Predictive Risk Analysis is becoming indispensable for contact centers striving to enhance their decision-making processes. By assessing past interactions and identifying patterns, organizations can pinpoint potential risks and opportunities for improvement. This proactive approach shifts the focus from merely reacting to issues towards anticipating and mitigating them before they escalate.
Incorporating data science techniques into Predictive Risk Analysis empowers contact centers to deliver better customer experiences. By analyzing various data sources, businesses can better understand customer behaviors and preferences. Ultimately, this knowledge fosters a culture of continuous improvement, enabling teams to engage with clients in more meaningful ways and reinforce their strategic goals.
Analyze qualitative data. At Scale.
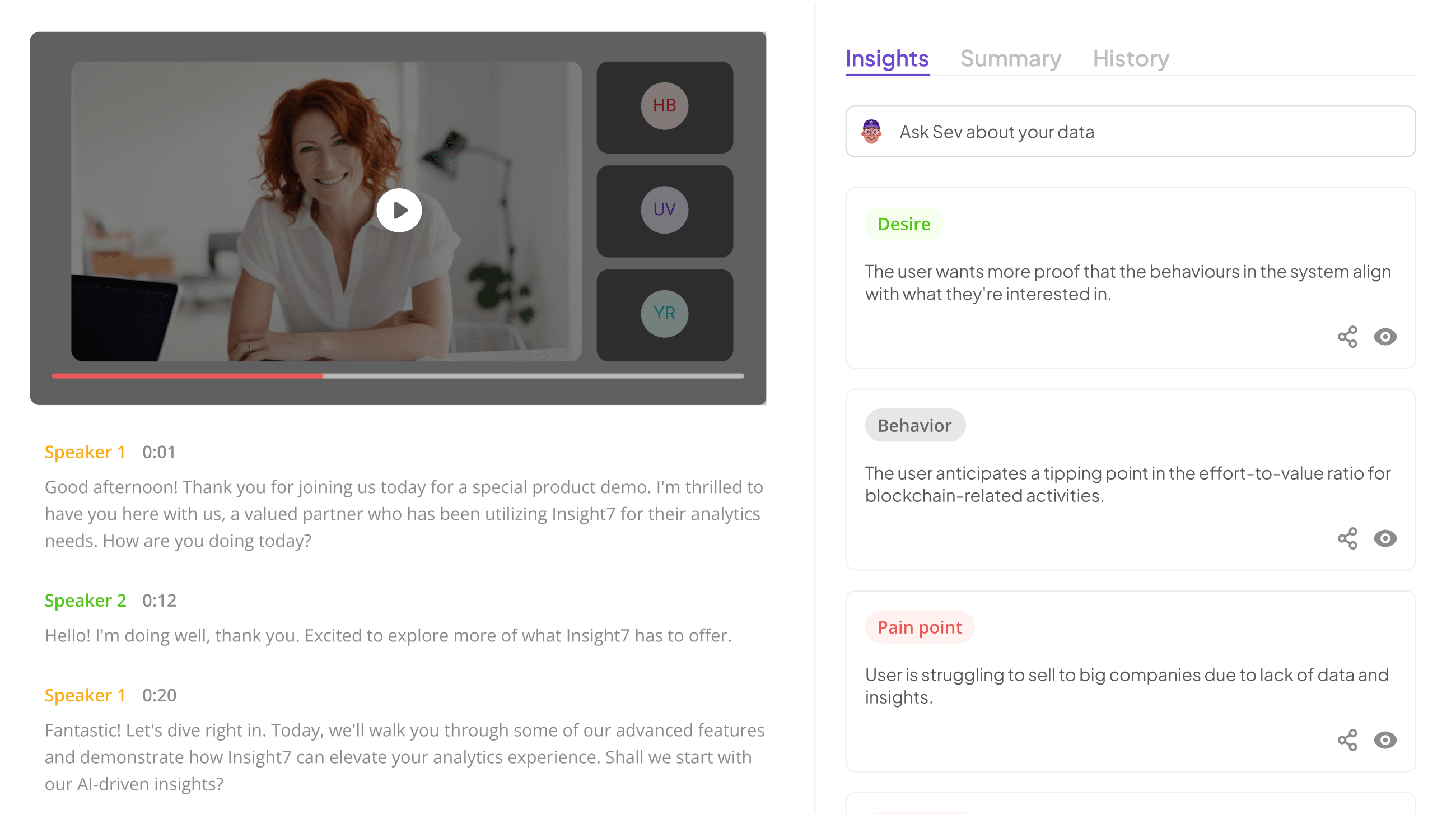
Understanding Predictive Risk Analysis for Better Decision Making
Predictive Risk Analysis is an essential approach for improving decision-making within contact centers. By interpreting data and understanding potential risks, organizations can preemptively address challenges, enhancing overall efficiency. This analysis utilizes historical data to forecast future events, allowing teams to anticipate customer needs and mitigate adverse outcomes before they occur.
To effectively implement Predictive Risk Analysis, it’s crucial to consider several components. First, data collection must be robust and varied, reflecting different aspects of customer interaction. Next, analysis techniques should be applied to identify patterns and correlations that signify risk. Lastly, actionable insights derived from these patterns guide informed strategic decisions. Embracing these components leads to more proactive management, fostering an environment where risks are managed effectively, and customer satisfaction is optimized.
Key Components of Predictive Risk Analysis
Predictive Risk Analysis relies on several integral components that facilitate effective risk identification in contact centers. Firstly, data collection is essential. It involves gathering comprehensive customer interaction data, which can highlight emerging trends and patterns. Secondly, data integration allows for the unification of diverse data sources, ensuring that analysis is comprehensive and accurate. This step often reveals underlying issues that may not be immediately apparent.
Next, the application of advanced analytical techniques, such as machine learning and natural language processing, enhances the predictive capability of risk analysis. These methodologies help in identifying potential risks based on historical data. Additionally, predictive models need continuous evaluation and refinement to adapt to new patterns and changing customer behaviors effectively. Lastly, visualization tools play a crucial role in presenting data insights, making it easier for decision-makers to translate findings into actionable strategies. Together, these components create a robust framework for implementing Predictive Risk Analysis in contact centers, ultimately improving service quality and customer satisfaction.
Benefits of Implementing Predictive Risk Analysis in Contact Centers
Implementing predictive risk analysis in contact centers offers significant advantages that can redefine operational efficiency. By harnessing data-driven insights, contact centers can proactively identify potential risks and refine their service strategies. Predictive risk analysis not only anticipates customer concerns but also enhances decision-making processes by providing data-backed recommendations.
One of the most compelling benefits is improved customer satisfaction. Understanding patterns in customer inquiries allows agents to address issues more effectively, reducing call resolution times. Additionally, this practice can help identify areas where staff training is essential, ensuring that customer service representatives are equipped to handle various scenarios. Furthermore, analyzing communication trends can lead to enhanced product offerings, aligning them with customer needs. Ultimately, the integration of predictive risk analysis fosters a proactive service environment, driving both operational excellence and customer loyalty.
Extract insights from interviews, calls, surveys and reviews for insights in minutes
Tools for Effective Predictive Risk Analysis in Contact Centers
Effective predictive risk analysis in contact centers relies on a variety of tools that facilitate data-driven decision-making. These tools analyze large sets of customer interaction data to identify potential risks and opportunities. By employing sophisticated algorithms, they help teams assess performance metrics, spot trends, and anticipate future challenges. This proactive approach enables contact centers to optimize operations and improve service quality, which is essential for maintaining customer satisfaction.
Among the tools available, IBM SPSS Modeler and RapidMiner stand out as powerful options. IBM SPSS Modeler offers advanced statistical techniques and predictive modeling capabilities, while RapidMiner combines ease of use with robust data integration features. Additionally, platforms like KNIME and SAS Predictive Analytics support seamless workflows for processing and analyzing data. As contact centers embrace these technologies, they can transform insights from customer interactions into actionable strategies, ultimately mitigating risks and enhancing overall performance.
insight7
Predictive Risk Analysis plays a crucial role in identifying potential threats within contact centers. By analyzing customer interactions and historical data, organizations can proactively mitigate risks before they escalate. This method allows contact centers to discern patterns indicative of customer dissatisfaction or fraudulent activities, enabling timely interventions.
Implementing Predictive Risk Analysis leads to several key advantages. First, it enhances decision-making by providing actionable insights derived from data analysis. Second, it streamlines communication among teams, improving collaboration around risk management. Third, companies can anticipate customer needs and prevent potential issues, thereby fostering a better customer experience. This proactive approach ensures risk factors are addressed swiftly, resulting in a more efficient operational environment. A well-integrated predictive analysis solution not only secures contact centers but also enhances overall service delivery.
IBM SPSS Modeler
IBM SPSS Modeler serves as a powerful tool in the realm of predictive risk analysis for contact centers. This software enables analysts to develop predictive models that not only identify risks but also prioritize areas requiring immediate attention. With its intuitive interface, users can rapidly integrate data from various sources, streamlining the process of drawing insights that are crucial for effective decision-making.
One of the standout features of IBM SPSS Modeler is its capability to handle complex datasets, transforming them into clear visualizations. These visual insights aid in recognizing patterns indicative of potential risks within the contact center environment. Furthermore, the tool facilitates the evaluation of different scenarios through simulation, allowing decision-makers to explore the impacts of various strategies. Utilizing such advanced analytic tools is essential for proactive risk management and ensuring operational efficiency in contact centers.
RapidMiner
RapidMiner is a powerful analytics tool widely used for predictive risk analysis, particularly in contact centers. By integrating data mining and machine learning capabilities, it allows organizations to uncover hidden patterns within customer interactions. These insights are essential for identifying potential risks and making informed decisions to enhance customer experience and operational efficiency.
One of RapidMiner's key features is its user-friendly interface, which enables teams to visualize data effectively. Users can quickly perform various queries to analyze customer feedback, both positive and negative. This function helps detect prevailing sentiments, allowing organizations to respond to issues proactively. Furthermore, RapidMiner's ability to process large datasets means that contact centers can continuously monitor trends over time, ensuring they remain agile in a competitive environment. This combination of capabilities positions RapidMiner as a vital tool for predictive risk analysis in the realm of customer service.
KNIME Analytics Platform
KNIME Analytics Platform is a versatile tool designed to facilitate effective data analysis in contact centers. It provides users with an intuitive interface, making it accessible to individuals without extensive technical training. With this platform, organizations can easily perform predictive risk analysis, enabling them to identify potential issues proactively.
The platform allows users to import and manage large datasets efficiently, such as call transcripts and customer interactions. Tools within KNIME enable teams to visualize data, extract essential insights, and analyze customer feedback systematically. By recognizing patterns and trends, contact centers can pinpoint areas of concern and address them swiftly. Additionally, the collaborative features of KNIME allow teams to work on multiple projects simultaneously, fostering a comprehensive understanding of customer needs and potential risks. This adaptability makes KNIME an essential asset in the ongoing effort to enhance customer experience and streamline operational efficiency.
SAS Predictive Analytics
SAS Predictive Analytics serves as a vital tool in the realm of predictive risk analysis within contact centers. This software harnesses advanced algorithms to analyze historical data and identify potential risks, enabling better decision-making. With features designed to model various scenarios, SAS provides the analytical rigor needed to assess customer interactions and trends through time.
Leveraging SAS Predictive Analytics allows contact centers to anticipate issues, optimize resources, and enhance customer satisfaction. By implementing these analytics, organizations can systematically uncover patterns that indicate risks, thus implementing proactive measures to mitigate them. In an environment where rapid decisions are crucial, the application of robust predictive analytics can significantly transform how risks are managed and, ultimately, improve overall operations.
Conclusion: Harnessing Predictive Risk Analysis in Contact Centers
As contact centers evolve, harnessing predictive risk analysis becomes essential for effective operations. This approach allows organizations to proactively identify potential issues, improving overall service quality. By analyzing historical data, contact centers can predict trends and customer behaviors, ultimately enhancing decision-making processes.
Implementing predictive risk analysis means prioritizing customer satisfaction while minimizing operational risks. With the right tools and methodologies, centers can fine-tune their training programs and adjust strategies based on real-time insights. This not only fosters a more engaged workforce but also ensures a better experience for customers, driving success in today's competitive landscape.