Escalation Predictive Analytics offers transformative insights for call centers, enabling them to preemptively address customer concerns. By analyzing historical data, these analytics identify patterns that predict when a call might escalate beyond initial support. Recognizing these potential escalations not only enhances customer satisfaction but also streamlines resolution processes.
In practice, Escalation Predictive Analytics involves examining various factors such as call duration, customer sentiment, and agent performance. Understanding these predictors helps organizations prioritize training, allocate resources effectively, and ultimately improve overall service quality. By harnessing this powerful analytical capability, call centers enhance their strategies for managing complex customer interactions.
Analyze & Evaluate Calls. At Scale.
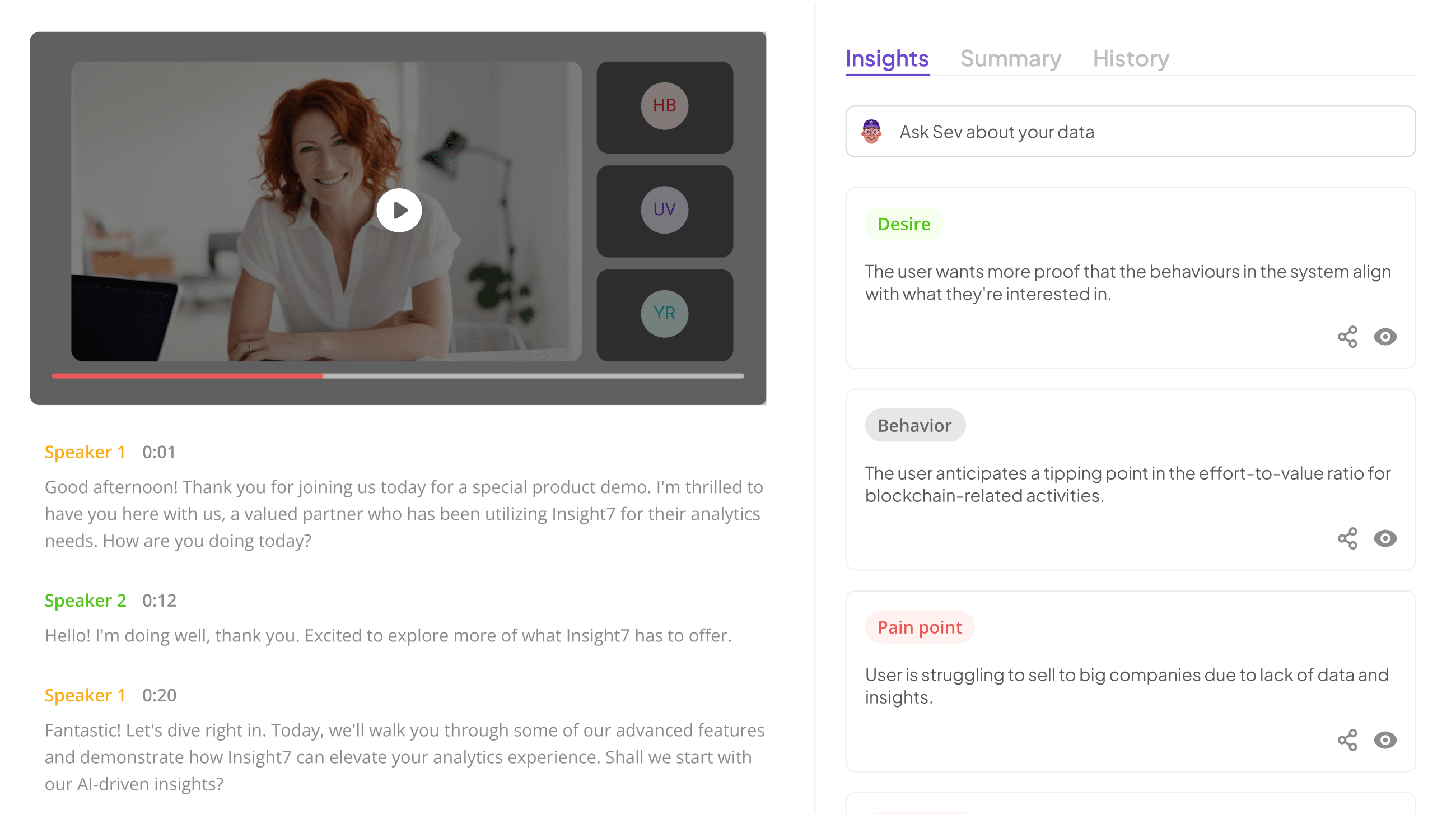
Understanding Call Escalation Predictive Analytics
Understanding Call Escalation Predictive Analytics involves grasping how analytics platforms can forecast when a call might need to be escalated. This forecast can help call centers manage resources effectively and enhance customer satisfaction. By analyzing various data points, such as call duration, frequency of similar issues, and customer sentiment, predictive analytics identifies trends that signal potential escalations.
The primary aim of escalation predictive analytics is to preemptively address issues before they escalate to higher-level support. This approach involves two key components: data indicators and behavioral patterns. Data indicators like call volume and resolution times provide essential insights into operational efficiency. Meanwhile, analyzing behavioral patterns can highlight customer frustrations, allowing agents to act swiftly to resolve issues. Ultimately, effective utilization of these insights positions call centers to improve both their service delivery and customer experiences.
What is Call Escalation?
Call escalation refers to the process of transferring a customer call to a higher authority or specialized agent when the current representative cannot resolve the issue. This often occurs in call centers where complex problems or customer dissatisfaction arise. Recognizing when to escalate a call can significantly impact customer satisfaction and operational efficiency. Understanding call escalation dynamics is essential for effective customer service management.
Escalation Predictive Analytics aims to identify specific triggers or indicators that suggest a call may need to be escalated. These indicators can include the customer’s tone of voice, the complexity of the issue, or even historical data related to similar calls. By analyzing these factors, call centers can proactively prepare representatives and improve overall customer interaction. Therefore, integrating predictive analytics into escalation processes can enhance both representative training and customer outcomes, leading to a more streamlined service experience.
In summary, understanding call escalation involves recognizing the importance of timely intervention and employing data-driven strategies to enhance customer support effectiveness.
The Role of Predictive Analytics in Call Centers
Predictive analytics transforms call centers into proactive environments by identifying escalation predictors that can enhance customer satisfaction. These analytics techniques analyze historical call data, helping to forecast which interactions may require additional assistance or supervision. With accurate data, call centers can streamline their processes, reduce average handling times, and ultimately provide a better experience for customers.
Understanding escalation predictive analytics allows call center managers to implement targeted training initiatives based on frequent customer inquiries. Analytics can reveal specific topics or issues that lead to escalated calls. By effectively addressing these areas, the call center can optimize training for customer service representatives (CSRs), enhancing their ability to resolve concerns on the first contact. Continual improvement driven by insights from predictive analytics can lead to reduced escalations, increased efficiency, and higher customer satisfaction levels.
Key Predictors in Escalation Predictive Analytics
In the realm of Escalation Predictive Analytics, specific data indicators can remarkably inform decision-making processes in call centers. First and foremost, call duration is a crucial metric. Longer calls may suggest unresolved issues, signaling a higher likelihood of escalation. Similarly, customer sentiment analysis serves as an equally vital predictor. Negative feedback patterns can be early warnings, prompting proactive measures to defuse potential escalations.
Another significant predictor lies in customer behavior patterns. Understanding how frequently a customer contacts support can uncover trends. Repeat contacts often indicate dissatisfaction, raising the risk of escalation. Furthermore, agent performance metrics should not be overlooked. Analyzing agent interactions can reveal which representatives may be struggling, allowing for targeted training. By focusing on these predictors, call centers can enhance their predictive models, ultimately improving customer satisfaction and reducing escalation instances.
Data Indicators and Their Importance
Data indicators play a crucial role in understanding and predicting call escalation outcomes in analytics platforms. By analyzing various metrics, organizations can identify potential escalation scenarios before they occur. These indicators provide insights that help in recognizing underlying issues in customer interactions. For instance, certain keywords in calls or customer sentiment trends may signal an increased chance of escalation.
Utilizing these data indicators empowers teams to implement proactive strategies. For example, if an indicator shows a pattern of dissatisfaction, agents can be trained to address specific concerns more effectively. Furthermore, the importance of continuous analysis cannot be overstated. Regularly monitoring data ensures that organizations remain agile and adapt to changing customer needs, ultimately improving service quality and customer satisfaction. Emphasizing the significance of these indicators helps cultivate a data-driven culture, vital for excelling in call escalation predictive analytics.
Behavioral Patterns Analysis
Behavioral Patterns Analysis plays a crucial role in understanding why certain interactions escalate within call centers. Within this context, identifying common behaviors and patterns among agents and customers can provide valuable insights. For instance, prolonged response times or unresolved issues often lead to heightened frustration. Recognizing these patterns allows call center managers to implement preventative measures.
Moreover, analyzing past interactions helps in forecasting potential escalations. By applying Escalation Predictive Analytics, organizations can segment data into meaningful categories. This might include examining customer sentiment from feedback or identifying recurring themes from unresolved calls. By doing so, call centers can better prepare their staff to handle similar situations in the future, ultimately improving both customer satisfaction and operational efficiency. Understanding how to interpret these behavioral patterns is essential for effective decision-making and resource allocation.
Extract insights from interviews, calls, surveys and reviews for insights in minutes
Steps to Implementing Escalation Predictive Analytics
To effectively implement escalation predictive analytics, the process begins with identifying key data sources. This involves gathering information from various channels, such as call logs, customer feedback, and agent performance metrics. Proper data integration ensures that the analytics model has a diverse range of inputs, enhancing its predictive accuracy.
Next, setting up analytical models is essential for interpreting the collected data. Choose suitable algorithms and techniques that can identify patterns in customer interactions, highlighting potential escalation points. This is where machine learning can play a crucial role in refining the predictions based on historical data.
Finally, continuous monitoring and optimization of the model are vital steps. As new data flows in, adjusting the analytical models accordingly will help maintain accuracy and relevance. Regular assessments will also enable businesses to adapt their strategies based on evolving customer behaviors and organizational needs, driving improved outcomes in call management.
Step 1: Identifying Key Data Sources
Identifying key data sources is a vital first step in performing Escalation Predictive Analytics effectively. Start by gathering data from multiple channels, such as customer calls, chat interactions, and email communications. These data points provide a comprehensive view of customer behavior and potential escalations. Each source enriches your analysis, helping to identify patterns that indicate when an issue may escalate.
Next, assess the quality and relevance of your data. This involves looking for trends within specific datasets, like common customer complaints or recurring issues during support calls. Utilize tools that allow you to cross-reference different information pieces, ensuring consistent and actionable insights. By thoroughly understanding where your data originates, you can build a robust predictive model that effectively anticipates call escalations, ultimately enhancing your call center's response strategy.
Step 2: Setting Up Analytical Models
Setting up analytical models is a crucial step in the implementation of escalation predictive analytics. In this phase, it's essential to select the right data sets and determine which variables will effectively influence prediction outcomes. By clearly identifying the patterns present in past escalation data, you can build a robust model that anticipates future call trends.
Start by exploring different algorithms that can suit your dataset, whether it's regression models or machine learning techniques. Documenting the rationale behind your selections is vital for later refinement stages. Next, ensure your models are trained and validated against historical data, enhancing their predictive accuracy. Testing different scenarios will allow you to fine-tune the model, ensuring it adapitates to the ever-changing dynamics of customer interactions. This iterative process not only boosts the effectiveness of escalation strategies but also significantly improves overall customer satisfaction.
Step 3: Continuous Monitoring and Optimization
Continuous monitoring and optimization are crucial for effective escalation predictive analytics. After implementing your analytical models, regularly reviewing their performance ensures that the insights remain relevant and actionable. This ongoing process allows organizations to identify trends, anomalies, and areas for improvement in real time. It’s essential to stay vigilant and adjust criteria based on evolving business needs or customer interactions.
Moreover, continuous optimization means refining existing models based on what data suggests, enhancing prediction accuracy. By responding to these insights, teams can proactively address potential escalation triggers, ensuring they remain responsive to customer needs. Creating a feedback loop with agents helps further clarify which strategies work best in practice, ultimately leading to improved call management and customer experiences.
Top Tools for Escalation Predictive Analytics
When exploring the realm of escalation predictive analytics, it's essential to understand the tools that can enhance your call center operations. Several notable platforms stand out for their ability to optimize workflows and deliver valuable insights. Top tools such as Zendesk and Freshdesk not only facilitate efficient ticket management but also analyze customer interactions, identifying patterns that may lead to escalations.
Genesys and Salesforce Service Cloud are critical for integrating data from various sources, offering detailed analytics that help anticipate customer needs. Moreover, insight7 shines with its user-friendly interface, allowing teams to easily visualize call data and extract meaningful insights. These platforms empower businesses to proactively manage customer interactions and prevent unnecessary escalations, ultimately leading to improved customer satisfaction and retention. The right tool can revolutionize how your organization approaches escalation predictive analytics, fostering a more responsive and informed service environment.
insight7
The integration of escalation predictive analytics in modern call centers transforms traditional customer service approaches. By examining key predictors, organizations can anticipate when a customer interaction may need to escalate beyond initial support tiers. This proactive strategy not only aids in improving customer satisfaction but also enhances operational efficiency.
Key factors influencing escalation include customer sentiment during interactions, response times, and the complexity of inquiries. For example, customers exhibiting frustration may require immediate intervention from higher-level support. Likewise, systems that analyze conversation patterns can help identify issues that typically lead to escalations. By implementing these insights, companies position themselves to respond swiftly and effectively, ultimately creating better outcomes for both the customer and the organization.
Understanding these dynamics allows organizations to streamline their processes, enhancing their ability to serve customers effectively while maintaining a competitive edge.
Zendesk
In the realm of customer support, certain tools stand out in facilitating effective communication and resolution strategies. One such tool offers insights into the patterns that signal when an escalation is likely to occur. By harnessing Escalation Predictive Analytics, organizations can better anticipate customer needs, reducing frustration and improving satisfaction rates.
The key components of this tool involve analyzing various data indicators, such as call duration, customer sentiment, and agent responses. Each variable feeds into a broader understanding of the customer experience. Behavioral patterns also play a crucial role. Understanding how customers typically react in certain scenarios allows teams to implement proactive measures. In this way, support teams can modify their approaches based on predictive insights, leading to an environment where escalations are managed with greater efficiency and effectiveness.
Freshdesk
Freshdesk offers a comprehensive approach to call escalation predictive analytics, enabling teams to enhance customer service management. By harnessing data from various interactions, it helps identify crucial patterns that may signal an impending escalation. Understanding these predictors allows customer service teams to intervene proactively, thus improving the overall customer experience.
At the core of Freshdesk’s capabilities are its robust analytical tools that interpret data points effectively. For instance, it can analyze call transcripts to uncover customer pain points or behavioral trends that might trigger escalations. When trained to recognize these signals, customer support agents can prepare strategies to manage potentially difficult conversations, streamlining processes and enhancing resolution times. This proactive stance on call management not only boosts efficiency but also fosters greater customer satisfaction and loyalty.
Genesys
In the realm of escalation predictive analytics, understanding the essence of data-driven decision-making is critical. Advanced analytics tools focus on identifying indicators that signal when a call may need to be escalated. This proactive approach in customer service enhances efficiency and elevates the overall user experience. Organizations can analyze customer interactions to determine patterns and signals that predict future escalation needs.
Key features of effective escalation predictive analytics include behavior analysis and scenario forecasting. By examining past interactions, companies can pinpoint recurring issues that prompt escalations. Further, integrating predictive models helps in crafting tailored responses to customer needs. Ultimately, the goal is to foster a more engaging customer service environment where representatives are equipped to address concerns promptly, reducing the need for escalations and enhancing customer satisfaction. Predictive capabilities lead to improved outcomes as businesses adapt their strategies to respond effectively to customer dynamics.
Salesforce Service Cloud
Salesforce Service Cloud is a powerful platform tailored for customer service and support teams. It enhances interaction by providing agents with tools to streamline communication and resolve issues effectively. With its robust features, organizations can leverage data to make informed decisions and improve service quality.
In the context of escalation predictive analytics, Salesforce Service Cloud plays a crucial role. It helps identify potential escalation scenarios by analyzing customer interactions and patterns. This insight enables teams to intervene proactively, reducing the likelihood of escalated calls. By monitoring customer behavior and sentiment, organizations can fine-tune their strategies and enhance customer satisfaction.
Ultimately, adopting Salesforce Service Cloud equips teams with necessary tools to maximize efficiency in handling customer inquiries while minimizing escalations. This proactive approach significantly contributes to maintaining service excellence and fosters long-term customer loyalty.
Conclusion: The Future of Escalation Predictive Analytics
As organizations continue to embrace Escalation Predictive Analytics, the future holds promising advancements that will reshape how support teams interact with customers. Enhanced algorithms and machine learning will enable platforms to analyze vast datasets more efficiently, identifying subtle patterns that indicate escalation risks. This progress will empower call centers to preemptively address issues, reducing customer frustration and improving satisfaction rates.
Moreover, as companies integrate these predictive models into their workflows, real-time feedback will become invaluable. Insights derived from analytics will not only drive immediate interventions but also inform long-term strategies for enhancing customer service. Embracing this future means prioritizing data-driven decision-making and cultivating a culture that values proactive responses over reactive measures.