Qualitative Pattern Recognition in Machine Learning opens the door to rich insights hidden within complex data sets. As we navigate the world of qualitative data, understanding its nuances becomes essential. This field leverages machine learning techniques to transform unstructured data into actionable insights, enabling organizations to identify patterns that inform decision-making.
Recognizing qualitative patterns allows businesses to delve into customer feedback, social media sentiments, and other forms of textual data. By employing advanced algorithms, machine learning can categorize and analyze qualitative information, revealing critical trends and relationships. This section provides a foundational understanding of how machine learning enhances qualitative analysis, setting the stage for the exploration of specific techniques and tools.
Analyze & Evaluate Calls. At Scale.
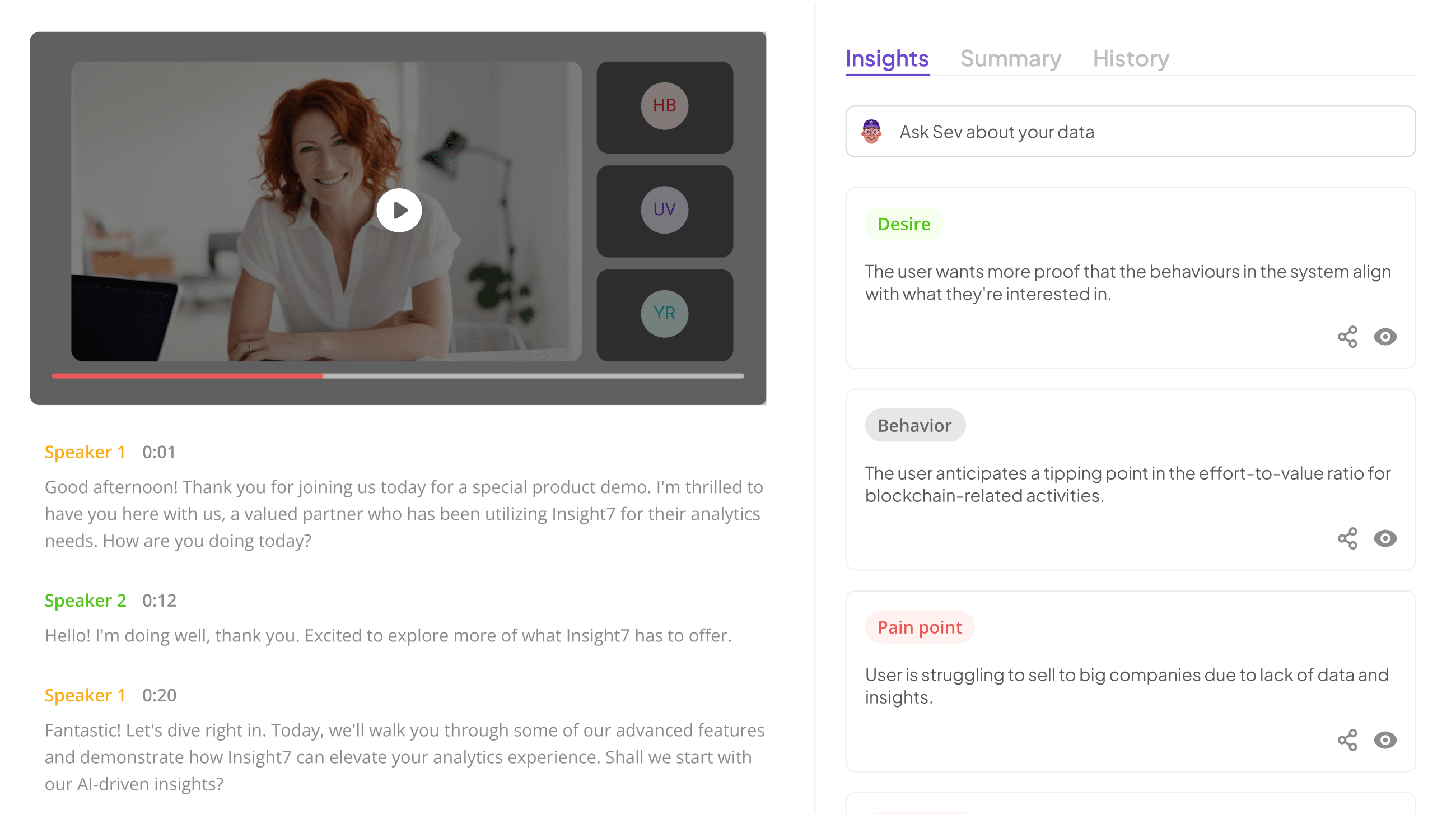
Understanding the Basics of Qualitative Pattern Recognition
Qualitative pattern recognition involves the systematic identification of non-numeric data patterns that convey meaning and context. Understanding the fundamentals of this concept is crucial as it lays the groundwork for detailed data analysis. This process primarily relies on techniques that extract themes and insights from qualitative data, such as interviews, transcripts, and open-ended survey responses.
Machine learning plays a vital role in enhancing qualitative pattern recognition by automating the analysis of large datasets and uncovering hidden insights. By applying algorithms to qualitatively rich data, machine learning simplifies deeper comprehension and interpretation. As a result, researchers can easily identify trends or behaviors that may not be immediately apparent, driving better decision-making and deeper insights across various fields. Candidates of machine learning, such as natural language processing, further amplify the effectiveness of qualitative pattern recognition.
Defining Qualitative Pattern Recognition
Qualitative Pattern Recognition involves identifying and interpreting complex patterns found within qualitative data, such as textual or verbal information. Unlike quantitative data, which relies on specific numbers and metrics, qualitative data focuses on understanding themes, concepts, and insights. This process is crucial in varied fields, including market research, customer feedback analysis, and social sciences.
Machine learning plays a pivotal role in enhancing qualitative pattern recognition. By utilizing algorithms, we can efficiently analyze vast amounts of unstructured data and uncover insights that might be overlooked through traditional methods. Machine learning tools can categorize the data, identify trends, and even generate predictive models based on past behavior. Ultimately, these advancements enable researchers and organizations to draw more profound and actionable conclusions from qualitative data, driving better decision-making across domains.
Importance of Machine Learning in Analyzing Qualitative Data
Machine learning plays a critical role in analyzing qualitative data, enabling researchers to uncover insights that may otherwise remain hidden. The application of algorithms to qualitative pattern recognition transforms raw data into structured information. This capability allows for efficient analysis of large text bodies, including interviews, surveys, and social media interactions. Without machine learning, the task of deciphering complex qualitative information can be time-consuming and prone to human bias.
In addition, machine learning algorithms can identify recurring themes and sentiments, enhancing our understanding of consumer behavior and preferences. By automating the coding process of qualitative data, researchers can focus on strategic decision-making rather than getting bogged down in manual analysis. Ultimately, machine learning not only streamlines qualitative pattern recognition but also promotes more reliable and actionable insights, significantly benefiting businesses and researchers alike.
Extract insights from interviews, calls, surveys and reviews for insights in minutes
Techniques and Tools for Qualitative Pattern Recognition
In the realm of qualitative pattern recognition, several techniques and tools offer effective solutions to harness data insights. Key techniques include thematic analysis, which identifies common themes across qualitative data, and sentiment analysis, which gauges emotional responses from textual sources. By employing these methods, practitioners can uncover patterns that inform decision-making processes and enhance user experiences.
Moreover, specific tools have emerged to facilitate qualitative pattern recognition. For instance, Insight7 provides a robust platform for analyzing feedback and extracting valuable insights efficiently. Other noteworthy tools include Orange, known for its user-friendly interface, and RapidMiner, which supports complex data processing tasks. Additionally, IBM Watson Analytics offers advanced analytical capabilities, while MonkeyLearn specializes in text analysis. Utilizing these tools can significantly streamline the qualitative research process and foster a deeper understanding of user sentiments and trends.
Key Techniques in Machine Learning for Pattern Recognition
In qualitative pattern recognition, certain key techniques in machine learning stand out for their effectiveness and adaptability. One significant approach is the use of supervised learning methods, which involve training models on labeled datasets. This allows the model to learn from examples and improve accuracy in recognizing patterns. Another essential technique is unsupervised learning, where algorithms identify patterns without prior labels, helping discover hidden structures in qualitative data.
Additionally, natural language processing (NLP) plays a crucial role in analyzing textual qualitative data, enabling machines to understand and interpret human language. Techniques like clustering and sentiment analysis provide valuable insights by grouping similar data points and gauging public sentiment. By utilizing these techniques, organizations can uncover actionable insights from qualitative data, thus improving their products and services. Combining these approaches leads to enhanced qualitative pattern recognition capabilities, ultimately benefiting decision-making and strategic planning.
Tools for Implementing Qualitative Pattern Recognition
For effectively implementing qualitative pattern recognition, familiarity with specific tools is crucial. Each of these tools offers unique capabilities that enhance the analysis of qualitative data. Some noteworthy options include advanced machine learning platforms like Orange and RapidMiner, which provide user-friendly interfaces for data visualization and analysis. These tools facilitate easier identification of patterns within qualitative data, allowing users to draw meaningful insights without requiring extensive programming knowledge.
Furthermore, platforms such as IBM Watson Analytics and MonkeyLearn enable automated sentiment analysis and text categorization. This feature simplifies the process of discerning themes and sentiments from the data you collect. By leveraging these tools, you empower your research efforts and can accurately interpret qualitative data, leading to informed decisions. The combination of these technologies can greatly enhance the efficiency and accuracy of qualitative pattern recognition endeavors.
- insight7
Machine learning has transformed how we interpret qualitative data. Insight7 serves as a self-service platform designed to facilitate qualitative pattern recognition by efficiently analyzing customer conversations. It empowers users to navigate overwhelming amounts of textual data from interviews and discussions, delivering insights in real-time. This efficiency is essential, as traditional methods often leave insights buried and unutilized, slowing down decision-making processes.
To maximize the benefits of qualitative pattern recognition, organizations must embrace a systematic approach. First, data collection should be streamlined, ensuring that all relevant customer interactions are captured. Next, implementing powerful tools like those provided by Insight7 allows teams to analyze this data at scale. Finally, effective collaboration among stakeholders is necessary to align insights with business strategies. By following these steps, companies can capitalize on valuable customer signals and maintain a competitive edge in their industries.
- Orange
Orange is a powerful tool designed to enhance qualitative pattern recognition through intuitive visualizations and user-friendly functionalities. The platform facilitates data analysis, offering various components such as data visualization, machine learning algorithms, and text mining, all while allowing users to interactively explore their datasets. By leveraging these capabilities, researchers and analysts can uncover hidden insights and trends more effectively.
One of the key strengths of Orange is its capability to handle both structured and unstructured data, supporting diverse qualitative research methods. Users can build workflows that encompass data preprocessing, feature selection, and model evaluation. This versatility encourages innovative approaches to qualitative pattern recognition, making it accessible for both novice and experienced users. Ultimately, Orange stands out as a crucial resource in the growing field of qualitative data analysis, assisting users in extracting valuable insights from their data efficiently.
- RapidMiner
RapidMiner stands out as a versatile tool in the realm of qualitative pattern recognition. By combining user-friendly interfaces with powerful processing capabilities, it allows researchers to analyze and derive insights from qualitative data efficiently. This makes it particularly appealing for those looking to identify patterns in customer feedback or interviews, offering a streamlined path to understanding complex datasets.
To harness its full potential, users can follow these key steps:
- Set Up a New Project: Initiate a project quickly by naming it and uploading relevant files, such as audio transcripts or survey results.
- Data Integration: Easily import data from various sources, including local storage and cloud services, to create a comprehensive dataset.
- Data Analysis: Utilize the matrix feature to formulate questions and extract insights that reveal trends from collected data systematically.
These steps empower users to gain clarity and actionable insights, enhancing their qualitative pattern recognition efforts significantly.
- IBM Watson Analytics
With a focus on qualitative pattern recognition, exploring advanced analytical tools can greatly enhance data interpretation. This technology enables users to sift through extensive datasets, revealing insights that may not be immediately apparent. By employing sophisticated algorithms, the platform enhances the userโs ability to locate trends in customer feedback, providing a nuanced understanding of sentiments expressed.
Utilizing this tool, users can effortlessly analyze positive and negative comments to inform product development and marketing strategies. For instance, cross-comparisons of data sets from different locations can uncover unique trends and insights. Visualizing this information through various formats helps stakeholders make data-driven decisions, significantly improving their strategic planning processes. Overall, leveraging this analytical capability supports organizations in deriving actionable insights from qualitative data, streamlining their approaches to understanding customer experiences better.
- MonkeyLearn
Integrating advanced tools into your qualitative data analysis can enhance the accuracy of insights. By utilizing specific platforms, you can automate the process of qualitative pattern recognition efficiently. A standout option in this realm offers a robust suite of features designed to analyze text data and extract meaningful patterns effortlessly. This tool allows users to upload their text datasets, offering customizable insights that cater to unique research needs.
For effective qualitative pattern recognition, itโs essential to understand key functionalities. Users can seamlessly create customized models tailored for their datasets, enabling more refined analysis. Additionally, the platform supports sentiment analysis, key phrase extraction, and topic classification, facilitating a deeper understanding of qualitative data trends. Engaging with such automated systems enables researchers to focus more on strategy and interpretation rather than manual data handling, ultimately improving the decision-making process in business contexts.
Conclusion: Advancing with Qualitative Pattern Recognition in Machine Learning
Advancements in qualitative pattern recognition have the potential to dramatically transform how we interpret and utilize qualitative data in machine learning. By enhancing our ability to understand complex datasets, we can uncover deeper insights that inform decision-making processes. This evolution not only improves the precision of our analyses, but it also broadens the scope of qualitative inquiries in various fields.
As we move forward, embracing innovative tools and methodologies will be essential. Integrating qualitative pattern recognition into machine learning workflows can lead to more informed products and services. This shift will empower practitioners to derive actionable insights, ultimately driving progress across industries and enhancing our understanding of consumer behaviors.