5 Agent Behavior Patterns You Can Detect with AI QA
-
Bella Williams
- 10 min read
Agent behavior detection serves as a crucial component in the landscape of AI-assisted quality assurance. Understanding how agents interact with customers can significantly enhance service delivery and operational efficiency. By analyzing communication patterns, organizations can identify strengths and weaknesses in their customer support efforts, leading to targeted improvements.
AI technologies empower businesses to uncover specific behaviors displayed by agents during interactions. This introduction to agent behavior detection outlines how technology can facilitate a deeper understanding of engagement strategies and compliance. Through ongoing monitoring and analysis, companies can refine their training programs, ultimately creating a more effective and responsive team.
Analyze & Evaluate Calls. At Scale.
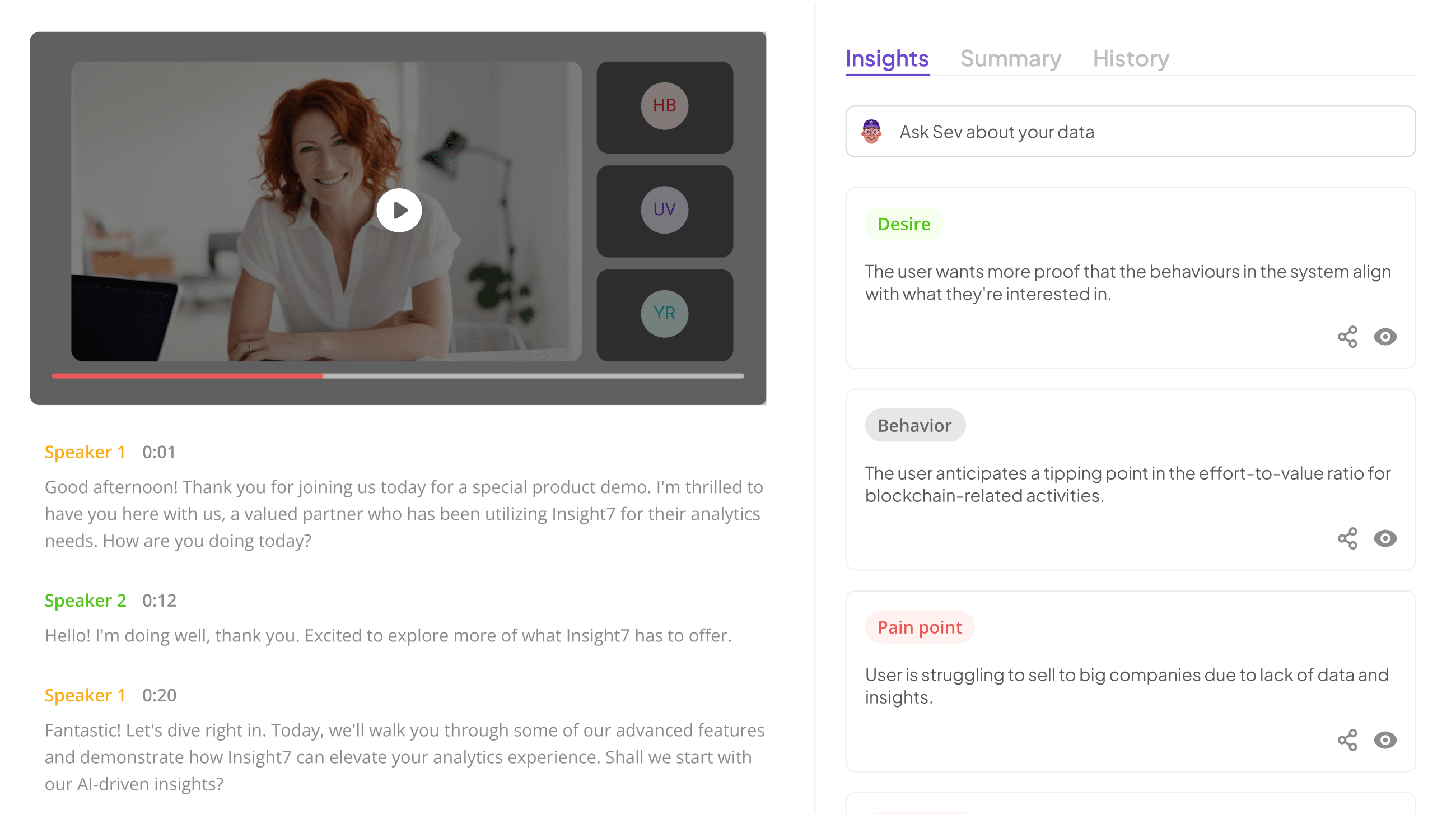
Understanding Agent Behavior Detection
Understanding Agent Behavior Detection is crucial for effectively evaluating agents within AI-powered Quality Assurance. This process involves monitoring and analyzing agents' interactions to identify patterns that could indicate strengths or weaknesses. By doing so, organizations can optimize training and improve overall performance.
One significant aspect of Agent Behavior Detection is recognizing communication trends. This involves tracking the types of phrases and responses agents use during conversations. Another key pattern to observe is the response times of agents, which can reveal their efficiency and effectiveness in handling customer inquiries. By examining these elements, you can gain insights into how agents engage with clients and adapt their strategies accordingly. Ultimately, mastering this detection empowers businesses to fine-tune their operations and enhance customer satisfaction through data-driven decisions.
Patterns of Interest in Agent Behavior Detection
Detecting patterns in agent behavior is essential for enhancing communication and efficiency in various domains. By focusing on communication trends, we can identify how agents interact with clients and understand the nuances of their response styles. Analyzing these interactions provides invaluable insights into optimizing performance, ultimately leading to improved customer satisfaction and operational success.
Another critical area in agent behavior detection is monitoring response times. This involves evaluating how quickly agents respond to inquiries and their ability to manage customer expectations effectively. By recognizing delays in response or identifying quick replies, organizations can pinpoint areas for improvement and foster a more responsive service culture, enhancing overall user experience. Embracing these patterns allows for a proactive approach in training and refining agents' skills, ensuring a more engaging and productive interaction with customers.
- Step 1: Identifying Communication Trends
In the journey of Agent Behavior Detection, the first crucial step is to identify communication trends. By closely examining conversational data, patterns emerge that reveal agent responses, tone, and engagement levels. These trends offer insights into how agents interact with customers, indicating whether they follow protocols or adapt to unique situations.
To effectively identify these trends, consider three key approaches: analyzing call transcripts, categorizing agent responses, and summarizing themes. First, reviewing transcripts allows for a detailed examination of conversations to pinpoint areas of improvement. Next, categorizing responses helps distinguish between appropriate and inappropriate communication. Finally, summarizing recurring themes provides a holistic view of agent behaviors, indicating overall performance and areas where further training may be necessary. Understanding these trends is essential for optimizing the effectiveness of AI in monitoring agent interactions.
- Step 2: Recognizing Response Times
In the realm of agent behavior detection, recognizing response times is crucial for evaluating the effectiveness of customer interactions. By meticulously tracking how long it takes agents to respond to customer inquiries, businesses can glean valuable insights into communication efficiency and service quality. This analysis not only highlights agents who excel in prompt responses but also identifies those who may require additional training or support.
To accurately assess response times, itโs essential to establish benchmarks tailored to your businessโs operational needs. Start by categorizing calls based on their length and complexity; longer interactions may indicate more profound customer needs. Additionally, integrating automated tools can facilitate this process, ensuring a consistent and unbiased evaluation of agent performance. By understanding response times through a focused lens of agent behavior detection, organizations can foster a more responsive and effective customer service team.
Tools for Agent Behavior Detection
Detecting agent behavior effectively relies on a combination of specialized tools designed for specific tasks. These tools enable a deeper understanding of interaction quality and agent performance. To implement efficient agent behavior detection, organizations harness advanced analytics software, machine learning algorithms, and natural language processing techniques. These technologies analyze conversations and identify patterns that flag anomalies in behavior or communication trends.
Key elements influencing agent behavior detection include tools that accurately record and process interactions, provide real-time feedback, and generate performance reports. For example, a reliable analytics dashboard allows supervisors to visualize agent performance metrics, such as engagement levels and response times. Additionally, integrating speech recognition technology enhances the capacity of these tools to attribute performance directly to individual agents, ensuring compliance with established standards. By employing these resources, companies can continuously refine their approaches to agent development and customer interaction, which ultimately leads to improved service outcomes.
- insight7
Detecting agent behavior patterns in AI QA is essential for improving customer interactions. By analyzing communication trends and response times, organizations can gain valuable insights into how agents engage with customers. Understanding these patterns enables businesses to identify strengths and weaknesses within their teams while unveiling opportunities for training and development.
Firstly, recognizing how often agents ask questions and encourage dialogue can influence customer satisfaction levels significantly. When agents shift from reactive responses to proactive inquiries, they foster better connections with customers. Secondly, monitoring response times provides clarity on agent efficiency and effectiveness. By combining these insights with AI-powered tools, companies can optimize agent training and enhance overall service quality. Ultimately, leveraging agent behavior detection leads to more productive teams and satisfied customers.
- Tool 2
In the realm of Agent Behavior Detection, Tool 2 brings a sophisticated approach to identifying communication trends within interactions. By utilizing advanced algorithms, this tool can analyze patterns in verbal and written exchanges, providing insights into typical responses and engagement levels. This analysis not only enhances understanding but also helps in tailoring interactions to meet clients' expectations effectively.
Additionally, Tool 2 enables the observation of response times, allowing organizations to gauge agent efficiency and customer satisfaction. Quick and accurate feedback can lead to improved service delivery and increased client trust. By implementing such a tool, businesses can gain a clearer picture of their agentsโ behaviors and performance metrics. This knowledge is pivotal in streamlining operations, ensuring compliance with industry standards, and ultimately elevating the customer experience. Through effective use of Agent Behavior Detection, organizations can drive performance improvements and foster stronger client relationships.
- Tool 3
Tool 3 introduces an innovative way to elevate your understanding of Agent Behavior Detection. This tool seamlessly analyzes conversations, allowing for the identification of specific patterns in agent interactions. As agents engage with customers, their communication styles and response strategies become evident, revealing valuable insights.
This analysis not only highlights communication effectiveness but also uncovers areas for improvement. By utilizing advanced algorithms, Tool 3 provides a comprehensive overview of agent behavior, focusing on key metrics such as tone, sentiment, and adherence to scripts. As a result, organizations can refine their training methodologies and enhance overall performance. Understanding these patterns fosters a more supportive environment for agents, ultimately leading to superior customer experiences. Integrating Tool 3 into your strategy ensures that you stay ahead in the ever-evolving landscape of customer interaction.
- Tool 4
Tool 4 plays a crucial role in the realm of Agent Behavior Detection by employing advanced analytics to assess agent interactions. This tool captures real-time data, which provides insights into communication effectiveness and agent efficiency. As agents engage with customers, the tool analyzes their responses, identifying patterns that may indicate strengths or areas for improvement.
To gain deeper insights, Tool 4 focuses on several key dimensions:
๐ฌ Questions about 5 Agent Behavior Patterns You Can Detect with AI QA?
Our team typically responds within minutes
- Communication Style: Understanding how agents phrase responses can highlight their adaptability and personalization skills.
- Empathy Levels: Evaluating the emotional tone in agent communications can uncover their ability to connect with customers, enhancing satisfaction.
- Adherence to Scripts: Monitoring compliance with standard scripts ensures agents maintain brand messaging, revealing their reliability.
By implementing these layers of analysis, Tool 4 not only aids in refining current agent practices but also enhances training protocols, fostering a more effective customer service environment. This continuous evaluation loop allows organizations to build stronger relationships with clients through improved agent performance.
- Tool 5
Tool 5 focuses on identifying communication trends within agent interactions. An effective method for Agent Behavior Detection involves analyzing dialogue patterns and response structures to uncover insights about agent performance. By utilizing this tool, organizations can assess how agents interact with clients, ensuring responses meet compliance standards and client expectations.
This tool also allows users to track key metrics, such as the frequency of specific phrases or the tone used during calls. Understanding these elements can help identify potential training needs or highlight top-performing agents. Additionally, enhanced feedback loops can be established based on this analysis, fostering a culture of continuous improvement. By closely monitoring agent behavior and communication styles, organizations can make informed decisions that promote both efficiency and customer satisfaction.
Extract insights from interviews, calls, surveys and reviews for insights in minutes
Implementing Agent Behavior Detection Strategies
To implement effective agent behavior detection strategies, one must approach this task with careful analysis and systematic evaluation. Begin by mapping behavioral patterns that emerge during interactions, focusing on key metrics such as communication trends and response times. Understanding these patterns helps in creating benchmarks for agent performance and improving customer interactions over time.
Next, integrating predictive analytics plays a crucial role in refining agent behavior detection. By identifying potential issues before they escalate, businesses can take proactive measures to enhance the customer experience. Additionally, optimizing AI models demands ongoing training for accuracy and continuous improvement cycles. This iterative process ensures that the system becomes more adept at identifying varying agent behaviors efficiently, leading to superior insights and operational effectiveness. Ultimately, a comprehensive strategy for agent behavior detection equips businesses with not only the ability to evaluate current performance but also the foresight to improve future interactions.
Analyzing Interaction Outcomes
Analyzing interaction outcomes is essential in understanding the effectiveness of communication and engagement strategies. Using AI to detect agent behavior patterns can lead to valuable insights. First, it is crucial to map behavioral patterns. By reviewing recorded interactions, you can identify what resonates with customers and what doesnโt, revealing significant trends in communication styles. Analyzing these outcomes enables businesses to tailor their approaches, ensuring that agents are equipped to handle different situations effectively.
Next, leveraging predictive analytics serves as a powerful tool. By analyzing past interactions, organizations can anticipate future behavior and improve their response strategies. This proactive approach enhances the overall customer experience and increases satisfaction rates. Delving into agent behavior detection not only transforms the way businesses interact with customers, but also facilitates continual growth and adaptation in an ever-changing market. By prioritizing these analyses, organizations can achieve more informed decisions and improved interaction outcomes.
- Step 1: Mapping Behavioral Patterns
Mapping behavioral patterns is the foundation of effective agent behavior detection in AI-driven quality assurance. This initial step involves examining how agents communicate, respond, and interact over time. By analyzing transcripts and notes from customer interactions, you can begin to see trends that reveal underlying behaviors and preferences. This process helps to identify consistent responses, as well as anomalies that may indicate areas for improvement.
To effectively map these patterns, follow three key approaches: first, aggregate data from various interactions to create a comprehensive overview. Next, identify common themes and pain points that emerge, focusing on specific keywords and phrases. Finally, visualize the findings through dashboards or reports to facilitate deeper insights. By adopting these strategies, organizations can create a clearer understanding of agent behavior, ultimately leading to enhanced performance and better customer experiences.
- Step 2: Leveraging Predictive Analytics
To effectively recognize agent behavior patterns, predictive analytics plays a crucial role in shaping insights. By analyzing historical data and trends, organizations can uncover significant behavior anomalies among agents, allowing for targeted interventions. This approach enhances the understanding of various response styles, communication preferences, and overall engagement levels. Consequently, the predictive nature of analytics empowers organizations to not only identify existing patterns but also forecast future behaviors.
Incorporating predictive analytics into agent behavior detection facilitates a proactive stance. This means addressing potential issues before they escalate, leading to improved agent performance and customer satisfaction. Key metrics such as response times and communication effectiveness can be analyzed to create an environment where agents thrive. Through continuous monitoring and data evaluation, organizations can adapt strategies to ensure optimal engagement and efficiency, thereby creating a robust foundation for future growth and innovation in their customer interaction processes.
Optimizing AI Models
To optimize AI models for effective agent behavior detection, a strategic focus on training and continuous improvement is essential. The accuracy of the AI relies heavily on the data it processes; therefore, comprehensive training datasets are crucial. This training should encompass diverse agent interactions, allowing the model to learn effective communication patterns and recognize variations in behavior. By being specific in the criteria for agent performance, we can better gauge an AI's capability to assess agent behavior accurately.
Continuous improvement is the next vital step in this optimization process. Regular assessments and updates to the model will help maintain its relevance as agent behaviors evolve. Utilizing feedback loops and real-time data, we can enhance predictive analytics and refine training algorithms. These enhancements empower organizations to adapt rapidly to changing market dynamics, ensuring that AI models not only detect agent behavior patterns accurately but also evolve alongside user expectations. In this way, the integration of robust AI systems supports increased efficiency and better decision-making.
- Step 1: Training for Accuracy
Training for accuracy is a crucial first step in agent behavior detection. To effectively detect and analyze the patterns exhibited by agents, thorough training ensures that the AI models can accurately interpret data. This process begins with gathering a diverse set of training data that reflects various communication styles, tones, and scenarios that agents might encounter. By utilizing this data, AI algorithms learn to identify subtle variations in behavior, leading to a more refined understanding of agent interactions.
Once the training data is established, ongoing assessments are essential. Regular evaluations allow for measurable insights into how well the AI performs in detecting agent behavior. Feedback loops incorporated into the training process help update and improve the AI models over time. These methods not only boost the accuracy of agent behavior detection but also enhance the overall quality of customer interactions, ultimately leading to increased satisfaction and trust in the system's capabilities.
- Step 2: Continuous Improvement
Continuous improvement is essential in the realm of agent behavior detection. This process involves regularly assessing the performance and effectiveness of AI quality assurance (QA) tools. By consistently analyzing the data generated from agent interactions, organizations can identify specific areas where enhancements can be made. For instance, recognizing patterns in communication can reveal trends that suggest necessary updates in training and procedure.
Furthermore, continuous improvement allows teams to be responsive to evolving customer needs and feedback. This adaptability ensures that agents are equipped with the most relevant skills and information, creating a more efficient service environment. The cycle of evaluating agent performance, implementing changes, and monitoring results is fundamental for growth. By embracing this iterative approach, organizations can better support their agents, ultimately leading to higher customer satisfaction and improved performance metrics.
Conclusion on Agent Behavior Detection in AI QA
Agent behavior detection plays a pivotal role in enhancing AI quality assurance practices. By closely examining communication trends and response times, organizations can gain valuable insights into agent performance. This proactive approach not only improves service delivery but also creates a roadmap for continuous training and development.
To effectively implement these insights, integrating predictive analytics is essential. This allows businesses to anticipate potential issues and optimize their AI models for accuracy and reliability. Ultimately, the focus on agent behavior detection not only boosts overall performance but also enhances customer experiences, promoting long-term success in the competitive landscape.
๐ฌ Questions about 5 Agent Behavior Patterns You Can Detect with AI QA?
Our team typically responds within minutes