Real-time AI metrics are transforming the landscape of quality assurance by shifting how organizations monitor and evaluate performance. Gone are the days when assessments relied solely on traditional, time-consuming review processes. Instead, embracing real-time AI metrics allows for immediate insights, enabling teams to respond to quality issues as they emerge.
These metrics provide a dynamic approach to quality assurance that enhances both accuracy and efficiency. Companies can now track performance across various channels with unprecedented precision. By integrating real-time AI into their QA processes, businesses not only streamline operations but also foster a culture of continuous improvement that meets the demands of today's fast-paced environment.
Analyze qualitative data. At Scale.
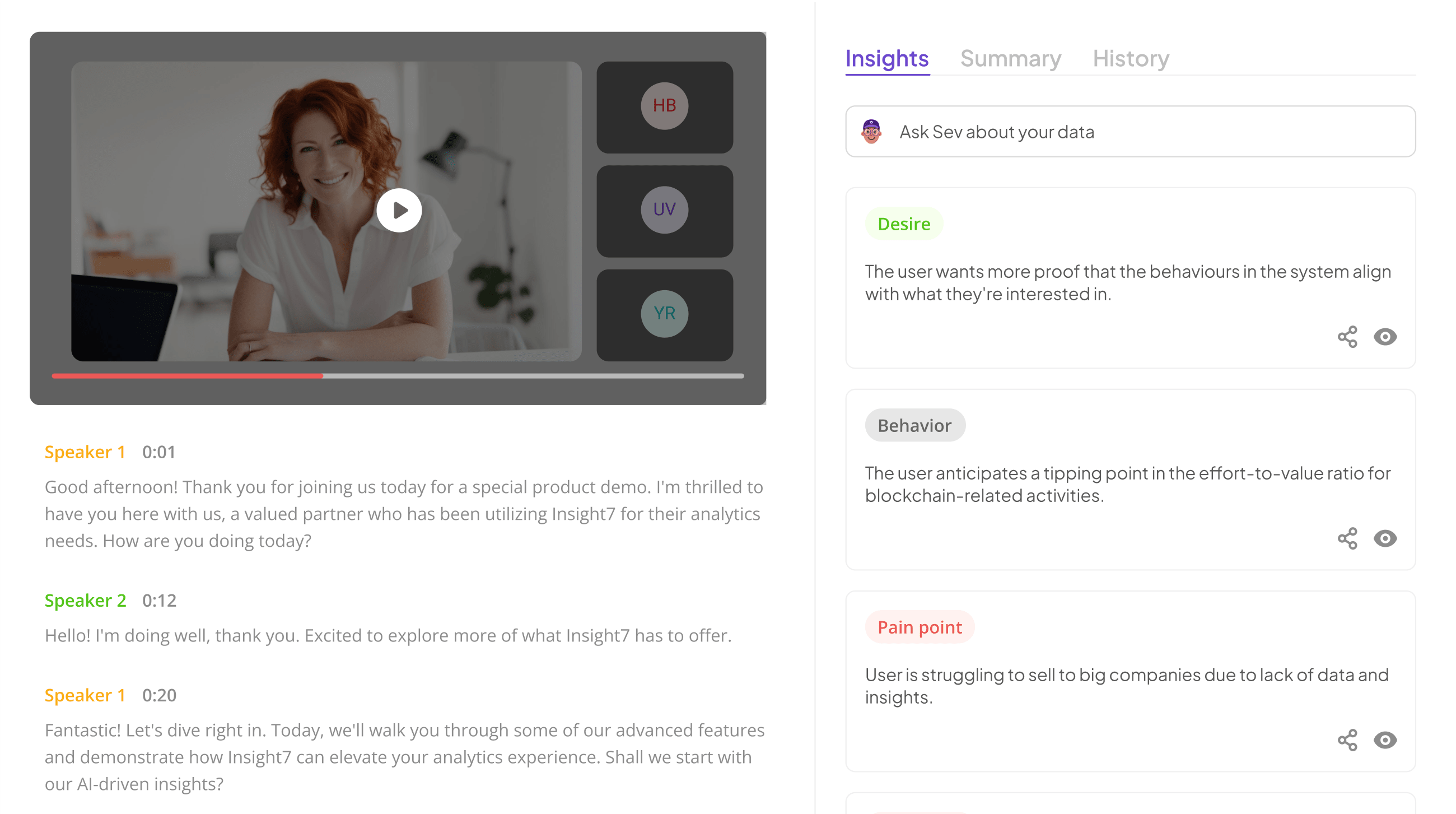
Understanding Real-Time AI Metrics for QA
Understanding Real-Time AI Metrics for QA allows organizations to fundamentally transform their quality assurance processes. Unlike traditional methods, which often rely on retrospective evaluations, real-time metrics provide instantaneous feedback, allowing teams to catch issues as they arise. This immediate insight can lead to an improved user experience and more efficient operational practices.
Real-Time AI Metrics are not just about data collection; they also emphasize actionable insights. For instance, these metrics can track performance across various parameters such as accuracy, speed, and user satisfaction. By focusing on these elements, organizations can adapt their strategies dynamically, responding to challenges in real time rather than waiting for periodic reviews. Embracing this innovative approach prepares businesses for a future where quality assurance is proactive, leveraging technology to enhance product offerings and customer interactions effectively.
The Shift from Legacy QA Reviews to Real-Time Monitoring
The shift from legacy QA reviews to real-time monitoring represents a fundamental change in how quality assurance functions are approached. Traditional methods often involved time-consuming evaluations after processes were completed, relying on static reports that provided insights too late for actionable change. In contrast, real-time monitoring allows for the immediate collection and analysis of quality metrics, enabling teams to address issues on the spot. Furthermore, organizations can harness Real-Time AI Metrics to gain continuous feedback and insights, significantly enhancing their responsiveness.
Companies increasingly find that analyzing customer interactions and feedback in real-time leads to improved service delivery and better decision-making. By utilizing these advanced metrics, teams can seamlessly identify recurring problems, inherent trends, and customer satisfaction levels without delay. This dynamic approach not only fosters a proactive culture but also cultivates a more robust framework for quality assurance, positioning businesses ahead of their competition in an ever-evolving market.
Key Benefits of Real-Time AI Metrics in QA
Real-Time AI Metrics in Quality Assurance provide significant advantages that enhance operational efficiency and improve overall quality. First, they offer immediate feedback, allowing teams to identify issues and address them promptly. This leads to enhanced teams’ engagement with the QA process, as they are equipped with tools for continuous improvement. Additionally, these metrics enable data-driven decision-making, ensuring that strategies are grounded in quantitative insights rather than subjective analysis.
Moreover, the adoption of Real-Time AI Metrics reduces the need for extensive manual reviews, facilitating quicker turnaround times for quality assessments. This not only streamlines processes but also alleviates the burden on quality teams, enabling them to focus on strategic initiatives. By embracing Real-Time AI Metrics, organizations can foster a proactive culture where quality is embedded into every stage of the operation, ultimately driving better customer satisfaction and loyalty.
Essential Real-Time AI Metrics for QA
In today's fast-paced digital environment, Essential Real-Time AI Metrics for QA play a pivotal role in elevating quality assurance processes. These metrics offer immediate insights that replace traditional, lagging methodologies, enabling teams to respond promptly to potential issues in real time. By harnessing advanced technology, organizations can monitor performance continuously, ensuring higher accuracy and user satisfaction.
Among the key metrics to consider are accuracy and precision analysis, which evaluate the effectiveness of AI systems in delivering reliable outputs. Understanding real-time user metrics, such as satisfaction scores, allows teams to gain valuable insights directly from user interactions. By prioritizing these essential metrics, organizations can create a robust framework that no longer relies on outdated QA reviews, leading to an overall enhancement of product quality and user experience. This transition not only optimizes operational efficiency but also fosters a culture of continuous improvement.
Accuracy and Precision Analysis
Accuracy and Precision Analysis is crucial for understanding the effectiveness of real-time AI metrics in quality assurance. These metrics enable organizations to monitor interactions and outcomes closely, allowing for immediate corrections based on data-driven insights. Accuracy refers to how closely a measured value aligns with the true value, while precision assesses the consistency of measurements, regardless of their accuracy.
Establishing a balanced framework for accuracy and precision empowers teams to evaluate AI performance effectively. Organizations can pinpoint specific areas of improvement by consistently analyzing these metrics. For instance, a high accuracy rate might suggest that the AI tool correctly identifies interaction outcomes, while enhanced precision indicates that repeated measurements yield consistent results. Together, these insights lead to better decision-making, enhancing overall service quality and customer satisfaction. Emphasizing accuracy and precision in real-time AI metrics serves as a foundation for transitioning from traditional QA reviews to a more dynamic, responsive approach.
Real-Time AI Metrics for User Satisfaction
Real-time AI metrics play a crucial role in assessing user satisfaction, enabling businesses to gather insights immediately as users engage with products. These metrics allow organizations to analyze user interactions on a granular level, understanding pain points and preferences in real time. The ability to respond swiftly to user feedback can significantly enhance product quality and customer satisfaction.
One of the key metrics includes sentiment analysis, which evaluates user emotions expressed in feedback or comments. Additionally, engagement rates provide a clear picture of how users interact with different features of a product. Monitoring user satisfaction through prompt surveys also fosters a deeper understanding of customer needs. Finally, churn prediction metrics can identify at-risk users before they disengage. By utilizing these advanced metrics, organizations can shift from reactive to proactive strategies, ensuring they meet user expectations effectively and efficiently.
Extract insights from interviews, calls, surveys and reviews for insights in minutes
Step-by-Step Implementation of Real-Time AI Metrics
Implementing Real-Time AI Metrics begins with identifying core quality metrics tailored to your specific processes. These metrics serve as benchmarks, allowing organizations to measure performance and areas that require improvement. Understanding the needs of your team and the types of insights desired forms the foundation of this transition. Key performance indicators (KPIs) should align with user satisfaction, accuracy, and operational efficiency to replace legacy QA reviews effectively.
Next, integrating Real-Time AI tools into your QA processes is vital. Start by evaluating your current QA framework, identifying gaps that AI can address. Choose robust AI tools that fit seamlessly into existing workflows; popular options include metrics for accuracy and user feedback. Once implemented, continuous monitoring is essential, ensuring that metrics provide actionable insights. Regularly reviewing the data aids in refining processes, fostering a culture of continuous improvement and adaptation. By following this structured approach, organizations can transition smoothly and efficiently into next-generation quality assurance practices.
Identifying Core Quality Metrics
Identifying core quality metrics is essential for transforming quality assurance practices from traditional methods to a more dynamic, responsive approach. Real-time AI metrics enable organizations to capture and analyze data as it occurs, allowing for timely adjustments and improvements. By defining these key metrics, teams can better understand aspects of performance that require attention and enhancement.
To effectively identify these metrics, organizations can focus on the following areas:
- Call Evaluation Metrics: Assessing the effectiveness and accuracy of interactions helps fine-tune training and support strategies.
- Customer Feedback Integration: Evaluating qualitative insights from customers can highlight areas that require immediate action or improvement.
- Performance Consistency: Monitoring team performance over time identifies trends and potential issues before they escalate.
By implementing these core metrics, companies can shift toward a focus on continuous improvement, ensuring that quality assurance evolves alongside consumer expectations and technological advancements.
Integrating Real-Time AI Tools into QA Processes
Integrating Real-Time AI tools into QA processes involves a strategic shift from traditional methods to advanced technologies that enhance quality assurance. Companies must first evaluate their current QA frameworks to identify areas needing improvement. This assessment not only helps in pinpointing specific pain points but also paves the way for the adoption of effective Real-Time AI Metrics.
Once the evaluation is complete, the next step is to select and deploy appropriate AI tools that align with business objectives. These tools can provide instant feedback and actionable insights, allowing for quicker adjustments in processes. The implementation of Real-Time AI Metrics streamlines communication within teams, reduces inefficiencies, and ultimately leads to improved product quality. By embracing these technologies, organizations can stay competitive, respond rapidly to customer signals, and foster a culture of continuous improvement in their QA processes.
Step 1: Evaluate Current QA Framework
Evaluating the current QA framework is essential for a successful transition to Real-Time AI Metrics. Begin by assessing existing processes, tools, and performance metrics. This evaluation allows you to identify gaps and inefficiencies that need addressing. Consider the aspects of your framework that align with modern data-driven practices. A thorough understanding of existing systems and their limitations will serve as a foundation for integrating new metrics and technologies effectively.
Next, collaborate with relevant stakeholders, such as QA teams and data analysts, to gather insights on current challenges. This process aligns your goals with organizational needs and ensures a smooth integration of Real-Time AI Metrics. Explore current performance indicators to understand how they can evolve into more dynamic, real-time assessments. By engaging in this evaluation phase, you pave the way for adopting metrics that enhance quality assurance and drive performance improvements.
Step 2: Select and Deploy Appropriate AI Tools
Selecting and deploying appropriate AI tools is crucial for effectively implementing Real-Time AI Metrics in quality assurance systems. First, assess your organization's specific needs and identify the metrics that matter most for your operations. This means understanding the pain points experienced in legacy QA reviews, such as time delays and lack of actionable insights. Choose AI tools that are user-friendly and require minimal training, allowing teams to access and utilize insights with ease.
Next, prioritize tools that offer robust analytics capabilities. Look for solutions that can visualize conversations, extract pain points, and summarize insights efficiently. Ensure these tools can handle large datasets, enabling comprehensive analysis across multiple projects simultaneously. Deploying the right AI tools not only streamlines workflow but also enhances the accuracy and relevance of quality assessments, thus replacing outdated methods and providing a scalable approach to quality assurance. By harnessing the power of Real-Time AI Metrics, businesses can drive continuous improvement and customer satisfaction.
Top AI Tools for Real-Time Quality Metrics
In the ever-evolving landscape of quality assurance, adopting effective tools for real-time AI metrics has become paramount. Several top AI platforms stand out, facilitating instant insights into quality performance. Insight7 is noted for its user-friendly interface, aiding teams in analyzing customer interactions swiftly. Its capabilities empower businesses to transform insights into actionable strategies seamlessly.
Additionally, Applitools offers visual testing solutions that help ensure application interfaces meet user expectations. Testim simplifies test automation through AI-driven approaches, while Functionize leverages machine learning to enhance testing processes. These tools collectively redefine how organizations monitor quality, enabling a shift from legacy methods to agile, real-time assessments. By embracing these innovative solutions, companies can significantly expedite their QA processes, allowing for enhanced decision-making and improved customer satisfaction.
Insight7
In the realm of quality assurance, Insight7 highlights the importance of adopting Real-Time AI Metrics to transform traditional processes. Organizations are increasingly inundated with customer data, making it essential to implement tools that provide instant insights. This method not only elevates the efficiency of analyzing customer feedback but also enhances the ability to make informed decisions rapidly. The challenge lies in the inadequacy of legacy systems to handle this fast-paced data flow effectively.
To harness the power of Real-Time AI Metrics, companies can focus on several key aspects. First, accuracy and precision must be thoroughly analyzed to ensure reliability. Second, assessing user satisfaction in real-time helps businesses stay agile and responsive to customer needs. Finally, integrating advanced AI technologies into existing QA frameworks can streamline operations, making it easier to respond to evolving market demands. Embracing these metrics is not just a trend but a significant move toward sustainable operational excellence.
Applitools
In the realm of quality assurance, real-time AI metrics have revolutionized how organizations monitor and evaluate their processes. One notable tool stands out for its capability to offer visual testing and intelligent monitoring, thus ensuring that applications meet high-quality standards. By employing advanced algorithms, this solution automatically detects visual discrepancies that could impact user experience, allowing teams to address issues proactively.
Moreover, the integration of these real-time AI metrics into daily operations empowers teams to focus on critical aspects of software quality. They can gain immediate insights into user satisfaction and application performance, aiding in the swift identification of problem areas. This shift from traditional methods enhances collaboration across departments, fostering a culture of continuous improvement. As organizations embrace these metrics, they can better ensure product excellence and satisfy customer needs effectively.
Testim
Testim plays a pivotal role in the transition to Real-Time AI Metrics, enhancing quality assurance processes drastically. It empowers teams by automating and streamlining testing procedures, making them more efficient and accurate. This innovative platform allows for immediate feedback on application performance, ensuring that developers can quickly identify and resolve issues.
Utilizing Testim's capabilities, organizations can shift away from traditional, slower QA reviews to a more agile and responsive quality assurance model. This shift not only increases productivity but also boosts overall product quality. Furthermore, the integration of Real-Time AI Metrics facilitates continuous monitoring and evaluation, allowing teams to maintain high standards as they adapt to changing user needs. By embracing Testim, companies can confidently navigate the complexities of modern software development, ensuring a seamless user experience while meeting their quality benchmarks.
Functionize
Functionize transforms quality assurance by utilizing innovative technology to provide real-time insights. This platform shifts the focus from traditional QA reviews, allowing teams to access and analyze data without the need for expert training. With its user-friendly interface, anyone in an organization can explore key metrics and generate insights quickly, democratizing the process of data analysis.
The primary feature of Functionize is its ability to analyze customer interactions and extract crucial pain points and desires from conversations. By employing real-time AI metrics, the platform enhances the understanding of user experiences, enabling businesses to address issues swiftly. Furthermore, the capacity to visualize data and uncover significant trends from multiple calls simultaneously streamlines decision-making processes. Ultimately, Functionize represents a significant step towards modernizing quality assurance, offering robust solutions that prioritize efficiency and user-centric insights.
Conclusion: Transitioning to Real-Time AI Metrics for Superior Quality Assurance
Transitioning to real-time AI metrics signifies a pivotal shift in Quality Assurance (QA) practices. By embracing these metrics, organizations can streamline their quality processes, ultimately enhancing product reliability and customer satisfaction. Real-time AI metrics provide immediate feedback, enabling teams to swiftly identify issues and make informed decisions that reflect current market demands.
Moreover, implementing real-time AI metrics fosters a culture of continuous improvement. This transformative approach allows businesses to move away from traditional, time-consuming reviews toward a more dynamic, data-driven process. Consequently, organizations can achieve superior quality assurance and stay competitive in a fast-evolving landscape. The transition is not merely beneficial; it sets the foundation for long-term success and customer trust.