AI Reliability for Healthcare Transcriptions plays a crucial role in enhancing communication within the industry. As healthcare professionals increasingly rely on technology, the accuracy of AI-based transcription becomes paramount for patient care and documentation. Understanding how AI tools operate and their effectiveness is essential for organizations aiming to streamline their communication processes.
In this exploration, we will examine the capabilities and limitations of AI tools designed for healthcare transcriptions. With many solutions available, determining which tools offer the most reliable results can be daunting. Therefore, this document will guide readers through evaluating these tools while addressing the pressing question: Are AI tools truly reliable for healthcare call transcriptions?
Analyze qualitative data. At Scale.
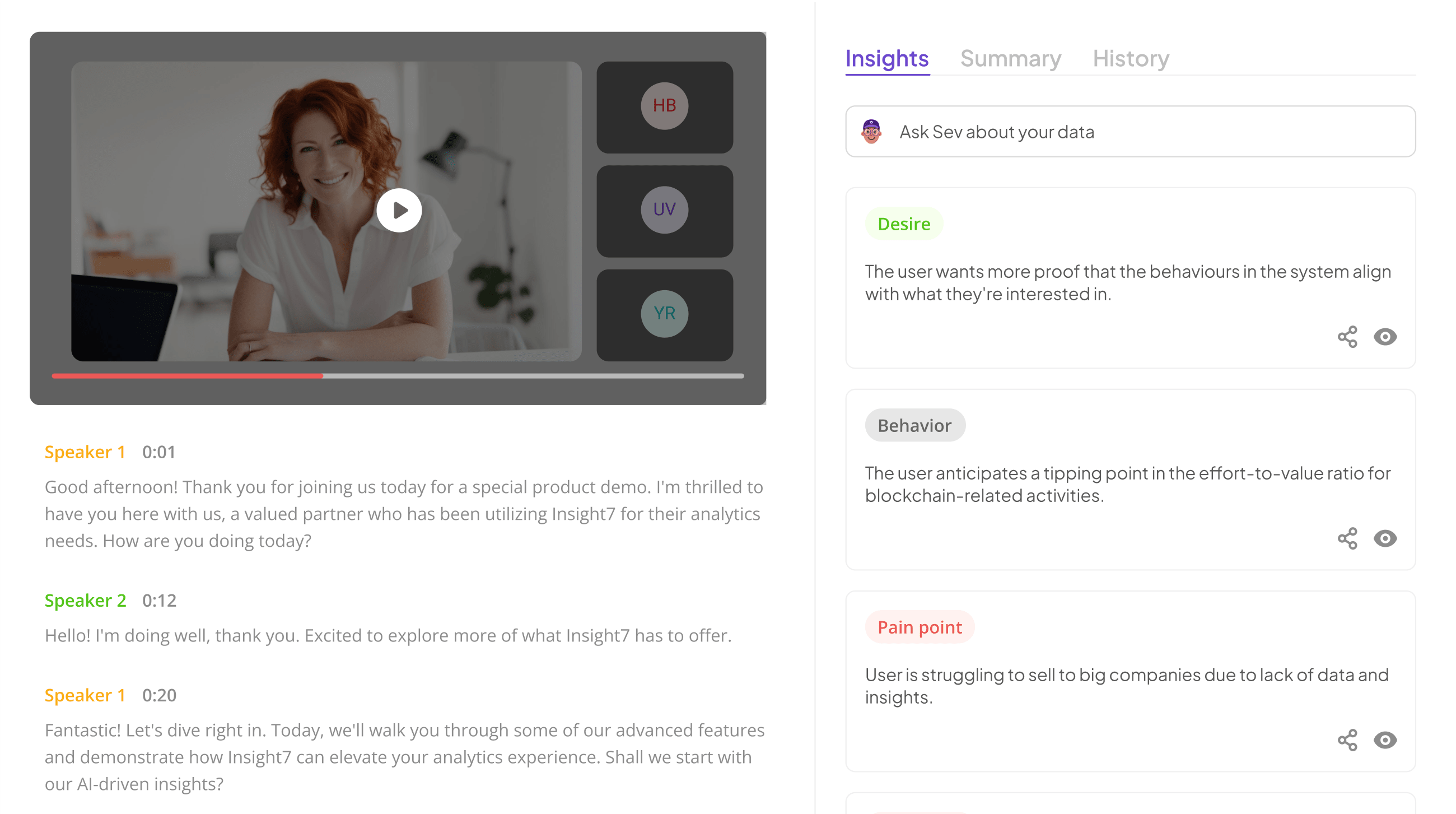
Understanding AI Reliability for Healthcare Transcriptions
Understanding AI Reliability for Healthcare Transcriptions is crucial for assessing the effectiveness of these technologies in clinical environments. AI reliability for healthcare transcriptions encompasses accuracy, consistency, and the ability to handle diverse medical terminologies. In healthcare, precise documentation is essential since even minor errors can lead to significant consequences for patient care.
To ensure effective AI reliability for healthcare transcriptions, several factors must be considered. First, the accuracy of voice recognition, particularly in distinguishing speakers, is vital. AI systems often rely on context and initial identification to improve transcription quality. Second, training the AI on specific medical vocabulary can enhance its performance in healthcare settings. Third, integrating human oversight can contribute to elevated accuracy levels, which is particularly important when managing compliance and documentation standards. In summary, while AI tools hold promise for reliable healthcare transcriptions, they require careful implementation and continuous refinement to meet clinical needs effectively.
The Role of AI in Modern Healthcare
AI is profoundly transforming modern healthcare, bringing enhanced efficiency and innovative solutions to patient care. The integration of AI technologies in various health applications is revolutionizing how healthcare professionals interact with patients and manage data. From diagnostics to administrative tasks, AI's capabilities streamline processes, reduce errors, and allow for improved focus on quality patient interactions.
Indeed, AI reliability for healthcare transcriptions plays a crucial role in this evolution. Accurate transcriptions are vital for maintaining comprehensive medical records, which are essential for effective treatment decisions. With advancements in AI tools, healthcare organizations can trust that their transcriptions will be precise and reliable. These tools not only facilitate better communication but also ensure that critical information is quickly accessible, ultimately leading to improved patient outcomes. As AI continues to evolve, its role in the healthcare sector will only expand, creating new opportunities for efficiency and effectiveness.
Challenges in AI-based Transcription for Healthcare
AI-based transcription for healthcare faces several significant challenges that can affect its reliability. One primary concern is the diverse range of accents and dialects present in health-related conversations. AI systems may struggle to accurately interpret these variations, leading to potential misunderstandings in clinical documentation. Additionally, medical terminology and jargon can pose obstacles for AI models, resulting in inaccurate transcriptions due to context misinterpretation.
Another challenge is ensuring data security and compliance with regulations like HIPAA. AI systems must maintain strict confidentiality of patient information, which is crucial for building trust among healthcare providers and patients alike. Furthermore, the integration of AI transcription tools into existing healthcare workflows can be complex. Healthcare professionals may experience hesitancy to adopt new technologies due to potential disruptions and the need for retraining. Addressing these obstacles is critical for improving AI reliability for healthcare transcriptions and ensuring that these tools can effectively support medical professionals.
Tools Ensuring AI Reliability for Healthcare Transcriptions
AI reliability for healthcare transcriptions is crucial in ensuring effective communication and maintaining patient records. Various tools enhance AI's capability to deliver precise transcriptions, thus fostering trust in technology. These tools work by utilizing advanced algorithms and natural language processing to improve accuracy, boosting efficiency in medical settings.
First, transcription tools evaluate data quality through rigorous assessments and benchmarks. They also implement feedback loops, where users can correct inaccuracies, contributing to continual learning. Additionally, these tools often incorporate domain-specific language models. This specialization enhances understanding of medical terminology, thereby increasing reliability in context. Utilizing such advanced features ensures that AI can accurately interpret nuanced medical conversations, ultimately improving patient care.
Extract insights from interviews, calls, surveys and reviews for insights in minutes
Overview of Top AI Transcription Tools
AI-powered transcription tools have become increasingly valuable in the healthcare sector. These tools offer the promise of transforming audio recordings into accurate text, allowing healthcare professionals to focus on patient care rather than administrative tasks. Understanding the landscape of top AI transcription tools is essential for evaluating AI reliability for healthcare transcriptions.
Among the most notable options are Insight7, Deepgram, Rev AI, Otter.ai, and Google Cloud Speech-to-Text. Each tool has unique features, such as bulk analysis, voice recognition accuracy, and the ability to extract actionable insights from transcripts. For instance, some tools allow users to transcribe multiple audio files simultaneously, enhancing efficiency in busy healthcare environments. By comparing these platforms, healthcare providers can determine which tools offer the best reliability and accuracy for their transcription needs, ultimately ensuring better patient documentation and care.
- Insight7
The advances in AI technology have increasingly influenced healthcare transcription, creating both opportunities and challenges. Organizations rely on AI to enhance the accuracy and efficiency of transcribing patient calls and conversations. However, this raises the question of AI reliability for healthcare transcriptions, a critical factor for patient safety and care quality.
To fully understand this concept, we should consider several critical points. First, understanding how AI interprets medical terminology and context is crucial. The AI's ability to comprehend specialized language can significantly affect transcription accuracy. Second, the integration of human oversight into the transcription process is essential for validating AI outputs. Human reviewers can catch errors that machine learning might miss, ensuring a higher reliability level. Lastly, continuous improvement of AI tools relies on feedback loops, allowing software updates tailored to healthcare needs. These factors collectively inform the assessment of AI reliability for healthcare transcriptions, guiding the best practices for successful implementation.
- Deepgram
AI tools have revolutionized the way healthcare organizations manage call transcriptions, with specific technologies enhancing AI reliability for healthcare transcriptions. This section explores one such technology, which simplifies the transcription process for medical professionals. Users can easily upload multiple audio files to streamline transcription, turning call recordings into written documents without hassle. This process allows healthcare providers to focus on patient care while ensuring valuable insights from conversations are retained.
The reliability of AI transcription tools heavily relies on their ability to accurately transcribe various accents, medical terminology, and nuanced dialogues. With advancements in machine learning, these tools are becoming increasingly adept at recognizing context and providing high accuracy rates. Furthermore, integrated analysis features enable users to extract critical insights from transcribed data quickly. As healthcare continues to evolve, enhancing AI reliability for healthcare transcriptions remains vital for improving patient outcomes and operational efficiency.
- Rev AI
Rev AI specializes in AI-driven transcription services, offering a robust solution for healthcare professionals. Its effectiveness hinges on various factors that contribute to AI reliability for healthcare transcriptions. This technology aims to automate the transcription process while ensuring accuracy and compliance with healthcare standards. The platform detects speakers based on name mentions, promoting personalized transcriptions for each call.
One noteworthy aspect of the system is its ability to generate performance insights from call data. The AI can provide analytics on engagement and compliance, crucial for healthcare settings. However, while the accuracy is generally favorable, typically around 80% to 90%, there still exists room for improvement as AI can occasionally misidentify speakers or key details. Proper customization of the platform can increase its precision and productivity, ensuring that healthcare professionals receive transcripts that genuinely reflect the discussions taking place in patient interactions.
- Otter.ai
AI tools can significantly change how healthcare call transcriptions are conducted. Among these tools, one stands out for its user-friendly interface and functionality. Healthcare professionals often report positive experiences with AI reliability for healthcare transcriptions, especially regarding its accuracy and time efficiency.
This tool captures audio and quickly converts it into written text, which aids in documenting patient interactions accurately. Its automatic speech recognition technology adapts to various accents and terminologies, enhancing its usability in diverse healthcare environments. Additionally, the ability to easily search and organize transcripts allows healthcare providers to efficiently retrieve information when needed.
However, it is crucial for users to regularly check the transcripts for errors. While technology has advanced greatly, occasional inaccuracies can be present. Understanding the strengths and limitations of this tool can ensure its effective integration into healthcare practices, thereby improving overall patient care.
- Google Cloud Speech-to-Text
The Google Cloud Speech-to-Text tool represents a significant advancement in the realm of AI reliability for healthcare transcriptions. It is designed to convert audio recordings into text with remarkable accuracy and efficiency. healthcare professionals can upload multiple files and receive transcriptions quickly, enabling them to focus more on patient care and less on administrative tasks. The user-friendly interface simplifies the entire process, allowing healthcare teams to extract valuable insights from their calls with ease.
One of the critical features of this tool is its bulk analysis capability. Users can transcribe multiple files simultaneously, streamlining workflows that require extensive documentation. Additionally, the platform offers templates tailored for specific needs, such as customer discovery or pain point analysis. These functionalities support AI reliability for healthcare transcriptions by ensuring that vital information is captured accurately and easily retrievable, fostering better communication and improved patient outcomes.
Criteria for Evaluating AI Tools in Healthcare
When assessing AI reliability for healthcare transcriptions, specific criteria are essential to ensure consistent and accurate results. Start by examining the technology's accuracy and precision, which directly impact patient data integrity. Moreover, ease of integration within existing healthcare systems is crucial, as seamless functionality enhances workflow efficiency for clinicians.
Another essential criterion is compliance with healthcare regulations, particularly data privacy and security standards. Trustworthiness also hinges on the tool’s performance in real-world scenarios, which should be validated through clinical trials and user feedback. Finally, the AI tool should exhibit adaptability to various medical dialects and terminologies, ensuring it can service diverse healthcare environments effectively. These evaluative factors collectively contribute to a comprehensive understanding of AI tools' potential in enhancing transcription reliability in healthcare settings.
Conclusion: Assessing AI Reliability for Healthcare Transcriptions
When assessing AI reliability for healthcare transcriptions, it is essential to consider accuracy and context. Healthcare calls often contain critical information that requires precise transcription, as even minor errors can have significant consequences. While many AI tools boast high accuracy rates, such as 80 to 90 percent, this still leaves room for improvement in error detection and context understanding. Thus, regular updates and adjustments are necessary to enhance transcription quality.
Furthermore, customization plays a vital role in ensuring that AI tools meet healthcare compliance standards. Feedback loops help refine AI algorithms based on user experiences and needs, directly impacting the overall effectiveness. As healthcare organizations increasingly rely on AI for transcriptions, ongoing evaluation and adaptation will be crucial in achieving trustworthy outcomes.