5 QA Scorecard Enhancements Using AI Analysis
-
Bella Williams
- 10 min read
AI-Driven QA Enhancements have transformed the landscape of quality assurance, revolutionizing how businesses assess their performance. As organizations strive for excellence, integrating AI into QA processes offers invaluable insights and efficiency. With the ability to analyze vast amounts of data quickly, AI helps teams identify trends and anomalies that traditional methods may overlook, ultimately fostering better decision-making.
By focusing on real-time feedback, AI empowers QA teams to address issues immediately, reducing response times and enhancing overall quality. These enhancements not only improve accuracy but also streamline workflows, paving the way for more effective quality assurance practices. In this document, we will explore five key enhancements that leverage AI analysis to elevate QA scorecards to new heights.
Analyze & Evaluate Calls. At Scale.
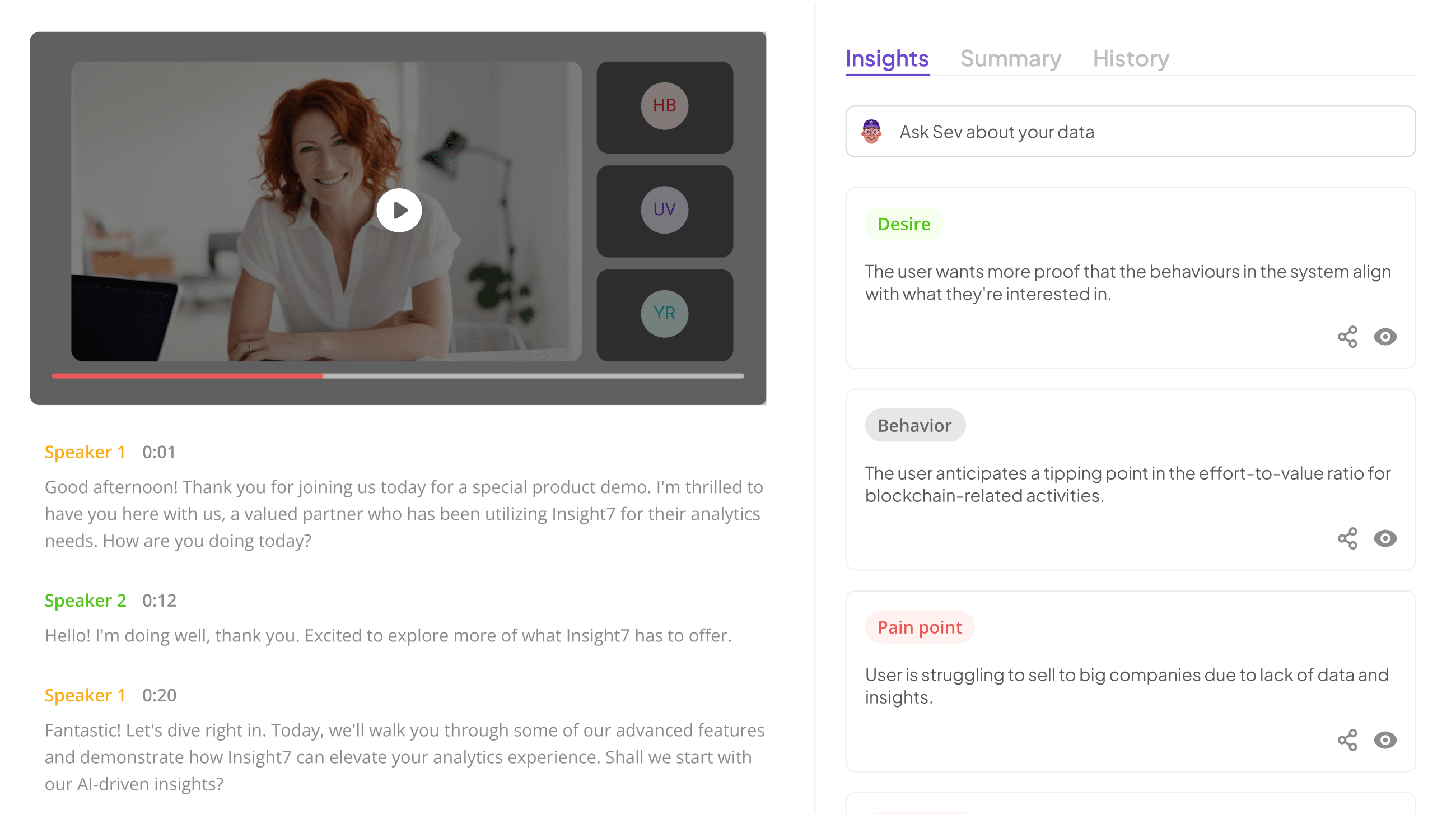
Unleashing the Power of AI in QA Scorecards
AI-driven QA enhancements offer a transformative approach to quality assurance scorecards, significantly increasing their effectiveness. By harnessing the analytical power of artificial intelligence, organizations can uncover intricate patterns that traditional methods might overlook. This improved accuracy can lead to more reliable insights, ensuring that every evaluation reflects the true quality of service or product delivery. Ultimately, this means that QA processes can become more robust, allowing for informed decision-making.
Additionally, AI capabilities extend to real-time feedback, enabling teams to address issues as they arise. AI systems can analyze data during QA assessments, identifying potential defects almost instantaneously. By facilitating quicker adjustments in workflow or performance, organizations reduce the time taken to rectify deficiencies. Embracing these enhancements not only streamlines operations but also fosters a culture of continuous improvement and accountability in quality assurance practices.
Leveraging AI-Driven QA Enhancements for Better Accuracy
AI-Driven QA Enhancements significantly improve the accuracy of quality assurance processes. By integrating advanced algorithms, these enhancements identify complex patterns and insights that traditional methods often overlook. For instance, machine learning models analyze historical data to refine QA processes, ensuring assessments are consistently reliable. This capability allows organizations to make informed decisions based on comprehensive analysis, increasing overall accountability in their quality evaluations.
Moreover, the implementation of AI in QA promotes a proactive approach to quality management. Real-time data analysis enables teams to quickly detect issues and make adjustments before they escalate. This swift response to defects enhances not only the quality of output but also fosters a culture of continuous improvement. As AI evolves, its application will only deepen, further transforming how organizations assess and ensure the quality of their products and services.
- Discuss how AI can improve the accuracy of QA scorecards by identifying patterns and insights that are not easily visible through traditional methods.
AI can significantly enhance the accuracy of QA scorecards by uncovering patterns and insights that traditional methods may overlook. By harnessing advanced algorithms, AI systems can analyze large datasets quickly, revealing correlations between various performance metrics. For instance, AI can identify common mistakes made by agents during calls, which might not be apparent through manual evaluations. This capability not only informs scorecard scores but also highlights areas where training or adjustments are needed.
Moreover, AI can continuously learn from ongoing interactions, adapting its analysis as new data is introduced. This means that the QA scorecards become increasingly reliable over time, providing an objective basis for performance assessment. By automating the identification of trends, organizations can streamline their quality assurance processes, ensuring a more consistent and precise evaluation of agent performance. AI-driven methods foster a deeper understanding of team dynamics and customer interactions, leading to more informed strategic decisions.
- Explain the role of machine learning models in refining QA processes to ensure more accurate quality assessments.
Machine learning models play a pivotal role in refining QA processes to ensure more accurate quality assessments. By analyzing vast amounts of data, these models can identify trends and patterns that might go unnoticed through traditional evaluation methods. This capability leads to a more nuanced understanding of quality metrics, enhancing the effectiveness of AI-Driven QA Enhancements. Consequently, organizations can fine-tune their evaluation criteria and focus on areas that need the most attention.
Moreover, machine learning algorithms continuously learn and adapt from new data inputs. As they process feedback from past assessments, they improve their accuracy over time. This iterative learning process allows for a dynamic approach to quality assurance, making it easier to spot potential issues before they escalate. Embracing AI technology in QA fosters a proactive mindset, enabling teams to deliver higher quality outputs and meet customer expectations more effectively.
AI-Driven QA Enhancements in Real-time Feedback
AI-driven QA enhancements in real-time feedback revolutionize how quality assurance teams operate. By harnessing the power of artificial intelligence, organizations can receive instant feedback during the QA processes, significantly increasing efficiency. This real-time capability allows for immediate identification of issues, enabling teams to make swift adjustments.
Moreover, AI analysis identifies patterns in data that traditional methods might overlook. This enhances the overall quality assessments by minimizing the time between detecting defects and implementing solutions. As a result, QA teams are empowered to deliver more effective and timely results. Embracing AI-driven QA enhancements not only streamlines operations but fosters a proactive approach to maintaining quality standards, ultimately driving improved outcomes for all stakeholders involved.
- Explore the capability of AI to provide instant feedback during QA processes, thereby increasing efficiency and effectiveness.
In today's fast-paced environment, the capability of AI to provide instant feedback during QA processes is transformative. With AI-driven QA enhancements, organizations can dramatically improve both efficiency and effectiveness. Real-time data analysis ensures that feedback is immediate, allowing teams to make timely adjustments and rectify defects without delay. This swift response contributes to a seamless workflow, preventing bottlenecks commonly experienced in traditional QA processes.
Furthermore, AI's predictive capabilities help identify potential issues before they escalate, streamlining communication among team members. By harnessing AI insights, teams can make informed decisions based on actionable data. This not only boosts productivity but also enhances the overall quality of outcomes, fostering a culture of continuous improvement. Ultimately, integrating AI into QA processes not only strengthens scorecard accuracy but also advances the organization's commitment to excellence and customer satisfaction.
- Detail how real-time data analysis allows for quicker adjustments and reduces the time between defect identification and resolution.
Real-time data analysis plays a pivotal role in enhancing the efficiency of Quality Assurance (QA) processes. By utilizing AI-driven analytics, teams can instantly detect defects during various stages of development. This immediate visibility allows for faster adjustments and mitigates potential setbacks before they escalate. Instead of waiting for periodic reports, stakeholders gain insights in real-time, enabling proactive decision-making.
Moreover, the swift identification of defects significantly reduces the time needed to resolve issues. With automated alerts and actionable insights, teams can prioritize fixes and allocate resources more effectively. This streamlined approach not only enhances product quality but also fosters a culture of continuous improvement. Embracing real-time data analysis ultimately leads to better collaboration among team members, ensuring that solutions are implemented swiftly and effectively. Leveraging these AI-driven QA enhancements is key to optimizing operational efficiency and maintaining high standards in product development.
Extract insights from interviews, calls, surveys and reviews for insights in minutes
Tools Revolutionizing AI-Driven QA Enhancements
In today's dynamic landscape, various tools are emerging to drive AI-Driven QA Enhancements. Such advancements focus on improving quality assurance processes by harnessing the power of artificial intelligence. These tools not only streamline workflows but also provide deeper insights that traditional methods struggle to uncover. With AI's ability to analyze vast amounts of data, organizations can gain immediate feedback and adjust their strategies in real time.
Several key tools are making significant strides in this domain. First, Qualitest employs AI for comprehensive testing, ensuring every aspect of quality is meticulously evaluated. Next, Testim.io enhances automation with its AI-driven testing features, simplifying the scorecard process and boosting overall efficiency. Similarly, Applitools leverages visual validation powered by AI to ensure that even the smallest interface nuances are accurately captured. Lastly, Tricentis integrates AI analytics to further refine testing processes and elevate QA effectiveness. These tools collectively shape a new era of AI-Driven QA Enhancements, streamlining quality assurance and fostering continuous improvement.
Insight7 as a Leading Tool for AI-Driven QA Enhancements
As organizations strive for excellence in quality assurance, Insight7 stands out as a leading tool for AI-driven QA enhancements. By integrating advanced artificial intelligence techniques, it enables seamless data analysis at scale, transforming customer insights into actionable quality metrics. This platform addresses the limitations of traditional methods, which often struggle to keep pace with the surge in customer signals generated today. By allowing teams to analyze customer interactions more effectively, it enhances their ability to extract valuable insights.
Insight7 also excels in its user-friendly design, promoting ease of use for teams across various sizes and sectors. With its focus on real-time feedback, it empowers organizations to identify issues quickly, reducing the time between defect detection and resolution. This responsiveness not only improves efficiency but also fosters collaboration by consolidating insights into a single source. Thus, utilizing Insight7 for AI-driven QA enhancements significantly elevates the quality assurance process, setting a new standard for efficiency and accuracy.
- Introduce Insight7 as a prominent tool offering AI-based solutions to enhance QA scorecards.
AI-Driven QA Enhancements are transforming how businesses approach Quality Assurance, particularly through tools that utilize advanced analytics for optimal performance. One such prominent tool is designed to enhance QA scorecards by integrating AI-based solutions. This platform simplifies complex data analysis and provides insightful feedback, enabling organizations to identify patterns and anomalies quickly.
With a self-service interface, the tool empowers users to analyze customer conversations efficiently. By automating tedious manual tasks, it helps streamline the translation of insights into actionable strategies. Furthermore, collaboration becomes more effective as insights are consolidated in one accessible space, eliminating scattered information that hampers decision-making. Ultimately, leveraging such an AI-driven solution can significantly boost QA processes, helping organizations stay agile and competitive in a fast-paced market.
Explore Other Key Tools
In the realm of AI-driven QA enhancements, exploring various tools can transform your approach to quality assurance. These tools leverage advanced artificial intelligence to automate testing processes, providing deeper insights into data that were previously challenging to extract. By embracing AI technologies, businesses can streamline their quality assessment practices and gain a competitive advantage in performance measurement.
Consider Qualitest, which integrates AI to deliver comprehensive testing solutions, thus ensuring thorough quality checks. Testim.io enhances automation through its AI-powered testing features, resulting in higher quality scorecards. Additionally, Applitools focuses on visual validation, using AI to ensure the accuracy of visual components, while Tricentis applies AI analytics to improve the efficiency of QA workflows. Each of these tools contributes to a more effective quality assurance strategy, ultimately leading to substantial improvements in QA scorecards through AI-driven enhancements.
- Qualitest
Qualitest exemplifies the transformative capabilities of AI in quality assurance processes. By harnessing AI technology, Qualitest significantly enhances testing and evaluation services, ensuring that businesses can maintain high-quality standards. Utilizing machine learning algorithms, it analyzes vast datasets to identify patterns that would remain unnoticed through conventional methods.
๐ฌ Questions about 5 QA Scorecard Enhancements Using AI Analysis?
Our team typically responds within minutes
This process not only improves the overall accuracy of assessments but also allows for tailored solutions based on specific industry needs. By integrating AI-driven QA enhancements, teams can receive immediate feedback, making real-time adjustments possible during the evaluation process. This agility ultimately minimizes the time required to identify defects and implement resolutions, promoting a more efficient workflow. With Qualitest leading the charge, organizations can expect a future where quality assurance evolves continuously, driven by intelligent analysis and data-driven decisions.
- Discuss how Qualitest utilizes AI for comprehensive testing and QA services.
Utilizing AI-driven QA enhancements transforms the quality assurance landscape by introducing innovative methodologies. By harnessing AI technologies, organizations streamline various testing processes, ensuring comprehensive evaluation and better quality outcomes. One significant advantage is AI's capability to analyze vast datasets rapidly, identifying trends that are often imperceptible to traditional techniques. This deeper analysis allows for more informed decision-making during QA assessments.
Moreover, the automation of routine tasks through AI technologies minimizes human error and accelerates testing cycles. AI systems can learn from past evaluations, adapting and refining algorithms to improve their effectiveness continuously. Additionally, real-time feedback mechanisms enable timely adjustments in quality assessments, enhancing overall efficiency. By integrating AI into QA practices, organizations can achieve higher accuracy and significantly reduce the time taken to resolve defects, promoting a culture of continuous improvement in quality assurance.
Employing AI-Driven QA Enhancements within quality assurance processes introduces a transformative approach to evaluating performance metrics. By leveraging advanced algorithms and machine learning models, these enhancements can uncover intricate patterns that traditional methods often overlook. This increased accuracy lies in the ability of AI to analyze vast amounts of data swiftly, providing clearer insights into agent performance and customer interactions.
Moreover, real-time feedback powered by AI significantly elevates the QA process. With instant evaluations, organizations can adapt strategies promptly, minimizing the delay between identifying issues and implementing solutions. This transformative effect not only boosts operational efficiency but also enhances customer satisfaction. The future of quality assurance lies in embracing these AI-driven enhancements, leading to more precise assessments and ultimately a superior customer experience. Embracing AI in quality assurance processes will be paramount to staying competitive in this ever-evolving landscape.
- Testim.io
Testim.io stands out as a significant player in the realm of AI-driven QA enhancements. The platform leverages intelligent automation to streamline the testing process, allowing teams to focus on strategic improvements rather than mundane tasks. By automating repetitive testing procedures, it not only boosts efficiency but also increases accuracy in quality assessments, ensuring that valuable insights are not overlooked.
The power of Testim.io lies in its ability to provide real-time feedback and adapt testing scenarios on the fly. This flexibility enhances the scoring metrics on QA scorecards by making them more responsive to emerging data and user interactions. As a result, organizations can swiftly rectify issues, thereby fostering a culture of continuous improvement. Ultimately, the integration of such AI-driven tools revolutionizes traditional QA, paving the way for more precise and effective evaluation methods.
- Highlight Testim.ios AI-powered testing features that enhance automation and improve scorecard quality.
Testim.io harnesses AI-powered features that significantly enhance the automation and quality of QA scorecards. By automating repetitive tasks, Testim.io allows teams to focus on higher-value activities. This efficiency is critical as it speeds up the overall testing process and minimizes human error, ensuring that quality assessments are both accurate and consistent.
Furthermore, AI-driven insights from Testim.io provide valuable data on software performance. The platform automatically identifies patterns and anomalies in testing outcomes, allowing for immediate adjustments. This capability not only improves the time-to-market for products but also elevates the overall quality of deliverables, leading to enhanced confidence in QA scorecards. The combination of automation and intelligent insights exemplifies how integrating AI in QA processes can lead to substantial improvements in accuracy and reliability, making it an essential component in todayโs software development landscape.
- Applitools
Applitools serves as a prime example of how AI-driven QA enhancements can transform quality assurance processes. By incorporating visual validation, it significantly improves the accuracy of QA scorecards. This technology focuses on identifying visual discrepancies that traditional testing methods might overlook, ensuring that user interfaces match design specifications perfectly.
The role of Applitools extends beyond simple visual checks. By utilizing machine learning algorithms, it analyzes vast amounts of data to learn from previous tests, making the QA process more efficient and reliable. It also enables teams to automate feedback loops effectively, which allows for quicker iterations and higher-quality outcomes. These capabilities highlight the significant contributions of AI in delivering robust QA enhancements, ultimately leading to products that meet and exceed user expectations.
- Examine Applitoolsโ use of AI-driven visual validation to boost QA scorecards.
Applitools employs AI-driven visual validation effectively, enhancing QA scorecards in substantial ways. By using advanced algorithms, it identifies visual discrepancies between expected and actual outputs, ensuring that every element of the user interface meets specified standards. This approach is crucial for maintaining a high-quality user experience, as even minor visual errors can significantly affect user perception.
The process begins with AI analyzing screenshots and comparing them against predefined visual baselines. Through this analysis, QA teams gain actionable insights that contribute directly to scorecard metrics. Moreover, automated visual testing reduces the time spent on manual reviews, allowing teams to focus on more complex testing scenarios. This real-time validation maximizes efficiency and accuracy, fostering a continuous improvement loop in quality assurance practices. Overall, employing AI-driven visual validation not only enhances the integrity of QA scorecards but also elevates the overall quality standard across various projects.
- Tricentis
Integrating AI analytics into Quality Assurance (QA) processes can significantly enhance scorecard outcomes. One prominent approach involves automating data analysis to streamline evaluations and provide rich insights into quality performance. Utilizing AI-driven capabilities allows for deep dives into call evaluations, identifying nuances that traditional methods may overlook. This leads to a comprehensive understanding of compliance and service delivery.
The application of AI also enables organizations to refine their criteria and evaluation templates dynamically. By leveraging automated systems, businesses can align their QA metrics with evolving industry standards and customer expectations. The result is a more adaptive QA strategy that not only boosts efficiency but also heightens the reliability of assessments. Therefore, incorporating AI-driven enhancements lays a foundation for transformative quality management, ensuring that feedback is timely, relevant, and actionable.
In summary, AI-driven QA enhancements offer a pathway to improved quality outcomes, empowering teams to deliver consistent and accurate performances while adapting swiftly to changing demands.
- Look at how Tricentis applies AI analytics to streamline and enhance QA processes.
The application of AI analytics has been a game-changer in streamlining and enhancing QA processes. With advanced algorithms, organizations can pinpoint inefficiencies and gaps that may have previously gone unnoticed. For instance, AI-driven analytics can automatically evaluate transcripts against established compliance criteria, ensuring quality checks are thorough and systematic. This automation reduces human error, allowing QA teams to focus on more strategic tasks rather than routine assessments.
Furthermore, AI analytics empowers teams to derive insights from large data sets quickly. By identifying patterns, trends, and anomalies, quality assurance processes become not only faster but also more precise. With the ability to provide real-time feedback, AI tools facilitate immediate adjustments in QA methodologies, further enhancing the reliability of outcomes. As these technologies continue advancing, the potential for even greater improvements in QA effectiveness and efficiency remains promising.
Conclusion: The Future of AI-Driven QA Enhancements
The future of AI-driven QA enhancements holds immense potential for redefining quality assurance processes. As organizations continue to adopt these technologies, the accuracy and efficiency of QA scorecards will significantly improve. By utilizing AI, teams can identify intricate patterns and insights, allowing for informed decision-making and targeted corrections. This shift enables a more proactive approach to quality management.
Looking ahead, AI-driven QA enhancements will likely evolve further, integrating deeper into existing frameworks. Ongoing improvements may include advanced machine learning algorithms and real-time analytics, which will facilitate quicker adjustments based on immediate feedback. Overall, embracing these innovations will be crucial for businesses seeking to maintain high-quality standards and competitive advantage.
- Summarize the impact of AI-driven strategies on the efficacy and efficiency of QA scorecards.
AI-driven strategies significantly enhance the efficacy and efficiency of QA scorecards, transforming traditional quality assurance processes. These advancements streamline data analysis, allowing for quicker insights into performance metrics that matter most. By employing sophisticated algorithms, AI can detect patterns in customer interactions and agent performance that human evaluators might overlook. This leads to more accurate assessments, resulting in actionable feedback that improves overall service quality.
Furthermore, AI technologies enable real-time monitoring, which reduces the time taken to identify and rectify errors. Rapid feedback loops ensure that agents receive timely insights into their performance, fostering continuous improvement. With AI-driven QA enhancements, organizations can expect more efficient evaluations that save time and resources, ultimately leading to improved customer satisfaction. This integration of technology not only optimizes workflows but also sets a new standard for quality assurance practices across industries.
- Predict the continued evolution and future trends of AI in Quality Assurance, emphasizing ongoing improvements and potential challenges.
The evolution of AI-Driven QA Enhancements promises notable advancements in quality assurance. Future trends indicate that AI will increasingly integrate with real-time data analytics, enabling organizations to identify flaws swiftly and adjust processes on the fly. As machine learning algorithms become more sophisticated, they will facilitate deeper insights into quality metrics, enhancing the accuracy of QA scorecards.
However, this evolution is not without challenges. Organizations must navigate concerns regarding data privacy and algorithmic bias, which can compromise the effectiveness of AI tools. Moreover, as AI assumes a more prominent role, the demand for skilled personnel capable of interpreting AI-generated insights grows. Balancing AI's automation with human oversight will be critical in maintaining the integrity of quality assurance processes. Embracing these advancements thoughtfully will provide organizations with a competitive edge in their quality assurance efforts.
๐ฌ Questions about 5 QA Scorecard Enhancements Using AI Analysis?
Our team typically responds within minutes