Introducing AI-enhanced QA evaluation marks a transformative shift in quality assurance practices. Traditional QA scorecards often fall short due to their subjective nature and limited scalability, resulting in inconsistency and bias. By integrating AI technology, organizations can significantly enhance the evaluation process, ensuring greater accuracy and reliability.
AI-enhanced QA evaluation not only addresses these traditional pitfalls but also introduces the ability to provide real-time feedback and leverage data analytics effectively. This modern approach empowers teams to make informed decisions quickly, leading to continuous improvements in customer experience and overall performance. Embracing this change is essential for organizations looking to stay competitive in today’s fast-paced landscape.
Analyze & Evaluate Calls. At Scale.
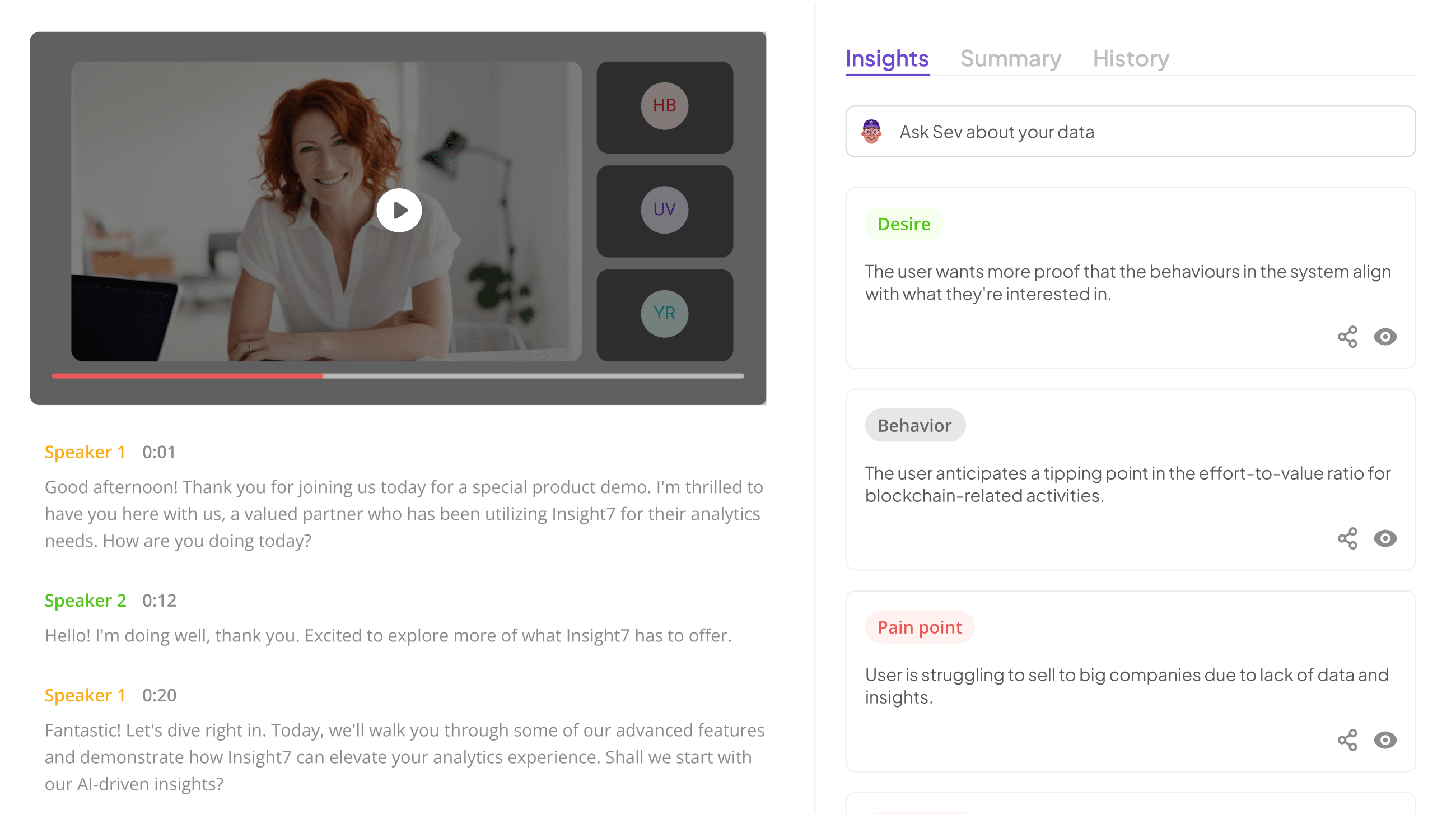
The Challenges of Traditional QA Scorecards
Traditional QA scorecards face several challenges that can significantly hinder their effectiveness in performance evaluation. One major issue is the limited scalability and subjectivity inherent in manual processes. When evaluators rely on manually filled scorecards, the likelihood of inconsistencies increases, leading to unreliable assessments. This subjectivity can create bias, where certain performances are viewed through a skewed lens, resulting in unfair evaluations of team members.
Another critical challenge is the lack of real-time feedback and optimal data utilization. Delayed feedback can severely hinder an individual’s ability to improve, as they are unaware of their shortcomings until the next review. Additionally, traditional methods often fall short in leveraging valuable data insights. Without harnessing analytics, organizations miss out on opportunities for performance enhancement, limiting their overall growth potential. By addressing these obstacles, the transition to AI-enhanced QA evaluation can transform the effectiveness of performance assessments.
Limited Scalability and Subjectivity
Manual processes in traditional QA scorecards present a significant bottleneck for organizations striving for efficiency. When evaluations rely on human input, they often become slow and prone to inconsistency. This slow pace can hinder timely performance reviews, resulting in missed opportunities for improvement. The subjectivity inherent in these evaluations introduces bias, which can undermine the credibility of assessments and lead to potential performance issues.
AI-enhanced QA evaluation addresses these challenges by automating assessments, promoting consistency, and scaling processes effectively. By minimizing human subjectivity, organizations can produce objective evaluations, allowing for a more accurate reflection of performance. Additionally, the ability to analyze large volumes of data quickly transforms how insights are derived and acted upon. With AI, organizations gain timely feedback and a clearer understanding of their quality metrics, enhancing decision-making and fostering continuous improvement.
- The Bottleneck of Manual Processes: Explore how manually filled scorecards often lack efficiency and consistency.
Manually filled scorecards represent a significant bottleneck in quality assurance processes. These scorecards often suffer from inefficiencies arising from human error, which can lead to incomplete evaluations. When agents or auditors manually record scores, the likelihood of inconsistency increases, casting doubt on the validity of the results. Inconsistent evaluations compromise the overall quality assurance efforts, making it difficult to trust the derived insights.
Moreover, manual scorecards often fail to capture real-time performance metrics. As a result, organizations might miss opportunities to promptly address issues or reinforce best practices. With AI-enhanced QA evaluation, these challenges can be effectively mitigated. By automating the scoring process, organizations can ensure greater accuracy and uniformity across evaluations. AI technology streamlines the collection and analysis of data, promoting a more effective quality assurance strategy that ultimately drives continuous improvement.
- Bias and Inconsistency: Discuss how subjectivity in evaluations leads to biased results and potential performance issues.
Subjectivity in evaluations often results in biased outcomes and inconsistencies that compromise performance. When relying on manual scorecards, evaluators bring their personal opinions and experiences into the assessment process. This subjectivity can skew results, leading to inconsistent evaluations of employee performance and even potential favoritism. As a result, team members may receive uneven feedback, which ultimately affects morale and productivity.
AI-enhanced QA evaluation addresses these challenges by providing objective assessments that reduce human bias. By standardizing evaluation criteria and utilizing machine learning algorithms, AI ensures that every evaluation is consistent and data-driven. This impartial approach not only increases fairness in assessments but also helps identify genuine performance issues more accurately. Transitioning to AI-based systems fosters a culture of trust, where employees feel recognized based on their true capabilities rather than subjective evaluations.
Lack of Real-time Feedback and Data Utilization
The lack of real-time feedback and effective data utilization poses significant challenges in traditional QA scorecards. When evaluations operate on delayed timelines, organizations miss opportunities for immediate corrective actions. This results in persistent issues remaining unaddressed, directly impacting performance and customer satisfaction. Moreover, without timely insights, teams struggle to implement necessary adjustments, stifling improvement and growth.
Additionally, traditional methods often underutilize valuable data analytics. Many organizations overlook the potential insights that could drive performance enhancement. By failing to analyze data comprehensively, these methods prevent businesses from understanding underlying patterns and trends. AI-enhanced QA evaluation addresses these shortcomings by providing instantaneous feedback and facilitating robust data analysis. Utilizing AI enables organizations to not only respond to issues more quickly but also to transform data into actionable strategies for continuous improvement. Embracing this technology allows for a more effective, proactive approach to quality assurance, resulting in improved outcomes for all stakeholders involved.
- Delayed Corrective Actions: Explain the consequences of not receiving immediate feedback.
Receiving immediate feedback is crucial in any quality assurance process, as delayed corrective actions can lead to several detrimental consequences. When feedback is postponed, agents may continue to repeat mistakes, which can affect overall service quality. This prolonged cycle of errors not only undermines team morale but also erodes customer trust and satisfaction, making it increasingly challenging to resolve issues effectively.
Moreover, without timely insights, organizations miss opportunities for improvement, stifling growth and innovation. This scenario frequently results in underperformance, decreasing the overall effectiveness of teams. By utilizing AI-enhanced QA evaluation tools, organizations can bypass these pitfalls. These tools provide real-time feedback, allowing teams to adjust their approaches swiftly, improving accountability and fostering a culture of continuous learning. Therefore, integrating instant feedback mechanisms is not just beneficial; it is essential for enhancing performance and maintaining customer trust.
- Underutilization of Data Insights: Discuss how traditional methods fail to leverage data analytics for performance improvement.
Traditional methods often leave data insights underutilized, leading to missed opportunities for performance improvement. Scorecards filled manually can overlook nuanced data trends and may rely on outdated metrics. This rarely provides a complete picture of performance, resulting in decisions based on insufficient evidence. Without leveraging data analytics effectively, organizations struggle to pinpoint areas for improvement, missing essential feedback loops that could enhance their operations.
Moreover, traditional approaches often fail to facilitate real-time analysis, leading to delays in implementing necessary changes. The inability to analyze data dynamically results in stagnant processes that do not adapt to ongoing performance trends. In contrast, AI-enhanced QA evaluation can transform these challenges into opportunities. By utilizing advanced analytics, AI harnesses vast amounts of data, identifying patterns and insights that inform proactive decision-making. Embracing these modern solutions not only drives consistent improvements but also fosters a culture of continuous learning and adaptability within organizations.
Extract insights from interviews, calls, surveys and reviews for insights in minutes
AI-enhanced QA Evaluation: Modern Solutions
AI-enhanced QA evaluation represents a paradigm shift in quality assurance methodologies. Traditional QA scorecards often suffer from inefficiencies due to manual processing and lack of real-time feedback. By harnessing the power of artificial intelligence, organizations can achieve objective assessments and overcome the biases inherent in human evaluations. Automation streamlines the QA process, ensuring consistency and scalability across evaluations.
Moreover, AI facilitates real-time reporting capabilities, enabling teams to act on insights immediately. As a result, businesses can adapt quickly to performance issues and refine their strategies more effectively. The integration of advanced analytics empowers organizations to derive actionable insights from vast amounts of data, fostering continuous improvement in QA practices. Overall, AI-enhanced QA evaluation not only improves accuracy but also enhances the agility and responsiveness of quality assurance teams, paving the way for superior customer experiences.
Harnessing AI for Objective and Scalable Assessments
Harnessing AI for Objective and Scalable Assessments transforms traditional quality assurance (QA) practices, creating a more reliable and efficient evaluation process. AI-enhanced QA evaluation offers a way to automate scoring, reducing the subjectivity that often complicates human evaluations. By utilizing advanced algorithms, organizations can ensure consistency, allowing for a fair comparison across evaluations and providing a uniform standard for performance assessment.
Moreover, AI technology enables real-time reporting capabilities, delivering instantaneous feedback. This immediacy allows teams to address performance issues promptly, enhancing learning and development opportunities. Automated systems analyze large volumes of data, yielding deeper insights that inform decision-making processes. Consequently, businesses can scale their QA operations effectively, adapting to changing needs while maintaining high standards of excellence. By embracing AI in this manner, companies pave the way for a more objective and scalable assessment strategy that drives continuous improvement in their quality assurance practices.
- Automation and Consistency: Detail how AI eliminates human bias and scales QA processes efficiently.
AI shines in its ability to automate processes and deliver consistent evaluations, addressing the biases that often muddy human assessments. When implementing AI-enhanced QA evaluation, organizations can ensure that evaluations are based on predefined metrics rather than personal interpretations, thus promoting objectivity. This shift significantly reduces the risk of biased evaluations that may emerge from individual preferences or misunderstandings.
By automating QA processes, AI enables organizations to scale their operations efficiently. With AI, performance evaluations can be swiftly generated, allowing for timely feedback and adjustments. This not only enhances the quality of assessments but also empowers teams to focus on growth and improvement, rather than getting bogged down by manual, time-consuming tasks. As a result, organizations that embrace AI technologies can achieve higher standards of quality assurance and foster a culture of continuous improvement.
- Real-time Reporting Capabilities: Explain the benefits of instantaneous feedback driven by AI technology.
AI-enhanced QA evaluation revolutionizes the way we perceive feedback. Real-time reporting capabilities provide instantaneous insights, allowing teams to address issues as they arise. This immediate feedback mechanism fosters a proactive approach, enabling swift corrective actions. Traditional scorecards often delay critical evaluations, which can hinder performance improvements. However, with AI, organizations can respond to training needs and market demands more rapidly.
These real-time insights not only eliminate bottlenecks but also cultivate a culture of continuous improvement. By utilizing advanced AI technologies, businesses can analyze interactions comprehensively, ensuring that quality assurance remains objective and scalable. This timely access to data enables teams to refine their strategies continuously, aligning closely with evolving customer expectations and enhancing overall operational efficiency. The transformation led by AI ultimately drives better customer experiences and stronger business outcomes.
💬 Ask About This Article
Have questions? Get instant answers about this article.
Leveraging Data with AI-enhanced QA Evaluation
AI-enhanced QA evaluation plays a pivotal role in transforming traditional quality assessment methods. It allows organizations to harness advanced analytics, turning vast datasets into actionable insights. This process not only improves the accuracy of evaluations but also ensures that feedback is swift and relevant. By utilizing AI, teams can monitor performance in real-time, drastically reducing delays in identifying and addressing issues.
Moreover, AI systems continuously learn from historical data, adapting to improve QA processes over time. This adaptability means organizations can respond effectively to dynamic market conditions and evolving customer expectations. In essence, AI-enhanced QA evaluation redefines how data is interpreted and utilized, promoting a proactive approach to quality assurance that traditional scorecards simply cannot match. By leveraging this technology, companies pave the way for more robust and informed decision-making, leading to enhanced operational performance.
- Advanced Analytics for Deep Insights: Discuss the role of AI in providing actionable insights from big data.
AI plays a transformative role in the realm of data analytics by turning big data into actionable insights. Traditional QA scorecards often fall short by relying on manual assessments, which can lead to biases and inconsistencies. In contrast, AI-enhanced QA evaluation provides objective, scalable, and immediate feedback. By tapping into vast datasets, AI identifies patterns and trends that human evaluators might overlook, enabling organizations to address performance issues swiftly and effectively.
Moreover, AI-driven analytics continuously learns from new information, adjusting its algorithms to improve accuracy over time. This adaptability ensures that businesses remain responsive to changing customer needs and market dynamics. Ultimately, the deployment of AI in QA processes not only refines data interpretation but also opens pathways for strategic decision-making grounded in empirical evidence. Embracing AI-enhanced QA evaluation equips companies with the deep insights necessary to drive sustained growth and operational excellence.
- Enhanced Adaptability and Learning: Explore how AI continuously learns and adapts, leading to ongoing improvement in QA processes.
AI-enhanced QA evaluation promotes continuous learning and adaptability, essential for evolving quality assurance processes. Unlike traditional methods, which often become stagnant and reliant on outdated metrics, AI systems leverage machine learning to consistently analyze new data. This iterative learning process enables the technology to fine-tune its algorithms, adapt to changing environments, and enhance its overall effectiveness in real-time evaluations.
Moreover, AI continuously updates its understanding of quality indicators by assimilating feedback and outcomes. This means that as more interactions are analyzed, the system can identify patterns and anomalies that escape human evaluators. Consequently, QA professionals can focus their efforts on strategic improvements rather than merely addressing past performance metrics. By fostering a culture of ongoing adaptability and learning, AI streamlines quality assurance processes, ensuring they remain relevant, accurate, and effective in meeting organizational standards.
Top Tools for AI-enhanced QA Evaluation
Incorporating AI-enhanced QA evaluation tools can significantly transform traditional quality assurance processes. These advanced tools help streamline evaluations, providing organizations with the ability to analyze vast amounts of data efficiently. For instance, tools like insight7 automate the transcription and analysis of calls, allowing businesses to extract meaningful insights without the burden of manual data handling. This automation results in faster evaluations and minimizes human error in judging performance.
Moreover, other noteworthy tools enhance the capabilities of AI-enhanced QA evaluation. Observe.AI excels at using AI to provide deep analytics, transforming how evaluations are conducted. CallMiner employs advanced AI algorithms to uncover insights hidden within conversations, improving overall QA strategies. Finally, NICE Uptivity delivers real-time feedback, ensuring that businesses can make immediate, informed adjustments. By harnessing these technologies, organizations can overcome traditional pitfalls in QA scorecards and achieve more accurate, objective results.
insight7
To enhance QA evaluation, one must understand the critical benefits AI brings to traditional scorecards. Traditional QA practices often struggle with inconsistencies and biases due to manual input, stifling performance reviews and leading to unproductive outcomes. By integrating AI-enhanced QA evaluation, organizations can create robust systems that promote objectivity and efficiency in performance assessments.
This modern approach not only automates routine processes, significantly reducing human error and subjectivity, but also provides real-time feedback. Instant feedback allows teams to react promptly to performance issues, facilitating quicker corrective actions. Moreover, AI’s ability to analyze massive datasets generates meaningful insights, enabling organizations to make informed decisions and continuously refine their QA strategies. Embracing AI-enhanced QA evaluation transforms traditional pitfalls into pathways for organizational excellence and long-term success.
- Highlighting its core features and unique benefits for QA processes.
AI-enhanced QA evaluation offers remarkable capabilities that transform traditional quality assurance processes. One of its core features is automation, which eliminates human bias and streamlines evaluations, allowing for consistent and objective assessments. This automation empowers teams to handle large volumes of evaluations swiftly, thereby improving overall efficiency. In addition, the real-time reporting capabilities ensure that feedback is delivered instantly, enabling organizations to correct course immediately when issues arise.
The unique benefits of AI-enhanced QA evaluation also extend to harnessing advanced analytics. These tools can mine vast amounts of data to extract actionable insights that drive continual improvement. Moreover, AI’s adaptability enables the system to learn from past evaluations, refining processes based on historical performance. As a result, organizations can achieve a dynamic and responsive QA process that not only ensures compliance but also enhances customer experience and satisfaction.
Other Noteworthy Tools
Beyond the primary tools for AI-enhanced QA evaluation, several noteworthy options can elevate your quality assurance processes. First on the list is Observe.AI, a platform tailored for transforming traditional QA methods. By integrating advanced speech recognition, it allows organizations to gain deeper insights from customer interactions, enabling proactive improvements rather than reactive measures.
Another valuable tool is CallMiner, renowned for its powerful analytics capabilities. It uses AI algorithms to dissect customer interactions and unearth performance trends that may not be visible through manual evaluations. This insight fosters a culture of continuous improvement by identifying key areas for development.
Lastly, NICE Uptivity brings a different perspective by offering real-time feedback capabilities. This feature ensures that teams receive immediate insights during customer interactions, which can significantly enhance service quality and operational efficiency. Together, these tools demonstrate how AI-enhanced QA evaluation can refine performance metrics, turning traditional pitfalls into opportunities for growth.
- Observe.AI: Discuss its capabilities in transforming QA processes.
AI-enhanced QA evaluation offers a powerful shift in how organizations handle quality assurance processes. One of the most significant capabilities is its ability to provide objective assessments that reduce bias. Traditional QA methods often rely on manual evaluations, which can introduce subjectivity and inconsistency. In contrast, AI-driven solutions automatically analyze interactions for adherence to guidelines, delivering real-time insights that empower teams to modify behaviors proactively.
Moreover, AI technologies transform the way performance data is utilized. Instead of waiting for periodic reviews, organizations can gain immediate feedback on individual and team performance. This allows managers to address issues as they arise, fostering a culture of continuous improvement. Enhanced reporting features generate detailed evaluation documents that can be customized according to specific compliance needs. With these advancements, businesses can not only enhance their QA processes but also achieve higher standards of service excellence, ultimately leading to improved customer satisfaction.
- CallMiner: Explain how it uses AI for enhanced analytics and insights.
AI-enhanced QA evaluation transforms the traditional quality assurance landscape by introducing advanced analytics and insights through sophisticated algorithms. By analyzing calls, interactions, and customer sentiments, organizations can swiftly identify critical trends and performance benchmarks. This shift from manual assessments to automated evaluations not only ensures objectivity but also enhances scalability, allowing deeper insights to be leveraged from extensive data sets.
One major benefit of this technology is its real-time reporting capabilities. Unlike conventional QA scorecards, which often delay feedback, AI-driven systems provide immediate analysis, enabling teams to adjust practices on-the-fly. Moreover, these systems continuously learn from user interactions, adapting their analytics to meet evolving standards. Consequently, organizations can foster ongoing improvement in their QA processes, ensuring a higher standard of customer service and operational efficiency through AI-enhanced QA evaluation.
- NICE Uptivity: Provide insights on its role in delivering real-time feedback.
Real-time feedback has emerged as a crucial element in modern quality assurance processes. NICE Uptivity serves as a powerful tool in this context, enabling organizations to garner immediate insights from customer interactions. Unlike traditional QA scorecards, which often provide delayed feedback, Uptivity facilitates an agile response to performance issues. This enhancement fosters an environment of continuous improvement, ensuring that agents receive timely guidance to refine their skills and elevate customer experiences.
The role of NICE Uptivity extends beyond mere performance reviews; it taps into data-driven insights that are critical for organizational growth. By analyzing interactions in real-time, organizations can identify trends and patterns, leading to informed decision-making. This immediacy allows for proactive adjustments in training and support, turning potential pitfalls into opportunities for development. Consequently, the integration of real-time feedback is essential in transforming traditional QA practices into an AI-enhanced quality evaluation system.
Conclusion: Embracing AI-enhanced QA Evaluation for Excellence
The journey towards excellence in quality assurance begins with embracing AI-enhanced QA evaluation. This innovative approach addresses the limitations often found in traditional scorecards, such as bias and inefficiency. By harnessing AI, organizations can achieve more objective assessments, allowing for scalable evaluations that enhance overall performance. This transformation fosters an environment where consistent and accurate feedback becomes a reality.
Incorporating AI enhances the ability to analyze large volumes of data in real-time, leading to insights that drive continuous improvement. By adopting this forward-thinking methodology, teams can shift from reactive processes to proactive strategies. Embracing AI-enhanced QA evaluation not only strengthens quality assurance efforts but also promotes a culture of excellence and accountability within organizations.
💬 Ask About This Article
Have questions? Get instant answers about this article.