AI QA Solutions are revolutionizing the quality assurance sector by addressing persistent challenges that have long plagued the industry. As organizations strive for higher efficiency and accuracy in their testing efforts, the integration of AI technologies has emerged as a game changer. These innovative solutions simplify complex processes, enabling teams to focus on strategic improvements rather than repetitive tasks.
With AI-powered QA tools, businesses can automate testing scenarios, reduce human error, and enhance overall quality assurance practices. This transformation is not merely a trend but a necessary evolution in ensuring that products meet high standards while saving time and resources. Embracing AI QA Solutions marks a significant step towards achieving reliable outcomes in today's fast-paced technological landscape.
Analyze qualitative data. At Scale.
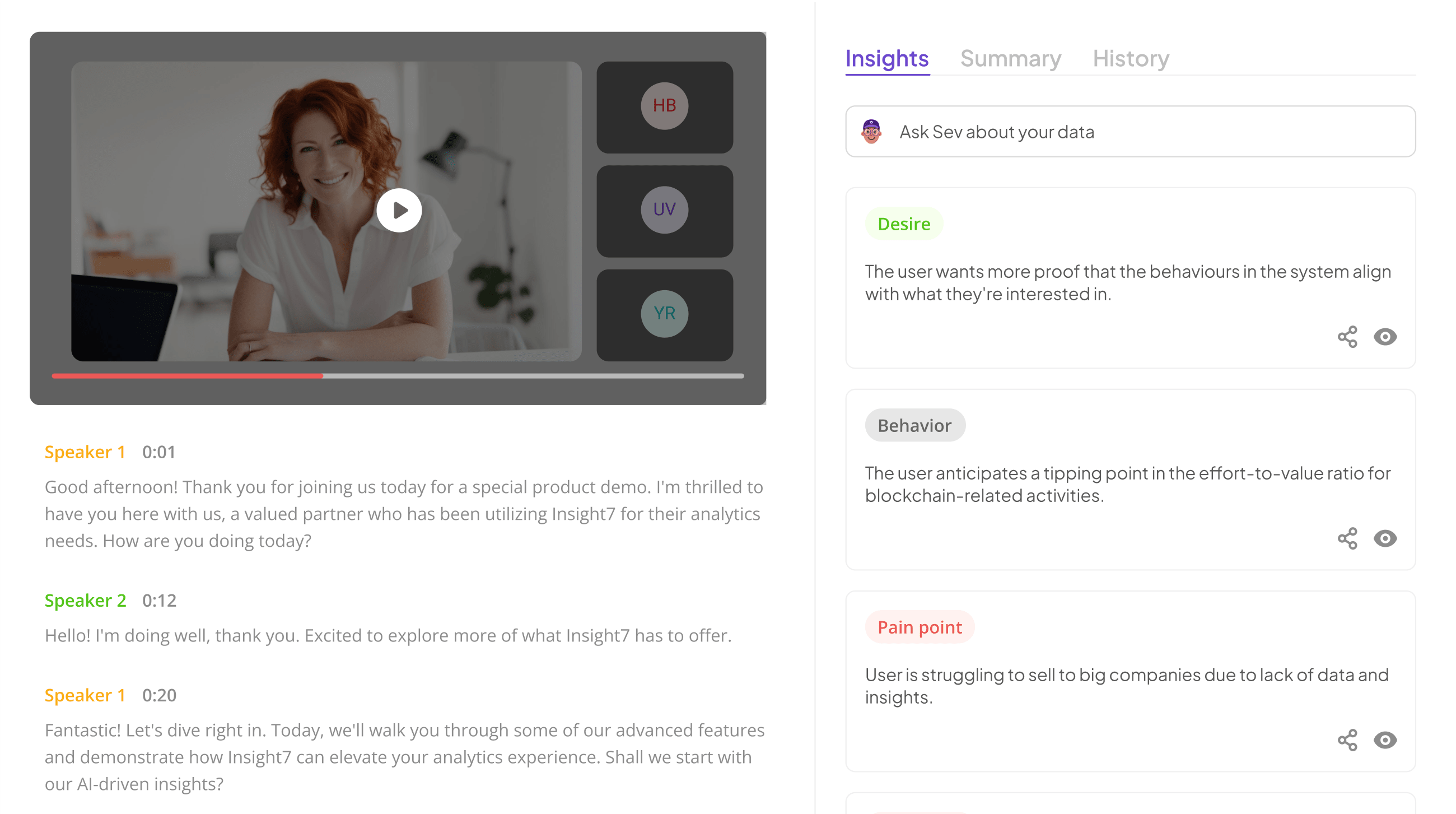
Overcoming Persistence Issues with AI QA Solutions
AI QA Solutions offer significant advantages in overcoming persistence issues commonly faced in quality assurance processes. One of the primary concerns in QA is the tendency for repetitive tasks to lead to oversight and inefficiencies. By automating these tasks, AI-powered systems allow teams to focus on more complex issues, which enhances productivity and reduces errors.
Additionally, AI QA Solutions excel at detecting human errors that can arise from manual testing. These tools utilize advanced algorithms to recognize patterns and inconsistencies, significantly improving the accuracy of quality assessments. By deploying AI-driven verification methods, organizations can ensure that their products meet the highest standards, fostering greater customer satisfaction and trust. In essence, AI QA Solutions are transformative in addressing the pitfalls of traditional QA efforts, ensuring sustained quality and performance.
Automating Repetitive Testing Tasks
In quality assurance, repetitive testing tasks can become a drain on resources and time. AI QA solutions offer a powerful way to automate these tasks, freeing teams to focus on more strategic activities. The first step involves identifying scenarios that frequently occur across different testing cycles. This could include regression tests or checks for common functionalities. By recognizing these patterns, teams can target their automation efforts effectively.
Next, implementing AI-driven automation streamlines the testing process. These tools can execute tests faster and more accurately than human testers, reducing the likelihood of errors. It's crucial, however, to continuously monitor and optimize the performance of these automated tests. Regular assessments help ensure that the AI remains efficient and aligns with the evolving specifications of the software. Overall, automating repetitive testing not only improves productivity but also enhances the overall quality of products delivered to users.
- Step 1: Identifying repetitive scenarios
Identifying repetitive scenarios is the first step towards optimizing quality assurance processes with AI QA solutions. In any testing environment, there are often routine tasks that can be automated to improve efficiency and reduce the burden on QA teams. These repetitive scenarios could include standard test cases that are executed multiple times or recurring evaluation criteria. By pinpointing these areas, teams can streamline their workflows significantly.
To effectively identify these repetitive tasks, teams should analyze previous testing cycles and pinpoint common patterns. This involves compiling data from past evaluations, such as customer support calls or product usability sessions. For instance, identifying specific criteria used in call evaluations, such as issue resolution or customer education, helps in recognizing where automation can be applied. This clarity not only enhances productivity but also drives more accurate results, streamlining the QA workflow with the capabilities of AI-powered tools.
- Step 2: Implementing AI-driven automation
Implementing AI-driven automation is a crucial step for organizations eager to enhance quality assurance processes. AI QA solutions facilitate repetitive tasks that previously consumed significant manual effort, allowing teams to focus on more strategic objectives. By automating testing scenarios, organizations can achieve quicker insights, ensuring that products meet quality standards efficiently and effectively.
As you embark on implementing AI-driven automation, consider the following key actions: First, identify specific testing tasks that can benefit from automation. Second, configure your AI QA solutions to execute these tasks accurately. Lastly, monitor the performance of your automation systems to optimize workflows continually. Through this approach, you can not only improve the efficiency of your team but also reduce the potential for human error, ultimately enhancing the overall quality of your product. Embracing AI-powered automation will ensure your QA processes remain competitive and adaptable in a rapidly evolving landscape.
- Step 3: Monitoring and optimizing automation performance
Monitoring and optimizing automation performance is crucial to ensure that AI QA Solutions deliver maximum value. This step involves regularly tracking key performance indicators (KPIs) to assess the effectiveness of automated processes. With detailed monitoring, teams can identify any issues that arise, such as test failures or execution delays. This proactive approach helps to maintain high quality in software products.
Additionally, optimization follows monitoring and focuses on refining automation processes. By analyzing collected data, teams can pinpoint bottlenecks and make necessary adjustments. This might include fine-tuning algorithms or reconfiguring testing environments to enhance efficiency. Continuous improvement not only boosts performance but also reduces the risk of errors, leading to a more robust final product. Effective monitoring and optimization empower teams to adapt swiftly, ensuring that AI QA Solutions align with evolving business needs and technological advancements.
Reducing Human Error in Quality Assurance
In quality assurance, the goal is to deliver products that meet high standards of quality and performance. However, human errors can lead to costly mistakes, compromising the effectiveness of QA processes. Recognizing common human errors, such as miscommunication, oversight, and inconsistent standards, is the first step toward improvement. By addressing these mistakes, teams can enhance their overall QA outcomes.
Deploying AI-powered verification tools significantly reduces the likelihood of such errors. These AI QA solutions automate repetitive tasks, ensuring consistency and accuracy in data analysis. By employing machine learning algorithms, these tools can flag potential issues before they escalate, enabling teams to focus on higher-value tasks. Consequently, human involvement shifts from execution to oversight, resulting in fewer errors, improved quality, and ultimately, a more reliable product.
- Step 1: Recognizing common human errors
Recognizing common human errors is essential for enhancing quality assurance (QA) processes. Human mistakes can range from overlooking critical details to misinterpreting client requirements, leading to increased operational inefficiencies. Firstly, itโs important to identify frequent human errors in QA, such as miscommunication during team discussions or assumptions made about user needs without proper validation. These errors can compromise the integrity of evaluations and lead to unreliable outcomes.
Secondly, integrating AI QA solutions can significantly reduce such errors. By employing analytics-driven tools, teams can automate feedback loops, ensuring more accurate assessments against defined criteria. A systematic approach to understanding and addressing these common human errors allows organizations to enhance the reliability of their QA processes. Emphasizing the need for continuous training and improvement becomes crucial in achieving and maintaining high quality standards. Therefore, everyone involved must commit to refining their skills while leveraging AI-powered solutions to support their efforts effectively.
- Step 2: Deploying AI-powered verification tools
Deploying AI-powered verification tools marks a crucial advancement in quality assurance. This step involves integrating AI QA solutions that enhance the accuracy and efficiency of testing processes. First, organizations should assess their existing verification procedures to pinpoint areas where AI can make a significant impact. By replacing manual checks with automated systems, businesses can minimize errors and accelerate verification timelines.
Next, training the AI tools with historical data from previous QA cycles is essential. This data allows the systems to learn patterns and improve accuracy over time. With AI-powered verification tools deployed, teams can expect quicker turnaround times and superior quality outputs. In essence, this approach transforms quality assurance, empowering organizations to focus more on strategic initiatives rather than routine checks. Embracing AI technology not only boosts productivity but also fosters a culture of continuous improvement in quality assurance practices.
Extract insights from interviews, calls, surveys and reviews for insights in minutes
Enhancing Efficiency and Accuracy with AI QA Solutions
AI QA Solutions are transforming the quality assurance landscape by significantly enhancing both efficiency and accuracy. By leveraging advanced algorithms, these solutions can automate various aspects of the testing process, enabling teams to focus on higher-order tasks. For instance, intelligent bug detection utilizes predictive analytics to foresee potential issues before they arise, reducing downtime and improving product quality.
Furthermore, streamlining the QA process might involve mapping out existing manual workflows. Transitioning to AI-driven processes can seamlessly identify inefficiencies and optimize testing efforts. In utilizing AI QA Solutions, organizations not only accelerate their testing cycles but also achieve a heightened level of accuracy, ensuring a more reliable product launch. By incorporating these systems, teams can navigate challenges more effectively while continuously improving their quality assurance practices.
Intelligent Bug Detection and Prediction
AI QA Solutions have revolutionized the way we handle bug detection and prediction, enabling teams to identify issues before they escalate. This is achieved through advanced predictive analytics that utilize historical data to anticipate potential bugs in the software development lifecycle. By leveraging large datasets, AI can forecast problematic areas, helping teams prioritize their testing and quality assurance efforts.
In addition to predictive capabilities, AI solutions provide real-time bug detection that alerts developers as soon as a flaw is introduced. This immediate feedback loop drastically reduces the time spent on identifying and fixing bugs, leading to faster release cycles and higher software quality. The combination of these intelligent features allows organizations to streamline their QA processes, resulting in more efficient workflows and enhanced product reliability. Embracing these AI-driven methods positions teams to effectively navigate the complexities of modern software development.
- How AI powers predictive analytics
AI QA Solutions harness the power of predictive analytics to enhance quality assurance efforts significantly. By analyzing vast amounts of data, these solutions can foresee potential defects before they impact the final product. This proactive approach enables teams to address issues efficiently, ensuring higher quality outcomes with reduced time investment.
One major benefit of using AI in predictive analytics is its ability to detect patterns from historical data. Over time, the AI learns what common errors occur and adapts its algorithms to identify these scenarios preemptively. Additionally, it can simulate how changes might affect outcomes, allowing teams to make informed decisions. Employing these AI-driven predictive capabilities can streamline QA processes, minimize errors, and ultimately lead to improved product reliability.
This approach not only empowers teams but also maximizes customer satisfaction by delivering higher quality products consistently. Embracing AI in quality assurance is essential for organizations aiming to stay ahead in todayโs competitive landscape.
- Implementing real-time bug detection
Implementing real-time bug detection significantly enhances the software quality assurance process. By utilizing AI QA solutions, teams can pinpoint bugs as they occur, allowing immediate resolution. Unlike traditional methods, which often rely on manual testing and lengthy review cycles, AI-driven tools continuously monitor code. This proactive approach minimizes downtime and improves overall product reliability.
To effectively implement real-time bug detection, organizations should consider the following steps:
Define Bug Metrics: Establish clear metrics for what constitutes a bug and prioritize responses based on severity.
Integrate AI Tools: Implement smart algorithms that automatically analyze code changes, flagging potential issues before they escalate.
Utilize Feedback Loops: Create systems for developers to receive instant alerts on detected bugs, allowing for swift interventions.
By adopting these strategies, teams can foster a more responsive and dynamic approach to quality assurance, ultimately ensuring a smoother user experience.
Streamlining the QA Process through AI
Integrating AI into the Quality Assurance (QA) process can significantly enhance efficiency and accuracy. To streamline workflows, organizations begin by mapping out existing manual processes. Understanding where bottlenecks and redundancies occur is crucial in identifying areas for improvement. This initial assessment sets the stage for a smoother transition into AI-driven methodologies.
Once the existing processes are mapped out, teams can transition to AI-enhanced solutions. These AI QA solutions automate repetitive tasks, leading to quicker test cycles and more consistent results. Continuous performance monitoring ensures that these automated processes adapt and improve over time. Finally, measuring changes in efficiency and accuracy enables organizations to quantify the benefits of AI integration, fostering a culture of continuous improvement in QA practices. By embracing this modern approach, teams not only enhance their output but also contribute to a more agile development environment.
- Step 1: Mapping out manual QA processes
To effectively utilize AI QA Solutions, start by mapping out your current manual QA processes. This step is vital for identifying existing bottlenecks and inefficiencies within the system. Begin by documenting each step, from test case creation to execution, and evaluation. By thoroughly understanding these workflows, you can pinpoint tasks that are repetitive or prone to human error, setting the stage for AI integration.
Next, assess how automated testing could enhance these processes. Determine the specific pain points you encounter with manual methodsโsuch as time consumption or inconsistency in results. This analysis will illuminate where AI can deliver significant improvements, such as predictive analytics or real-time bug detection. By addressing these challenges systematically, teams can transition toward a more efficient, accurate QA process powered by AI. This foundation will ultimately empower future enhancements and ensure long-term success.
- Step 2: Transitioning to AI-enhanced processes
Transitioning to AI-enhanced processes is a pivotal step for teams aiming to improve their quality assurance (QA) efforts. Embracing AI QA Solutions allows organizations to automate labor-intensive tasks, ensuring higher efficiency and reduced error rates. Implementing AI-driven tools requires careful planning, so itโs essential to align existing processes with AI capabilities.
Start by surveying current manual QA workflows, identifying areas that can benefit from automation. Prioritize specific repetitive tasks and deploy AI solutions designed for that purpose. Once in place, monitor the performance of these AI-enabled processes closely. Measurement and analysis will help in understanding their impact on efficiency and accuracy, ultimately leading to enhanced quality assurance outcomes. Transitioning effectively signifies not just the adoption of technology, but a strategic shift towards innovation and excellence in quality management.
- Step 3: Measuring improvements in efficiency and accuracy
Measuring improvements in efficiency and accuracy is a pivotal aspect of assessing the effectiveness of AI QA solutions. To commence, it's essential to establish a baseline for both efficiency and accuracy before implementing any AI-driven tools. By capturing key performance indicators (KPIs) such as testing cycle time and error rates, organizations can transparently evaluate progress over time.
Once AI-powered solutions have been integrated, continuous monitoring is vital. This includes regularly analyzing the impact on productivity and accuracy via reports and data analytics. Effective AI QA tools provide real-time insights that can reveal trends or areas for improvement, ensuring your quality assurance team remains agile and adaptive. Regular feedback loops from users allow for adjustments that fine-tune performance, ultimately leading to a more reliable and efficient QA process. This systematic approach not only enhances outcomes but also reinforces the organization's commitment to quality and accuracy.
Top AI QA Tools for Modern Teams
Modern teams are increasingly turning to AI QA solutions to address their quality assurance challenges effectively. These tools offer automation capabilities that significantly reduce manual workload, enabling teams to focus on critical tasks. One key benefit of AI-powered QA tools is their ability to detect and predict bugs in real-time, thereby enhancing the overall accuracy and efficiency of the testing process.
Among the top AI QA tools available, several stand out in today's market. Applitools excels in visual testing, ensuring that applications look and function as intended across various devices. Testim streamlines automated testing with customizable workflows, making it easier for teams to deploy and maintain tests. Functionize emphasizes simplified test creation through AI, allowing users to focus on strategy rather than execution. Finally, Test.ai leverages machine learning to adapt tests dynamically, drastically improving adaptability. Each of these tools brings unique benefits, effectively equipping modern teams to tackle QA challenges head-on.
Insight7: Leading the AI QA Tools
In the dynamic world of quality assurance, Insight7 stands out as a front-runner in AI QA tools. Offering cutting-edge capabilities, Insight7 addresses the pressing needs of modern teams navigating complex testing scenarios. By automating repetitive testing tasks, it not only enhances efficiency but also significantly reduces human error, allowing teams to focus on deeper analytical insights.
Organizations increasingly recognize the value of AI QA solutions, particularly in streamlining their QA processes. By integrating AI-enhanced methods, teams can effectively map out existing workflows and transition to smarter processes. This transformation not only leads to improved accuracy but also accelerates the overall quality assurance timeline. As a leader in this arena, Insight7 empowers businesses to analyze customer conversations and insights at scale, ensuring they remain competitive in todayโs fast-paced environment.
- Overview of Insight7s capabilities
AI QA Solutions fundamentally reshape quality assurance by automating and enhancing traditional processes. These sophisticated tools streamline repetitive testing tasks, significantly reducing the workload on teams while ensuring high accuracy. By effectively identifying and automating repetitive scenarios, companies can focus on more complex and value-driven activities. This smarter approach not only improves efficiency but also enhances the overall quality of software products.
Moreover, these solutions minimize human error by implementing AI-driven verification technologies. By recognizing common human pitfalls and utilizing predictive analytics, organizations can ensure that their products meet the highest standards. Transitioning from manual to AI-enhanced processes allows for continuous improvement, leading to a more streamlined QA process. Ultimately, embracing AI-powered quality assurance capabilities prepares organizations for future challenges and fosters innovation within teams seeking to improve their workflows.
Additional Tools to Consider
When exploring AI QA solutions, itโs essential to consider additional tools that can enhance your quality assurance process. Each tool offers unique functionalities capable of complementing your existing workflows. Here, we highlight a few noteworthy options to integrate into your strategy.
Applitools excels in visual testing, providing advanced algorithms for accurate UI validation. Its ability to detect visual changes across interfaces ensures that user experiences remain consistent. Testim, on the other hand, focuses on scalable automated testing, utilizing machine learning to optimize test creation and execution. For teams prioritizing end-to-end testing, Functionize provides a cloud-based platform that can adapt tests based on application behavior. Lastly, Test.ai employs AI to generate and maintain tests autonomously, significantly reducing the manual effort required.
Incorporating these AI-powered tools can not only streamline your processes but also redirect focus towards more strategic aspects of quality assurance. Explore these solutions further to elevate your QA capabilities.
- Tool 1: Applitools
AI QA Solutions have transformed the way quality assurance is conducted in software development. One notable tool in this space is Applitools, which specializes in visual testing and monitoring. This tool addresses challenges associated with manual testing by automating visual verification processes. It effectively identifies inconsistencies between expected and actual visual outputs across different devices and environments.
Applitools utilizes advanced image comparison technology, enabling users to quickly spot visual bugs that may not be detectable through traditional testing methods. This capability significantly reduces the time and effort involved in quality assurance, allowing teams to focus on enhancing user experience. Moreover, its integration with various development and testing frameworks enhances flexibility, making it a great fit for modern agile teams. By adopting this AI-powered tool, organizations can improve both the speed and accuracy of their QA processes, ultimately leading to a more robust and user-friendly product.
- Tool 2: Testim
Testim stands out as a powerful AI QA solution specifically designed to streamline and enhance quality assurance processes. By leveraging AI technology, Testim automates the testing of applications, allowing teams to focus on more strategic tasks. This tool enables users to create resilient tests effortlessly through a simple interface, making it accessible to both technical and non-technical team members.
One of the primary advantages of Testim is its ability to efficiently adapt tests to evolving applications. As changes are made to the software, Testim's AI capabilities ensure that test cases remain accurate and relevant, reducing the likelihood of false positives and negatives. This adaptability not only speeds up the testing process, but it also significantly lessens the burden on QA teams. Consequently, Testim exemplifies how AI-powered QA solutions can effectively address challenges related to test maintenance and reliability, paving the way for more robust software delivery.
- Tool 3: Functionize
AI QA Solutions have been pivotal in addressing common issues faced in quality assurance, particularly through innovative platforms. One such tool presents a user-friendly interface that democratizes access to quality insights. It allows team members without technical expertise to analyze customer interactions and generate actionable insights quickly. By simply uploading calls, users can visualize conversations, pinpoint common pain points, and understand customer behaviors without extensive training.
Moreover, the ability to derive insights from numerous conversations simultaneously enhances the organization's capacity to identify trends swiftly. This tool not only captures voice-of-customer data but also presents it in easily digestible summary formats, making it possible for teams to address issues proactively. Ultimately, implementing these AI-powered solutions guarantees improved efficiency and accuracy, making it an indispensable addition to modern quality assurance strategies.
- Tool 4: Test.ai
AI QA Solutions have significantly transformed quality assurance practices, making them more efficient and effective. Tool 4: Test.ai utilizes advanced machine learning algorithms to facilitate automated testing in software development, especially in mobile and web applications. This tool intelligently analyzes user behavior and adapts test scenarios accordingly, ensuring comprehensive coverage of potential usage patterns. By leveraging this predictive capability, development teams can enhance their test accuracy while decreasing the manual efforts typically associated with QA processes.
The platform also addresses challenges like human error and repetitive tasks. By automating these areas, it allows testers to focus on strategic issues that require human insight, rather than mundane testing routines. Furthermore, Test.ai streamlines collaboration between development and QA teams, fostering a dynamic workflow that speeds up product launches and improves overall software quality. Embracing AI-powered solutions like Test.ai ensures your approach to QA is not only modern but also robust enough to meet evolving market needs.
Conclusion: Embracing AI QA Solutions for Future Success
The journey towards embracing AI QA solutions is pivotal in ensuring sustained success for modern organizations. By addressing challenges like human error, inefficiency, and the complexities of repetitive tasks, these tools offer transformative benefits. Automating testing processes serves not just to save time, but also to enhance accuracy by reducing variability caused by human oversight.
As we look to the future, integrating AI-powered QA tools seamlessly into existing workflows become essential. This integration fosters an environment where quality assurance not only meets present demands but also anticipates future challenges. By adopting AI QA solutions today, organizations position themselves at the forefront of innovation and operational excellence, paving the way for long-term success.