AI error elimination is transforming how businesses approach forecasting, leading to unprecedented accuracy and reliability. Traditionally, forecasting is plagued by human biases and data processing mistakes, resulting in unreliable predictions. However, with the integration of AI, organizations can significantly reduce these errors, providing insights grounded in data rather than intuition.
In this section, we will explore how AI-driven technologies streamline the forecasting process, effectively addressing common pitfalls. By automating data analysis and enhancing predictive models, AI not only refines accuracy but also empowers decision-making. As we delve deeper, you'll discover six specific forecasting errors that AI helps to eliminate, paving the way for smarter strategies and improved outcomes.
Analyze qualitative data. At Scale.
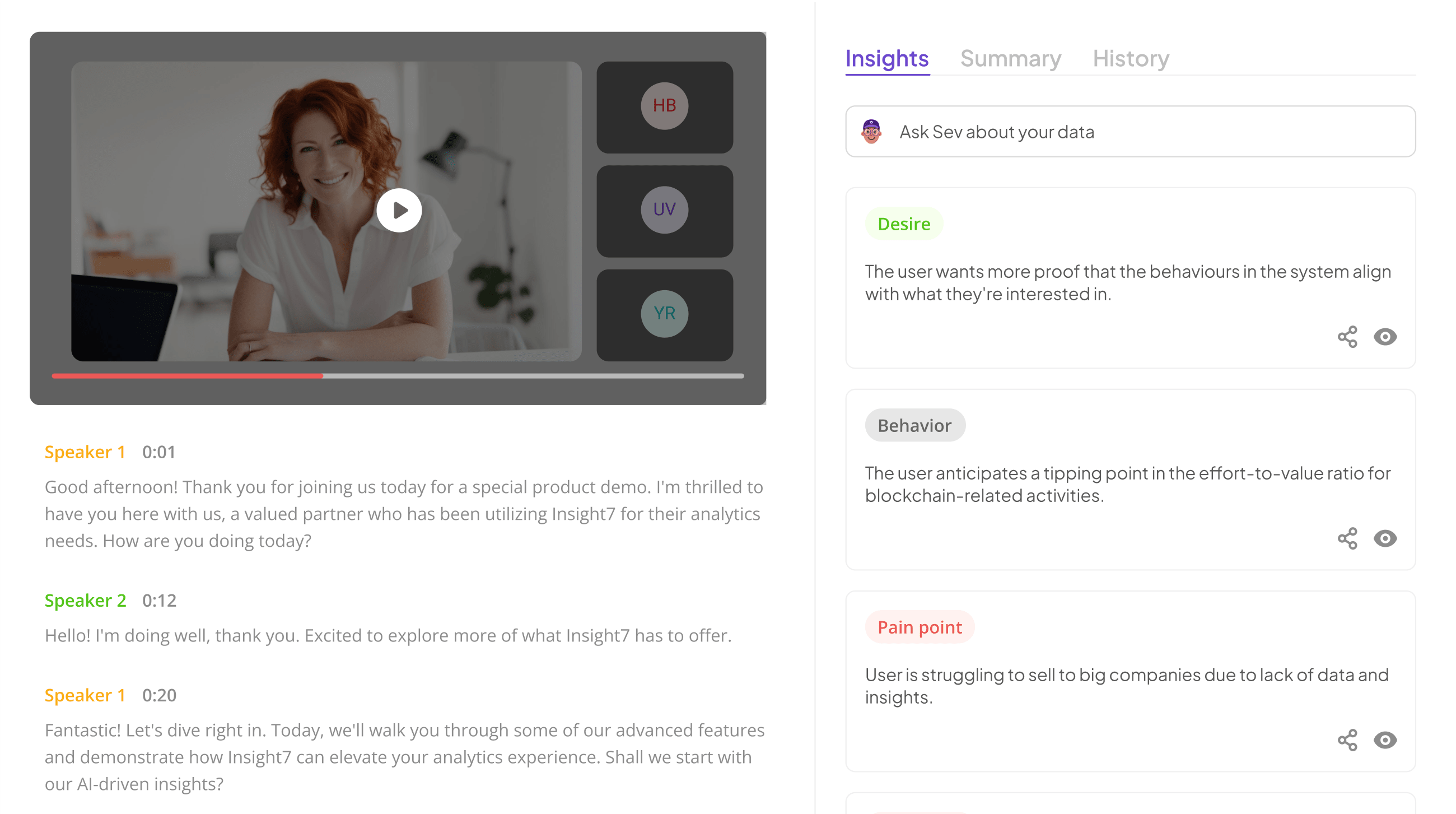
Understanding Common Forecasting Errors
Understanding common forecasting errors is essential for improving prediction accuracy. Many businesses struggle with inaccurate forecasts due to internal and external biases. Human factors such as overconfidence, confirmation bias, and emotional decision-making often lead to flawed estimations. By recognizing these pitfalls, organizations can begin to mitigate their impact and enhance the forecasting process.
AI error elimination plays a significant role in refining forecasts. It employs advanced algorithms to sift through enormous data sets, minimizing the influence of human biases. Additionally, AI can identify patterns and anomalies that may not be immediately apparent to human analysts, helping to streamline data processing and enhance predictive accuracy. Embracing AI for these tasks substantially reduces common errors, fostering more strategic and informed decision-making across various sectors.
The Impact of Human Bias on Forecasts
Human bias significantly impacts the accuracy of forecasts, leading to misguided decisions and erroneous predictions. Cognitive biases, such as confirmation bias and overconfidence, often cloud judgment. When individuals rely on historical data filtered through their subjective perspectives, the resulting forecasts lack objectivity. This dynamic can not only skew outcomes but also perpetuate long-standing misconceptions within organizations.
AI error elimination techniques can effectively mitigate these human biases. By harnessing vast amounts of unbiased data, AI models provide a clearer, more objective view of trends and patterns. These advanced systems analyze information devoid of personal prejudice, thus enhancing the reliability of forecasts. Ultimately, targeting human biases in forecasting through AI can foster better decision-making and help organizations achieve their strategic goals with greater confidence. Embracing this technology is essential for adapting to an increasingly complex market landscape.
Common Data Processing Mistakes
Data processing mistakes can significantly distort forecasting outcomes, hinder decision-making, and ultimately lead to poor business strategies. Common errors occur when data is incorrectly analyzed, resulting in misleading insights. For instance, overlooking data normalization can skew results and cloud judgment. Ignoring data quality can introduce dramatic inaccuracies, leading to incorrect assumptions about market trends.
AI plays a crucial role in error elimination, automating the detection of inconsistencies and improving data reliability. By implementing sophisticated algorithms, businesses can mitigate human biases that often seep into data handling. For example, an AI tool can highlight discrepancies in data sourcing, allowing teams to address issues proactively. Embracing AI-driven technologies not only enhances data accuracy but also streamlines workflows. As a result, organizations can more effectively identify market patterns and make informed decisions, ultimately gaining a competitive edge.
AI Error Elimination: Improving Accuracy and Precision
AI Error Elimination focuses on enhancing the accuracy and precision of forecasting by addressing inherent data vulnerabilities. In a world where predictive insights are paramount, leveraging AI technologies can dramatically reduce errors that typically stem from human biases or data processing mistakes. The integration of AI creates a framework where historical data is analyzed systematically, providing forecasts that are not only reliable but also relevant.
Furthermore, this error elimination process ensures that predictive models remain robust. By utilizing advanced algorithms, AI can fine-tune forecasts, adapting to new data patterns with remarkable efficiency. In this evolving landscape, understanding specific forecasting errors enables organizations to implement AI-driven strategies effectively. As a result, enhanced accuracy and precision are within reach, making AI Error Elimination a critical component of modern forecasting methodologies. Embracing these changes can lead to significantly improved decision-making and operational success.
Extract insights from interviews, calls, surveys and reviews for insights in minutes
AI Error Elimination in Time-Series Analysis
In time-series analysis, AI error elimination is essential for enhancing the reliability of forecasts. Traditional methods often grapple with human biases and inaccuracies, leading to unreliable predictions. AI technologies help to systematically reduce these errors by analyzing vast datasets and recognizing patterns that escape human detection. This efficiency not only streamlines the forecasting process but also significantly boosts accuracy.
Several factors highlight AI error elimination's role in time-series analysis. First, AI can effectively handle complex data patterns, which helps in identifying and correcting anomalies. Second, it minimizes the impact of human error by standardizing data input and processing. Lastly, AI continuously learns and adapts, improving the predictive model with every iteration. These advancements make forecasts more reliable and actionable, ultimately guiding better decision-making in various sectors. This is a pivotal transformation that demonstrates the importance of integrating AI in time-series forecasting.
Boosting Predictive Models with AI Algorithms
Predictive models are essential for making informed business decisions. By incorporating AI algorithms, businesses can enhance their forecasting capabilities significantly. These algorithms excel at identifying patterns and making predictions based on vast datasets, improving accuracy and reducing errors in forecasts.
AI error elimination is a game-changer in this context. First, AI can detect anomalies and inconsistencies in historical data, allowing organizations to rectify inaccuracies before they impact predictions. Second, it automates complex calculations, minimizing human error and bias that often contaminate forecasts. Finally, the predictive power of AI helps teams adapt to market changes swiftly, ensuring they stay ahead of competitors. By boosting predictive models with AI algorithms, businesses can transform their forecasting processes, paving the way for better strategic decisions and enhanced performance in uncertain environments.
Tools for AI Error Elimination in Forecasting
AI Error Elimination plays a vital role in enhancing the reliability of forecasting by integrating specialized tools. These tools are designed to identify discrepancies, streamline data processing, and reduce human biases that often skew predictions. Adopting AI-driven platforms means businesses can leverage advanced algorithms that improve accuracy and optimize models, yielding more precise forecasts.
Several tools stand out in the realm of AI error elimination. First, Tool A minimizes forecasting errors through its adaptable platform, allowing users to input diverse datasets seamlessly. Next, Tool B harnesses powerful machine learning capabilities, enabling dynamic adjustments to predictive models based on new data. Tool C focuses on data normalization and cleansing, ensuring that input data is dependable and ready for analysis. Finally, Tool D offers intuitive interfaces for AI model training, making it accessible for users without extensive technical backgrounds. Together, these tools create a comprehensive toolkit for organizations seeking to enhance their forecasting accuracy through effective AI error elimination.
insight7: Leading the Charge in AI-Driven Forecasting
AI Error Elimination plays a vital role in modern forecasting by tackling prevalent challenges. Traditional forecasting methods often fall prey to human biases and data inaccuracies that can skew results. However, AI-driven approaches are revolutionizing this space, allowing organizations to refine their forecasts through sophisticated algorithms and real-time data analysis. By integrating AI, businesses can enhance predictive accuracy while minimizing errors that stem from manual processes.
Leading the charge in AI-driven forecasting involves leveraging advanced tools that automate data normalization, model training, and error detection. These tools empower businesses to turn raw data into actionable insights swiftly. As companies embrace AI, they not only overcome the limitations of traditional forecasting but also create a competitive edge in the marketplace, ensuring their decisions are backed by reliable information. The future of forecasting is data-driven, and AI is at the forefront of this transformation.
Additional AI Tools for Forecasting Accuracy
AI Error Elimination plays a vital role in refining forecasting processes, ensuring greater accuracy in predictions. Several tools have emerged as frontrunners in this arena, each tailored to address specific forecasting challenges. First on the list is Tool A, which provides users with a versatile platform designed for minimizing errors across various datasets. Its adaptable interface helps streamline the forecasting process, allowing for quick adjustments based on real-time data.
Next, Tool B stands out for its robust machine learning capabilities. By analyzing vast amounts of historical data, it improves predictive accuracy over time. Additionally, Tool C specializes in data normalization and cleansing, eliminating inconsistencies that often lead to errors in forecasting. Finally, Tool D offers intuitive interfaces for AI model training, making it accessible for users with varying levels of expertise. Together, these tools form a comprehensive toolkit for organizations aiming to enhance forecasting accuracy and efficiency.
- Tool A: A versatile platform for error minimization.
A versatile platform for error minimization simplifies the forecasting process significantly. This tool operates through intuitive interfaces that allow users of all skill levels to access and analyze data effectively. With a few clicks, it can synthesize vast amounts of information, identifying key insights that help refine predictions. By streamlining these analytical workflows, businesses can achieve higher accuracy in their forecasts, limiting the potential for human errors.
The platform plays a critical role in AI error elimination by automatically detecting biases and inaccuracies in existing data. Users can easily visualize conversations, uncover pain points, and extract customer feedback directly from the platform. Furthermore, it enables the integration of multiple data sources into cohesive projects, making it an essential resource for teams aiming to enhance their forecasting accuracy. Adopting such tools empowers organizations to embrace data-driven decision-making while mitigating the forecasting errors that typically arise.
- Tool B: Known for robust machine learning capabilities.
Tool B is an essential solution well-regarded for its powerful machine learning capabilities. It excels in transforming raw data into actionable insights, directly addressing common forecasting errors that organizations encounter. This tool efficiently analyzes historical data trends and detects anomalies that often lead to inaccurate predictions.
By utilizing advanced algorithms, Tool B minimizes human biases and various data processing mistakes. It effectively enhances accuracy through continuous learning and adaptation, allowing businesses to refine their forecasting models. With its sophisticated analytics, organizations can confidently make data-driven decisions, cutting down on uncertainties that may jeopardize their strategic objectives. Embracing Tool B represents a significant step towards sustained AI Error Elimination, paving the way for more precise and reliable forecasting in an ever-evolving market landscape.
- Tool C: Specializes in data normalization and cleansing.
Data normalization and cleansing are critical steps in the forecasting process, especially when managing large datasets. Tool C excels at transforming raw data into clean, consistent formats, which is essential for achieving accuracy. By eliminating duplicates, filling in missing values, and standardizing data entries, this tool helps mitigate the risks associated with chaotic data. Proper data organization lays the foundation for effective forecasting and supports AI Error Elimination.
Moreover, clean data enhances the performance of predictive models significantly. When data is normalized, AI algorithms can identify patterns and trends more efficiently, leading to more accurate forecasts. This ensures that decision-makers can rely on actionable insights derived from robust analysis, reducing the likelihood of making errors rooted in poor data quality. With Tool C, organizations can confidently harness the power of AI to refine their forecasting processes and embark on a path toward greater accuracy and success.
- Tool D: Provides intuitive interfaces for AI model training.
Intuitive interfaces play a crucial role in democratizing AI model training. Tool D offers users an accessible way to engage with advanced AI functionality without needing extensive technical expertise. Anyone from a diverse range of backgrounds can harness the potential of AI error elimination simply by navigating its user-friendly platform. This ease of use encourages collaboration and makes data-driven insights available to everyone in an organization.
Moreover, the tool allows users to visualize and analyze data seamlessly. Users can easily upload files, explore insights, and examine key themes across multiple datasets. This streamlined process not only enhances user experience but also significantly reduces the risk of human bias and data misinterpretation. As a result, organizations can achieve superior forecasting accuracy and make informed decisions based on reliable, actionable insights. With Tool D, the path to effective AI model training and forecasting correction is simplified and straightforward.
Conclusion: Embracing AI Error Elimination for Future-Ready Forecasts
AI Error Elimination is essential for businesses aiming to enhance forecasting accuracy. By integrating AI into forecasting processes, organizations can significantly reduce common errors that plague traditional methods. These advancements lead to not only improved decision-making but also a more agile response to market changes.
As we embrace AI's capabilities, the focus shifts towards not just eliminating errors, but also fostering a culture of precision and reliability in forecasts. Future-ready forecasts hinge on the successful application of AI tools, empowering businesses to transform uncertainty into actionable insights while staying ahead of their competition.