5 Ways AI is Changing the Role of QA Analysts
-
Bella Williams
- 10 min read
The introduction of AI technology into the world of quality assurance is reshaping traditional approaches. The AI-Driven QA Evolution signifies a transformational shift where automation enhances accuracy and efficiency. QA analysts find themselves at a crossroads, where the interplay between human insight and AI capabilities leads to novel ways of ensuring quality in products.
As AI tools streamline repetitive tasks, QA analysts can redirect their focus toward strategic analysis. This shift allows them to cultivate deeper insights into product performance and user experience. In embracing this evolution, QA professionals are not just adapting; they are becoming essential players in fostering continuous quality improvement across various domains.
Analyze & Evaluate Calls. At Scale.
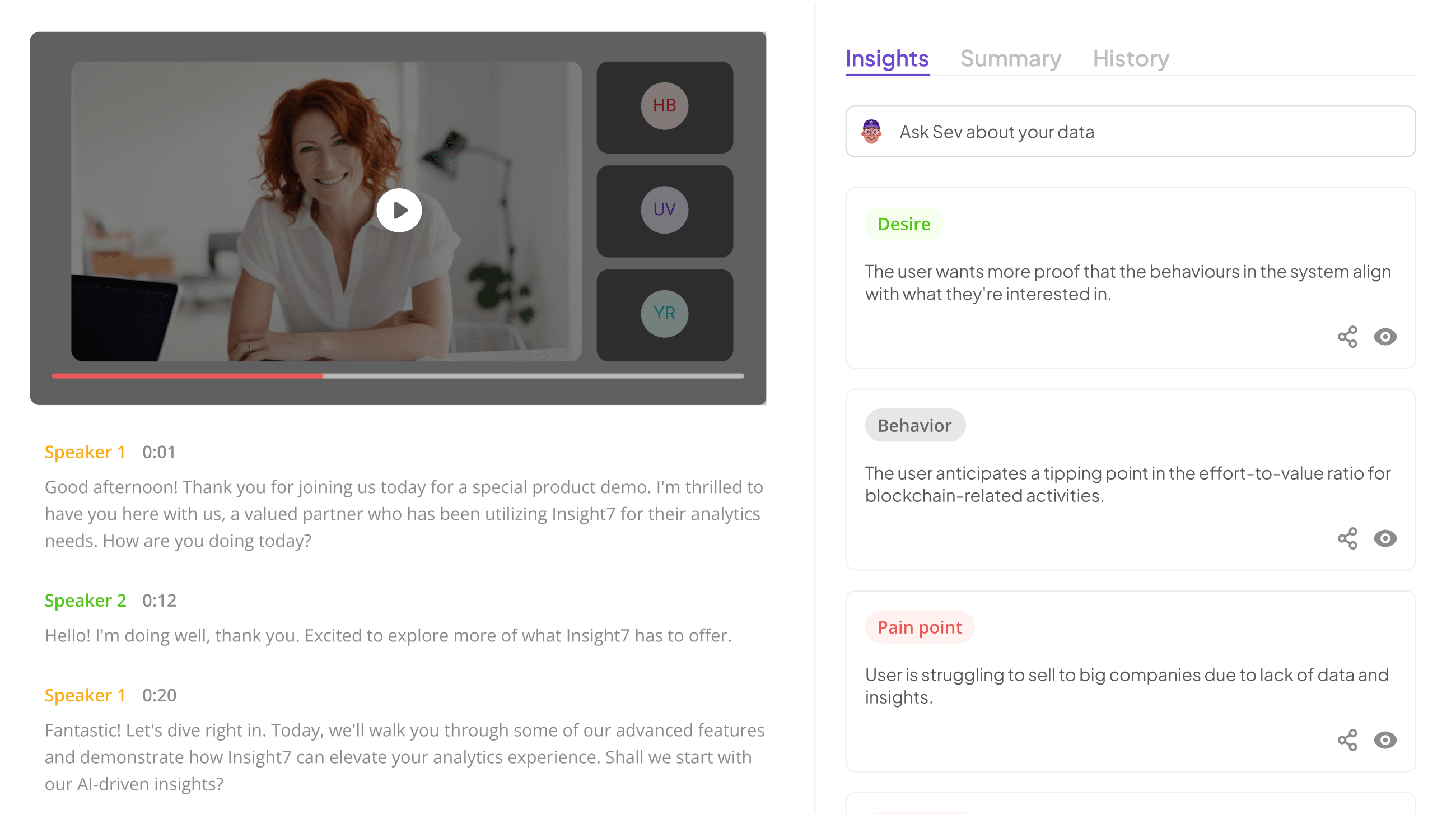
The AI-Driven QA Evolution: Transforming Traditional Testing
The AI-Driven QA Evolution is redefining how quality assurance analysts approach their work, transitioning from manual methods to more automated processes. Traditional testing often relied heavily on human effort, which could be both time-consuming and prone to errors. With the advent of AI technologies, repetitive tasks are now performed with remarkable speed and accuracy. This shift not only enhances productivity but also allows analysts to redirect their efforts toward more strategic initiatives.
Moreover, AI tools are improving test coverage through advanced algorithms that identify gaps in testing processes. By analyzing vast amounts of data, these tools offer insights that humans might easily overlook. Consequently, QA analysts can focus on delivering high-quality outcomes while fostering a culture of continuous improvement. The AI-Driven QA Evolution is not merely a trend; itโs a transformative force that is reshaping the landscape of quality assurance analysis, leading to smarter business practices and enhanced product reliability.
Automating Repetitive Tasks with AI
AI-Driven QA Evolution encompasses the ability to automate repetitive tasks that consume valuable time for QA analysts. Automation tools can take over mundane responsibilities, allowing professionals to concentrate on more strategic functions. Tasks such as test case execution, data collection, and bug tracking can now be handled swiftly and accurately by AI systems, significantly reducing human error and increasing efficiency.
By employing AI, QA analysts can not only streamline their workflows but also adapt to the rapid development cycles seen in software projects today. Automation allows for faster feedback loops, enabling teams to respond quickly to issues and enhance product quality. As tasks become automated, analysts can focus on interpreting results and making data-driven decisions. This evolution marks a significant shift in the QA landscape, transforming traditional roles and contributing to ongoing improvements in quality assurance practices.
Enhancing Test Coverage Through AI Algorithms
AI-Driven QA Evolution emphasizes how artificial intelligence can significantly enhance test coverage through its advanced algorithms. AI algorithms quickly analyze vast datasets, identifying potential gaps that traditional testing might overlook. This capability allows QA analysts to see a broader range of issues, thereby increasing overall software reliability and performance.
Further, AI can prioritize test cases based on risk assessment and historical data, ensuring that high-risk areas receive the most attention. By automating test generation and execution, QA teams can significantly reduce the time spent on manual testing. These advancements not only enhance test coverage but also provide QA analysts with the insights required to make informed decisions, ultimately leading to superior product quality. Embracing AI technology equips teams with the tools necessary to adapt in the rapidly evolving digital environment, marking a pivotal moment in the QA evolution.
Extract insights from interviews, calls, surveys and reviews for insights in minutes
AI-Driven QA Evolution: Empowering QA Analysts
AI-Driven QA Evolution has ushered in a transformative phase for QA analysts, empowering them to enhance their roles significantly. As AI continues to automate repetitive tasks, analysts can shift their focus from mundane processes to strategic analysis. This shift allows them to identify trends, improve test coverage, and enhance overall product quality.
Further, AI tools promote continuous quality improvement by providing real-time feedback and insights. This capability enables QA analysts to foresee potential issues before they escalate, ensuring smoother operations. As a result, QA analysts become vital players in the development lifecycle, bridging the gap between technical specifications and user expectations. Through this evolution, they are not just testers but strategic partners, enhancing collaboration and driving business success. The AI-Driven QA Evolution empowers analysts to deliver more value to their teams and the organization as a whole.
Shifting Focus to Strategic Analysis
As the role of QA analysts transforms, a significant shift is occurring toward strategic analysis. This evolution encourages QA professionals to look beyond merely identifying defects in software. By embracing an AI-driven approach, analysts are empowered to extract meaningful insights from data, enabling a more comprehensive understanding of user experiences and pain points.
In this new landscape, QA analysts are taking on roles that involve synthesizing data to inform business decisions. They focus on understanding trends that influence product development, customer satisfaction, and overall quality improvement. By analyzing data patterns, these professionals can predict potential issues and recommend solutions that enhance both user experience and product viability. In this way, QA's shift toward strategic analysis contributes to a more holistic approach to quality assurance, positioning analysts as vital contributors to long-term business success.
Promoting Continuous Quality Improvement
AI-driven QA evolution emphasizes the importance of continuous quality improvement in software testing. By integrating AI technologies, QA analysts can now monitor and assess quality in real-time, leading to faster identification of defects and enhanced overall product functionality. This shift not only allows for more proactive quality management but also encourages a culture that values ongoing enhancement of processes and products.
To promote continuous quality improvement effectively, consider the following key aspects:
- Real-Time Feedback: Utilize AI tools to provide immediate insights into testing progress and quality levels, enabling quicker adjustments when necessary.
- Data-Driven Decisions: Embrace AI for analyzing vast datasets to uncover patterns and trends that inform future testing strategies and design improvements.
- Collaborative Tools: Implement collaborative platforms where testers can share AI-generated insights and foster discussions around continuous improvements.
By assimilating these practices, organizations can ensure their QA processes keep pace with evolving technology and customer expectations, demonstrating the transformative power of AI-driven QA evolution.
Conclusion: The Future of QA in the AI-Driven QA Evolution
As we look towards the future of QA in the AI-Driven QA Evolution, it becomes clear that adaptability will be crucial. QA analysts are transitioning from routine verification tasks to strategic roles, ensuring that AI technologies enhance overall quality. Automation tools are not simply replacements but powerful aids that complement human insight and creativity, enabling analysts to focus on complex problem-solving.
The integration of AI will continue to shape the QA landscape, encouraging analysts to engage in more thoughtful analysis and continuous improvement practices. Ultimately, embracing this evolution will empower QA professionals to lead efforts in fostering higher quality and innovation within their organizations, ensuring that they remain indispensable in an increasingly automated world.
Analyze & Evaluate Calls. At Scale.
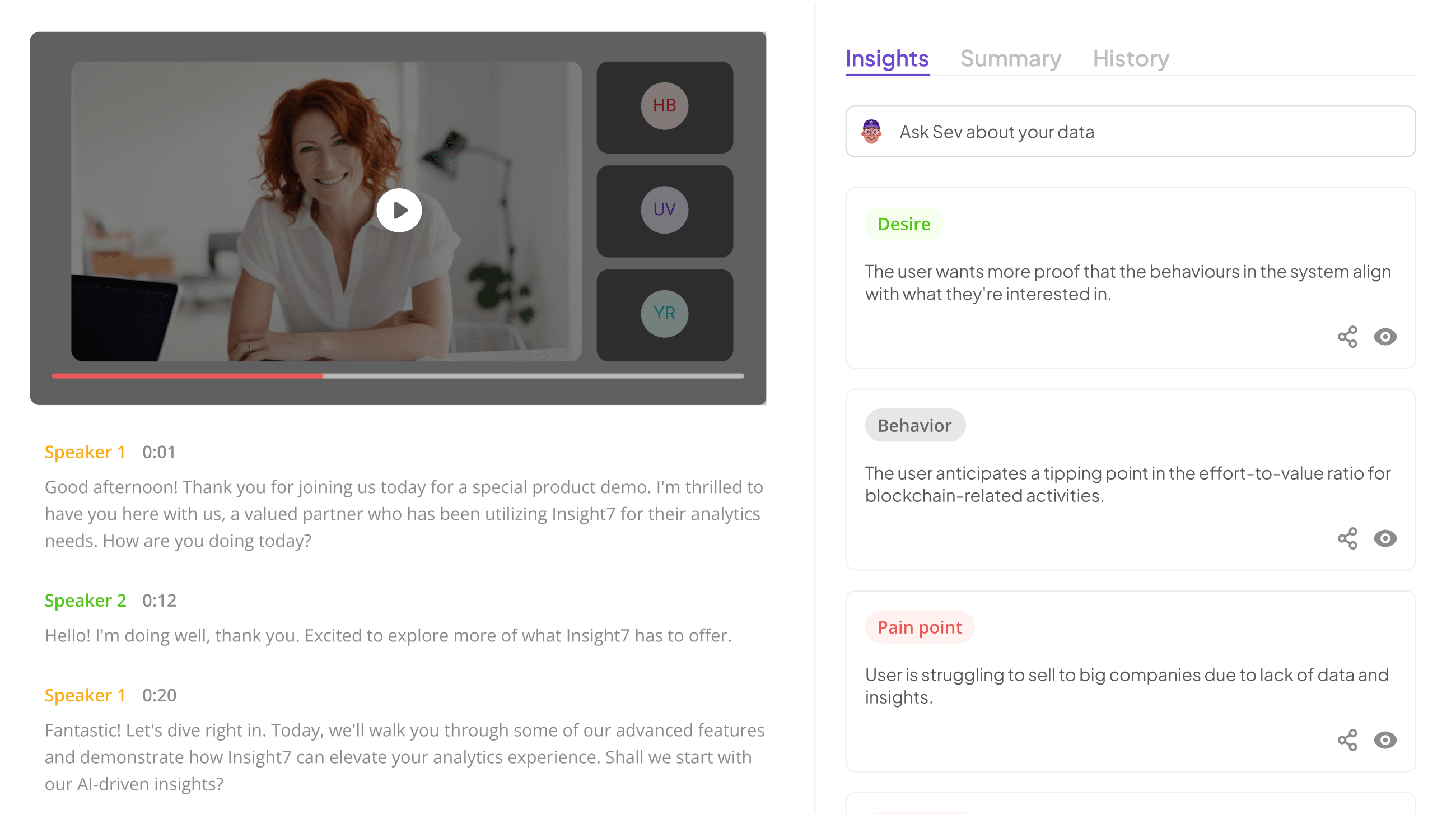