5 Hidden Productivity Gains From AI-Enhanced QA Monitoring
-
Bella Williams
- 10 min read
In today's high-speed digital environment, the demand for quality assurance (QA) in software development has never been higher. Companies are seeking innovative ways to improve efficiency, and AI-driven QA productivity presents a transformative solution. By harnessing the power of artificial intelligence, organizations can not only enhance their quality monitoring processes but also uncover hidden productivity gains that were previously overlooked.
AI-driven QA productivity streamlines testing and evaluation, enabling teams to focus on more strategic initiatives. This introduction sheds light on the untapped potential of AI in quality assurance, emphasizing how it can dramatically improve processes, reduce labor costs, and elevate overall software quality. As we delve deeper into this topic, you will discover five distinct advantages that AI-enhanced monitoring can bring to your QA workflows.
In todays fast-paced digital world, maintaining quality assurance (QA) in software development is crucial. However, integrating artificial intelligence (AI) can significantly enhance productivity. This article will explore five hidden productivity gains from AI-Enhanced QA monitoring.
In todayโs fast-paced digital world, ensuring robust quality assurance (QA) in software development is not just essential; itโs a necessity. The relentless demand for faster releases can sometimes compromise the effectiveness of traditional QA methods. Enter artificial intelligence (AI), a game changer that can substantially improve productivity in QA processes. By harnessing AI-driven QA productivity, organizations can uncover hidden gains that enhance efficiency and accuracy in software development.
One of the most significant advantages is the reduction of manual testing time. With AI automating repetitive tasks, teams can redirect their efforts towards more intricate challenges, yielding higher quality outcomes. Additionally, real-time analysis allows for immediate insights, enabling swift corrections before issues escalate. AI also enhances decision-making through data-driven strategies and predictive analytics, empowering teams to address potential problems proactively. Overall, embracing AI tools revolutionizes QA monitoring and positions organizations to excel in quality while meeting rapid market demands.
Analyze & Evaluate Calls. At Scale.
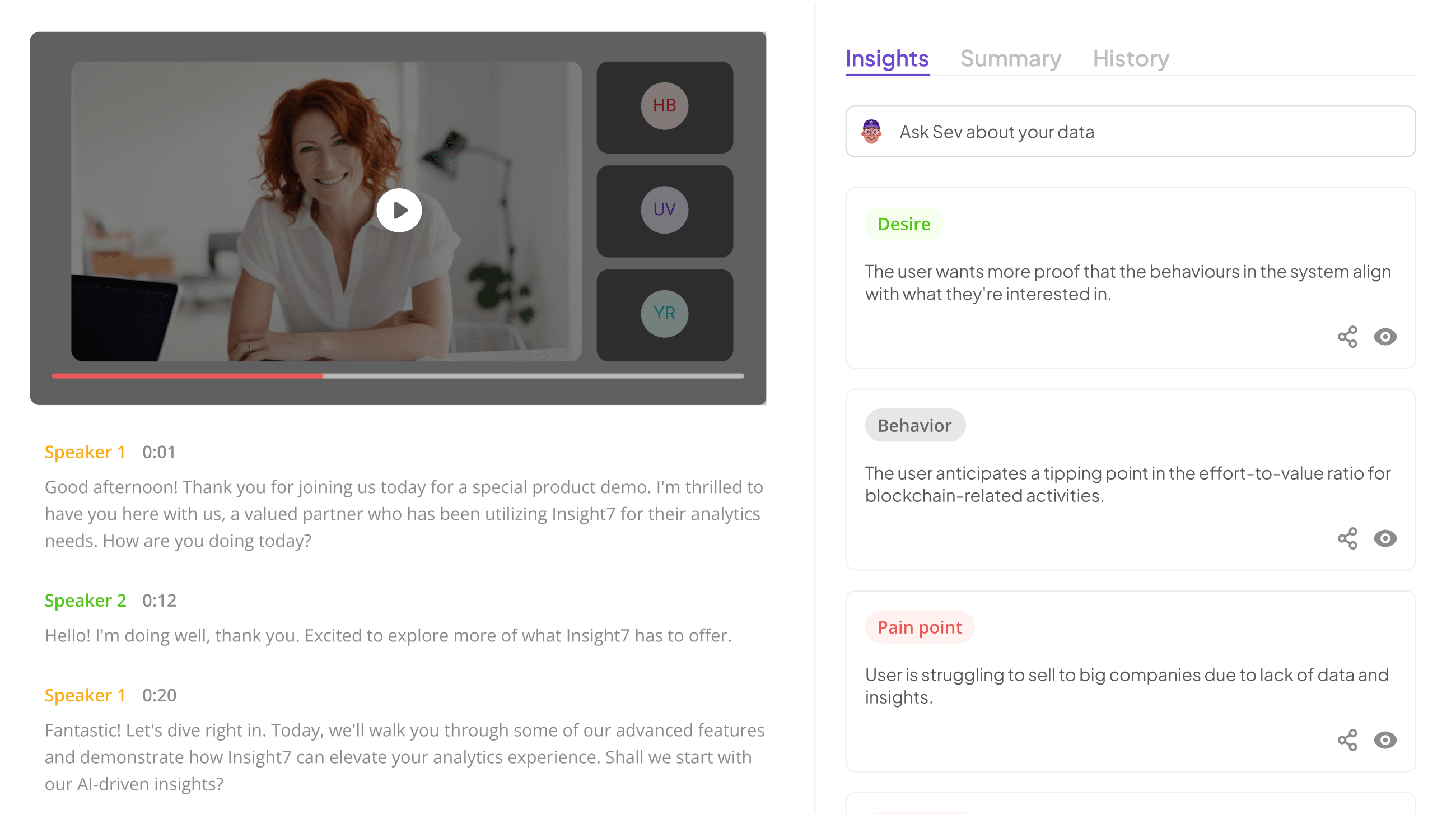
Unlocking AI-Driven QA Productivity: Key Benefits
AI-Driven QA Productivity unlocks transformative benefits that can streamline workflows and boost overall efficiency in software quality assurance. One key advantage is automation, reducing the time spent on manual testing. This allows teams to devote their energies to more complex tasks that demand human ingenuity. Additionally, AI provides real-time data analysis, offering immediate insights and prompting quick corrections, improving the overall quality of the software being tested.
Moreover, AI-driven productivity enhances decision-making through data-driven insights. Quality assurance teams can utilize predictive analytics to anticipate potential issues, facilitating proactive measures rather than reactive fixes. This blend of efficiency and foresight ensures that quality assurance processes are not only faster but also more effective, setting a foundation for long-term success and reduced costs. Ultimately, embracing AI in QA practices cultivates an atmosphere of continuous improvement and higher quality output.
AI-driven QA productivity isnt just about efficiency; its about unforeseen benefits that streamline processes and drive quality.
AI-driven QA productivity is transformative, reaching beyond just speeding up tasks. The integration of AI can lead to remarkable unforeseen benefits that fundamentally improve the quality of processes. For instance, automated tools can reduce the time spent on manual testing, allowing teams to focus on more intricate and strategic aspects of their projects. This shift not only enhances productivity but also increases job satisfaction as team members engage in more meaningful work.
Another unexpected gain comes from enhanced collaboration. With AI-powered systems consolidating data and insights, teams can access real-time information effortlessly. This streamlining of communication fosters a culture of transparency and informed decision-making, leading to higher quality outcomes. Companies discovering these hidden advantages of AI-enhanced QA monitoring can transform their approaches, ultimately driving superior products and services while achieving impressive productivity gains.
Reduced Manual Testing Time
Integrating AI technology into quality assurance processes leads to significantly reduced manual testing time, streamlining operations. Automation advantages play a key role here, minimizing the need for manual input and allowing teams to prioritize complex problem-solving tasks. As a result, QA professionals can allocate their talents more effectively, focusing on strategic initiatives rather than repetitive testing scenarios.
In addition, real-time analysis capabilities offered by AI enhance testing accuracy. By analyzing data on-the-fly, AI systems can provide immediate insights and corrections, ensuring that issues are identified and rectified swiftly. This immediate feedback loop not only shortens testing cycles but also elevates the overall quality of software products. Organizations embracing AI-driven QA productivity will experience a remarkable enhancement in operational efficiency.
- Automation Advantages: Learn how AI minimizes manual input, allowing teams to focus on more complex tasks.
AI-driven QA productivity significantly reduces the need for manual input in quality assurance processes. By automating repetitive tasks, AI frees teams from routine testing duties, enabling them to channel their expertise into more complex challenges. This shift allows professionals to focus on analyzing results, improving processes, and innovating solutions that drive business growth.
Moreover, automation improves the speed and accuracy of data processing. AI systems can analyze large volumes of test data instantly, providing insights that were previously time-consuming to gather. This real-time analysis helps identify pain points and trends quickly, ensuring that teams can address issues proactively. With less time spent on manual tasks, organizations can enhance their overall productivity and deliver higher quality products, cementing their competitive edge in the market.
Incorporating AI-driven tools into your QA strategy not only streamlines operations but also transforms how teams utilize their skills for maximum impact.
- Real-Time Analysis: Understand how AI analyses data in real-time to provide immediate insights and corrections.
AI continuously analyzes data in real-time, offering immediate insights and making corrections on the fly. This capability is vital for enhancing overall productivity in QA processes. As data flows in from various sources, AI utilizes advanced algorithms to assess information rapidly. Immediate feedback allows teams to address issues as they emerge, preventing small problems from escalating into significant setbacks.
Additionally, real-time analysis facilitates informed decision-making by providing actionable data insights instantly. This minimizes delays commonly caused by manual data processing. By streamlining these processes, AI-Driven QA Productivity empowers teams to maintain high-quality standards while freeing them to focus on complex tasks. The result is a significant improvement in efficiency and a proactive approach to quality assurance, driving better outcomes for organizations. Embracing this technology not only enhances operational speed but also supports continuous learning and improvement, ultimately reshaping how companies approach QA monitoring.
[ optional: ]- Increased Test Coverage: Ensure broader and deeper testing practices without additional resources.
AI-Driven QA Productivity expands the scope of testing, ensuring broader and deeper test coverage without the need for extra resources. By integrating AI tools, teams can automate repetitive test scenarios, which provides extensive coverage across various environments and platforms. This increased reach helps identify hidden defects early in the development cycle, ultimately saving time and costs associated with late-stage bug fixes.
Moreover, AI technology allows for continuous monitoring, enabling teams to adapt quickly to changes in user behavior or application requirements. Enhanced data analysis capabilities facilitate the identification of patterns and trends, which can lead to smarter, more targeted testing efforts. By maximizing test coverage through AI, organizations can improve product quality, enhance user satisfaction, and streamline the overall QA process, thus gaining a competitive edge in the marketplace.
Unlocking AI-Driven QA Productivity: Key Benefits
AI-driven QA productivity isn't merely focused on efficiency; it encompasses many hidden benefits that can transform everyday operations. One significant advantage is the reduction in manual testing time. By automating routine processes, teams can direct their energies toward more complex and strategic tasks, enhancing overall productivity.
Moreover, AI facilitates real-time analysis, allowing for prompt insights and corrections. This capability not only streamlines workflows but also significantly improves the accuracy of QA processes. Additionally, AI empowers increased test coverage, enabling teams to perform broader and deeper testing practices without straining resources. Embracing these hidden benefits greatly enhances the quality of deliverables and positions organizations to thrive in a competitive landscape.
Extract insights from interviews, calls, surveys
and reviews for insights in minutes
Extract insights from interviews, calls, surveys and reviews for insights in minutes
Extract insights from interviews, calls, surveys and reviews for insights in minutes
Optimizing Resource Allocation with AI-Driven QA Productivity
Optimizing resource allocation with AI-driven QA productivity enhances the effectiveness of quality assurance processes. By leveraging AI, organizations can streamline resource deployment and ensure that skilled personnel focus on critical tasks. This approach not only reduces waste but also improves overall efficiency, allowing teams to allocate their time better across various projects.
Data-driven insights play a crucial role in this optimization. With AI's ability to analyze large volumes of data quickly, decision-makers can identify trends and allocate resources where they are most needed. Furthermore, predictive analytics assists in foresee potential issues, allowing teams to proactively address problems before they escalate. This proactive stance ultimately leads to more strategic resource allocation and maximizes the return on investment for QA efforts.
Ensuring effective resource allocation can lead to significant productivity gains in quality assurance processes.
Ensuring effective resource allocation in quality assurance processes unlocks the potential for substantial productivity gains. By strategically aligning resources, organizations can minimize waste and streamline operations, allowing teams to focus on high-impact activities. AI-driven QA productivity plays a pivotal role here, as it facilitates smarter resource distribution based on data analytics and real-time insights.
When resources are allocated effectively, teams experience improved collaboration and faster response times. AI tools help identify bottlenecks within the QA process, enabling swift adjustments to workloads or project priorities. Moreover, these tools provide predictive analytics, empowering teams to foresee potential challenges and proactively address them. By harnessing AI-driven solutions in quality assurance, organizations can realize notable efficiencies, ultimately leading to enhanced overall performance and fulfilling project objectives more effectively.
Enhanced Decision-Making Processes
Enhanced decision-making processes are crucial for maximizing AI-driven QA productivity. By employing AI tools, organizations can access vast amounts of data, leading to more informed and effective decisions. Traditional QA monitoring often involves subjective assessments, which can introduce bias. AI removes this subjectivity by analyzing performance metrics with precision, fostering a culture of data-driven decisions.
Predictive analytics further empowers businesses to foresee potential issues before they arise. By recognizing patterns in data, teams can proactively address problems, enhancing efficiency and reducing downtime. This forward-thinking approach allows for timely interventions and greater resource allocation. Ultimately, integrating AI into decision-making processes elevates the quality assurance landscape, paving the way for improved performance and sustainability in software development practices.
- Data-Driven Decisions: Explore how AI empowers data-driven decision-making to enhance QA monitoring.
Data-driven decision-making is essential for optimizing quality assurance (QA) monitoring within software development. AI technology empowers this process by analyzing vast datasets to uncover patterns and insights that human analysts may overlook. As teams implement AI-driven QA productivity frameworks, they gain the ability to make informed decisions based on accurate data analytics rather than intuition alone.
The impact of such technology is profound. AI can facilitate real-time data examination, allowing QA teams to address issues before they escalate into significant problems. By leveraging predictive analytics, organizations can anticipate quality pitfalls and deploy preventive measures accordingly. Furthermore, AI tools can streamline resource allocation, ensuring that effort and focus are directed toward areas that will yield the highest productivity returns. In these ways, AI enhances QA monitoring, driving data-focused decisions that ultimately lead to improved software quality.
- Predictive Analytics: Utilize predictive analytics for proactive problem-solving and prevention.
Harnessing predictive analytics can be a game-changer in the realm of AI-driven QA productivity. This powerful tool enables organizations to anticipate potential issues before they evolve into significant problems. By analyzing historical data patterns, teams can pinpoint emerging trends that might signal future challenges, allowing for proactive interventions.
๐ฌ Questions about 5 Hidden Productivity Gains From AI-Enhanced QA Monitoring?
Our team typically responds within minutes
The first step in leveraging predictive analytics involves identifying key performance indicators (KPIs) that matter most to your QA processes. Next, organizations can implement predictive models to forecast outcomes, providing crucial insights for informed decision-making. Finally, ongoing monitoring and adjustments in strategy based on predictive findings create a culture of continuous improvement, ultimately leading to enhanced QA efficiency and effectiveness. Ensuring that teams utilize these insights not only enhances productivity but also fosters a more resilient quality assurance process.
Cost-Effectiveness
The integration of AI-Driven QA productivity tools can lead to considerable cost-effectiveness for organizations. By automating repetitive tasks, these tools reduce the hours spent on manual quality assurance. This allows teams to concentrate on high-value activities that require human insight. The real-time data analysis provided by AI also identifies issues promptly, preventing costly errors before they escalate.
Moreover, AI tools streamline resource allocation and minimize overhead costs associated with traditional QA processes. By analyzing multiple scenarios and outcomes, these technologies provide insights that guide budgetary decisions. Investing in AI for quality assurance can yield a significant return, as it not only lowers operational costs but also enhances product quality. Thus, the cost-effectiveness of AI-enhanced QA monitoring lies in its ability to optimize resources, reduce errors, and increase overall productivity.
- Tools for Cost Management: Discover how AI helps manage QA costs effectively.
AI-driven tools for cost management are transforming how organizations handle quality assurance expenditures. By integrating AI technologies, businesses can streamline their QA processes, significantly reducing operational costs. One way AI achieves this is through automation, minimizing the need for labor-intensive manual testing. With AI tools analyzing data in real time, teams can identify and rectify issues swiftly, ultimately leading to less expenditure on fixing defects after release.
Moreover, AI enhances long-term ROI by allowing for more effective resource allocation. As QA teams prioritize high-risk areas based on predictive analytics, they can focus their efforts where they are most needed. This proactive approach not only optimizes testing processes but also cuts down overall costs associated with delayed project timelines and post-release bugs. Consequently, AI-driven QA productivity is an essential component in managing QA costs effectively, fostering a culture of continuous improvement and financial awareness.
- Long-term ROI: Consider the return on investment from reduced errors and manual labor.
In evaluating the long-term return on investment (ROI) of AI-driven QA productivity, it's essential to recognize the significant savings realized from reducing errors and minimizing manual labor. Implementing AI technologies enhances precision in quality assurance processes, thereby decreasing the likelihood of costly mistakes. Each error rectified translates to considerable financial savings over time, as the expenses associated with corrective actions can be substantial.
Moreover, reduced manual labor allows staff to focus on higher-value tasks. This shift not only improves morale by alleviating repetitive duties but also fosters innovation within teams. When employees engage in more strategic roles, their contributions can lead to process improvements and higher overall productivity. Therefore, investing in AI-driven QA monitoring is not merely a cost but a transformative decision that can yield lasting benefits, fundamentally reshaping how organizations approach quality assurance.
Embracing AI Tools for Enhanced QA Productivity
Incorporating AI tools into QA processes can fundamentally reshape productivity dynamics. These innovative solutions not only streamline testing but also foster significant improvements in overall QA effectiveness. By integrating AI-driven QA productivity, teams can focus on more strategic tasks rather than being bogged down by repetitive processes.
First among the benefits is automation, which minimizes manual testing and significantly cuts down the time required to assess product quality. This enables teams to deliver faster results, thus enhancing product timelines. Another key element is real-time analysis, where AI processes data instantly. It identifies issues promptly, allowing for immediate corrections and preventing delays caused by overlooked errors. Overall, embracing AI tools fosters a culture of efficiency and precision, essential for success in todayโs fast-paced digital environment.
As organizations adapt to these changes, their commitment to quality assurance becomes more profound, paving the way for not just enhanced productivity, but also superior product quality.
Incorporating the right AI tools in QA monitoring can make a significant difference. Hereโs a list of top tools:
Incorporating the right AI tools in QA monitoring can make a significant difference in enhancing productivity. The right technology can transform traditional quality assurance processes into streamlined and efficient operations. By embracing AI-driven QA productivity, teams can unlock various benefits that were previously hidden, such as faster analysis and improved accuracy.
Here are some top tools that can elevate your QA monitoring:
- Insight7: This tool automates QA processes and maximizes operational productivity through intelligent insights.
- Testim: Take advantage of advanced AI features to automate your testing procedures thoroughly.
- Mabl: A seamless integration with DevOps workflows allows for real-time updates, keeping projects agile.
- Applitools: This tool specializes in visual testing automation, ensuring that all UI elements appear as intended.
- Functionize: Leverage machine learning capabilities that enhance the automation of testing processes.
- Rainforest QA: An intuitive platform that boosts testing efficiency while simplifying the user experience.
Incorporating these tools can drive productivity, improve decision-making, and significantly reduce testing times. Embrace AI to fully realize the benefits in your QA efforts.
- Insight7: A leading tool in automating QA processes and maximizing productivity.
Insight7 stands out as a leading tool in automating QA processes and maximizing productivity in the realm of software testing. This platform simplifies the traditionally arduous task of monitoring, allowing teams to analyze vast amounts of data efficiently. By streamlining QA processes, it eliminates the drudgery of manual testing, enabling teams to focus on more strategic initiatives.
This tool leverages AI to provide real-time insights, identifying issues before they escalate into larger problems. Consequently, teams can respond promptly, ensuring higher quality outputs. With Insight7, organizations can seamlessly integrate QA monitoring into their workflows, significantly enhancing collaboration among team members. This capability cultivates a proactive environment, where insights are utilized to drive actionable improvements in production cycles. In today's competitive landscape, using such powerful tools is essential for staying ahead and achieving sustained productivity gains.
- Testim: Utilize advanced AI features for thorough testing automation.
Incorporating advanced AI features into testing automation represents a significant leap forward in optimizing QA processes. Utilizing sophisticated algorithms, these features streamline test case generation, execution, and maintenance, dramatically reducing the time and effort traditionally spent. This not only accelerates the software development cycle but also enhances the quality of the final product through consistent, automated testing scenarios.
One of the critical advantages of these AI-driven tools is their ability to learn from previous tests, adapting to new changes and providing insights that would be impossible through manual testing methods. This adaptability results in increased test coverage, ensuring a broader and deeper assessment of software quality. As teams leverage these advanced capabilities, they can shift their focus to higher-level problem-solving, ultimately driving significant productivity gains and fostering innovation within their organizations.
- Mabl: Integrate seamlessly with DevOps workflows for real-time updates.
Integrating advanced testing solutions into DevOps workflows can significantly enhance AI-driven QA productivity. Real-time updates ensure that teams are always informed about the testing process and can address issues as they arise. This integration streamlines communication between development and quality assurance teams, leading to faster deployment cycles without compromising on quality.
AI-driven tools provide automated insights that help identify potential problems early in the development cycle. By utilizing these real-time updates, teams can continuously monitor applications, ensuring they meet quality standards before reaching the end user. This setup not only improves overall efficiency but also fosters a collaborative environment, eliminating silos between departments. Ultimately, seamless integration of testing tools within DevOps workflows empowers teams to deliver high-quality software faster and with greater confidence.
- Applitools: Specializes in automating visual testing with AI.
AI-Driven QA Productivity relies heavily on the innovative automation of visual testing, a game-changer in the landscape of quality assurance. This advancement enables teams to detect interface anomalies swiftly and accurately, leading to higher-quality software deployment. By integrating AI into visual testing, teams can reduce the time spent on manual checks and instead focus on critical thinking tasks that drive product excellence.
In practice, this results in not just faster testing cycles but also consistent verification of visual elements across multiple platforms. Companies can maintain their branding integrity while efficiently addressing any discrepancies that may appear post-deployment. This holistic approach to QA monitoring ensures comprehensive coverage and guarantees that end-users encounter only the best versions of software products. Embracing AI for visual testing can lead to unforeseen productivity gains, making it an essential element in contemporary QA strategies.
- Functionize: Use machine learning for smarter testing automation.
AI-Driven QA Productivity significantly boosts testing automation through the strategic application of machine learning. By harnessing AI, teams can intelligently leverage data to automate repetitive tasks, minimizing the burden of manual testing. This transformation allows QA teams to allocate their time to more critical areas, such as problem-solving and product enhancement.
Moreover, machine learning algorithms adapt to the ever-evolving testing landscapes, providing real-time insights and precise predictions. With AI analyzing vast amounts of data, testing coverage expands dramatically without the need for additional resources. This not only enhances productivity but also improves the overall quality of software products. Organizations that embrace AI-driven approaches in QA can expect to see not only increased efficiency but also more innovative solutions that stem from smarter testing practices. Overall, the integration of machine learning into testing processes revolutionizes the way QA teams operate, offering substantial productivity gains and elevating the standard of software quality.
- Rainforest QA: Provides an intuitive platform for increased testing efficiency.
The intuitive platform designed for QA offers a revolutionary approach to testing efficiency. By simplifying processes, this tool allows users from various backgrounds to engage with testing tasks, breaking down traditional barriers associated with expertise. This democratization of testing means that teams can activate insights quickly, driving innovation and improving output without the need for prolonged training sessions.
With an easy-to-navigate interface, users can analyze multiple files simultaneously, extracting valuable insights automatically. The platform's featuresโsuch as real-time analysis and pain point identificationโenable faster decision-making and encourage collaboration across teams. By streamlining the QA process, this tool not only enhances accuracy but also promotes AI-driven QA productivity, ultimately allowing organizations to focus on delivering superior software solutions more efficiently.
Conclusion: Maximizing AI-Driven QA Productivity
Embracing AI-Driven QA Productivity fundamentally changes the landscape of quality assurance. Organizations can harness powerful AI tools to enhance efficiency and uncover hidden gains not previously accessible, resulting in an optimized QA process. By minimizing manual tasks and enabling real-time data analysis, companies can significantly reduce testing timelines and allocate resources more effectively.
Moreover, AI's predictive capabilities empower teams to anticipate and address potential issues proactively. This not only enhances decision-making but also leads to improved software quality and a higher return on investment. Ultimately, maximizing AI-Driven QA Productivity leads to a leaner, more effective development lifecycle, where quality assurance becomes an integral part of continuous improvement.
AI-Driven QA Productivity offers hidden gains that can transform how companies handle quality assurance. By understanding and implementing AI-enhanced QA monitoring, businesses can significantly boost productivity, optimize resources, and ultimately enhance their software development lifecycle.
AI-Driven QA Productivity uncovers significant hidden benefits that can redefine how organizations manage quality assurance. By embracing AI-enhanced monitoring, businesses can elevate their operational efficiency and redirect valuable resources to strategic tasks. This shift not only streamlines workflows but also introduces a more agile approach to quality assurance, allowing teams to respond promptly to project demands.
One of the most notable gains from AI-driven productivity is the reduction in manual testing time. AI automates tedious processes, freeing up your team to tackle complex issues that require deeper analysis. Additionally, real-time data processing enables immediate insights, allowing for rapid corrections and adjustments. As businesses increasingly leverage these AI capabilities, they can expect enhanced decision-making processes rooted in data-driven analytics and predictive insights, ultimately leading to a more efficient software development lifecycle.
๐ฌ Questions about 5 Hidden Productivity Gains From AI-Enhanced QA Monitoring?
Our team typically responds within minutes