CX Resourcing Forecasting plays a crucial role in ensuring that customer experience (CX) teams are adequately prepared to meet fluctuating demands. In today's fast-paced environment, businesses must anticipate resource needs accurately to provide exceptional service while managing costs effectively. This section delves into the fundamentals of CX resourcing forecasting, emphasizing the importance of data-driven decision-making.
As teams strive to enhance their operational efficiency, predictive data modeling emerges as a powerful tool in this process. By analyzing historical data and customer interactions, organizations can gain insights into future needs. Understanding these patterns allows for better alignment of resources, ultimately leading to improved customer satisfaction and team performance. Embracing CX resourcing forecasting is essential for staying competitive and responsive to ever-changing customer expectations.
Analyze & Evaluate Calls. At Scale.
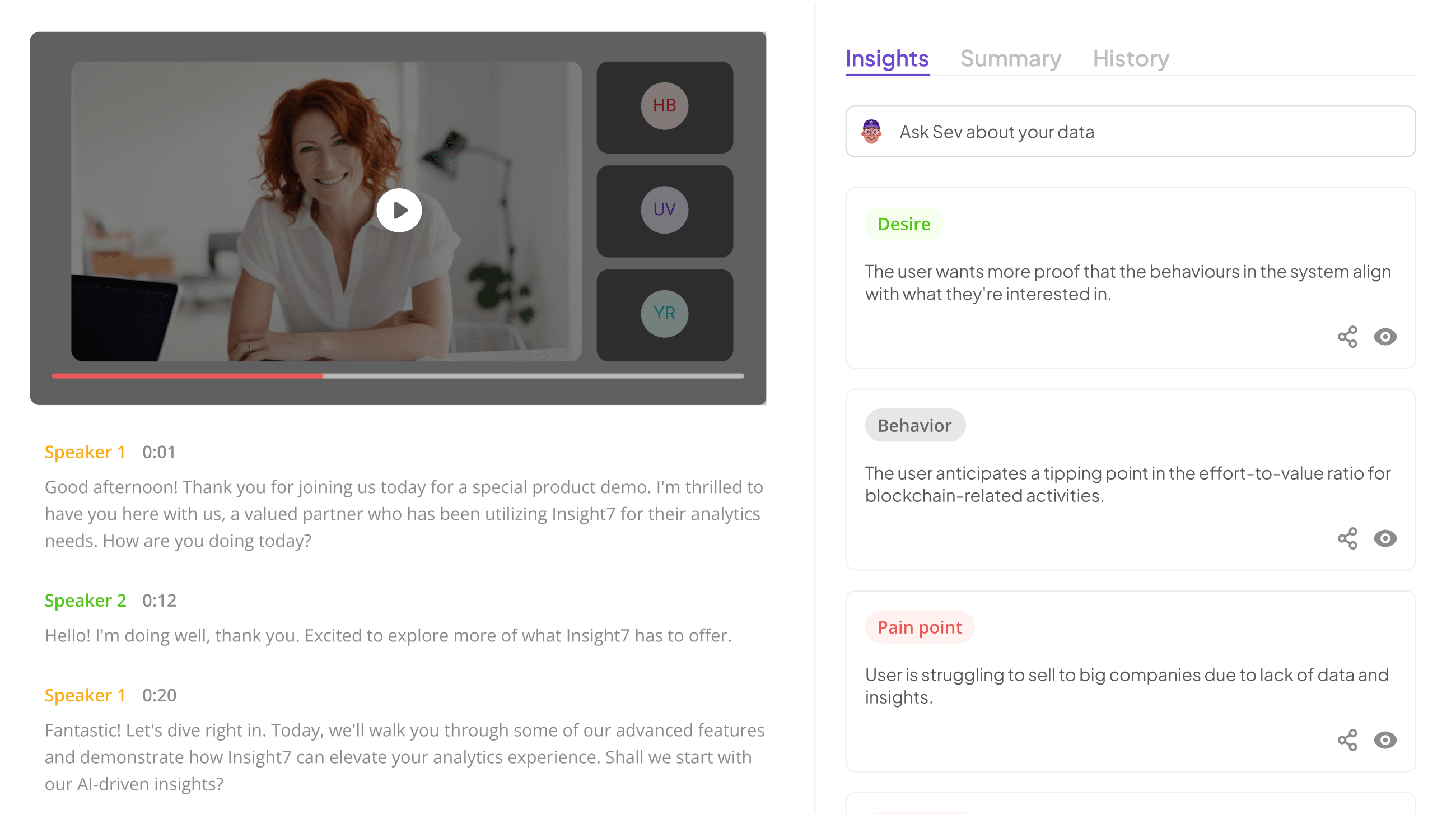
Understanding Predictive Data Modeling in CX Resourcing Forecasting
Predictive data modeling plays a crucial role in enhancing CX resourcing forecasting by analyzing historical trends and patterns. This approach utilizes advanced algorithms to predict future needs and optimize workforce planning. By interpreting data from customer interactions, organizations can foresee demand fluctuations, enabling them to allocate resources more effectively.
Understanding predictive data modeling involves grasping its fundamental components. First, data collection is essential; this involves gathering relevant historical data to identify key trends. Next, model selection and development are pivotal, as various statistical techniques help tailor predictions specific to the organizationโs needs. Finally, evaluating and refining the model ensures ongoing accuracy and relevancy of forecasts.
Implementing these steps allows organizations to make informed decisions, ultimately leading to enhanced customer satisfaction and operational efficiency. Predictive data modeling, when employed effectively, transforms CX resourcing forecasting from reactive to proactive, ensuring that customer needs are met efficiently.
Overview of Predictive Data Modeling
Predictive data modeling plays a crucial role in forecasting resource needs for customer experience (CX) teams. This analytical approach utilizes historical data and advanced algorithms to identify patterns and trends, enabling organizations to anticipate future demands. By employing predictive models, CX teams can strategically allocate resources, ensuring that they are prepared to meet customer expectations and enhance overall satisfaction.
To implement effective CX resourcing forecasting, organizations must focus on several key elements. First, data collection and preparation are vital, as raw data needs to be cleaned and organized for accurate analysis. Next, selecting the appropriate model is essential, as different algorithms may yield varying insights. Finally, ongoing evaluation and refinement of the model allow teams to adjust their forecasting methodologies to align with changing customer behaviors and market dynamics. Through this informed approach, predictive data modeling helps CX teams proactively manage their resources, ultimately leading to a more positive customer experience.
Benefits of Predictive Data Modeling for CX Resourcing
Predictive data modeling brings significant advantages to CX resourcing by enhancing decision-making and optimizing team allocation. Firstly, it allows organizations to anticipate customer needs based on past interactions, which can lead to a more effective training program for customer service representatives. By understanding the types of questions frequently asked, teams can align their training content to address these issues proactively. This ongoing refinement creates an agile CX team that can adapt quickly to changing customer queries and improves overall satisfaction.
Moreover, CX resourcing forecasting simplifies staffing needs by predicting peak times and required personnel levels. Organizations can analyze trends, enabling them to allocate resources efficiently and minimize costs associated with overstaffing or understaffing. This data-driven approach not only fosters better employee morale but also enhances customer experiences, ultimately leading to sustained business growth. By systematically applying predictive data modeling, companies position themselves as proactive service providers in a competitive marketplace.
Extract insights from interviews, calls, surveys and reviews for insights in minutes
Steps to Implement CX Resourcing Forecasting
To implement CX Resourcing Forecasting effectively, start with thorough data collection and preparation. Gather historical data related to customer interactions, call volumes, and team performance metrics. This data will create a robust foundation for your predictive models. Clean and organize the data to ensure its accuracy and relevance.
Next, focus on model selection and development. Choose the appropriate predictive models based on your specific business requirements and customer insights. Experiment with various algorithms to identify those that provide the most accurate forecasting results. Once models are developed, evaluate their performance using a clear set of criteria, such as accuracy, precision, and recall. Refine your models based on these evaluations to enhance their predictive capabilities continually. By following these steps, you can create a reliable system for forecasting CX team resourcing needs effectively.
Step 1: Data Collection and Preparation
The first step in effective CX Resourcing Forecasting is data collection and preparation. Start by identifying all relevant data sources, including customer feedback, interaction logs, and market trends. Gathering diverse data provides a more comprehensive view of customer behavior, which is essential for accurate forecasting. Ensure that the data collected is clean and organized to avoid any inconsistencies during analysis.
Once data is collected, the preparation phase involves processing and formatting the information for modeling. Itโs crucial to filter out any irrelevant or outdated data, as well as to standardize metrics across sources for cohesion. By investing time in thorough collection and preparation, you lay a robust foundation for subsequent forecasting steps. This initial work significantly enhances the accuracy and reliability of your CX resource planning efforts, enabling your team to anticipate needs effectively.
Step 2: Model Selection and Development
In the process of CX Resourcing Forecasting, the selection and development of the predictive model is crucial. The right model can turn your data into actionable insights, helping you anticipate your teamโs resourcing needs. Begin by evaluating different modeling techniques, such as linear regression, decision trees, or clustering, based on the specific requirements of your analysis. Each modeling method has strengths and weaknesses that can significantly affect the outcomes.
Next, youโll need to develop the chosen model using your prepared data. Ensure that you validate your model against known outcomes to refine its accuracy. Monitoring the model's performance in real-time is also essential, as variations in customer behavior can impact its effectiveness. A well-developed model will provide clear guidance on resource allocation, ultimately enhancing your customer experience strategy. By addressing these steps carefully, you can confidently navigate the complexities of CX resourcing.
Step 3: Evaluation and Refinement
In the Evaluation and Refinement stage, CX Resourcing Forecasting takes shape through systematic analysis. This critical phase involves assessing the predictive models you've developed to ensure they align with real-world scenarios. By comparing forecasts against actual team needs, organizations can identify trends and discrepancies, allowing for timely adjustments to their strategies. Such analysis empowers teams to refine their forecasts based on fresh data, ultimately enhancing accuracy and reliability.
Continually iterating on forecasts is crucial for optimization. This includes gathering feedback from stakeholders, examining performance metrics, and adjusting model parameters for better outcomes. The ongoing evaluation helps to pinpoint areas for improvement, ensuring that the CX team is effectively resourced. Ultimately, this step fosters a proactive approach to CX resourcing, enhancing responsiveness to evolving customer needs and preferences. Each iteration contributes to more precise forecasting, driving valuable insights for future planning efforts.
Top Tools for CX Resourcing Forecasting
When considering top tools for CX resourcing forecasting, it's essential to explore platforms that simplify predictive data modeling. The right tools can help organizations evaluate customer interactions and anticipate team needs effectively, leading to informed decision-making. Tools like insight7, IBM SPSS Modeler, and others excel in turning raw data into actionable insights, enabling businesses to foresee demand changes and allocate resources accordingly.
These tools typically offer features such as data visualization, trend analysis, and automated reporting functions, which streamline the forecasting process. By employing these technologies, teams can focus on improving customer experience rather than getting bogged down in data management. In todayโs fast-paced environment, ensuring your team is well-resourced requires staying ahead of customer expectations, making the choice of the right forecasting tool crucial for success.
insight7
In the realm of CX resourcing forecasting, understanding the importance of analytics becomes crucial for success. Companies today are inundated with customer data, necessitating effective strategies to filter and interpret these insights. This is where predictive data modeling comes into play. By utilizing these advanced modeling techniques, organizations can anticipate staffing needs based on historical trends and projected demands, ensuring they maintain efficient operations.
Moreover, fostering a data-driven culture within teams allows for better decision-making and resource management. As businesses aim to enhance customer interactions, integrating predictive models helps identify key areas for improvement. This proactive approach not only alleviates staffing shortages but also enhances overall customer experiences. Ultimately, companies equipped with predictive data capabilities are poised to thrive, consistently meeting and exceeding the needs of their customers.
IBM SPSS Modeler
IBM SPSS Modeler is a sophisticated tool designed to assist in predictive data modeling, particularly for tasks like CX resourcing forecasting. This platform provides users with a robust environment for data mining and analytics. By integrating statistical techniques and machine learning algorithms, it enables teams to analyze customer data effectively. This insight is crucial in planning resource needs within customer experience teams.
The user-friendly interface allows for visual data exploration and model building, making it accessible even for those without deep technical knowledge. Through its capabilities, organizations can enhance their decision-making processes, aligning team resourcing with anticipated customer demands. Effectively utilizing IBM SPSS Modeler ensures that CX teams are optimally staffed, ultimately driving improved service delivery and customer satisfaction. This approach not only streamlines operations but also prepares companies to respond proactively to shifts in customer needs.
SAS Advanced Analytics
SAS Advanced Analytics offers powerful capabilities for CX resourcing forecasting, enabling teams to gain deeper insights into operational needs. With its sophisticated data analysis tools, users can efficiently process large datasets and uncover hidden patterns. This analytical approach helps organizations predict staffing requirements with greater accuracy by examining various customer interactions and trends.
In the context of CX resourcing forecasting, SAS Advanced Analytics encompasses various functionalities, including predictive modeling, data visualization, and reporting. These tools allow teams to assess historical data, identify key performance indicators, and foresee shifts in customer demand. By utilizing these insights, organizations can make informed decisions on resource allocation, ensuring they effectively meet customer needs while optimizing team performance. In essence, integrating SAS Advanced Analytics into CX strategies transforms data into actionable forecasts that support better planning and operational efficiency.
RapidMiner
In the realm of CX Resourcing Forecasting, RapidMiner stands out as a powerful tool that simplifies predictive data modeling. This platform offers intuitive features for analyzing extensive datasets, enabling teams to derive actionable insights quickly. With its user-friendly interface, RapidMiner allows users to interactively explore data, making it an ideal choice for those looking to predict customer experience needs accurately.
Utilizing RapidMiner, organizations can automate data processing and apply various machine learning algorithms to assess patterns. These capabilities foster informed decision-making regarding resourcing needs. Furthermore, the tool supports visualizations that clarify trends over time, aiding in strategic planning for CX teams. With RapidMiner, businesses can efficiently allocate resources, enhancing overall operational effectiveness and customer satisfaction as they navigate ever-changing market demands.
Microsoft Azure Machine Learning
Microsoft Azure Machine Learning is a powerful platform that provides advanced tools for building predictive models tailored for CX Resourcing Forecasting. Its capabilities enable teams to analyze data through user-friendly interfaces and robust algorithms, making the forecasting process more intuitive and effective. By utilizing Azure, organizations can significantly enhance their understanding of customer behaviors, leading to informed decisions about resourcing needs.
The platform supports comprehensive data preparation and offers various machine learning tools that streamline model development. Users can quickly test different algorithms and refine their models based on real-time feedback. Moreover, Azureโs integration with other data sources allows for seamless collaboration, ensuring data accuracy and consistency across projects. By strategically employing Microsoft Azure Machine Learning, teams can harness predictive insights, optimize their CX strategies, and efficiently allocate resources to meet customer demands.
Conclusion: Leveraging Predictive Data Modeling for Optimal CX Resourcing Forecasting
Predictive data modeling plays a crucial role in enhancing CX resourcing forecasting. By employing sophisticated algorithms, organizations can analyze historical data to predict future customer interaction patterns. This predictive capability allows CX teams to allocate resources effectively, ensuring they can meet customer demands without overextending their workforce.
Moreover, accurate forecasting minimizes the risk of underperformance by identifying peak times and adjusting staffing levels accordingly. As a result, teams can achieve a balance that optimizes productivity while enhancing customer satisfaction. In this way, predictive data modeling becomes an essential tool for organizations striving to improve their customer experience through informed resourcing decisions.
Analyze & Evaluate Calls. At Scale.
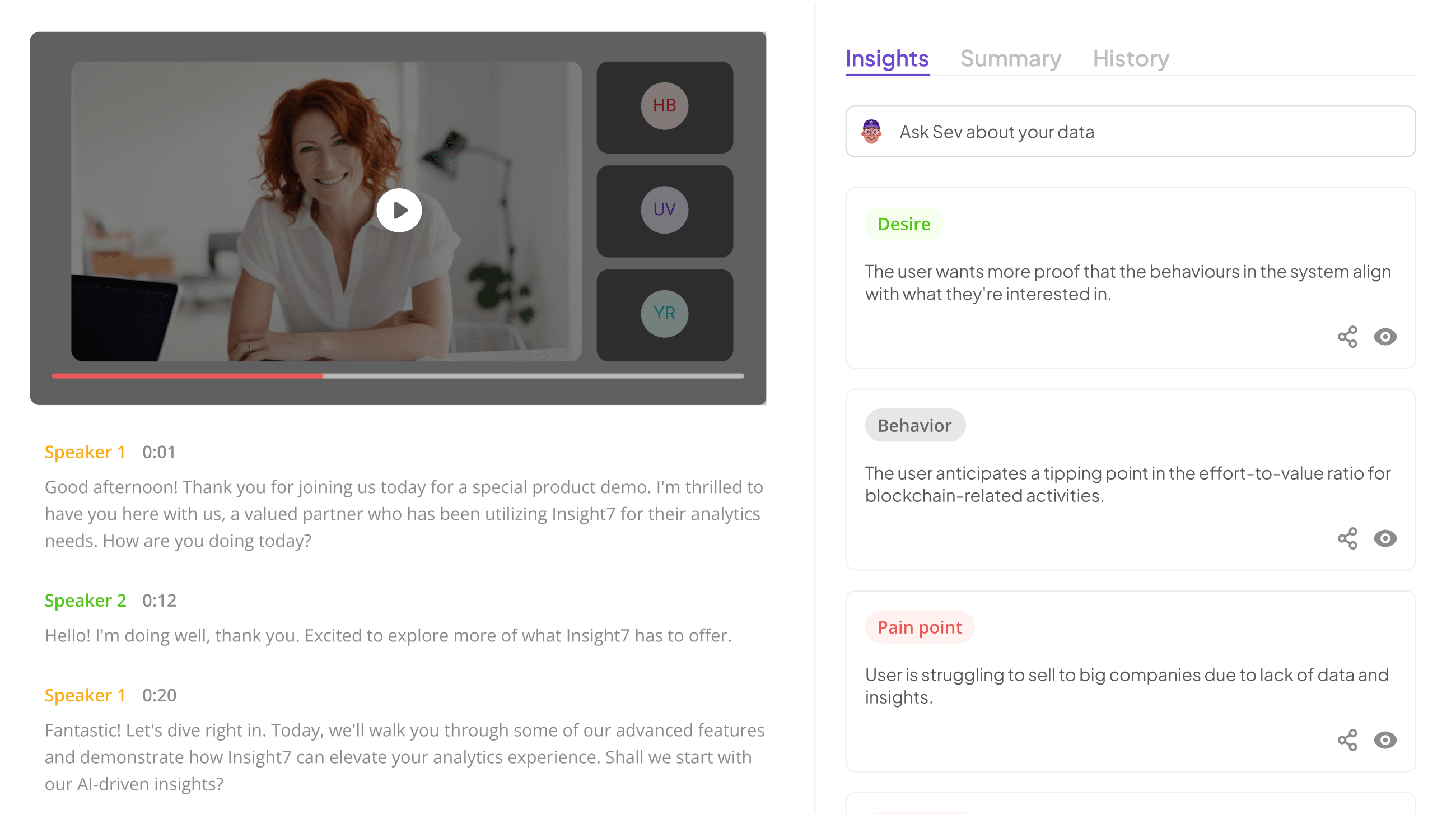