AI-Powered KPI Forecasting is reshaping the way businesses anticipate and manage operational needs. As market dynamics evolve, traditional forecasting methods often fall short in adapting to rapidly changing environments. By harnessing the power of artificial intelligence, organizations can leverage nuanced data signals to drive more precise forecasting, improving key performance indicators like Forecast Accuracy, Volume Variance, and SLA Risk Probability.
The integration of AI into KPI forecasting transforms the way insights are derived and decisions are made. With powerful tools at their disposal, companies can predict shifts in demand and resource allocation more effectively. This foundational shift not only streamlines operations but also enhances customer satisfaction through capabilities like Call Deflection Modeling and Channel Surge Readiness. Embracing AI in forecasting will position organizations to proactively navigate future challenges while optimizing their performance metrics.
Analyze & Evaluate Calls. At Scale.
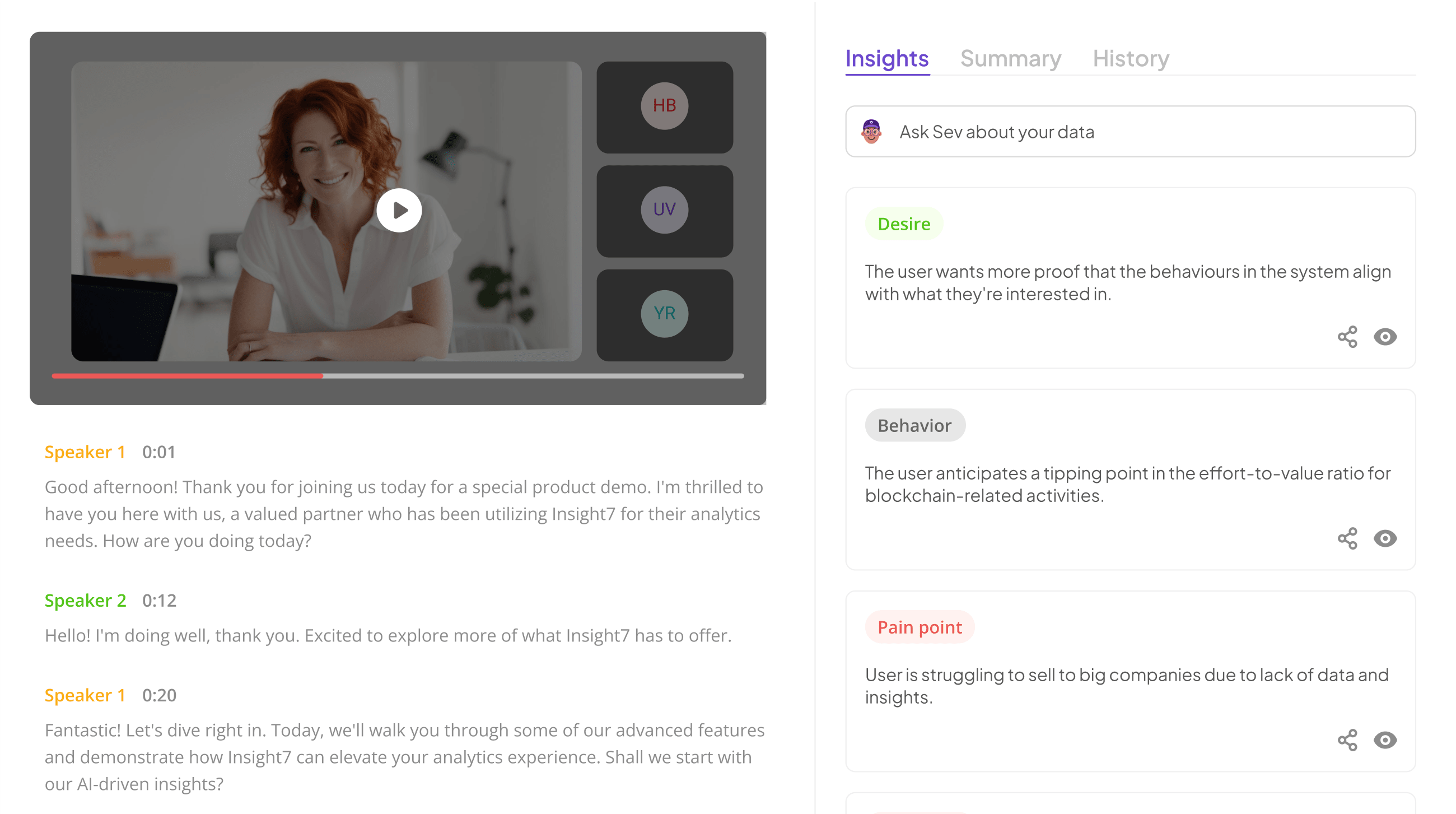
Discover how AI data signals are revolutionizing the way we forecast KPIs such as Forecast Accuracy, Volume Variance, SLA Risk Probability, and more. These powerful tools enable organizations to anticipate needs and allocate resources more efficiently.
AI-Powered KPI Forecasting is redefining how we understand and predict key performance indicators (KPIs). By harnessing AI data signals, organizations can not only measure Forecast Accuracy and Volume Variance but also assess SLA Risk Probability with unprecedented precision. This revolutionary approach allows for proactive resource allocation, enabling better strategic planning amidst fluctuating demands.
In this evolving landscape, the integration of advanced algorithms facilitates the forecasting of numerous KPIs. For instance, Agent Load Prediction and Occupancy Forecast help organizations anticipate workforce requirements based on predicted customer interactions. Furthermore, AI enhances insights into Intraday Deviation and Shrinkage Impact Index, ensuring that businesses stay responsive to real-time changes. The culmination of these factors results in improved Call Deflection Modeling and Channel Surge Readiness, empowering organizations to navigate uncertainties effectively and optimize operational efficiency.
Navigating the Landscape of AI-Powered KPI Forecasting
AI-Powered KPI Forecasting combines advanced data analytics with key performance indicators to enhance forecasting capabilities significantly. Understanding how to navigate this innovative approach begins with recognizing the critical KPIs transformed by AI. For instance, Forecast Accuracy % improves dramatically as AI analyzes historical data, enabling organizations to predict outcomes with unmatched precision.
Key areas such as Volume Variance and SLA Risk Probability also benefit from AI insights, allowing for strategic resource allocation and preparedness. Another fascinating advancement is Agent Load Prediction, which helps predict staffing needs to match expected call volumes. Understanding Occupancy Forecast, Intraday Deviation, Shrinkage Impact Index, and Channel Surge Readiness equips organizations to adapt to fluctuations in demand. Call Deflection Modeling further demonstrates how AI can optimize customer interactions, enhancing overall satisfaction. Embracing these AI-enhanced KPIs is essential for staying proactive in a rapidly evolving landscape.
Understanding the Key AI-Powered Forecasting KPIs
AI-Powered KPI Forecasting has dramatically transformed how organizations measure and predict performance indicators essential for operational success. Understanding the key KPIs in this realm is crucial for harnessing the true potential of AI data signals. Among these, metrics such as Forecast Accuracy %, Volume Variance, and SLA Risk Probability stand out as vital components that allow businesses to anticipate changes and allocate resources effectively.
The integration of AI into forecasting enhances the ability to predict agent load and occupancy while assessing intraday deviations. Additionally, KPIs like the Shrinkage Impact Index and Call Deflection Modeling offer deeper insights into operational efficiency. In the face of unexpected activity surges, understanding Channel Surge Readiness can be the differentiator between effective management and chaos. By meticulously analyzing these AI-powered KPIs, organizations can achieve unparalleled accuracy and preparedness, ultimately leading to wiser decision-making and better outcomes.
Explore the critical KPIs that have been transformed by AI data signals, providing greater insight and accuracy in predictions.
AI has significantly enhanced the critical KPIs used in forecasting, enabling organizations to gain insights that were previously unimaginable. The advent of AI data signals has transformed metrics such as Forecast Accuracy %, Volume Variance, and SLA Risk Probability. These advancements allow businesses to anticipate shifts in demand and resource needs more effectively, ensuring they can respond to customer requirements swiftly and accurately.
Moreover, AI empowers organizations by improving predictions around Agent Load, Occupancy Forecasts, and Shrinkage Impact Index. The ability to model Call Deflection and prepare for Channel Surge Readiness greatly enhances operational efficiency. Companies can now optimize their resources through advanced analytics, ultimately leading to better service delivery and increased satisfaction. Embracing AI-powered KPI forecasting is essential for staying competitive and informed in a rapidly evolving landscape.
Forecast Accuracy %: Measuring Precision
Forecast Accuracy % is vital in determining how closely actual outcomes align with predicted ones. Utilizing AI-Powered KPI Forecasting, organizations can significantly enhance their prediction accuracy. This precise measurement not only streamlines decision-making processes but also aids in resource allocation and strategy formulation. Leveraging advanced data analytics tools, decision-makers can translate complex datasets into actionable insights, transforming the forecasting landscape.
To effectively measure Forecast Accuracy %, consider the following key steps:
- Data Quality Assurance: Ensure data integrity and consistency to improve prediction foundations.
- Historical Analysis: Leverage historical data to identify patterns that can enhance future forecasts.
- Continuous Monitoring: Regularly assess forecast outcomes against real results for ongoing refinement.
- Model Adjustment: Adapt forecasting models based on performance feedback and emerging trends.
By following these steps, organizations can harness AI-driven insights to achieve a more reliable and precise forecasting process. This proactive approach ultimately empowers business strategies and contributes to operational excellence.
Learn how AI data signals enhance the precision of forecasts, allowing organizations to make more reliable predictions.
AI-powered KPI forecasting is revolutionizing how organizations understand and predict future trends. By harnessing the power of AI data signals, organizations can achieve enhanced accuracy in their forecasts. This technological advancement allows for intricate analysis of key performance indicators, such as Forecast Accuracy %, Volume Variance, and SLA Risk Probability. The result is a more streamlined approach to decision-making and resource allocation, ensuring organizations can effectively meet their operational demands.
AI dramatically improves the quality of predictions by analyzing vast amounts of data to identify patterns and trends. For instance, accurate modeling of Agent Load Prediction and Occupancy Forecast can help adjust staffing needs in real-time. Furthermore, insights derived from Intraday Deviation and Shrinkage Impact Index can aid in anticipating fluctuations and mitigating risks. As organizations embrace these powerful AI data signals, they unlock the potential for more reliable and informed forecasting solutions, ultimately intersecting technology and strategic planning for optimized performance outcomes.
Volume Variance and SLA Risk Probability
Volume variance refers to the discrepancies between expected and actual call volumes, which can significantly impact workforce management and service delivery. AI-powered KPI forecasting enhances the ability to predict these fluctuations accurately, allowing organizations to better prepare for changes in demand. By analyzing historical data and patterns, AI can identify trends, helping businesses allocate resources more effectively and mitigate potential risks.
Simultaneously, SLA risk probability analysis is crucial for maintaining service standards. AI tools assess the likelihood that service level agreements will be violated based on current performance metrics and predicted volumes. This proactive approach enables organizations to address potential shortfalls before they affect customer satisfaction. By integrating these AI insights, businesses can enhance their operational readiness, ensuring they meet service expectations and ultimately drive improved customer outcomes.
Delve into how AI aids in predicting volume fluctuations and assessing the probability of SLA risks, ensuring better preparedness.
AI enhances the accuracy of predicting volume fluctuations and assessing the probability of SLA risks, significantly improving preparedness. By analyzing historical data and current trends, AI systems can identify patterns that indicate incoming volumes, allowing organizations to allocate resources efficiently. This capability not only reduces uncertainty in call handling but also supports effective agent load predictions and occupancy forecasting, ensuring that staff and resources are optimally utilized.
Moreover, the assessment of SLA risk probability through AI models provides crucial insights for operational planning. Organizations can proactively address potential SLA violations, minimizing the risk of non-compliance. By forecasting channel surge readiness, businesses can equip themselves to handle unexpected spikes in demand. Combined, these AI-powered tools create a robust framework for efficient operations, mitigating risks while enhancing customer satisfaction and operational efficiency.
Tools Enhancing AI-Powered KPI Forecasting
While AI-Powered KPI Forecasting brings significant advancements, the effectiveness of these forecasting models hinges on the tools employed for implementation and analysis. Various innovative tools play critical roles in enhancing the accuracy and reliability of KPIs like Forecast Accuracy %, Volume Variance, and SLA Risk Probability. These tools effortlessly integrate AI data signals to produce actionable insights, which in turn empower organizations to make informed decisions.
A few prominent tools that enhance AI-Powered KPI Forecasting include solutions that specialize in predicting customer demand and managing resource allocation. For instance, certain platforms focus on accurately predicting agent load needs and optimizing occupancy levels, which helps maintain operational efficiency. Additional tools assess shrinkage impacts and intraday deviations, while others specialize in preparing systems for unexpected spikes in activity, ensuring optimal channel surge readiness. By leveraging these advanced tools, organizations can transform their forecasting strategies, achieving greater precision and improved performance in meeting business objectives.
Extract insights from interviews, calls, surveys and reviews for insights in minutes
Top AI Tools for KPI Forecasting
Incorporating AI tools into KPI forecasting dramatically enhances decision-making capabilities across organizations. By leveraging these tools, businesses can optimize key performance indicators such as forecast accuracy, volume variance, and SLA risk probability. These AI-powered platforms provide predictive insights that enable companies to allocate resources effectively and respond to customer needs more swiftly.
The most effective AI tools for KPI forecasting utilize advanced algorithms to interpret large datasets, identify trends, and enhance forecasting precision. For instance, tools specialized in agent load prediction can accurately forecast staffing needs, while those focused on occupancy forecasting provide vital insights into resource allocation during peak times. Furthermore, platforms that assess intraday deviations and model call deflections are essential for companies seeking to improve operational efficiency and customer satisfaction. Embracing AI-powered KPI forecasting not only streamlines processes but also empowers organizations to make informed, data-driven decisions for a competitive advantage.
- insight7: A leading tool that integrates AI signals to enhance accuracy in KPI forecasting.
The integration of AI signals into KPI forecasting represents a transformative shift in how organizations approach predictive analytics. Insight7 serves as a pioneering tool designed to harness these signals, enhancing accuracy across various key performance indicators such as Forecast Accuracy %, Volume Variance, and SLA Risk Probability. By analyzing substantial data sets in real time, organizations can anticipate trends and allocate resources effectively, ultimately optimizing their operational efficiency.
One of the standout features of Insight7 is its ability to improve predictive precision. By processing rich datasets, this tool augments KPI forecasting with advanced algorithms that learn from historical patterns. Consequently, businesses benefit from a strategic edge, empowering them to respond proactively to market changes. As the demand for accurate forecasting grows, the role of AI-Powered KPI Forecasting will become increasingly crucial, enabling organizations to thrive in a dynamic environment. Through the use of AI, Insight7 not only fosters organizational agility but also ensures teams are equipped to meet evolving customer needs.
- Tool 2: Another supportive platform known for its precision in predicting customer demand and behavioral shifts.
This supportive platform stands out for its exceptional ability to accurately predict customer demand and behavioral shifts. By harnessing advanced AI-driven data signals, it refines forecasting capabilities, enabling organizations to prepare for fluctuations in customer needs. Such precision allows businesses to adjust resources effectively, ensuring they meet demand without overextending themselves.
Furthermore, the platform addresses multiple forecasting KPIs, seamlessly integrating metrics like Forecast Accuracy Percentage and Volume Variance. It empowers organizations to anticipate shifts, thus minimizing SLA Risk Probability and facilitating smarter decisions regarding agent load and occupancy forecasts. This proactive approach not only enhances operational efficiency but also fosters a deeper understanding of customer behavior, helping organizations remain competitive in an ever-changing market environment. With these insights readily available, organizations can transform data into actionable strategies, ensuring they remain responsive to evolving consumer demands.
- Tool 3: Focuses on resource allocation, using AI to predict occupancy and agent load requirements.
Incorporating AI into resource allocation allows organizations to predict occupancy rates and agent load requirements with remarkable accuracy. This AI-powered approach transforms decision-making processes, ensuring that teams are properly equipped to handle varying demands in real-time. By forecasting occupancy levels, businesses can optimize workforce deployment, minimizing idle time and enhancing overall productivity.
AI-Powered KPI Forecasting significantly impacts how businesses allocate resources. It relies on advanced analytics to assess historical interaction patterns, customer behavior, and current market trends, which culminates in actionable insights. The precision of these forecasts reduces the risk of operational disruptions caused by unforeseen spikes in demand. Organizations can thus adopt a proactive stance, better preparing their teams for increased workloads and improving customer satisfaction by ensuring timely support and engagement during peak periods.
- Tool 4: Provides AI-driven solutions to assess shrinkage impacts and intraday deviations.
AI-driven solutions are essential for evaluating the impacts of shrinkage and understanding intraday deviations. By utilizing advanced algorithms, these tools provide organizations with the ability to quickly assess how fluctuations in performance metrics can affect overall operational efficiency. This capability allows for timely adjustments, ensuring projects remain aligned with strategic objectives.
First, the Shrinkage Impact Index quantifies loss in productivity due to unforeseen factors, enabling teams to anticipate challenges proactively. Next, understanding intraday deviations enhances accuracy in operational planning, as teams can adjust resources and schedules in real-time. The seamless integration of these AI-driven insights into existing KPIs not only optimizes workforce management but also greatly improves customer service outcomes. By embracing these AI-powered solutions, organizations can foster a more responsive and agile operational environment, setting the stage for future growth and success.
- Tool 5: Specializes in channel surge readiness, offering insights into handling unexpected spikes in activity.
Understanding how to manage sudden increases in activity is critical for organizations operating in dynamic environments. Tool 5 focuses specifically on channel surge readiness, empowering teams to navigate unexpected spikes effectively. By harnessing advanced AI techniques, this tool provides actionable insights that help organizations prepare for fluctuating demand.
When an unforeseen surge occurs, having the right data signals enables businesses to maintain service quality. Key factors to consider include real-time monitoring, resource allocation strategies, and proactive communication. Real-time analytics allow businesses to detect shifts in activity as they happen, enabling immediate adjustments to staffing or support channels. Additionally, a clear strategy for resource reallocation minimizes customer wait times during peak periods, ensuring continued satisfaction.
By prioritizing channel surge readiness with AI-powered KPI forecasting, organizations can not only withstand surges but also thrive through them. This approach ultimately enhances organizational resilience and customer trust, positioning businesses for long-term success.
Implementation and Optimization of AI-Powered KPI Forecasting
To effectively implement AI-Powered KPI Forecasting, organizations should start by focusing on data collection and integration. Identifying relevant data signals is crucial to building a strong foundation for forecasting models. This involves gathering historical data on various key performance indicators, such as Forecast Accuracy %, Volume Variance, and SLA Risk Probability. Integrating these diverse signals not only enhances the quality of predictions but also prepares the framework for more advanced analytical techniques.
Once the data foundation is established, organizations must proceed with model training and testing. Utilizing historical data to train AI models can improve their accuracy and adaptability to changing conditions. Additionally, continuous testing of these models ensures they remain reliable in various scenarios, adapting to fluctuations in Agent Load Prediction and occupancy levels. Maximizing the benefits of AI in KPI forecasting allows organizations to not only anticipate needs but also drive efficiency and enhance decision-making processes throughout their operations.
Step-by-Step Guide to Implementing AI-Driven Forecasting
Successfully implementing AI-driven forecasting requires a structured approach that aligns your forecasting strategy with technology. The first step involves data collection and signal integration, where you gather various data signals from multiple sources. This diverse data pool serves as the foundation for AI models, ensuring your forecasts are informed by substantial input.
Next, model training and testing is crucial. In this stage, use historical data to train your AI models and rigorously test their accuracy across different scenarios. This training will allow the models to identify patterns and improve their predictive capabilities. Once your AI systems are operational, you can maximize the benefits by focusing on key KPIs like forecast accuracy and agent load prediction. Aligning the implementation process with these steps ensures that your AI-powered KPI forecasting system operates efficiently, significantly enhancing accuracy and efficiency across your organization.
Walk through the essential steps to effectively implement AI in your forecasting strategy, enhancing accuracy and efficiency.
To successfully implement AI in your forecasting strategy, start with robust data collection. Gather diverse data signals, including historical trends and real-time inputs. This foundation enables AI-powered KPI forecasting to analyze patterns effectively, ensuring your models can adapt to changing circumstances.
Next, engage in model training and testing. Use your collected data to train AI models, refining their accuracy over time. Conduct rigorous testing in various scenarios to guarantee they perform reliably under different conditions. This iterative process improves the precision of key KPIs like Forecast Accuracy and Volume Variance.
Finally, maintain ongoing monitoring and adjustment. Regularly assess your forecasts against actual outcomes to identify gaps and opportunities for enhancement. By fostering an environment of continuous improvement, you not only strengthen your forecasting accuracy but also increase overall efficiency. Embrace these steps to revolutionize your forecasting strategy through AI integration, positioning your organization for success.
Step 1: Data Collection and Signal Integration
In the first step of our AI-Powered KPI Forecasting framework, the focus is on data collection and signal integration. This foundational stage is crucial, as it determines the quality and reliability of the forecasts generated later. Gathering diverse data signals from various sources, such as customer interactions and operational metrics, allows for a comprehensive view of current trends and patterns. These data points provide the raw material essential for developing robust forecasting models.
Once data is collected, integrating these signals becomes critical. This process involves synthesizing information from multiple channels to create a unified dataset. Effective integration enhances the predictive accuracy of KPIs like Forecast Accuracy %, Volume Variance, and Occupancy Forecast. By establishing a solid groundwork through meticulous data collection and signal integration, organizations can better anticipate customer demands and optimize their resource allocation strategies. This sets the stage for improving metrics such as SLA Risk Probability and Agent Load Prediction, leading to a more efficient operation overall.
Integrate diverse data signals to lay the foundation for AI-driven forecasting models.
Integrating diverse data signals is vital for establishing a solid foundation in AI-Powered KPI Forecasting. Leveraging a variety of data sourcesโsuch as historical trends, real-time analytics, and external market factorsโenables organizations to create robust forecasting models that yield accurate predictions. With these varied signals, businesses can better analyze metrics like Forecast Accuracy %, Volume Variance, and SLA Risk Probability, thus optimizing resource allocation and improving operational efficiency.
To effectively integrate data signals, follow these key steps:
- Data Aggregation: Collect comprehensive data from internal and external sources to ensure a holistic view.
- Signal Interpretation: Analyze data signals to understand patterns and correlations between different KPIs.
- Model Development: Use machine learning algorithms to develop predictive models that autonomously adapt to incoming data signals.
- Continuous Learning: Implement feedback loops for models to learn from real-time outcomes, refining predictions over time.
This structured approach ensures that organizations build adaptive forecasting models, ultimately enhancing decision-making and strategic planning processes.
Step 2: Model Training and Testing
Training and testing your AI models marks a critical phase in AI-powered KPI forecasting. This step ensures your models effectively learn from historical data to make accurate predictions about key performance indicators, such as Forecast Accuracy % and Agent Load Prediction. By feeding your models diverse datasets, you enhance their ability to recognize patterns and nuances that drive performance outcomes.
Furthermore, rigorous testing is vital. This involves evaluating the models under various scenarios to ensure reliability and precision in real-world applications. Assessing the models' predictions can help determine their effectiveness in predicting SLA Risk Probability and Channel Surge Readiness, ultimately refining the forecasting process. Through continuous training and testing, organizations can achieve substantial improvements in forecasting accuracy, thus enabling more informed decision-making and strategic planning.
Train your AI models using historical data and test them for accuracy in different scenarios.
To enhance AI-Powered KPI Forecasting, training models using historical data is vital. Start by gathering relevant data that reflects past performance across key indicators like Forecast Accuracy % and Volume Variance. This foundational step allows the AI to learns patterns and trends, creating a robust predictive framework. Once the data is collected, begin the model training process, ensuring that the selection of algorithms aligns with your specific forecasting needs.
After training your AI models, rigorously test them in various scenarios to assess their accuracy. Incorporate measures such as SLA Risk Probability and Agent Load Prediction to evaluate how well your models predict different outcomes. Conducting these tests helps refine the models further, identifying areas for improvement. By continuously revisiting your historical data and testing conditions, you can significantly boost the reliability of your AI models, ensuring they contribute effectively to your forecasting objectives.
Maximizing the Benefits of AI for KPI Assessment
To maximize the benefits of AI for KPI assessment, organizations must first embrace AI-powered KPI forecasting as a transformative tool. This approach not only enhances accuracy in predictions but also enables deeper insights into critical areas such as Forecast Accuracy %, Volume Variance, and SLA Risk Probability. By effectively leveraging these AI data signals, organizations can anticipate fluctuations, allocate resources efficiently, and improve overall performance.
Implementing AI-driven strategies involves several key steps. First, robust data collection and signal integration forms the foundation of successful forecasting. Next, organizations should focus on training AI models using historical data to ensure efficiency and accuracy in diverse scenarios. Aspects such as Agent Load Prediction and Call Deflection Modeling gain significant improvements through continuous model refinement. Ultimately, the optimization of these AI forecasting tools paves the way for proactive decision-making and enhanced customer satisfaction.
Optimizing for Call Deflection Modeling
Optimizing for Call Deflection Modeling involves harnessing AI data signals to accurately predict customer behaviors, enhancing operational efficiency and customer experience. By analyzing historical call data and user interactions, organizations can identify patterns that lead to successful call deflection strategies. This predictive approach not only anticipates call volumes but also helps in redirecting customers to self-service options, thereby reducing the need for agent intervention.
Utilizing AI-Powered KPI Forecasting, companies can refine their call deflection models, ensuring that resources are optimized effectively. Key factors such as customer journey mapping and previous interactions can be integrated into these models. In doing so, businesses can elevate customer satisfaction while simultaneously minimizing operational costs. With advanced analytics, organizations become equipped to handle fluctuations in call volume efficiently, improving overall service delivery. Implementing such forward-thinking methodologies is crucial for any organization aiming to thrive in todayโs competitive climate.
Understand how AI can predict call deflection, optimizing resources and improving customer satisfaction.
AI can significantly improve call deflection modeling, a key aspect of optimizing resources and enhancing customer satisfaction. With advanced machine learning algorithms, AI analyzes historical call data to identify patterns and predict when customers might opt for alternatives to traditional voice support, such as chatbots or self-service options. This foresight enables organizations to adjust their resources proactively, ensuring efficient handling of customer interactions.
Moreover, understanding potential call deflection allows businesses to enhance customer experience by providing timely alternatives. When customers can easily access the support they need without waiting for an agent, satisfaction increases, enhancing loyalty. By integrating AI-powered KPI forecasting, organizations not only streamline their staffing but also create a more responsive and satisfying customer journey. This ultimately leads to optimized operations, reduced operational costs, and improved customer engagement.
Preparing for Channel Surge Readiness
Unexpected surges in customer interactions can significantly impact an organization. Preparing for Channel Surge Readiness involves understanding and anticipating these fluctuations to ensure services remain uninterrupted. By utilizing AI-powered KPI forecasting, businesses can aggregate essential data signals that predict surges in volume and help align resources effectively.
To achieve optimal channel surge readiness, organizations should focus on two key aspects:
Data Integration: Gather various data signals, including historical trends and agent performance metrics. This comprehensive collection lays the groundwork for more reliable forecasts.
Scenario Planning: Develop strategies by using predictive insights to simulate different surge scenarios. This proactive approach helps organizations prepare for potential fluctuations, minimizing risks associated with sudden increases in demand.
Harnessing these strategies will enhance not only readiness but also overall customer satisfaction during peak times. The capabilities of AI in forecasting are invaluable in navigating these complexities effectively.
Ensure your systems are ready for unexpected surges by leveraging AI-driven insights.
Preparing your systems for unexpected surges is vital in todayโs fast-paced environment. By utilizing AI-driven insights, organizations can predict and adapt to sudden increases in demand. AI-powered KPI forecasting equips companies with actionable data, allowing them to respond efficiently. For instance, understanding channel surge readiness can enable teams to optimize resource allocation in real-time, ensuring customer needs are met without compromising service quality.
Moreover, AI can refine several KPIs, such as agent load prediction and occupancy forecasts. These insights allow organizations to forecast staffing requirements and manage agent availability during peak times. By integrating these advanced methodologies, teams can stay ahead of potential disruptions, enhance overall efficiency, and improve customer satisfaction. Ultimately, leveraging AI-driven insights becomes essential for achieving operational resilience against unexpected demand surges, making your systems not only more capable but also ready for future challenges.
Conclusion: Embracing the Future of AI-Powered KPI Forecasting
Embracing the future of AI-powered KPI forecasting signifies a transformative leap in how organizations understand and anticipate their operational needs. By harnessing advanced data signals, businesses can achieve unprecedented levels of accuracy in forecasting critical metrics such as Forecast Accuracy % and Volume Variance. This technological evolution not only enhances decision-making but also prepares companies for potential SLA risks and demand fluctuations.
As we look ahead, it is crucial to adopt AI-powered KPI forecasting as a standard practice. The insights gained from metrics like Agent Load Prediction and Call Deflection Modeling will optimize resource allocation and improve customer experiences. By acknowledging the potential of AI in shaping forecasting strategies, organizations can position themselves effectively, ensuring resilience and growth in an increasingly complex landscape.
AI-powered KPI forecasting is more than just a trend; its a pivotal shift towards more intelligent and predictive resource management. Embrace these advancements to stay ahead of the curve and ensure your organization is fully prepared for the challenges of tomorrow.
AI-powered KPI forecasting signifies a transformative leap in how organizations approach resource management. This evolution goes far beyond a mere trend; it signifies a move towards strategic decision-making driven by data-driven insights. By using advanced AI algorithms, organizations can enhance their ability to predict needs, optimize resource allocation, and improve operational efficiency. This shift enables companies to anticipate challenges before they arise, allowing for proactive solutions rather than reactive responses.
As businesses embrace these advancements, they position themselves to navigate the complexities of future landscapes effectively. The importance of staying ahead of the curve cannot be overstated, especially in an era defined by rapid change and increasing competition. By leveraging AI-powered insights into KPIs like Forecast Accuracy, Volume Variance, and SLA Risk Probability, organizations can make smarter decisions that pave the way for sustained success. It is essential to recognize these tools as vital assets for preparing to meet the challenges of tomorrow.