer inquiries more accurately, organizations can tailor their responses and training to address specific concerns effectively. This targeted approach leads to increased customer satisfaction and loyalty, as clients feel their needs are being understood and addressed swiftly. Overall, the implementation of AI Call Tagging not only optimizes internal processes but also creates a more customer-centric support structure, driving long-term success.
Enhancing Support Efficiency with AI Call Tagging
AI Call Tagging significantly enhances support efficiency by automating the classification of calls based on issue types. With this technology, support teams can quickly gauge the effectiveness of their responses. Instead of manually reviewing lengthy calls, AI can generate insightful reports that score Customer Service Representatives (CSRs) on their performance. This reduces the time spent on evaluating calls, allowing teams to focus more on improving service quality.
Furthermore, AI Call Tagging equips organizations with valuable customer intelligence. By analyzing data trends, it identifies prevalent inquiries, tailoring training sessions to address the most common issues faced by customers. This targeted approach helps CSRs to ramp up quickly and effectively, ensuring they are well-prepared to handle customer concerns. In sum, integrating AI in the call tagging process streamlines operations, fosters a data-driven environment, and ultimately enhances customer satisfaction.
Improving Customer Experience with AI Call Tagging
AI Call Tagging plays a significant role in elevating the customer experience by enhancing how support calls are managed and analyzed. When calls are automatically tagged according to the issues discussed, organizations can quickly identify the most common customer concerns. This streamlining not only helps in analyzing customer interactions more efficiently but also enables teams to proactively address recurring problems. As a result, customer satisfaction increases and response times improve significantly.
Moreover, AI Call Tagging allows for targeted training of customer service representatives. When support teams access data on the most prevalent inquiries, they can refine their training modules. By aligning training with real customer needs, representatives become more effective in resolving issues. Implementing this technology thus enhances the overall customer journey, fosters trust, and contributes to lasting relationships between customers and support teams.
Extract insights from interviews, calls, surveys and reviews for insights in minutes
Implementing AI Call Tagging: A Step-by-Step Guide
To implement AI call tagging effectively, begin by analyzing and categorizing common support issues. This foundational step allows you to create a clear set of tags that represent the various problems customers encounter, thus setting the groundwork for accurate tagging. By understanding and organizing these issues, your AI system can classify incoming support calls more accurately, streamlining the process significantly.
Next, select the right AI tools tailored for call tagging. Various platforms offer unique features, so it's crucial to find one that aligns with your specific needs. Once you've chosen your tools, move on to training your AI models. Ensure they learn to recognize distinct issue types based on the tags you've created. Finally, integrate AI call tagging into your existing support systems. This seamless integration allows your team to access insights quickly, enhancing responsiveness and ultimately improving customer satisfaction.
Step 1: Analyzing and Categorizing Common Support Issues
To effectively analyze and categorize common support issues, begin by reviewing past support calls for insights. This process involves collating a selection of calls and examining their transcripts to identify recurring problems and themes. By synthesizing this data, you can pinpoint common pain points that customers experience. High-frequency issues reveal patterns that can inform how your support team addresses inquiries in the future.
Next, employ AI Call Tagging to streamline the categorization process. With AI-driven tools, you can automate the tagging of calls based on identified issues, saving time and effort for your support staff. Assess the efficiency of your tagging system by regularly reviewing the tags assigned and ensuring accuracy. By creating a clear categorization framework, your organization can enhance its response strategies, leading to improved customer satisfaction and support performance. This foundational analysis paves the way for more advanced AI tools and strategies in later steps.
Step 2: Selecting the Right AI Tools for Call Tagging
Choosing the right AI tools for call tagging is essential to effectively categorize support calls by issue type. Start by evaluating your specific requirements and how different AI platforms can meet them. Look for tools that specialize in AI Call Tagging, offering features like speech recognition, natural language processing, and accurate tagging capabilities. Additionally, usability is crucial—opt for tools with intuitive interfaces that enhance your team’s productivity.
Next, consider the integration options of these tools with your existing systems. Can they easily sync with your customer relationship management (CRM) software? It's important for analysis and reporting to streamline your processes. Finally, don't overlook scalability. As your support volume grows, your chosen AI tools should grow with you, ensuring consistency and reliability in call tagging. Selecting the right AI tools forms the backbone of an efficient support system and ultimately enhances customer experience.
Insight7
To effectively leverage AI call tagging, it becomes crucial to understand the dynamic between context and customer signals. The first step involves analyzing and categorizing common support issues. By mapping out the typical queries and concerns voiced by customers, businesses can lay the groundwork for AI models to gain context and relevance. This not only streamlines the tagging process but also enhances the accuracy of issue identification.
Once the common issues have been categorized, the next step is selecting the right AI tools for call tagging. Tools like MonkeyLearn, CallMiner, or Observe.AI can offer robust solutions for automating the tagging process. These systems use natural language processing to accurately label calls, ensuring that support teams can respond effectively to customer needs. In this way, leveraging AI call tagging transforms how organizations interact with their customers, paving the way for a more efficient support operation while simultaneously improving the customer experience.
MonkeyLearn
When implementing AI Call Tagging, it’s essential to choose the right tools, and one option stands out for its user-friendly interface and robust capabilities. This platform allows teams to efficiently analyze support calls, categorizing them by various issues without requiring technical expertise. Users can easily upload call transcripts and dive straight into insights directly from the homepage.
The system excels in pinpointing key pain points, customer desires, and behaviors through intuitive visualization. Users can create projects that aggregate multiple calls, allowing for comprehensive analysis across diverse datasets. By utilizing this platform, businesses can comprehensively manage and enhance their support operations, identifying recurring issues and improving overall customer satisfaction. Embracing this innovative tool can transform how organizations handle support calls, ensuring quicker resolutions and better insights into customer needs.
CallMiner
CallMiner plays a pivotal role in the process of AI call tagging for support calls by issue type. Its advanced technology facilitates the identification and categorization of various call issues effectively. When users upload their call recordings, the software analyzes key aspects such as compliance, sales parameters, and customer inquiries, providing actionable insights. This capability enhances the overall efficiency of call management and improves the quality of customer service.
To utilize CallMiner effectively, follow these crucial steps. First, ensure that you categorize calls based on common issues, which lays the groundwork for effective tagging. Next, upload a diverse set of calls for analysis, allowing the AI to learn from different scenarios. Finally, regularly review the insights provided by CallMiner to adjust your support strategies. Implementing these strategies ensures a robust AI call tagging system that not only streamlines operations but also elevates customer satisfaction.
Observe.AI
Implementing AI Call Tagging involves using advanced technology to improve the efficiency of support teams. Tools designed for this purpose can significantly enhance the accuracy of categorizing calls based on issue type. They facilitate not just the identification of agents speaking, but also capture the essence of customer interactions. By extracting insights from conversations, these tools generate performance evaluations for each representative, offering valuable data for coaching and compliance.
To maximize the utility of AI in tagging calls, customization is often required. Organizations can tailor the system to suit their specific needs, adjusting how data is presented and ensuring a focus on compliance and quality assurance. This adaptability allows teams to edit reports easily and integrate actionable insights into their workflows. Thus, employing such AI systems transforms customer support roles and enhances overall operational effectiveness.
Step 3: Training AI Models to Recognize Issue Types
Training AI models to recognize issue types is a crucial step in the AI Call Tagging process. Begin by gathering a diverse set of support call data that reflects various issues encountered by customers. This data will serve as the foundational training set for your AI models. Each call transcript should be accurately tagged with its corresponding issue types, enabling the model to learn from real-world examples.
Next, use supervised learning techniques where the AI is trained with labeled data. This allows the model to understand patterns and features associated with different issues. As the training progresses, continuously evaluate the model’s performance through metrics like accuracy and precision. Regular updates and retraining of the model ensure it adapts to evolving support issues effectively. By prioritizing this training phase, you will enhance the model’s ability to classify calls accurately, leading to improved service and customer satisfaction.
Step 4: Integrating AI Call Tagging into Support Systems
Integrating AI Call Tagging into support systems involves several key steps that streamline operations and enhance efficiency. First, determine how AI will interface with your existing infrastructure. This could include linking AI tools to your CRM or support platform to ensure seamless data flow and accurate tagging of support calls. Consider providing the AI system with access to historical call data, allowing for better training and improved tagging accuracy.
Next, focus on customizing the AI's tagging criteria to align with your specific support needs. By defining issue types and incorporating representative samples, you help the AI distinguish between various customer concerns effectively. Additionally, establish a feedback loop where support agents can validate or refine AI-generated tags. This continuous learning process not only optimizes AI performance but also fosters a more responsive support environment. Ultimately, the integration of AI Call Tagging transforms how support teams manage inquiries, leading to quicker resolutions and enhanced customer satisfaction.
Conclusion: Transforming Support Operations with AI Call Tagging
Integrating AI call tagging transforms support operations, offering a streamlined approach to managing customer interactions. By automating the tagging process, organizations can significantly reduce the time spent manually analyzing calls. This efficiency allows teams to focus on refining their support strategies and enhancing service quality. Furthermore, AI call tagging provides crucial insights by identifying common issues and trends based on customer inquiries, enabling prompt adjustments to training and resources.
As customer needs evolve, leveraging AI call tagging becomes essential for maintaining a high level of service. Organizations can align their training efforts more closely with the actual questions customers ask, thereby enhancing team preparedness. Ultimately, the transformation brought about by AI call tagging not only improves operational efficiency but also drives customer satisfaction through timely and relevant support. This innovative approach paves the way for continuous improvement in service delivery.