AI QA Efficiency has revolutionized quality assurance by streamlining processes and enhancing accuracy. In an era where rapid decision-making is crucial, organizations are turning to AI to outperform traditional manual reviews. This shift is not merely about adopting new technologies; it signifies a fundamental change in how quality is managed and assessed at scale.
Understanding AI QA Efficiency means recognizing the potential to handle vast amounts of data with minimal human intervention. This capability addresses the challenges of volume while maintaining high standards of consistency. As we explore the five reasons AI-backed QA surpasses manual review, it’s essential to grasp how these systems create a more effective, efficient, and reliable quality assurance environment.
Analyze qualitative data. At Scale.
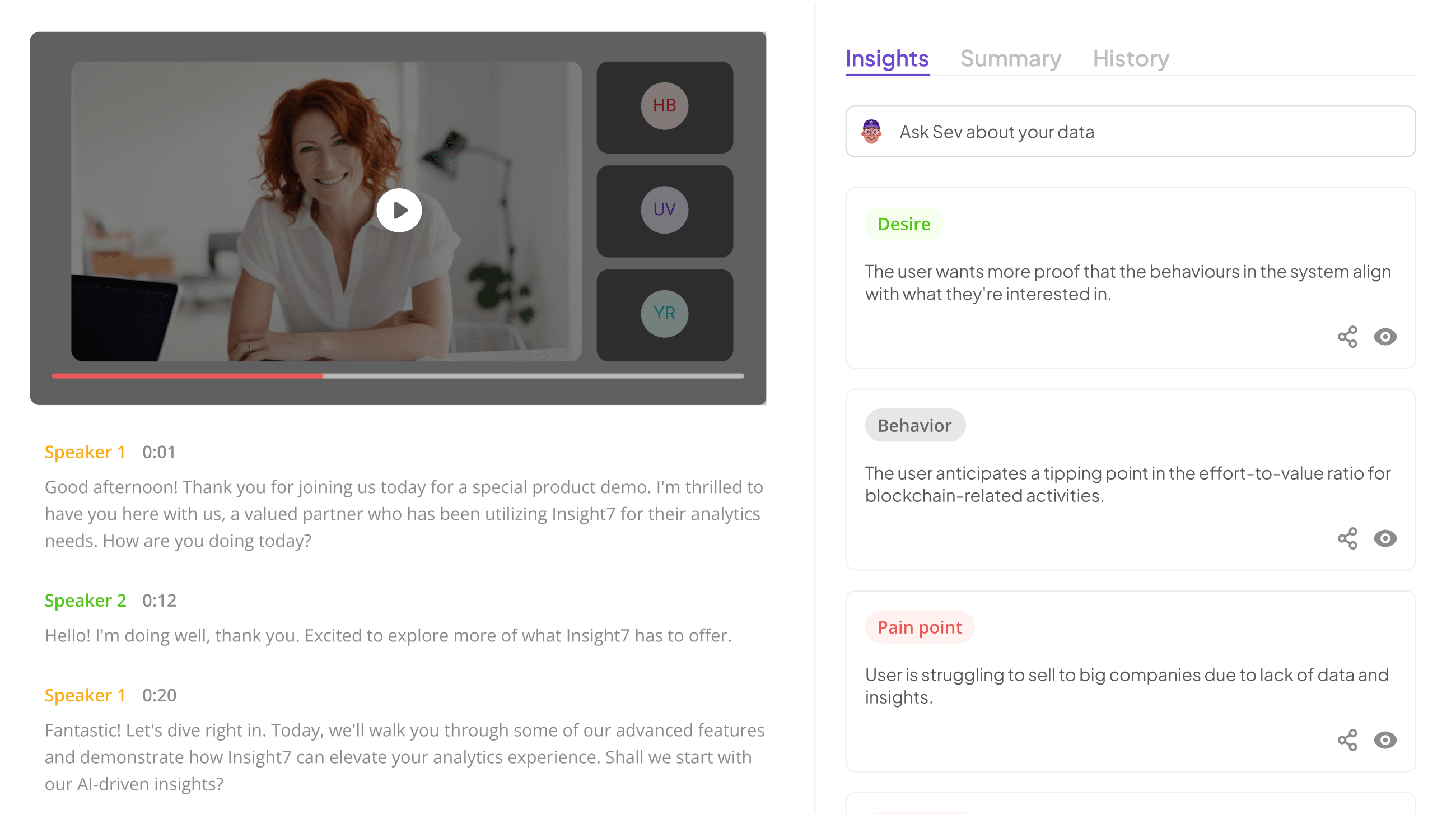
The Power of AI QA Efficiency in Quality Assurance
AI QA Efficiency transforms traditional quality assurance by streamlining processes and enhancing performance. When organizations implement AI in QA, they significantly accelerate review cycles, resulting in quicker feedback and improved response times. This swift pace not only meets growing demands but also ensures that quality standards are consistently upheld. The incredible ability of AI to learn from past data enables it to detect patterns and anomalies that human reviewers might overlook, raising overall efficiency.
Furthermore, AI tools facilitate a standardized approach to quality assurance. With machine learning algorithms analyzing data sets, the potential for human error diminishes considerably, ensuring more reliable outcomes. As a result, businesses benefit from higher accuracy rates and better compliance with industry regulations. Ultimately, the synergy of speed, consistency, and precision offered by AI QA Efficiency redefines how organizations approach quality assurance, allowing them to thrive in competitive environments while maintaining exceptional standards.
Accelerating QA Processes
AI QA Efficiency plays a crucial role in accelerating quality assurance processes. By automating routine tasks, AI significantly reduces the time it takes to complete assessments. This means teams can focus on more strategic work while still ensuring high standards. Furthermore, AI systems analyze data at remarkable speeds, allowing for quicker identification of errors and issues that may not be apparent to human reviewers.
In addition to speed, AI capabilities enhance thoroughness and form a comprehensive view of quality metrics. The integration of AI technology creates an agile QA environment where teams are empowered to respond swiftly to test results. As a result, products and services reach the market faster, thus enhancing overall efficiency. Embracing AI-backed quality assurance not only streamlines processes but also leads to more reliable outcomes, paving the way for continuous improvement and innovation in any organization.
Ensuring Consistent Accuracy
Ensuring consistent accuracy is paramount in any quality assurance process. AI-backed systems excel in this area by applying standardized criteria across all reviews. Unlike manual reviews, where subjective interpretations can lead to inconsistencies, AI utilizes precise algorithms to maintain a high level of reliability. This objectivity eliminates the potential for human error and bias, ensuring that quality metrics remain uniform.
Moreover, AI-driven tools continuously learn from data patterns, refining their accuracy over time. They analyze vast amounts of information in a fraction of the time it would take a human, providing actionable insights that enhance overall quality. By integrating real-time feedback mechanisms, these tools ensure that accuracy is not just consistent but also continually improving. In an environment where maintaining the highest standards is vital, AI QA efficiency stands out as the solution for delivering unparalleled accuracy at scale.
[optional: hfe_template id=22694]Leveraging AI QA Efficiency for Scale
AI QA Efficiency is a game-changer in the realm of quality assurance, particularly when it comes to scaling tasks. By automating analysis and review processes, AI significantly increases operational speed. Organizations can handle substantial volumes of data efficiently, eliminating bottlenecks that often come with traditional methods. This not only allows for timely decision-making but also ensures responsiveness to customer needs.
Moreover, the implementation of AI reduces human error, a common issue in manual reviews. While human insights are invaluable, AI can continuously learn from data patterns, providing insights that are consistently reliable. As companies strive to enhance their QA processes, focusing on AI QA Efficiency enhances accuracy and scalability, ultimately leading to greater customer satisfaction and business success. This transformative approach empowers teams to focus on strategic initiatives rather than being bogged down by repetitive tasks.
Handling Large Volumes of Data
Handling large volumes of data can present significant challenges for businesses, especially when relying on traditional manual review methods. AI QA efficiency allows organizations to process extensive datasets rapidly and accurately, significantly reducing the time and resources needed. With machine learning algorithms, AI tools can quickly identify patterns, flagging anomalies and ensuring consistent quality across large data sets that would overwhelm manual reviewers.
Moreover, AI's ability to continuously learn from previous inspections enables it to adapt and improve its processes over time. Unlike human reviewers, who may experience fatigue and oversight, AI systems maintain high vigilance, ensuring that no detail goes unnoticed. This capability is vital as data volumes continue to grow, making it essential for organizations to adopt AI-backed quality assurance methods to keep pace efficiently. Transitioning to this advanced approach not only enhances data handling but also unlocks new opportunities for insights and decision-making.
Reducing Human Error
Human error is a common issue that can significantly impact the quality of work, especially in quality assurance. When tasks rely on manual review, inconsistency and oversight are almost inevitable. In contrast, AI QA efficiency minimizes human involvement, thereby reducing the chances of mistakes. By automating routine checks, organizations can ensure a consistent and reliable assessment of quality at scale.
Furthermore, AI systems are designed to learn from past errors, which means they continually improve over time. This adaptability makes them far more reliable than human reviewers who may be prone to fatigue or distraction. Consequently, the incorporation of AI not only expedites the process but also enhances the overall accuracy of quality checks. As such, organizations embracing AI-backed QA can mitigate risks associated with human error, fostering a more efficient and dependable system.
Conclusion: Embracing the Future with AI QA Efficiency
As we conclude our exploration of AI QA efficiency, it’s clear that embracing this innovation transforms the future of quality assurance. AI systems streamline the review process, making it faster and more accurate than traditional methods. This shift not only enhances productivity but also minimizes human error, enabling teams to focus on higher-value tasks.
The potential of AI in quality assurance is vast, promising not just efficiency but also improved insights into performance metrics. By adopting AI-driven solutions, organizations can better navigate the complexities of large-scale data reviews. Embracing AI QA efficiency is not just an option; it is a crucial step towards a smarter, more effective operational future.