In an age where data drives decisions, the integrity of the QA data is paramount for accurate audits. QA data integrity serves as the foundation for maintaining trust and reliability in the evaluation processes that organizations rely on. As a quality assurance professional, understanding the nuances of data collection and analysis is critical. Missteps can result not only in data inaccuracies but also in biased outcomes that can skew interpretations.
By ensuring QA data integrity, organizations can foster a culture of transparency and accountability. It's essential to identify common sources of inaccuracy and bias that may emerge during the data lifecycle. This proactive approach is key to conducting effective audits, ultimately leading to better decision-making and enhanced operational efficiencies. A robust understanding of QA data integrity ensures that audits are accurate, reliable, and free of bias.
Analyze qualitative data. At Scale.
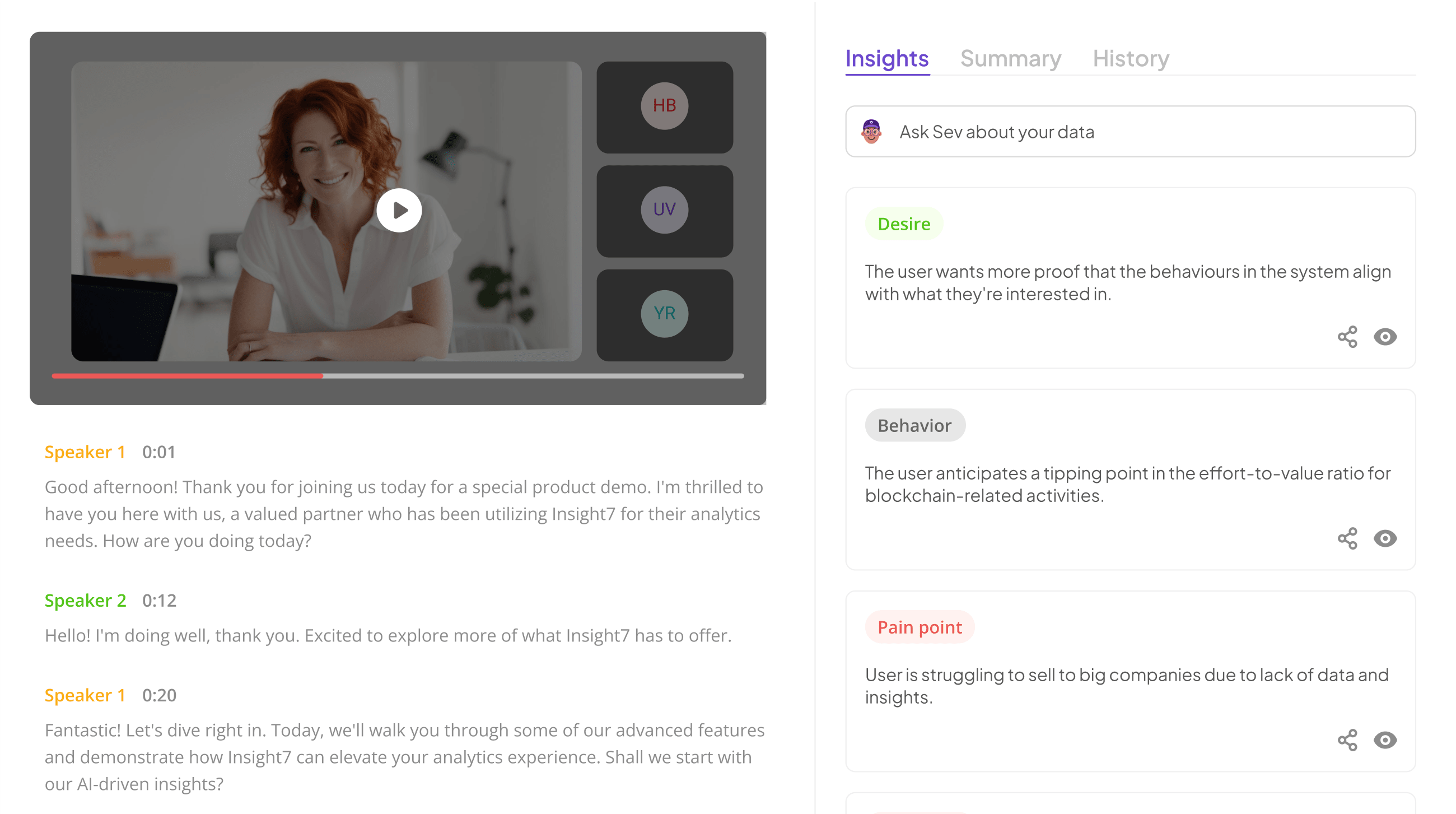
Understanding the Principles of QA Data Integrity
Quality Assurance (QA) Data Integrity is fundamental to any successful auditing process. Understanding this principle involves recognizing the importance of reliable data in decision-making and compliance. Without QA Data Integrity, organizations risk drawing incorrect conclusions based on flawed information, which could lead to costly errors and misinterpretations that hinder efficiency.
There are several key aspects to consider in maintaining QA Data Integrity. Firstly, establishing clear data collection guidelines ensures consistency and accuracy. Next, continuous monitoring is vital for identifying any discrepancies or potential sources of bias that may arise over time. Lastly, educating all team members about data integrity practices fosters a culture of accountability, enabling everyone to play a role in preserving data quality. By adhering to these principles, organizations can significantly enhance their auditing processes, ensuring that their data is not only accurate but also free from bias.
Defining QA Data Integrity
QA Data Integrity, in the context of auditing, refers to the accuracy, consistency, and reliability of data throughout its lifecycle. Ensuring that QA data remains integral is crucial for making sound decisions based on that data. Integrity demands rigorous processes to validate and verify data inputs, maintain their authenticity, and preserve their quality over time. Without this assurance, organizational decisions may rely on flawed insights, ultimately affecting performance and trustworthiness.
Moreover, QA Data Integrity plays a significant role in identifying and mitigating biases that can skew results. By establishing clear definitions and guidelines for maintaining integrity, organizations can recognize the importance of data verification. This diligence is essential for enhancing transparency and upholding credibility across all QA processes. Effectively managing these aspects ensures that data remains a reliable foundation for strategic initiatives and contributes positively to organizational goals.
- Clarify what QA Data Integrity means in the context of auditing.
QA Data Integrity refers to the accuracy, completeness, and consistency of data used in auditing processes. In the context of auditing, it embodies the principles that ensure data remains trustworthy throughout its lifecycle. This reliability is critical in making informed decisions, as compromised data can lead to erroneous conclusions and misinformed strategies. Establishing QA Data Integrity means implementing strict protocols to safeguard against data loss, manipulation, or misinterpretation during collection and analysis.
Moreover, ensuring QA Data Integrity involves identifying potential sources of erosion within data sets. Auditors must consider human error, technological malfunctions, or even process inefficiencies that can introduce inaccuracies or biases. By employing systematic checks and balances, organizations can strengthen their auditing framework, leading to more robust outcomes. Ultimately, committing to QA Data Integrity enhances the overall effectiveness of audits and fosters greater trust in the insights generated from the data.
- Explore its significance in maintaining the reliability of data.
Maintaining the reliability of data is crucial for effective decision-making and ensuring valid outcomes. One primary factor contributing to this reliability is QA Data Integrity. By ensuring all collected data is accurate and unbiased, organizations can trust their analyses and results more thoroughly. This leads to sound strategies and informed decisions based on solid foundations.
To explore its significance further, there are several key aspects to consider. First, quality assurance practices help identify and correct inaccuracies, which can arise from data collection methods or inherent biases. Second, consistent auditing demonstrates a commitment to data reliability. Regular audits foster confidence among stakeholders about the organization's commitment to integrity. Third, utilizing advanced tools and techniques enhances data verification processes, ensuring that the data remains free from manipulation or external influences. Together, these elements contribute to a robust framework for auditing QA data, which ultimately upholds the integrity of the data used in decision-making processes.
Identifying Common Sources of Inaccuracy and Bias
QA data integrity is essential for identifying and addressing inaccuracies and biases during audits. Common pitfalls in data collection and analysis can arise from misinterpretations, human error, and sampling biases. These issues not only diminish the reliability of results, but they can also lead to misguided decisions based on flawed information. It's crucial to be aware of these pitfalls to maintain data integrity and protect against negative outcomes.
Inadvertent biases often stem from flawed survey designs or leading questions, which can skew results. Systemic biases, on the other hand, may arise from long-standing practices or organizational cultures that overlook diverse perspectives. Regularly reviewing and refining data collection methods can help mitigate these biases. Recognizing these common sources of inaccuracy and bias will set a firm foundation for developing a robust auditing strategy, ensuring that the insights derived from the data remain accurate and trustworthy.
- Discuss typical pitfalls encountered in QA data collection and analysis.
In the realm of QA data collection and analysis, several common pitfalls can undermine QA data integrity and compromise the reliability of the findings. One major issue is inadequate training for data collectors, which can lead to inconsistent data entry and a lack of understanding of the metrics being measured. This can introduce errors that distort results and mislead stakeholders about quality outcomes.
Furthermore, limited data oversight can result in unchecked biases stemming from the data sources themselves. For example, if data is collected from a singular demographic, it may not accurately represent the broader population's experiences, leading to skewed conclusions. Additionally, poor data management practices can foster incomplete datasets, further exacerbating inaccuracies. Awareness of these pitfalls is crucial for effective summative analysis, ensuring that QA data remains robust and trustworthy.
- Explore both inadvertent and systemic biases.
Understanding inadvertent and systemic biases in QA data is crucial for maintaining data integrity. Inadvertent biases often occur unintentionally, stemming from factors like human error or unclear data collection methods. For instance, an auditor might unconsciously favor certain data points, skewing analysis results. This can lead to flawed conclusions that impact decision-making.
On the other hand, systemic biases arise from established processes and institutional norms, affecting the entire data collection and analysis cycle. These biases can include standardized surveys that inadvertently favor specific demographics, leading to unbalanced data representation. Addressing both types of biases is vital for a thorough QA audit, ensuring that the final results reflect true data integrity. By critically examining and adjusting these biases, organizations can enhance their audit processes and foster more accurate and reliable outcomes.
Extract insights from interviews, calls, surveys and reviews for insights in minutes
Conducting an Effective QA Audit: Ensuring Data Accuracy and Neutrality
Conducting an effective QA audit requires a systematic approach to ensure data accuracy and neutrality. The first step is preparation, where you establish clear objectives for the audit. Determine what elements of the data need scrutiny and gather the necessary resources, such as documentation on existing criteria and tools for analysis. By developing a well-defined framework, you enhance the chance of achieving QA data integrity during the audit process.
Next, analyze the data critically to uphold its integrity. This involves cross-checking the information against established benchmarks and scrutinizing it for inconsistencies. Utilize tools that facilitate the identification of errors, as well as methodologies designed to detect potential biases. Implementing bias detection strategies is equally essential. This could include using specialized software that highlights discrepancies and influences that may affect data neutrality. By following these steps, you position your audit to accurately reflect the quality and reliability of your data.
Step-by-Step Guide to Auditing QA Data
Auditing QA data involves a systematic approach to ensure data integrity and detect potential biases. The first step, Preparation for the Audit, is crucial. Begin by defining clear objectives, understanding what you aim to achieve. Gather the necessary resources, including access to data sets and analytical tools, to facilitate a thorough examination. This foundational stage sets the tone for a successful audit, allowing you to focus on the specific attributes that impact data integrity.
Next, proceed to Analyzing Data for Integrity. Utilize various methods, such as cross-referencing multiple data sources, to verify the accuracy of collected information. Look for discrepancies that might indicate errors, whether from human error or technical faults. Finally, in the step of Implementing Bias Detection Strategies, apply established tools and methodologies that help recognize and address biases in your data sets. By systematically conducting these steps, you can effectively uphold the integrity of QA data within your auditing processes.
- Step 1: Preparation for the Audit
Preparing for an audit is crucial for ensuring QA Data Integrity, which lays the foundation for accurate and unbiased outcomes. Start by clearly defining the audit objectivesโwhat you aim to achieve and how it aligns with your compliance requirements. Gathering necessary resources, such as team members and relevant data, sets the stage for a successful audit. Ensure that everyone involved understands their roles and responsibilities, creating a cohesive effort toward accurate data assessment.
Next, familiarize yourself with the tools you will use during the audit. This includes software solutions that help organize and analyze data efficiently. With thousands of records to sift through, establishing a systematic approach to select samples will help focus your analysis. Additionally, consider potential sources of bias in your data and prepare a strategy to address them. By laying out these initial steps, you create a roadmap that enhances the integrity of your QA data audit and bolsters your commitment to accuracy.
- Detail the initial steps required to set clear objectives and gather necessary resources.
Setting clear objectives is crucial when initiating a QA data audit, as it helps define your goals. First, identify the specific outcomes you wish to achieve, such as enhanced data accuracy or reduced bias. Once your objectives are established, gather the necessary resources, including data integrity tools, subject matter experts, and relevant documentation. These resources will provide the foundation for conducting a thorough audit, ensuring you have everything required to assess and enhance QA data integrity effectively.
Next, develop a comprehensive checklist to guide your audit process. This should include a timeline, key performance indicators, and any compliance standards applicable to your work. By systematically organizing your approach, you can streamline the auditing process and ensure that all areas are covered. Remember, the initial steps lay the groundwork for successful audits, as they determine how effectively you can evaluate data integrity and address potential biases inherent in your QA practices.
- Step 2: Analyzing Data for Integrity
In this step, the focus will be on analyzing QA data integrity. This process is essential for identifying inconsistencies and ensuring the accuracy of collected information. Begin by systematically reviewing the data entries and looking for anomalies. Utilize processes such as cross-referencing data sets against established benchmarks to verify each entryโs reliability. Create a record of any discrepancies to investigate further.
A standardized approach is crucial. First, categorize data into manageable sections for analysis. This will help in isolating specific trends while reducing the complexity of the review process. Next, consider implementing automated tools designed to flag irregularities. A thorough data integrity analysis not only enhances accuracy but also bolsters the trustworthiness of future findings.
In summary, analyzing QA data integrity is vital to establishing a strong foundation for your audits. By systematically addressing inaccuracies and biases, you create a more reliable and insightful data landscape.
- Explore methods to cross-check data accuracy and detect errors.
To ensure QA Data Integrity, it is vital to utilize systematic methods for cross-checking data accuracy and detecting errors effectively. One foundational approach is to establish a baseline for comparison by using trusted datasets or historical records. By comparing current data against these reliable sources, discrepancies can be quickly identified and addressed, thus enhancing overall data validity.
Another effective method involves employing data validation techniques. This includes automatically checking for outliers or entries that violate predefined criteria. Additionally, implementing peer reviews can promote collaborative scrutiny of data, enabling multiple perspectives to catch potential inaccuracies. Regular audits of data entry processes also contribute to minimizing human errors, further solidifying data integrity. By integrating these strategies, organizations can create a more robust framework for maintaining high standards of accuracy and reliability in their QA data.
- Step 3: Implementing Bias Detection Strategies
To ensure that QA Data Integrity is maintained, implementing effective bias detection strategies is essential. In this phase of the audit, various tools and techniques can be employed to identify biases affecting data quality. Start by utilizing statistical methods to analyze datasets for trends that may reveal inconsistencies or biases. For example, applying tests like Chi-squared can uncover significant discrepancies, enabling auditors to address underlying issues.
Next, focus on qualitative analysis through peer reviews and expert assessments. Engaging diverse teams for insight can expose biases that numerical data may not fully represent. This collaborative approach not only enhances the accuracy of findings but also nurtures a culture of awareness regarding bias within teams. Employing these strategies ensures robust QA Data Integrity, ultimately contributing to more precise analytics and informed decision-making across various domains.
- Discuss tools and methodologies to recognize and mitigate bias in data sets.
Recognizing and mitigating bias in data sets is crucial for maintaining QA Data Integrity. Employing tools such as fairness measurement algorithms can help identify both direct and indirect biases present in datasets. Techniques like data augmentation can address disparities by ensuring more equitable representations in samples, which in turn supports diverse perspectives.
Furthermore, methodologies such as stratified sampling minimize bias by ensuring that various subgroups are adequately represented. Automated bias detection frameworks can also analyze patterns within the data to highlight discrepancies, prompting further investigation where necessary. Regular audits and user feedback loops contribute to continuously refining these strategies, ensuring that identified bias is addressed promptly. By integrating such tools and methodologies, organizations can enhance their QA processes, fostering a culture of accuracy and inclusivity in data utilization.
Tools for Enhancing QA Data Accuracy and Bias Detection
In today's data-driven environment, maintaining QA data integrity is vital for effective auditing. Various tools enhance the accuracy of QA data while also identifying biases that may distort insights. Effective technologies allow teams to analyze larger datasets efficiently, revealing hidden patterns and inconsistencies. Such tools empower users to visualize data interactively, enabling them to discern both positive and negative feedback efficiently.
Several essential tools stand out in their ability to improve QA audits. For instance, Tool B excels in checking data accuracy, helping to uncover discrepancies between expected and actual data. Another notable option, Tool C, focuses on bias detection, identifying systemic issues that can skew results. Lastly, Tool D demonstrates its effectiveness in conducting comprehensive QA audits, ensuring that data remains reliable and valid over time. By leveraging these tools, organizations can enhance their QA data integrity and significantly improve the reliability of their insights.
- insight7: Overview of its functionalities in improving QA audits.
The functionalities of Insight7 are pivotal in enhancing QA audits, fundamentally bolstering QA Data Integrity. By offering tools that efficiently record and transcribe conversations, teams can analyze data at scale. This facilitates the identification of themes and specific quotes while also enabling users to create automated reports. The straightforward interface allows individuals, regardless of expertise, to engage with the tool effectively.
Furthermore, Insight7 provides customizable evaluation templates tailored for various quality assurance scenarios, such as compliance and customer engagement. The platform allows users to benchmark transcripts against predefined criteria, ensuring thorough assessments. Through this structured approach, organizations can systematically evaluate their QA processes, identify gaps, and refine their strategies. Overall, leveraging these functionalities significantly contributes to maintaining data accuracy and eliminating biases in QA audits, ultimately fostering trust in the data and supporting informed decision-making.
- Other Essential Tools:
Beyond standard tools, several essential resources enhance the assurance of QA data integrity. These tools support the meticulous process of auditing, enabling more accurate assessments and unbiased outcomes. Utilizing specialized software and methodologies can streamline the auditing process, making it efficient and effective.
One valuable addition is Data Visualization Tools. These empower teams to illustrate data trends clearly, making discrepancies immediately noticeable. Meanwhile, Statistical Analysis Software allows deeper dives into data sets, identifying anomalies and potential biases in a supportive environment. Additionally, incorporating Machine Learning Algorithms can automate bias detection, offering predictive insights about the quality of the data.
Implementing these resources collectively fosters an environment where QA data integrity is prioritized. As biases and inaccuracies are proactively addressed, organizations can trust their audit processes and make more informed decisions based on reliable data.
- Tool B: Examine its features pertinent to data accuracy.
In exploring Tool B for QA data integrity, we find a sophisticated platform designed to enhance data accuracy through various features. The tool allows users to visually assess data by categorizing insights into positive and negative feedback. This visualization enables auditors to quickly identify patterns and anomalies within the dataset, facilitating targeted investigations. By comparing data across different locations or sources, users can spot inconsistencies that may indicate potential biases or inaccuracies.
Furthermore, Tool B incorporates advanced querying capabilities, similar to conversational AI models. This feature empowers users to pose specific questions about dataset characteristics and retrieve precise responses, promoting deeper analytical insights. Such comprehensive analysis not only streamlines the auditing process but also reinforces data integrity by ensuring that all relevant factors are considered. Overall, Tool B serves as a crucial asset in conducting effective QA audits, driving towards greater accuracy and reliability in data management.
- Tool C: Highlight its capabilities in bias detection.
Tool C offers advanced capability in bias detection, playing a crucial role in ensuring QA data integrity. It employs sophisticated algorithms to analyze datasets, identifying potential biases that might lead to skewed results. By examining various data variables, Tool C can highlight trends and patterns indicative of systemic bias. This approach allows teams to understand not just where inaccuracies may exist, but also the underlying causes.
Furthermore, the tool integrates seamlessly with existing data workflows. Users can implement its functionality to run preliminary audits, which can help flush out irregularities before more in-depth analysis is conducted. Tool C also enables comparative evaluations across different datasets, illuminating discrepancies that may arise from biased data collection methods. By utilizing these capabilities, organizations can maintain rigorous standards for data accuracy and neutrality, ultimately supporting informed decision-making.
- Tool D: Review its effectiveness in comprehensive QA audits.
Gaining insights into the effectiveness of Tool D in comprehensive QA audits is essential for ensuring robust QA Data Integrity. This tool enhances the auditing process by allowing evaluators to analyze data efficiently and reliably. With the ability to customize evaluation templates, users can align the tool's assessments with specific criteria, making it adaptable for various QA needs and ensuring a thorough examination of calls against pre-defined standards.
Tool D facilitates the identification of inaccuracies in data by offering clear tracking of individual metrics. Users can quickly benchmark against established compliance and quality assurance criteria. The tool's effective reporting features enable stakeholders to uncover potential biases within datasets and rectify them, thereby bolstering overall accuracy. Regular use of Tool D not only enhances the immediate audit process but also contributes to a culture of continuous improvement in QA Data Integrity.
Conclusion: Upholding QA Data Integrity in Continuous Auditing
Maintaining QA Data Integrity is vital for ensuring the reliability and credibility of audits. Continuous auditing practices necessitate a steadfast commitment to accurate and unbiased data. By regularly validating data and employing structured methodologies, organizations can effectively capture and review metrics, ensuring compliance with established standards.
As auditing evolves, embracing technology and innovative tools is crucial. Implementing automated systems can streamline data checks, enabling quicker identification of issues. Ultimately, prioritizing QA Data Integrity not only safeguards the audit process but also enhances decision-making and drives trust across the organization. Continuous refinement and vigilance are necessary to sustain accurate, bias-free data that supports informed insights.