AI Voice Analysis is revolutionizing quality assurance by offering innovative ways to assess interactions effectively. As businesses face increasing demands for accuracy in performance evaluation, traditional methods often fall short. The integration of AI enables the analysis of voice patterns and stress levels, providing insights that can enhance operational efficiency. This technology is designed to detect nuances in conversations that human evaluators might miss, allowing for a more comprehensive quality assessment.
With AI Voice Analysis, organizations can benefit from data-driven decision-making, ultimately improving customer satisfaction. As we explore its accuracy and reliability in voice stress detection, it becomes crucial to understand the underlying technology and its implications for quality assurance practices. The accuracy of AI in measuring stress levels can shape the future of compliance and performance evaluations across industries.
Analyze & Evaluate Calls. At Scale.
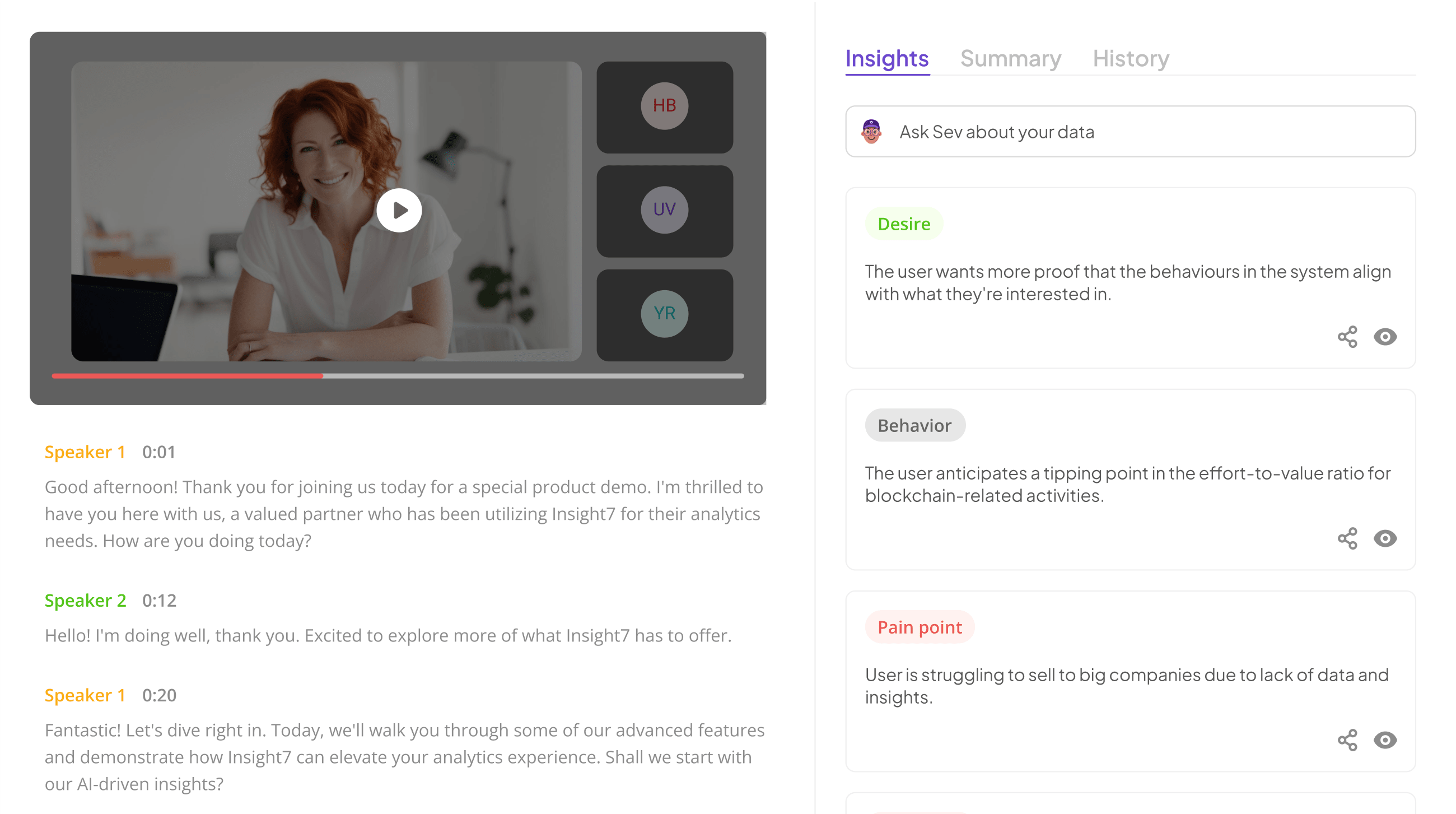
The Role of AI Voice Analysis in Stress Detection
AI Voice Analysis plays a pivotal role in stress detection by analyzing vocal patterns and identifying subtle changes triggered by emotional states. When a person's stress levels rise, their voice can exhibit specific characteristics, such as increased pitch or changes in tone. This technology uses machine learning algorithms to interpret these nuances and provide insights into emotional well-being, thus allowing for timely intervention in various contexts, from call centers to mental health assessments.
The effectiveness of AI in voice analysis lies in its ability to process vast amounts of data quickly and accurately. By harnessing advanced analytical techniques, AI can uncover hidden patterns that may elude human analysts. This capability not only enhances the accuracy of stress detection but also underscores the necessity for ongoing research and development in the field. As accuracy concerns persist, understanding how AI Voice Analysis can evolve to meet industry standards remains crucial for ensuring its reliability in quality assurance processes.
Understanding Voice Stress Detection Technologies
Voice stress detection technologies utilize sophisticated algorithms to analyze vocal parameters, helping to identify stress levels through voice analysis. These systems often focus on features such as pitch, tone, and speech patterns, which can subtly change under different emotional states. AI voice analysis represents a leap forward in understanding how individuals express themselves, capturing nuances that might evade human detection.
Moreover, advancements in AI contribute to enhancing the accuracy of these technologies. By leveraging machine learning, systems can adapt and improve their detection capabilities based on vast datasets. This evolution raises questions about reliability and accuracy, as variations in individual speaking styles can affect the outcomes. Understanding the mechanisms behind voice stress detection is essential to assess the potential of AI in quality assurance measures effectively. When harnessed appropriately, AI voice analysis can provide valuable insights for businesses aiming to enhance communication standards and overall performance metrics.
The Science Behind AI Voice Analysis
AI Voice Analysis relies on advanced algorithms that interpret various vocal attributes to assess emotional and psychological states. It captures elements like pitch, tone, and speech patterns, which collectively help in identifying stress levels in a speaker's voice. By analyzing these vocal characteristics, AI can derive insights into a person's emotional well-being, allowing for effective stress detection.
The science behind this technology integrates multiple disciplines, including linguistics, psychology, and machine learning. The AI model processes audio data, transforming it into meaningful patterns that reflect stress indicators. Notably, accuracy is contingent upon the quality of the input data and the robustness of the algorithms employed. Understanding how these factors interplay is crucial for ensuring that AI Voice Analysis serves its intended purpose in quality assurance applications. As developments continue, refinement in AI techniques promises enhanced precision in voice stress detection.
Extract insights from interviews, calls, surveys and reviews for insights in minutes
Accuracy and Reliability of AI Voice Analysis in QA
AI Voice Analysis is transforming quality assurance by enhancing how organizations assess interactions and conversations. However, this technology's accuracy and reliability are subjects of ongoing scrutiny. Various elements influence its performance, including voice recognition algorithms, contextual understanding, and environmental factors. As a result, users can expect a general accuracy rate of around 80% to 90%, which, while promising, still leaves room for improvement.
Reliability in AI Voice Analysis is further affected by the capability to accurately identify speakers and analyze emotional undertones in their voices. Contextual awareness plays a crucial role in minimizing misunderstandings, making it essential to consider the specifics of each interaction. Consequently, organizations should be aware of these influencing factors when implementing AI in their quality assurance processes. Balancing technological limitations with the nuances of human communication remains vital for achieving optimal outcomes in voice stress detection.
Factors Influencing Accuracy in Voice Stress Detection
Several factors significantly influence the accuracy of voice stress detection in the context of AI voice analysis. One prominent aspect is the quality of audio input, which can be compromised by background noise or poor recording conditions. High-quality recordings allow the algorithm to analyze vocal nuances more effectively, leading to more accurate stress and emotion detections.
Additionally, the algorithms used for voice analysis play a crucial role in determining accuracy. Variations in machine learning models can result in different interpretations of vocal data. Itโs essential to continuously train these models on diverse datasets to ensure they can recognize stress in various contexts and vocal characteristics. Furthermore, speaker variability, including accent and pitch, can impact detection fidelity. Thus, personalization and ongoing adjustments to the AI voice analysis systems are necessary to enhance their performance across different speakers and situations.
Comparative Analysis: AI Voice Analysis vs. Traditional Methods
AI Voice Analysis has emerged as a disruptive force in the realm of voice stress detection, presenting a compelling alternative to traditional methods. Traditional techniques often rely on subjective evaluations by trained professionals, which can lead to inconsistencies and biases. In contrast, AI Voice Analysis utilizes advanced algorithms to objectively assess vocal patterns and stress indicators, minimizing human error and variability.
When comparing the two methods, we notice a few key distinctions. First, the efficiency of AI systems typically allows for faster analysis, producing results in a matter of minutes rather than hours. Second, AI can process large volumes of data simultaneously, providing insights across multiple calls, something traditional methods struggle to achieve. Lastly, AI systems can continually learn from new data, thereby enhancing their accuracy over time. Overall, while traditional methods offer valuable insights, AI Voice Analysis holds significant potential for improving quality assurance in voice stress detection.
Conclusion: Evaluating the Future of AI Voice Analysis in QA
As we look to the future of AI voice analysis in quality assurance, its potential becomes more significant. This technology can streamline processes by providing quick insights into call performance, enabling organizations to improve their service quality. However, challenges remain in ensuring accuracy and reliability, particularly in voice stress detection, which is crucial for assessing emotional responses.
Moving forward, continuous advancements in AI will likely enhance its precision, thereby building trust among users. As companies implement AI voice analysis solutions, they must prioritize transparency and customization to meet their unique needs. This evolution could make AI voice analysis an indispensable tool in the QA landscape, driving improved outcomes and higher satisfaction for both customers and agents.
Analyze & Evaluate Calls. At Scale.
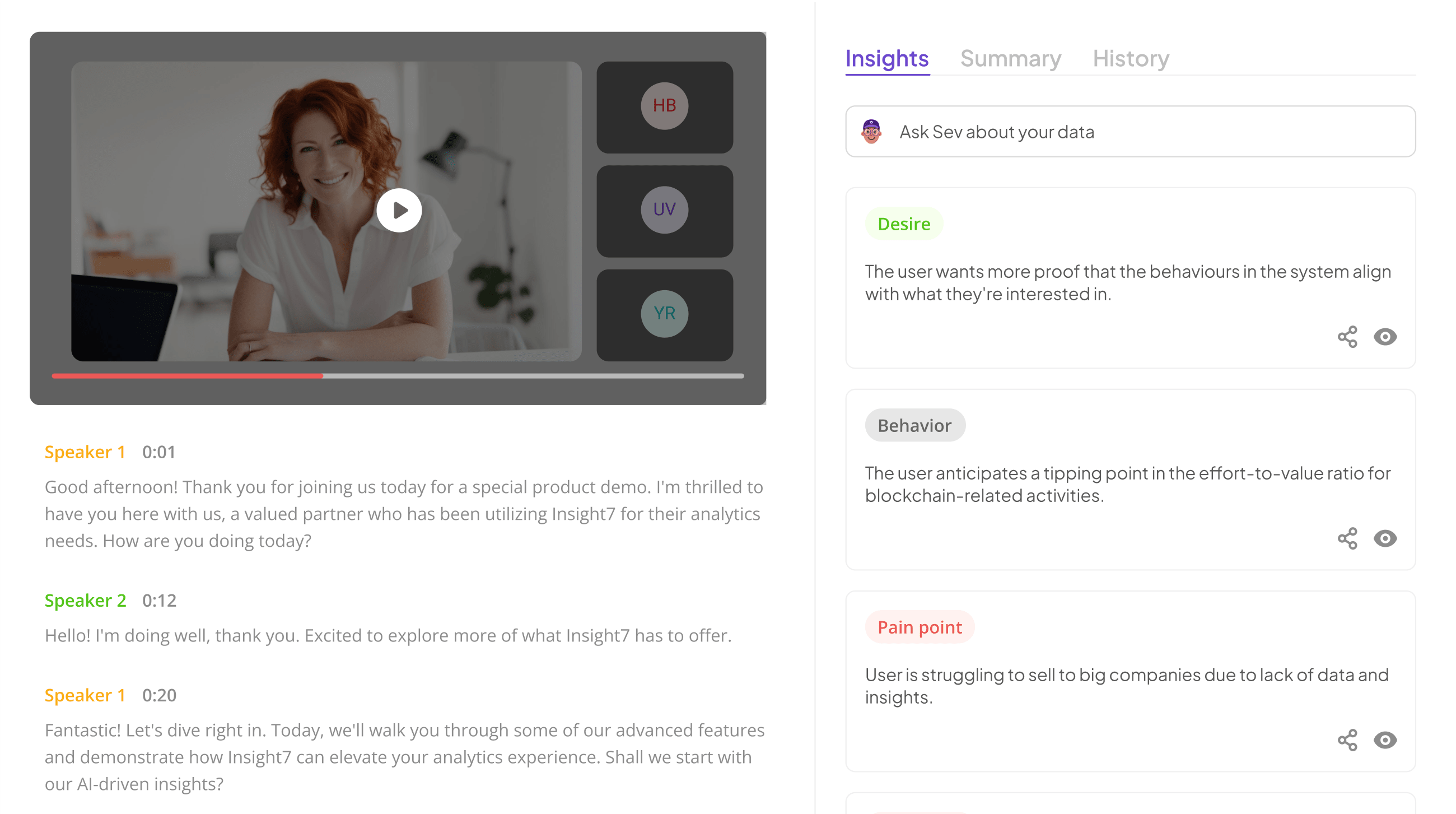