AI Sentiment Enhancement in Detection has revolutionized how organizations interpret emotions and opinions from textual data. By harnessing advanced algorithms, AI can analyze vast amounts of feedback at unprecedented speeds, ensuring improved accuracy in detecting underlying sentiments. This technology not only identifies feelings but also understands context, allowing for a more nuanced interpretation of user emotions.
As businesses increasingly rely on digital interactions, optimizing sentiment detection becomes vital for understanding customer experiences and market trends. With AI sentiment enhancement, organizations can detect shifts in consumer feelings and respond proactively, thereby tailoring their approaches to meet user expectations effectively. The impact of such enhancements extends to multiple sectors, paving the way for innovation in how sentiment analysis is performed.
Analyze qualitative data. At Scale.
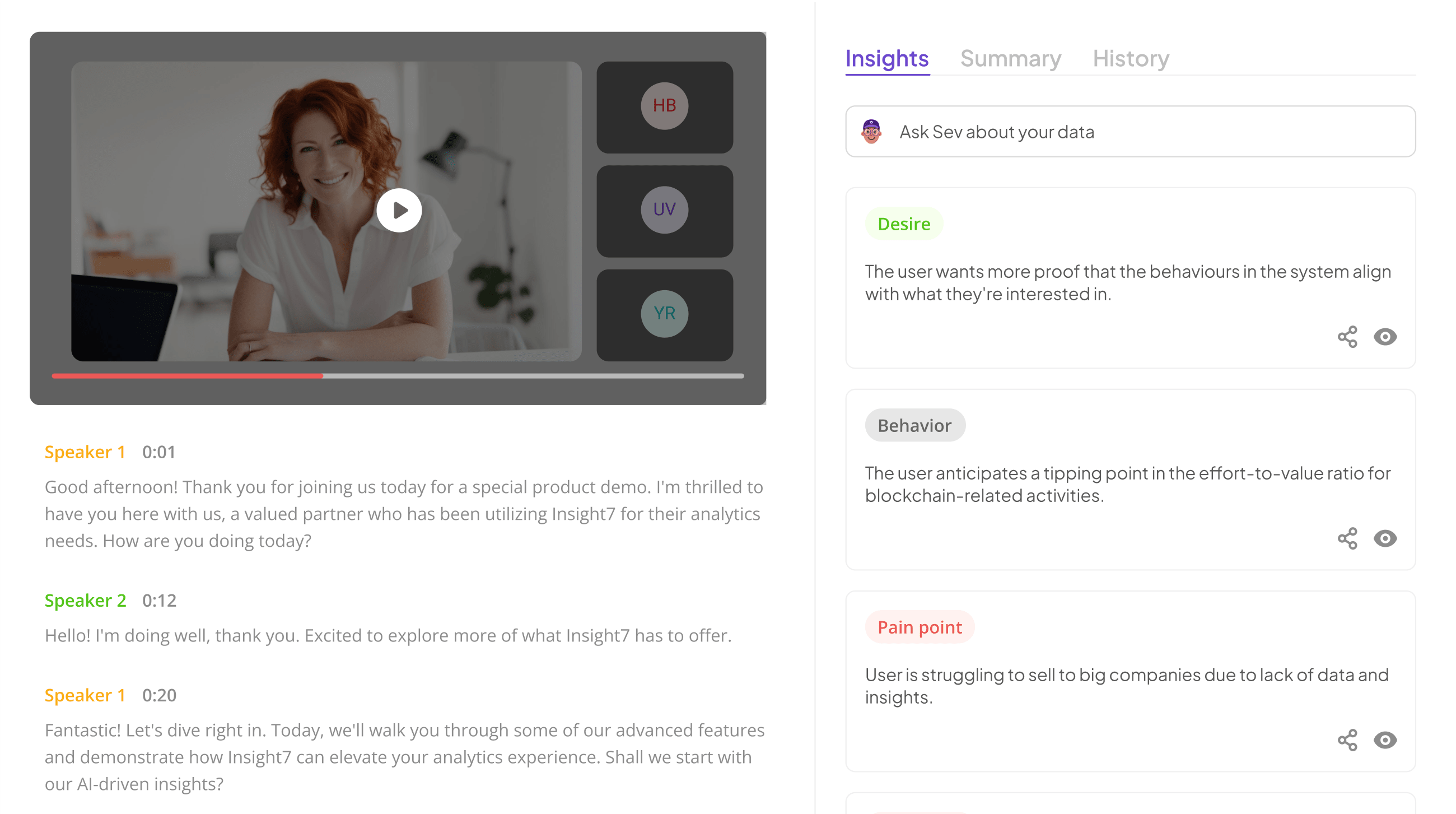
Leveraging AI Sentiment Enhancement for Social Media Monitoring
AI Sentiment Enhancement plays a crucial role in social media monitoring by refining the accuracy of sentiment detection. Social media platforms are inundated with vast amounts of user-generated content, making it challenging for organizations to extract meaningful insights. By employing AI-driven approaches, businesses can effectively analyze this data to capture the nuances of customer opinions and sentiments.
To harness AI Sentiment Enhancement, organizations typically follow several key steps. First, data collection and preprocessing are vital, involving the aggregation of relevant social media posts and comments. Next, AI model training and fine-tuning ensure that the tools can accurately identify emotional expressions, whether positive or negative. Finally, integrating these models with social media platforms enables real-time monitoring, providing companies with timely insights into public perception and emerging trends. By effectively implementing these strategies, businesses can enhance their understanding of audience sentiment significantly.
Enhancing Accuracy in Social Media Analytics
To enhance accuracy in social media analytics, organizations must adopt advanced AI sentiment enhancement techniques tailored for robust data interpretation. Understanding user sentiments through accurate analysis not only deepens engagement but also drives strategic decision-making. The first step involves effective data collection and preprocessing, which ensures the captured data is clean and reliable. This foundational work sets the stage for meaningful insights.
Following data preparation, AI model training and fine-tuning is crucial. This process helps refine the models to detect nuances in sentiment more accurately. Finally, integrating these models with social media platforms allows for real-time monitoring of audience sentiment, enabling timely responses and adaptations. By focusing on these steps, businesses can significantly improve their capacity to engage with their audience, ultimately leading to enhanced brand loyalty and customer satisfaction. Effective sentiment detection is not merely a technical endeavor, but a strategic advantage for organizations striving to connect authentically with their audience.
- Steps to Improve Sentiment Detection with AI
To enhance sentiment detection with AI, a structured approach is essential. The first step is data collection and preprocessing, which involves gathering relevant text data from diverse sources. Ensuring that this data is cleaned and formatted correctly lays a solid foundation for subsequent analysis. Accurate preprocessing minimizes noise and allows AI algorithms to recognize sentiments more effectively.
Next, AI model training and fine-tuning are crucial. This stage involves using historical data to train the model so that it can accurately identify sentiment orientations such as positive, negative, or neutral. Finally, integration with social media platforms is vital for real-time sentiment analysis. When seamless integration occurs, businesses can receive immediate feedback, enhancing their ability to respond to customer sentiments. Implementing these steps supports AI sentiment enhancement, ultimately leading to better insights and more informed decision-making.
1. Data Collection and Preprocessing
Data collection is the foundation of effective AI sentiment enhancement, ensuring accurate analysis and interpretation. In this phase, diverse data sources are tapped to gather relevant information, such as social media posts, customer reviews, and surveys. The preprocessing step involves cleaning this data, removing noise, and ensuring uniformity. By eliminating irrelevant content and standardizing formats, the data becomes suitable for sentiment analysis.
Subsequently, various techniques are employed for effective data preprocessing. Key steps include:
Data Cleaning: This involves removing duplicates, correcting inconsistencies, and filtering out irrelevant entries, allowing for clearer insights.
Normalization: The data is transformed into a standard format, ensuring consistency in tokenization, stemming, and lemmatization.
Sentiment Labeling: In this step, human annotators or algorithms classify data based on sentiments such as positive, negative, or neutral.
Data Annotation: This involves enriching the dataset with additional context or metadata, which helps enhance the training of AI models.
By systematically collecting and preprocessing the data, organizations position themselves to leverage AI effectively, enhancing sentiment detection accuracy in various applications.
2. AI Model Training and Fine-tuning
The process of AI Model Training and Fine-tuning is crucial for achieving effective AI Sentiment Enhancement. During this phase, machine learning algorithms are trained using large datasets to recognize and interpret emotions expressed in text. This involves selecting relevant features and labels to guide the model's understanding of sentiment. Once initial training is completed, fine-tuning allows for improved accuracy. This is accomplished by adjusting parameters, employing diverse examples, and iterating on feedback to optimize performance further.
Key steps in this training and fine-tuning process include data collection, where diverse, representative data is gathered to enhance model understanding. The next step involves model evaluation, which assesses initial results and identifies areas for improvement. Finally, continuous updates ensure the model adapts to evolving language patterns and sentiment expressions. By implementing these strategies, organizations can significantly boost their sentiment detection capabilities, resulting in more accurate insights into customer opinions and feelings.
3. Integration with Social Media Platforms
Social media platforms serve as rich sources of data for businesses seeking to enhance their AI sentiment detection capabilities. By integrating AI technology, brands can process vast amounts of social interactions, from tweets to Facebook posts, in real time. This not only helps identify customer sentiments but also allows companies to engage more meaningfully with their audiences.
The integration process involves several steps that ensure effective AI sentiment enhancement. First, businesses need to implement robust data collection methods to gather relevant information. After that, AI models must be carefully trained and fine-tuned to accurately interpret emotional nuances present in online conversations. Finally, direct integration with social media platforms enables instantaneous sentiment analysis, allowing businesses to respond to customer feedback promptly and effectively. This comprehensive approach fosters a better understanding of customer needs, ultimately leading to improved satisfaction and loyalty.
Tools for AI-Driven Sentiment Analysis
Tools for AI-Driven Sentiment Analysis play a crucial role in enhancing accuracy and providing deeper insights. Various platforms utilize advanced algorithms that can process vast amounts of data instantaneously, identifying sentiments in user feedback. By harnessing natural language processing (NLP), these tools understand context, tone, and overall sentiment, which allows businesses to make informed decisions.
Several notable tools exemplify AI sentiment enhancement capabilities. IBM Watson Natural Language Understanding provides comprehensive sentiment analysis, supporting businesses in decoding customer emotions effectively. Similarly, MonkeyLearn and Lexalytics offer user-friendly interfaces that facilitate easy integration, ensuring organizations can swiftly implement sentiment detection. Aylien stands out with its focus on news and media, providing valuable insights into public opinion trends. Each tool enhances the understanding of customer sentiment, enabling organizations to tailor strategies that resonate with their audience. Employing these tools equips businesses with a competitive edge in today's data-driven landscape.
- insight7: A leading tool in sentiment analysis
One of the foremost tools in sentiment analysis is a platform designed to simplify the process of engaging with customer data. With its intuitive interface, users can efficiently analyze interviews and extract actionable insights from customer conversations. This tool caters to various organizations, enabling them to process vast amounts of feedback in real-time, thereby enhancing their decision-making capabilities.
By adopting AI sentiment enhancement techniques, businesses can shift from traditional, time-consuming methods to a more agile approach. This shift not only improves accuracy in identifying customer sentiments but also empowers teams to collaborate more effectively. When insights are readily available and organized, organizations can quickly adapt their strategies based on customer preferences. Ultimately, the integration of this leading tool can drive significant improvements in understanding and responding to customer needs, setting businesses apart in todayโs competitive landscape.
- IBM Watson Natural Language Understanding
IBM Watson Natural Language Understanding offers powerful capabilities for enhancing sentiment detection accuracy. This tool utilizes advanced natural language processing to analyze text and discern emotional nuances. By interpreting context, it goes beyond basic sentiment analysis, enabling businesses to gain deeper insights into customer feelings.
The system employs machine learning to refine its algorithms, continually improving accuracy. It interprets language elements such as tone, intent, and sentiment scores, which aids in understanding consumer sentiment on various platforms. With its user-friendly interface, organizations can easily integrate this technology into their systems. As a result, companies can harness AI sentiment enhancement to better gauge customer perceptions and enhance overall engagement strategies. Accurate sentiment detection ultimately leads to improved decision-making and better customer experiences.
- MonkeyLearn
MonkeyLearn stands out as a robust tool for achieving AI Sentiment Enhancement in various applications. Its main strength lies in transforming unstructured data into actionable insights, thereby boosting accuracy in sentiment detection. By utilizing tailored algorithms, it effectively analyzes customer expressions across multiple channels, enabling businesses to adapt their strategies based on real-time feedback.
This tool enhances sentiment analysis by offering features like data preprocessing, personalized training of models, and seamless integration with different data sources. Moreover, companies can customize their sentiment analysis frameworks, allowing for a more precise understanding of consumer sentiment and preferences. As a result, organizations can cultivate stronger customer relationships and make data-driven decisions that resonate with their audience. Embracing this technology can ultimately lead to a more engaged and satisfied customer base, paving the way for sustained success and growth.
- Lexalytics
The role of AI in sentiment detection significantly contributes to enhancing analytics accuracy, particularly in understanding customer emotions. Utilizing advanced algorithms, sentiment detection tools effectively analyze conversations, enabling businesses to uncover customer pain points and preferences. This process not only streamlines feedback collection but also democratizes insights, empowering various teams within an organization.
One noteworthy capability is the automated extraction of relevant insights from extensive data. This includes identifying key themes, compliments, and concerns expressed by customers. By efficiently summarizing calls and feedback, companies can glean actionable information. Additionally, visualizing these insights helps in recognizing trends and areas requiring attention, thus driving informed decision-making. AI sentiment enhancement transforms raw data into comprehensible narratives, benefiting strategic planning and customer experience initiatives alike.
- Aylien
Aylien serves as a powerful catalyst in the realm of AI sentiment enhancement. This advanced tool harnesses the capabilities of natural language processing, allowing businesses to analyze textual data with exceptional accuracy. By interpreting nuances in language, Aylien enhances sentiment detection, providing insights into customer emotions and opinions that traditional methods often miss.
Employing Aylien can lead to significant improvements across various industries. For instance, businesses can utilize this tool to evaluate customer feedback on products or services, enabling a deeper understanding of consumer sentiment. Additionally, its integration with social media platforms allows companies to monitor brand reputation in real-time, swiftly reacting to negative sentiments. With the reliability of Aylien, organizations can confidently make informed decisions that enhance customer satisfaction, ultimately driving growth and loyalty in an increasingly competitive market.
Extract insights from interviews, calls, surveys and reviews for insights in minutes
AI Sentiment Enhancement in Customer Feedback Analysis
AI Sentiment Enhancement in customer feedback analysis focuses on transforming raw data into actionable insights that improve customer satisfaction. By employing advanced algorithms, businesses can effectively understand customer emotions expressed through feedback. This technology analyzes patterns within reviews or survey responses, leading to enriched insights and more informed decision-making.
To harness AI Sentiment Enhancement effectively, companies should consider several key steps. First, aggregating feedback data allows for a comprehensive view of customer opinions. Next, applying sentiment analysis algorithms ensures that both positive and negative sentiments are accurately detected. Finally, interpreting these results equips businesses with the knowledge to enhance their products or services. Embracing AI Sentiment Enhancement can lead to improved customer relations, strengthening brand loyalty and driving growth in competitive markets.
Benefits in Understanding Customer Satisfaction
Understanding customer satisfaction is crucial for businesses striving to excel. By harnessing AI sentiment enhancement, companies can gain deeper insights into customer feedback. This technology analyzes vast amounts of data, unveiling trends and patterns that traditional methods often overlook. Consequently, organizations can make informed decisions that directly address customer needs and preferences.
One of the primary benefits of understanding customer satisfaction through AI is the ability to respond swiftly to feedback. By aggregating feedback data effectively, businesses can identify issues early and adjust their strategies accordingly. Additionally, applying sentiment analysis algorithms enables companies to interpret data accurately, leading to actionable business insights. Ultimately, understanding customer satisfaction can enhance overall brand loyalty, foster improved relationships, and drive success in highly competitive markets.
- Steps to Employ AI in Customer Feedback
To effectively employ AI in customer feedback, begin by aggregating feedback data from various sources, such as surveys, social media, and support tickets. This initial step is crucial, as it creates a comprehensive dataset that reflects authentic customer sentiments. Once the data is collected, apply sentiment analysis algorithms to discern patterns in emotions and opinions. These algorithms leverage natural language processing techniques to yield insights into customer satisfaction.
After implementing AI sentiment analysis, the next key step is interpreting results to inform business decisions and strategies. This involves translating data findings into actionable insights that can enhance product development and customer service processes. Furthermore, consistent monitoring of AI-driven insights ensures ongoing improvements in understanding customer feelings and experiences, driving your business forward. With AI sentiment enhancement, organizations can foster a deeper connection with their customers and create tailored strategies to meet their needs effectively.
1. Aggregation of Feedback Data
Aggregating feedback data is a critical first step in AI sentiment enhancement. By collecting feedback from various sourcesโsuch as customer surveys, social media interactions, and online reviewsโorganizations can create a comprehensive dataset. This diverse feedback enables a clearer understanding of customer opinions, preferences, and pain points. Effective aggregation ensures that both positive and negative sentiments are captured without bias, setting the foundation for accurate sentiment analysis.
Once collected, the feedback data undergoes preprocessing, which prepares it for analysis. This includes removing irrelevant information and standardizing formats. Following preprocessing, advanced AI algorithms analyze the consolidated data to identify patterns and sentiments accurately. The insights obtained from this aggregation inform strategic decisions, allowing businesses to enhance customer experiences and tailor offerings effectively, ultimately maximizing their impact in a competitive market. By prioritizing the aggregation of feedback data, organizations can significantly improve the accuracy of sentiment detection, paving the way for targeted improvements and successful engagement with their audiences.
2. Application of Sentiment Analysis Algorithms
Sentiment analysis algorithms play a crucial role in enhancing AI's capability to detect emotional nuances in text data. By employing advanced machine learning techniques, these algorithms can analyze customer feedback, social media interactions, and reviews to determine whether sentiments are positive, negative, or neutral. This analysis allows businesses to glean meaningful insights that inform product development, marketing strategies, and customer service improvements.
The application of sentiment analysis algorithms can be broken down into key components. First, data collection and preprocessing are essential to ensure that the information fed into the algorithms is clean and relevant. Next, AI model training and fine-tuning are necessary to enhance detection accuracy. Finally, integrating these algorithms with various platforms, such as social media or customer feedback systems, maximizes their effectiveness. By utilizing these algorithms, businesses not only detect customer sentiment more accurately but also adapt to changing consumer preferences, ultimately driving better customer engagement and loyalty.
3. Interpretation of Results for Business Insights
In the realm of AI Sentiment Enhancement, interpreting results for business insights is crucial for informed decision-making. Understanding sentiment analysis outcomes allows organizations to pinpoint customer emotions and reactions accurately. By analyzing feedback, businesses can uncover valuable insights that aid in refining strategies and improving services.
Utilizing AI-driven tools enhances the interpretation of sentiment data by identifying patterns and trends across various data points. This leads to actionable insights that help in addressing customer pain points effectively. For example, data aggregation can reveal recurring issues, while sentiment algorithms provide a nuanced exploration of customer feelings. Furthermore, visualizing these results aids in simplifying complex data, making it more accessible for stakeholders. This structured approach will empower businesses to respond proactively, ensuring alignment with customer expectations and fostering loyalty.
Case Studies of AI Success Stories
In exploring the realm of AI sentiment enhancement, case studies illustrate how various organizations have successfully transformed their sentiment detection processes. One noteworthy success story revolves around a business that implemented advanced AI algorithms to analyze customer feedback more effectively. By adopting this technology, they were able to identify emotional trends and respond to customer needs in real time, significantly boosting satisfaction rates.
Another compelling example highlights a company that integrated AI sentiment analysis for its social media monitoring. This approach allowed the organization to capture intricate nuances in customer opinions, enabling them to tailor their marketing strategies accordingly. Such case studies not only demonstrate the power of AI-based sentiment detection but also provide a roadmap for others aiming to enhance their own sentiment analysis capabilities. Through these successes, businesses can glean insights that drive improvements in customer interactions and overall brand perception.
Conclusion: The Future of AI Sentiment Enhancement
As we look towards the future of AI sentiment enhancement, the potential for further advancements is immense. Continuous improvement in algorithms will increase accuracy, allowing organizations to interpret emotional nuances in communication with unprecedented precision. Greater reliance on AI in various sectors will facilitate enhanced customer understanding and enrich engagement strategies.
Emerging technologies promise to integrate AI sentiment enhancement seamlessly into broader analytical frameworks. This will enable businesses to not only react to customer sentiments but also forecast trends and behaviors with greater confidence. Embracing these capabilities can empower companies to create genuinely personalized experiences, fostering stronger relationships with their customer base.