The rapid evolution of technology has fundamentally reshaped the landscape of quality assurance (QA). With businesses striving for efficiency and accuracy, the debate between traditional manual QA reviews and the adoption of AI-driven QA tools has gained unprecedented relevance. Understanding this QA methods comparison is essential for organizations aiming to maintain high-quality standards while balancing cost and resources.
In this section, we delve into the strengths and limitations of both approaches. Manual reviews emphasize human insight and personalized evaluation, whereas AI tools promise speed and scalability. As we explore the nuances of each method, we will highlight key factors influencing decision-making, including accuracy, cost-effectiveness, and implementation challenges. Data-driven insights will illuminate the current trends, helping organizations navigate this crucial choice in the modern era of QA.
Analyze qualitative data. At Scale.
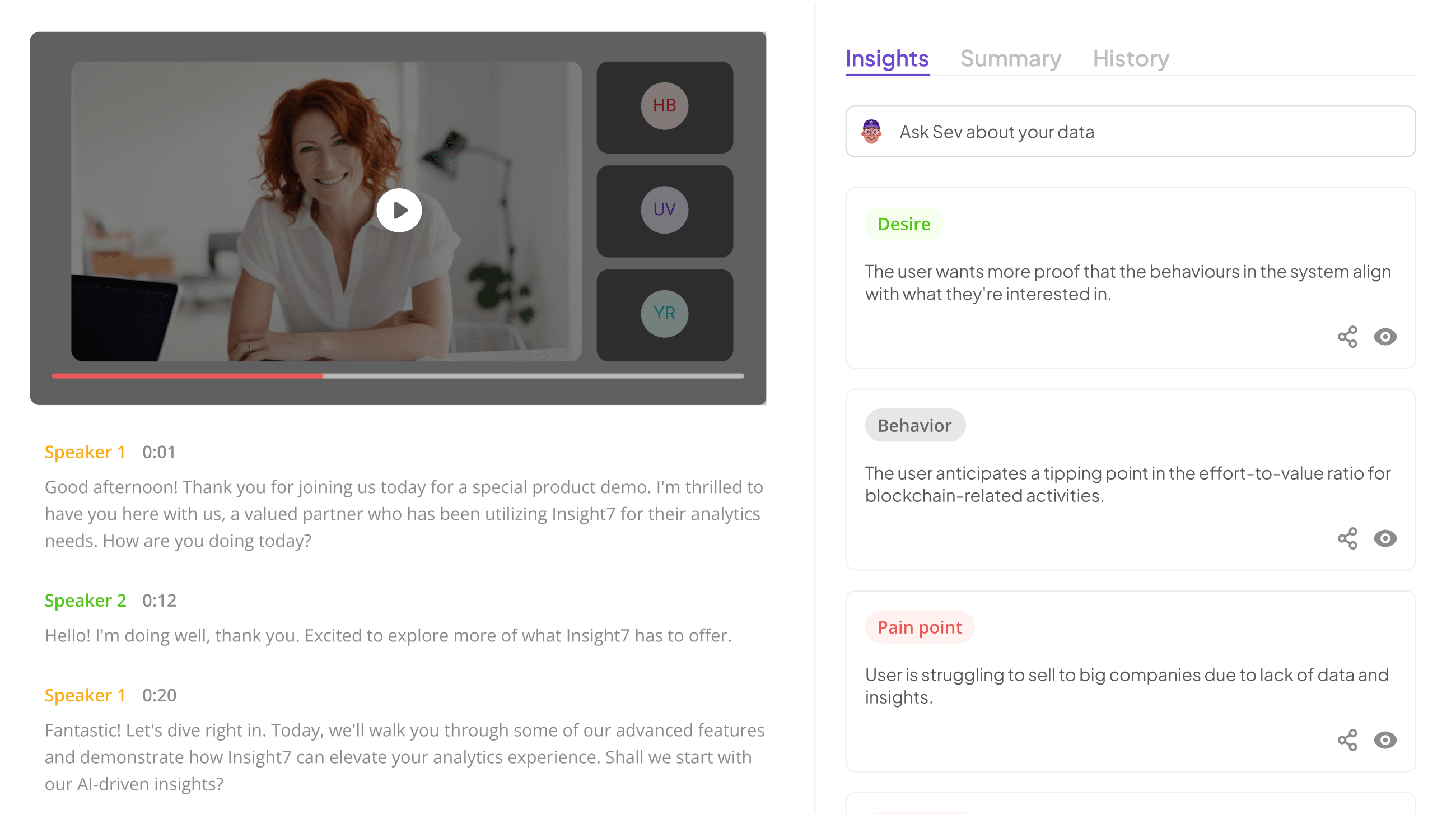
Understanding Manual QA Reviews
Manual QA reviews are a vital part of ensuring software quality and maintainability. They involve human testers thoroughly analyzing functionalities, user experience, and compliance with predefined criteria. This hands-on approach allows for nuanced feedback that automated systems may overlook, particularly regarding user experience and overall satisfaction.
When understanding manual QA reviews, it's essential to recognize their strengths. First, they provide direct insight into user interactions, identifying issues that may arise in real-world scenarios. Second, human testers can adapt their assessments based on context and relevancy, offering a level of flexibility that AI tools currently cannot match. Lastly, they foster collaboration across teams by discussing qualitative feedback, enhancing the overall product lifecycle. In contrast to AI-driven methods, manual QA remains a critical mechanism for maintaining software quality through comprehensive and context-aware evaluations.
The Traditional Approach: Manual QA Explained
In the realm of quality assurance, the traditional approach, known as Manual QA, relies heavily on human expertise. Manual QA typically involves testers meticulously reviewing product functionality and user experience through hands-on testing. This method serves as a foundational practice for ensuring that software meets specified requirements. Testers manually execute test cases, identify issues, and provide a detailed report, informing development teams of necessary improvements.
One significant aspect of Manual QA is the focus on context and user interaction. Human testers can understand nuances and identify unexpected behaviors that automated tools may overlook. This subjective insight is critical for applications requiring a high level of user engagement, as testers can assess aesthetics, usability, and emotional responses. The manual review process plays a pivotal role in the QA methods comparison. While it may seem time-consuming, its thoroughness can lead to higher-quality releases. This approach allows for more flexibility when addressing unforeseen issues, setting the stage for a comprehensive comparison with AI QA tools.
Key Advantages of Manual Review for QA Methods Comparison
Manual reviews in quality assurance offer significant advantages that set them apart from automated AI tools. First, human reviewers bring unique insights, identifying subtle nuances and contextual elements that AI may overlook. Their ability to interpret and understand the user experience ensures that critical feedback aligns with real-world scenarios. This level of understanding is pivotal in delivering quality results that meet user expectations.
Another key advantage lies in adaptability. Manual reviews allow for flexibility in evaluation criteria, enabling teams to customize assessments based on specific project requirements. This responsiveness creates a more tailored approach, enhancing overall product quality. Moreover, skilled human reviewers can collaborate and communicate effectively with stakeholders, fostering a shared understanding of quality objectives. These strengths highlight the importance of incorporating manual reviews in the QA methods comparison, ensuring a holistic evaluation process that balances both automation and human insight.
Extract insights from interviews, calls, surveys
and reviews for insights in minutes
Extract insights from interviews, calls, surveys and reviews for insights in minutes
Extract insights from interviews, calls, surveys and reviews for insights in minutes
Exploring AI QA Tools and Their Impact
AI QA tools are transforming the quality assurance landscape by delivering rapid feedback and enhanced accuracy in testing processes. With the increasing complexity of software applications, these tools allow teams to maintain high quality without the extensive time commitment traditionally associated with manual reviews. By automating tests, AI tools can identify bugs and functionality issues more quickly, giving developers insights that can inform immediate improvements.
The impact of AI QA tools can be measured through several key factors: speed, accuracy, cost-effectiveness, and scalability. First, the speed of AI tools facilitates faster release cycles, enabling teams to respond more swiftly to market demands. Second, their ability to analyze vast amounts of data enhances accuracy, minimizing the risk of human error. Third, the cost-effectiveness comes from reduced manual labor and associated expenses. Finally, scalability allows for handling larger projects or increased test cases without a proportional increase in resources. As the comparison between manual QA reviews and AI QA tools continues, the advantages of incorporating AI become increasingly evident.
AI Tools for QA Methods Comparison
AI tools are reshaping how we compare QA methods, enhancing efficiency and precision in the evaluation process. As organizations strive to improve their quality assurance structures, a detailed comparison of AI tools against traditional manual review methods becomes essential. This comparison reveals how AI-driven solutions can streamline tasks that would typically consume significant time and resources when done manually.
Firstly, automated QA tools can analyze data from calls and transcripts with remarkable speed, far surpassing human capabilities. While manual reviews rely on subjective insights, AI tools offer data-driven analysis, allowing for statistical comparisons and trend identification. Additionally, these tools adapt easily to various evaluation templates, catering to diverse compliance needs. In the landscape of QA methods comparison, it is evident that AI tools can significantly enhance both accuracy and scalability, thus prompting organizations to consider their integration into existing QA frameworks for optimal results.
insight7
When examining the landscape of QA methods, insights reveal important trends in both manual reviews and AI-driven tools. The data highlights a growing inclination towards automation for efficiency, scalability, and accuracy. However, it also emphasizes the irreplaceable value provided by human insights during manual QA reviews. The balance between these approaches is pivotal in determining overall effectiveness in quality assurance.
Moreover, companies adopting AI tools experience quicker turnaround times while also managing larger volumes of data. These tools can swiftly analyze customer feedback and make sense of insights that might take manual reviewers significantly longer to process. Conversely, manual QA reviews often excel in contextual interpretation of nuanced feedback, ensuring that subtle customer sentiments are not overlooked. Understanding these dynamics helps organizations create a strategic approach tailored to their specific needs and limitations in QA methods comparison.
Testim
Testim represents a significant advancement in the realm of automated testing, particularly for software quality assurance. Unlike traditional manual testing, it leverages AI to streamline the QA process, enhancing both speed and accuracy. With features such as intelligent test creation and automatic maintenance, Testim aids teams in achieving efficient test coverage while allowing them to focus on more complex, nuanced issues that require human oversight.
In the ongoing QA methods comparison, Testim stands out for its ability to quickly adapt to application changes. Whereas manual testers might take time to rewrite tests after updates, Testim's AI-driven approach minimizes this friction. As organizations strive to balance the advantages of both manual reviews and automated tools, understanding how Testim fits into this landscape can help teams optimize their QA strategies effectively. Embracing such innovative tools not only fosters productivity but also ensures a higher quality software release.
Applitools
AI-driven visual testing tools harness the power of automation to enhance quality assurance processes. One prominent capability is their ability to continuously monitor application changes, identifying potential issues before they affect end users. By automating visual comparisons across different browsers and devices, these tools significantly reduce the time needed for manual testing, ensuring a more efficient QA process.
Integrating automated visual testing into your QA methods offers several benefits. Firstly, it allows teams to focus on more strategic tasks rather than repetitive checks. Secondly, AI tools can quickly adapt to frequent changes in applications, maintaining high quality amidst evolving development cycles. This dynamic capability positions AI effectively alongside traditional manual reviews, enhancing the overall QA methods comparison and paving the way for greater agility within QA teams.
Selenium
Selenium stands out as a powerful tool in the realm of automated testing. It enables testing across various web browsers and platforms, ensuring compatibility and user experience. By allowing testers to write scripts in multiple programming languages, Selenium enhances flexibility and accessibility for QA teams. This agility contributes significantly to the QA methods comparison, showcasing the strengths of automation in delivering reliable software products.
Moreover, Selenium's integration capabilities with other testing frameworks, such as TestNG and JUnit, provide a comprehensive environment for managing test cases and reporting results. Teams using Selenium can execute tests in parallel, which accelerates the testing process. Given the increasing complexity of applications, this efficiency becomes crucial. Overall, Selenium not only streamlines the testing process but also plays a pivotal role in the ongoing discussion about the effectiveness of various QA methods. In this context, understanding how automation compares with traditional manual reviews will shape future testing strategies.
Advantages of AI QA for Efficiency and Scalability
AI Quality Assurance (QA) tools present significant advantages in enhancing efficiency and scalability compared to traditional manual QA reviews. First, by automating repetitive testing tasks, AI tools drastically reduce the time required for quality checks, allowing teams to focus on more complex, value-added activities. This results in faster deployment cycles and quicker iterations, which are essential in today’s fast-paced development environments.
Second, the scalability of AI QA tools means they can handle vast amounts of testing data and integrate seamlessly with other systems, adapting to various project sizes and complexities. This flexibility not only meets increasing demands but also ensures consistent quality across projects on a larger scale. The insights drawn from comprehensive data analyses enable teams to identify bottlenecks and performance issues effectively, contributing to continuous improvement in quality assurance processes. Thus, the shift to AI QA tools signifies a strategic move towards optimizing the QA methods comparison, ultimately driving better outcomes for organizations.
Data-Driven Insights: QA Methods Comparison Results
The analysis of data-driven insights reveals essential findings in the QA methods comparison between manual reviews and AI-driven tools. Our research indicates significant performance differences, with AI tools often delivering faster results while maintaining high accuracy. Numerical metrics from various case studies show that AI can reduce testing time by up to 60%, while manual reviews tend to be more labor-intensive, consuming more resources.
Cost-benefit analyses also illustrate disparities across industries. In sectors demanding rapid software releases, AI tools emerge as the preferred option, maximizing efficiency and cost-effectiveness. Conversely, industries where human insight and contextual understanding are paramount may still see value in manual QA approaches. Therefore, it's clear that the QA methods comparison must consider both technological capabilities and the nuanced needs of specific industries. Balancing these elements will guide stakeholders in making informed decisions about their QA strategies.
Key Findings from Industry Data
Key findings from industry data regarding manual QA reviews versus AI QA tools reveal significant insights into their effectiveness. First, performance metrics indicate that while manual reviews offer thorough attention to detail, they often fall short in speed compared to AI tools. AI QA tools excel in processing vast amounts of data rapidly, providing comprehensive coverage and minimizing human error.
Moreover, the cost-benefit analysis shows various trends across industries. Manual QA methods, while traditionally cost-effective for small projects, become increasingly expensive when scaled. In contrast, AI tools, although initially requiring higher investment, demonstrate long-term savings through automation and efficiency gains. This QA methods comparison underscores the importance of selecting the right approach based on project size, complexity, and resource availability, ultimately informing best practices in quality assurance.
Performance Metrics: Manual QA vs. AI QA
Performance metrics are essential for evaluating the effectiveness of different quality assurance methods. In comparing Manual QA and AI QA, data reveals significant differences in their performance outcomes. Manual reviewers often provide nuanced insights typical of human understanding. They can identify subjective issues that an algorithm might overlook. However, this approach generally requires more time and resources, leading to longer testing cycles.
Conversely, AI QA tools excel at efficiency and scalability. They can process vast amounts of data quickly and consistently, delivering reports in real-time. This enables organizations to identify and rectify issues faster, ultimately enhancing productivity. The critical takeaway from our QA methods comparison is to balance the strengths of both approaches. While manual reviews add qualitative depth, AI tools offer quantitative speed, suggesting that a hybrid strategy may yield superior results for quality assurance efforts.
Cost-Benefit Analysis in Different Industries
In evaluating the various costs and benefits of QA methods comparison, different industries demonstrate distinct trends and outcomes. Manual QA reviews often incur higher labor costs due to the extensive time detail-oriented tasks demand. In contrast, AI QA tools can initially involve hefty investment but contribute to significant long-term savings through automation. This comparison reveals that while manual reviews excel in nuanced understanding, AI tools provide unmatched efficiency, especially in large-scale environments.
Industries such as software development benefit substantially from AI capabilities, enhancing productivity without compromising quality. Conversely, sectors like financial services may still prefer manual reviews for their depth of regulatory compliance and decision-making. Ultimately, the cost-benefit analysis in various industries illuminates the unique strengths of each QA method, guiding organizations to select optimal strategies that align with their specific operational needs and objectives.
Conclusion: Finding the Right Balance in QA Methods Comparison
Finding the right balance in QA methods comparison is critical for organizations looking to improve their quality assurance processes. Both manual QA reviews and AI QA tools offer unique advantages that can contribute significantly to overall efficiency. While manual reviews provide nuanced insights into user experiences, AI tools enhance scalability and speed, allowing for a more comprehensive analysis of data.
To optimize quality assurance outcomes, organizations must assess their specific needs and capabilities. By combining the strengths of manual and AI-driven methods, companies can create a harmonized approach that fosters accuracy and efficiency. Ultimately, striking this balance will lead to more reliable insights and improved product quality in the long run.
Analyze qualitative data. At Scale.
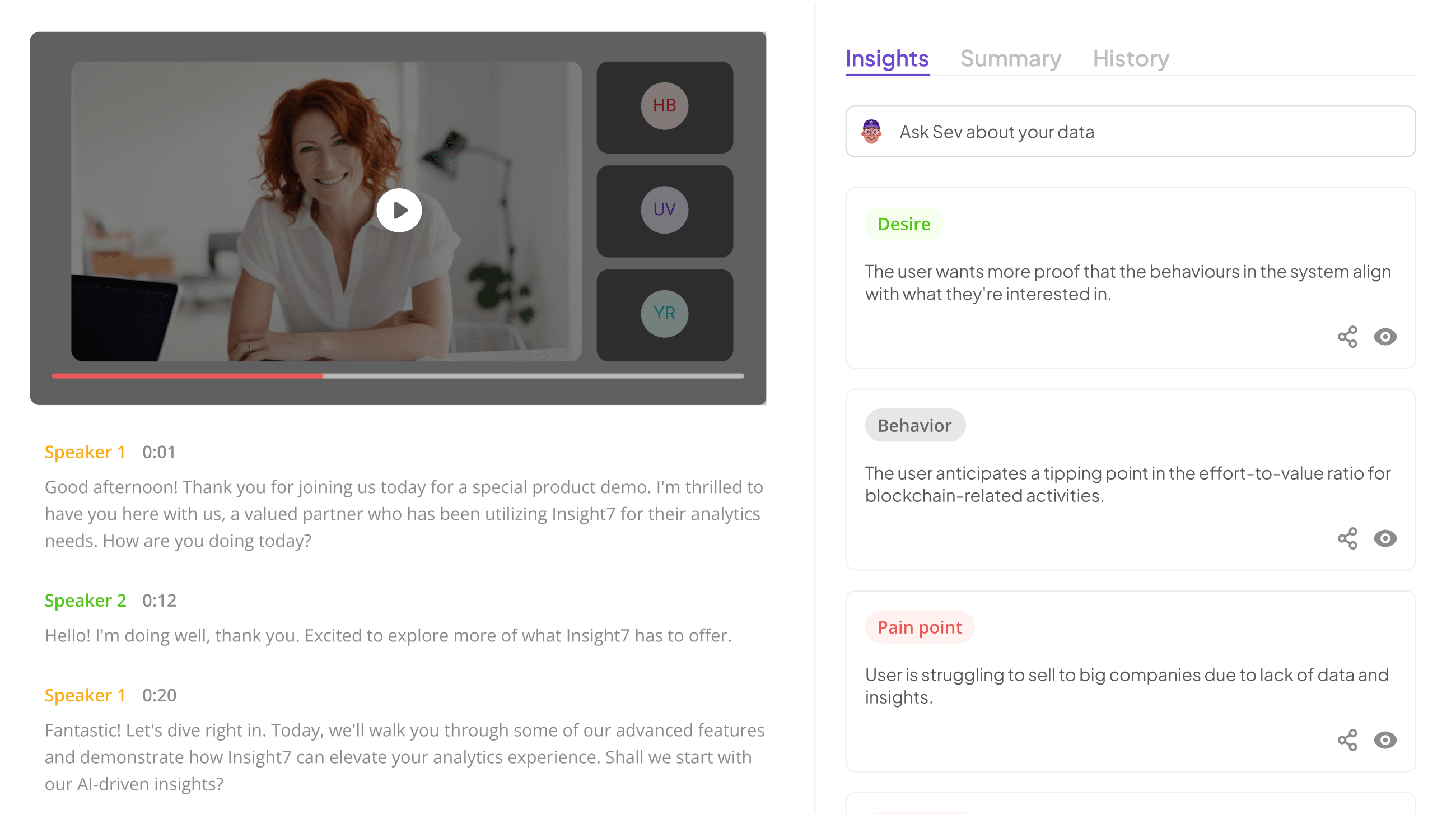