7 Pitfalls in QA Data Interpretation and How to Avoid Them
-
Bella Williams
- 10 min read
In navigating the complexities of QA data misinterpretation avoidance, understanding the journey is pivotal. Quality assurance is often a meticulous process where the risk of misunderstanding data can lead to significant consequences. Organizations may find themselves misinformed, leading to erroneous decisions and flawed strategies. By recognizing common pitfalls, teams can work toward effective solutions, ensuring that data is interpreted correctly and applied appropriately.
The key to successfully avoiding misinterpretation lies in fostering a culture of clarity and continuous learning. Building strong data literacy among team members not only enhances their analytical skills but also promotes a deeper understanding of the data's context. As we delve into this exploration of pitfalls and prevention strategies, we focus on how teams can leverage these insights for improved decision-making and successful outcomes in QA practices.
Extract insights from Customer & Employee Interviews. At Scale.
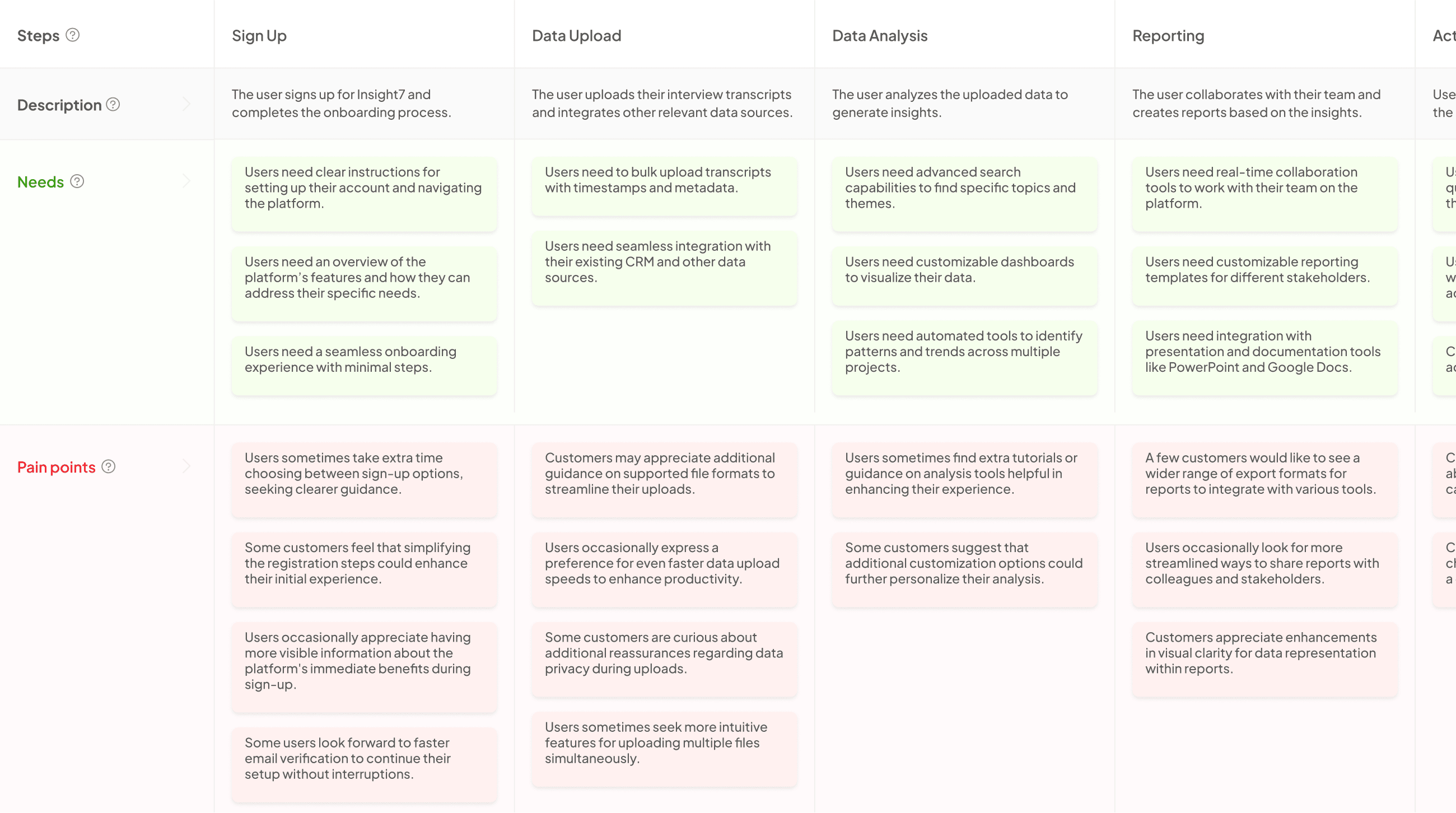
Common Pitfalls in QA Data Interpretation
In the realm of QA data interpretation, common pitfalls can lead to significant errors in conclusions. One frequently encountered issue is the tendency to overlook context. When data is examined in isolation, the nuances and factors driving those numbers may be missed. This oversight can result in misguided strategies that fail to address the actual problems at hand, highlighting the importance of thorough contextual understanding in QA Data Misinterpretation Avoidance.
Another notable pitfall is the misinterpretation of patterns due to statistical anomalies. False positives and negatives can create misleading narratives, potentially skewing key decisions. Teams must be diligent in identifying these anomalies to mitigate their influence. Training team members in statistical analysis and encouraging critical thinking can help minimize these issues. By recognizing these common pitfalls and adopting proactive strategies, organizations can greatly enhance the reliability of their QA data interpretation efforts.
Understanding False Positives and Negatives in QA Data Misinterpretation Avoidance
In understanding false positives and negatives, it's crucial to recognize their significant impact on quality assurance data interpretation. False positives occur when data suggests a problem exists while there is none, leading to unnecessary alarms. Conversely, false negatives risk downplaying issues that do exist, which could lead to larger problems down the road. Both outcomes can skew decision-making processes and hinder effective strategy development.
To minimize these misunderstandings, teams can adopt specific strategies. First, validating data sources ensures the reliability of insights gathered. Next, implementing robust testing methodologies helps differentiate between true signals and noise in data. Regular feedback sessions within teams can further illuminate different perspectives, ultimately fostering a deeper understanding of potential pitfalls. By focusing on these strategies, organizations can enhance their QA data misinterpretation avoidance, ensuring more informed and accurate decision-making.
- Identifying the Impact on Decision Making
The impact of data misinterpretation on decision making can be significant, often leading to misguided strategies. QA data misinterpretation avoidance is essential for providing accurate insights that guide informed decisions. When data is misunderstood, it can result in flawed conclusions, which ultimately affects the outcomes of projects. Therefore, organizations must prioritize the integrity of their data interpretation processes.
First, clear communication regarding data criteria and metrics is vital. By ensuring that all team members understand the parameters surrounding the data, decision makers can minimize confusion. Secondly, regular training and updates about data assessment techniques are essential in cultivating a more knowledgeable team. This ongoing development allows teams to approach data with a critical mindset, reducing the chances of misinterpretation. By striving for clarity and understanding, organizations can significantly enhance the quality of their decision-making processes.
- Strategies to Minimize Misunderstanding
To minimize misunderstandings in QA data interpretation, it’s essential to foster a culture of clarity and communication among all team members. Key strategies for QA data misinterpretation avoidance include ensuring that team members are well-trained in data analysis principles and equipped to recognize the nuances in data, such as false positives and negatives. Regular training sessions can help enhance their ability to interpret data accurately and develop critical thinking skills essential for effective analysis.
Another important strategy involves the use of collaborative tools that promote shared understanding. Creating a platform for discussions about data findings encourages questions and clarifications, which reduces miscommunication. Additionally, establishing clear guidelines for documentation can serve as a reference point, allowing team members to review past discussions and interpretations. By implementing these strategies, teams can significantly reduce misunderstandings, leading to more accurate and informed decision-making in QA projects.
Over-Reliance on Quantitative Data in QA Projects
In QA projects, an over-reliance on quantitative data can lead to significant misinterpretation. While numerical data offers valuable insights, it often lacks the contextual depth that qualitative data provides. For instance, a high defect rate may indicate a problem, but understanding user sentiments and feedback is crucial to fully grasp the underlying issues. Without this holistic view, teams risk making decisions based solely on numbers, potentially overlooking critical factors that could impact the project's success.
To mitigate this pitfall, it is essential to balance both quantitative and qualitative data. Incorporating user feedback or team insights alongside metrics allows for a more comprehensive understanding of the data. Furthermore, relaying findings in context enhances their relevance and utility. By maintaining this balance, teams can drive informed decision-making and effectively avoid QA data misinterpretation. Data literacy and critical analysis skills will also empower teams to scrutinize quantitative results meaningfully.
- Balancing Quantitative and Qualitative Data
In the quest for effective QA data interpretation, balancing quantitative and qualitative data is crucial. Quantitative data provides measurable metrics, while qualitative insights offer depth and context. This balance ensures a comprehensive understanding of user experiences and potential issues. Relying solely on numbers can lead to an incomplete picture, whereas qualitative feedback can illuminate nuances that statistics alone can't capture.
To avoid QA data misinterpretation, it is essential to integrate these two forms of data seamlessly. Begin by collecting a mix of both data types, ensuring decision-making is informed by thorough analysis. This approach fosters a more nuanced understanding of customer feedback, addressing discrepancies that may not be apparent through quantitative data alone. Furthermore, discussing findings with team members can uncover differing perspectives, enriching the analysis process and ensuring all voices are heard. By prioritizing this balance, organizations can navigate pitfalls in QA data interpretation more effectively.
- Importance of Contextual Understanding
Understanding the context surrounding data is crucial for effective QA Data Misinterpretation Avoidance. Without context, data points can become misleading, leading to erroneous conclusions. For instance, interpreting a spike in user complaints without recognizing seasonal fluctuations could prompt unnecessary operational changes or resource allocation. This necessity becomes evident when analyzing qualitative insights alongside quantitative data, as one provides depth and the other provides breadth.
Contextual understanding fosters a deeper appreciation of user experiences. It allows QA teams to ask meaningful questions about the data they gather. For instance, it’s essential to delve into why users prefer one feature over another. This exploration not only identifies critical areas for improvement but also helps in crafting strategies that align with user needs and expectations. Ultimately, staying aware of the full circumstances surrounding data ensures that decisions are made with a holistic view, minimizing the risk of misinterpretation and its associated pitfalls.
Generate Journey maps, Mind maps, Bar charts and more from your data in Minutes
Effective QA Data Misinterpretation Avoidance Techniques
To effectively address QA data misinterpretation avoidance, consider the following techniques designed to empower teams in making accurate assessments. First, continuous training and development is crucial. Investing in regular workshops enhances the team's understanding of data analysis principles, ensuring everyone is proficient in identifying critical metrics. With improved data literacy, team members are better equipped to spot potential misinterpretations early.
Second, promoting critical thinking and analytical skills within QA teams fosters an environment where thoughtful analysis thrives. Encourage team discussions around data findings to explore various interpretations. This collaborative approach can illuminate different perspectives and help mitigate biased conclusions.
In addition, implementing specialized QA tools can streamline data analysis and provide insightful interpretations. Tools like Insight7 can significantly support teams in analyzing data accurately. By combining these strategies, organizations can create a robust framework for effective QA data misinterpretation avoidance.
Steps to Enhance Data Literacy within QA Teams
Building data literacy within QA teams is essential to minimize QA data misinterpretation avoidance. The first step is to implement continuous training and development programs focused on data analysis skills. Regular workshops can introduce team members to newer data interpretation technologies and methods, thereby enhancing their confidence and competence in working with data. Encouraging participation in online courses related to data analytics can also ensure that everyone stays updated on best practices.
Next, fostering a culture of critical thinking and analytical skills is crucial. QA teams should be encouraged to question data sources, methodologies, and their implications on the end product. This can be achieved by creating a collaborative environment where team members regularly discuss findings and interpretations. By engaging in these discussions, team members can develop a deeper understanding of data context and avoid relying solely on quantitative metrics, ultimately leading to improved decision-making processes.
💬 Questions about 7 Pitfalls in QA Data Interpretation and How to Avoid Them?
Our team typically responds within minutes
- Continuous Training and Development
Continuous training and development are vital components for minimizing QA data misinterpretation avoidance. Regular training sessions equip team members with the latest methodologies and insights by refining their analytical skills. For instance, understanding the intricacies of both qualitative and quantitative data fosters improved decision-making, leading to more accurate interpretations. Additionally, ongoing development opportunities can cultivate a culture of learning and adaptability within the team.
To effectively ensure continuous training, organizations should prioritize an assessment of current knowledge gaps. This allows for tailored training programs that address specific areas of potential misinterpretation. Furthermore, tracking performance metrics post-training helps measure its effectiveness. This gives insights into which aspects have improved and where further attention is needed. Ultimately, investing in continuous training and development not only enhances the team's capabilities but also drives better outcomes in QA data interpretation.
- Promoting Critical Thinking and Analytical Skills
In QA environments, fostering critical thinking and analytical skills is essential for preventing data misinterpretation. Teams that encourage a questioning mindset can better analyze data, moving beyond surface-level conclusions. By promoting discussions around potential biases and assumptions, team members learn to challenge their perspectives and examine the broader context of their data, ultimately enhancing their decision-making abilities.
To cultivate these skills, organizations can implement several strategies. Continuous training focused on problem-solving can sharpen analytical abilities. Creating a culture that values inquiry encourages team members to actively engage with the data they encounter. Incorporating group analysis sessions can foster collaboration, allowing diverse perspectives to uncover insights that might be overlooked. These steps are vital as teams strive for QA data misinterpretation avoidance, ensuring data integrity and making informed decisions that lead to optimal outcomes.
Implementing QA Tools for Accurate Data Analysis
Implementing QA tools for accurate data analysis is crucial for avoiding common pitfalls in QA data interpretation. The right tools can enhance accuracy and provide actionable insights, ultimately supporting better decision-making. Effective QA data misinterpretation avoidance begins with selecting tools that align with your team's needs and integrating them into your workflow.
To achieve optimal results, consider utilizing a mix of comprehensive data interpretation tools like Insight7, TestRail, and QA Wolf. These technologies allow for seamless data tracking, trend analysis, and collaborative projects. Tools such as Zephyr and PractiTest offer specialized functions that help teams manage and interpret data effectively. By establishing a systematic approach to tool implementation, organizations can foster a data-driven culture equipped to address misunderstandings. Empowering QA teams with these essential tools will not only enhance their analytical capabilities but also promote confidence in data-driven outcomes.
- Insight7: Comprehensive Data Interpretation Support
Accurately interpreting data is pivotal for efficient quality assurance (QA), and Insight7 offers comprehensive support in this area. By utilizing state-of-the-art tools, QA teams can significantly reduce the risks associated with misinterpretation. Proper data analysis not only streamlines the decision-making process but also fosters a culture of collaboration. When insights are readily accessible and well-organized, teams are more likely to translate data into actionable strategies that align with business goals.
A major challenge in QA data interpretation is the overwhelming volume of data generated from customer interactions. To mitigate this issue, businesses can implement structured methodologies for analyzing information. Incorporating frameworks that emphasize active listening and thorough questioning can further enhance understanding. This proactive approach aids in avoiding common pitfalls in QA data misinterpretation avoidance. By prioritizing a comprehensive analysis of both qualitative and quantitative data, organizations will ultimately see improved outcomes and a better competitive edge in the market.
- Other Essential Tools:
In navigating QA Data Misinterpretation Avoidance, selecting the right tools is imperative. Our exploration of essential tools begins with QA Wolf, designed for seamless integration and automated testing capabilities. This tool enables teams to identify and rectify data inconsistencies efficiently. Following closely is TestRail, renowned for its robust test case management system. TestRail aids in tracking test results, ensuring that every detail is analyzed accurately, which is crucial to avoiding misinterpretation.
Next, we have Zephyr, a leading tool known for its flexibility and real-time insights. It supports teams in managing tests directly within the agile framework, which enhances collaboration and decision-making. Lastly, PractiTest provides a comprehensive approach to QA management, offering insights that unify both management and project teams. By effectively leveraging these tools, organizations can significantly reduce the risks associated with QA data misinterpretation. Integrating these resources promises a clearer pathway toward informed decision-making based on accurate data analysis.
- QA Wolf
In QA processes, tools play a critical role in ensuring compliance and accuracy in data interpretation. QA Wolf represents a streamlined solution that facilitates evaluations by allowing teams to establish criteria for assessing calls and compliance. This capability is essential for preventing QA data misinterpretation avoidance. By defining specific metrics and leveraging automated scoring, users can easily benchmark calls against predetermined standards. This not only saves time but also enhances the reliability of the analyses conducted.
However, proper implementation is crucial. Users must have a clear understanding of their evaluation criteria to maximize the tool's effectiveness. Continuous training on the features and functionalities of QA Wolf can empower teams to utilize the software fully, thereby mitigating risks associated with data misinterpretation. By fostering an environment where data is meticulously evaluated against established standards, organizations can improve their overall quality assurance processes.
- TestRail
In the realm of QA data analysis, tools like TestRail play a pivotal role in QA data misinterpretation avoidance. TestRail, known for its user-friendly interface, helps teams structure their testing processes efficiently. By integrating detailed test cases and outcomes, it provides a clear visual representation of testing metrics that minimize the chances of misinterpretation.
One of the key advantages of using TestRail is its ability to generate comprehensive reports, emphasizing key performance indicators. This function enables QA teams to compare data over time, identifying trends and potential pitfalls in data interpretation. Additionally, organizing tests by parameters ensures a structured approach that aids in aligning results with project objectives. Ultimately, employing TestRail not only enhances data clarity but also fosters a collaborative environment that empowers teams to discuss findings and insights, thus reinforcing effective QA data misinterpretation avoidance.
- Zephyr
In the realm of QA, Zephyr stands out as a tool that can significantly enhance data accuracy and streamline interpretation processes. It allows teams to manage their test cycles and track results with great precision. The integration of Zephyr into QA workflows can ensure that the data collected is aligned with project goals and is easily accessible, facilitating better data interpretation.
However, reliance solely on the features of Zephyr may introduce pitfalls if teams overlook the context of the data. To avoid QA data misinterpretation, it is crucial to combine Zephyr’s capabilities with proper training. This includes understanding how to analyze the data generated effectively and applying critical thinking to discern larger trends. By fostering a culture of inquiry and continuous learning, teams utilizing Zephyr can minimize misinterpretation risks and enhance overall quality assurance outcomes.
- PractiTest
In the realm of quality assurance, the choice of tools significantly influences the accuracy of data interpretation. PractiTest emerges as a vital resource, assisting teams in managing and interpreting data with precision. This platform seamlessly integrates different testing processes, making it easier to track and analyze data efficiently. By consolidating information, teams can avoid the common pitfalls of misinterpretation, ensuring that decisions are based on reliable insights.
To effectively utilize PractiTest for QA data misinterpretation avoidance, it's essential to understand its key features. Customizable templates allow teams to align evaluations with specific criteria, promoting consistency in assessments. Additionally, the ability to generate detailed reports provides clarity that aids in understanding outcomes. With such capabilities, quality assurance teams can better navigate challenges and enhance their decision-making processes, ultimately leading to improved quality outcomes.
Conclusion: Mastering QA Data Misinterpretation Avoidance for Better Outcomes
Mastering QA Data Misinterpretation Avoidance is crucial for achieving better outcomes in any QA initiative. By acknowledging the common pitfalls identified in data interpretation, teams can proactively develop strategies to prevent misinterpretations. This involves fostering a culture of continuous learning and adaptation, where both quantitative and qualitative insights are valued equally.
Furthermore, implementing effective tools that support accurate data analysis is essential. Empowering QA teams through enhanced data literacy and critical thinking skills can significantly mitigate risks associated with data misinterpretation. Ultimately, focusing on QA data misinterpretation avoidance not only ensures better decision-making but also contributes to overall project success and stakeholder satisfaction.
💬 Questions about 7 Pitfalls in QA Data Interpretation and How to Avoid Them?
Our team typically responds within minutes