Outlier Detection Tools are essential for quality assurance teams striving to deliver exceptional results. As customer interactions become increasingly complex, the need to identify anomalies in call data instantly has never been more pressing. These tools enable QA teams to swiftly pinpoint outlier calls that may indicate underlying issues, leading to improved decision-making and enhanced customer satisfaction.
Understanding the nuances of outlier detection is crucial for QA teams. This process not only involves recognizing what constitutes an outlier but also understanding its potential impact on overall analysis. By employing effective outlier detection techniques, teams can streamline their workflow, ensuring that insights derived from customer interactions are not only timely but also actionable.
Analyze qualitative data. At Scale.
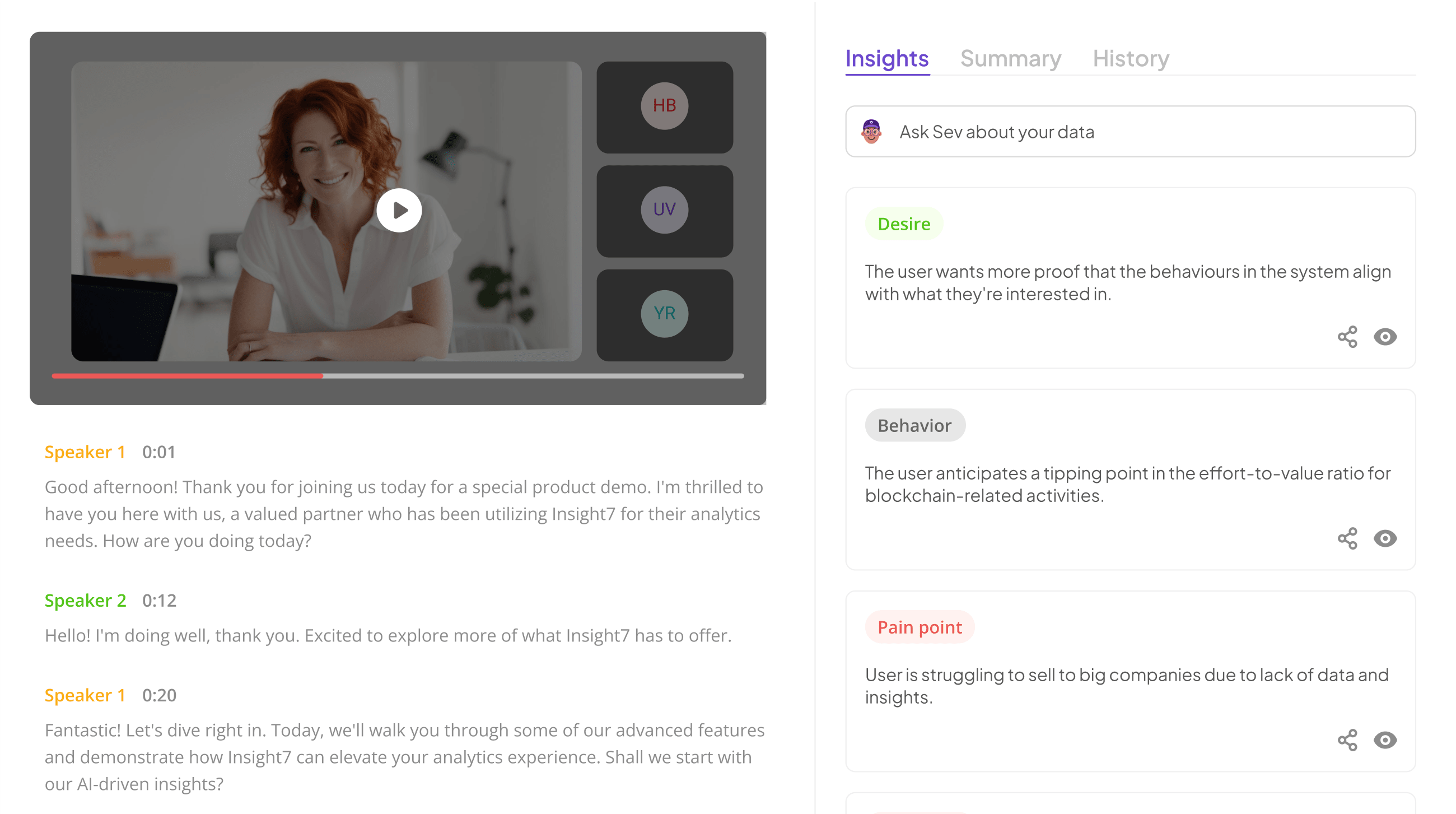
In the dynamic realm of quality assurance, the ability to surface outlier calls instantly can drastically enhance team efficiency and accuracy. This blog post explores critical Outlier Detection Tools that QA teams can leverage to pinpoint these anomalies swiftly, ensuring the quality and reliability of their processes.
In quality assurance, timely identification of outlier calls can significantly boost both team efficiency and accuracy. Outlier Detection Tools are essential in this dynamic environment, allowing QA teams to swiftly pinpoint anomalies that could disrupt the quality of service provided. By leveraging these tools, teams can ensure that they remain vigilant against irregularities that might compromise their processes.
The importance of surfacing outliers quickly cannot be understated. Without these tools, anomalies may go unnoticed, resulting in skewed interpretations of data and misguided decision-making. Effective outlier detection enhances collaboration among team members, enabling faster responses and reducing the time consumed in manual analysis. As we dive deeper into the types of tools available, it becomes clear that their role in improving the reliability of QA operations is invaluable.
When considering the options for Outlier Detection Tools, QA teams often focus on three critical categories: automated audio analysis tools, real-time call monitoring solutions, and advanced analytics platforms. Each of these tools plays a unique role in assisting QA teams to excel in their objectives. By implementing these tools effectively, teams can optimize their processes and deliver consistent, high-quality outcomes.
Understanding the Basics of Outlier Detection in QA
To effectively implement outlier detection tools, QA teams must first grasp the concept of outliers and their implications. An outlier refers to any data point that deviates significantly from the rest of the dataset, potentially indicating errors or anomalies in the quality assurance process. Identifying these outliers is crucial, as they can distort overall analysis and lead to misguided conclusions. By understanding what constitutes an outlier in specific contexts, QA teams can better tailor their approach in monitoring call quality.
Typically, outliers can arise from various sources, including unusual customer responses or procedural deviations. Their impact can extend to skewed data interpretations, affecting key decisions and strategies. Consequently, it is vital for QA teams to utilize dedicated outlier detection tools, which can allow for swift identification and resolution, thereby maintaining the integrity of the quality assurance processes. These tools serve as a pivotal resource in ensuring that all QA assessments are both accurate and reliable.
To effectively implement tools for outlier detection, its essential for QA teams to understand what constitutes an outlier in their specific context and how it can impact the overall analysis.
To effectively implement outlier detection tools, QA teams must deeply understand what constitutes an outlier in their unique context. Outliers are data points significantly different from the norm, which can skew results and lead to misinterpretations. Understanding this definition is crucial, as the impact of an outlier can extend far beyond just the immediate dataset. For instance, a single outlier might highlight a problem in product quality, customer service issues, or even flawed data collection processes.
Furthermore, the way outliers interact with the overall analysis deserves attention. Ignoring them can lead to poor decision-making, as the insights drawn may not reflect true performance. By training QA teams to recognize the implications of outliers, organizations can enhance the quality and reliability of their analyses. This understanding paves the way for using outlier detection tools more effectively, ensuring that teams can swiftly surface anomalies and take corrective actions before they escalate.
Common Types of Outliers in QA Processes
In quality assurance processes, outliers can significantly skew results and affect decision-making. The most common types of outliers include process deviations, coding errors, and anomalous behavior during evaluations. Process deviations often occur when agents fail to adhere to established protocols, leading to inconsistencies in service delivery. Identifying such instances requires diligent monitoring of call transcripts and performance metrics, where Outlier Detection Tools can prove invaluable.
Coding errors may arise from misassigned attributes or flagged issues that do not align with the customer experience. These discrepancies can lead to inaccurate assessments and misguided conclusions. Anomalous behavior during evaluations can stem from unexpected agent interactions or customer responses, which might indicate either exceptional service or areas needing improvement. Understanding these outliers is essential for maintaining accurate quality assurance, as they highlight areas requiring attention and interaction refinement. By effectively utilizing Outlier Detection Tools, QA teams can swiftly navigate these complexities, ensuring more reliable evaluations.
Identify various kinds of outliers that can appear during quality checks and the typical errors they might introduce.
In quality assurance, various types of outliers may emerge during quality checks, each posing unique risks. Firstly, temporal outliers can arise from unexpected spikes in call duration or frequency. Such anomalies might indicate abnormal customer behavior or volatile service disruptions, leading to misinterpretations of data trends. Secondly, categorical outliers can relate to irregularities in customer feedback or satisfaction ratings, often suggesting dissatisfaction that may not be representative of the overall service performance.
Moreover, data entry errors often introduce outliers, such as misclassified call types or incorrect tagging. These inaccuracies can skew analysis, resulting in misguided decisions about operational strategies or resource allocation. Lastly, systemic outliers could stem from broader organizational issues, such as an underperforming team or faulty processes leading to consistent issues in specific categories. Identifying and understanding these outliers is crucial for ensuring accurate quality assessments. Effective Outlier Detection Tools enable QA teams to filter these anomalies, ensuring data integrity and supporting informed decision-making.
Impact of Outliers on QA Outcomes
Outlier detection plays a vital role in quality assurance, particularly in interpreting call data effectively. When outliers go unnoticed, they can heavily skew results, leading to erroneous conclusions and misguided decisions. These anomalies often reflect underlying issues, from customer dissatisfaction to unexpected patterns in agent performance, which, if ignored, can create a cascade of problems for QA teams.
To mitigate these risks, implementing effective outlier detection tools becomes crucial. These specially designed technologies allow teams to swiftly identify and analyze outlier calls, providing a clearer view of overall performance. By understanding the impact of these outliers, QA professionals can make informed decisions that enhance both the quality of service and customer satisfaction. Through targeted analysis, teams can address their root causes, ensuring continuous improvement in QA outcomes and paving the way for sustained operational excellence.
Discuss how undetected outliers can lead to skewed data interpretations and affect decision-making.
Undetected outliers can severely distort data interpretations, leading to misguided decisions that could negatively impact an organization. When quality assurance teams overlook these anomalies, the insights drawn from their analyses may not reflect the true state of the data. This misrepresentation can result in misallocated resources, flawed strategy implementations, and even reputational damage if customer experience is compromised.
Outlier detection tools are essential in identifying these extreme values that deviate from expected patterns. By collapsing reliable data and creating an inaccurate narrative, undetected outliers can influence key business decisions, ultimately affecting performance outcomes. Therefore, implementing robust outlier detection tools is crucial not only for accurate data analysis but also for ensuring that decisions are grounded in reliable information. Investing in such tools empowers QA teams to uphold the integrity of their analyses and safeguard their decision-making processes.
[optional]Effective Tools for Outlier Detection in Calls
In quality assurance processes, effective tools for outlier detection in calls play a significant role in enhancing overall performance. Outlier detection tools allow QA teams to identify calls that deviate from established standards quickly. This identification can lead to timely interventions, thereby improving the quality of customer interactions. Tools like Insight7 streamline analytics, allowing teams to evaluate multiple dimensions of call data efficiently, thus promoting a proactive approach to quality assurance.
Moreover, tools utilizing automated audio analysis can recognize nuances in conversation patterns that may signal outlier calls. Real-time monitoring solutions further enable QA teams to detect issues as they arise, facilitating immediate corrective actions. Each of these tools empowers teams by providing critical insights, ultimately leading to improved training and performance assessments. By effectively leveraging these outlier detection tools, QA teams can optimize their evaluation processes and enhance customer service quality consistently.
Extract insights from interviews, calls, surveys and reviews for insights in minutes
Numerous tools are available to help QA teams streamline the process of identifying and addressing outlier calls instantly. Here, we provide a detailed overview of some top tools, starting with insight7.
In the ever-evolving landscape of quality assurance, the quest for efficiency often leads teams to explore innovative Outlier Detection Tools. These tools are designed to empower QA teams, enabling them to swiftly pinpoint outlier calls that could potentially skew data analysis. By leveraging advanced analytics and real-time monitoring capabilities, these tools facilitate the immediate identification and response to anomalies in call data.
Starting with Insight7, this platform delivers comprehensive analytics that enhance the QA process, allowing teams to sift through customer interactions seamlessly. Additionally, automated audio analysis tools represent another layer of sophistication, employing cutting-edge technology to discern anomalies in recorded calls. Furthermore, real-time call monitoring solutions play a crucial role by flagging deviations from standard call quality as they occur. Together, these tools not only streamline operations but also ensure that QA teams can maintain high levels of accuracy and responsiveness in their quality checks.
Insight7: Streamlining QA Processes
In today's competitive market, streamlining QA processes is essential for ensuring high-quality customer interactions. Effective outlier detection tools can automate the analysis of calls, allowing teams to quickly identify anomalies and mitigate potential issues. By adopting these tools, QA teams can enhance their workflow, making data analysis more efficient and timely.
One of the primary advantages of utilizing outlier detection tools is the reduction of manual effort. Traditional methods often involve sifting through extensive data sets, a process that can be tedious and prone to human error. By integrating automated solutions, teams can focus on interpreting actionable insights rather than getting bogged down by data collection. In turn, this increases collaboration and allows for faster decision-making, ultimately driving better customer experiences and improving overall quality assurance outcomes.
Explore how Insight7 offers advanced analytics to detect outlier calls, providing a comprehensive solution for QA teams.
In the ever-evolving field of quality assurance, the ability to detect outlier calls is crucial. Advanced analytics play a vital role in this process, empowering QA teams to identify deviations swiftly and accurately. Insight7 provides these teams with intuitive outlier detection tools that are user-friendly and capable of analyzing data at scale. This streamlined approach allows teams to focus on the signals within customer interactions, driving targeted improvements.
By harnessing sophisticated algorithms and real-time data processing, QA teams can spot unusual call patterns and trends. This not only enhances efficiency but also helps in translating insights into actionable strategies. Moreover, when insights are readily accessible and organized, collaboration becomes more effective. As a result, teams can quickly address any issues, ensuring higher quality outcomes in customer service interactions. Embracing these advanced analytics fosters a proactive culture, ultimately leading to better overall performance in quality assurance.
Steps to Implement Outlier Detection Tools Effectively
To maximize the benefits of Outlier Detection Tools, QA teams must follow specific implementation steps that guide them through integration and usage. First, it's essential to assess your QA needs. Understanding the specific challenges your team faces will help identify the most appropriate tools. For example, if your calls frequently show a variance in customer satisfaction, select a tool that analyzes call quality thoroughly.
Next, focus on integration and training. Once you've chosen a tool, ensure it's seamlessly integrated into your workflow. This might require collaboration with your IT department for technical setup. Additionally, training sessions for team members are crucial for maximizing the tool's functionality. Providing comprehensive training will empower your team to use the software confidently, leading to more effective outlier detection.
By following these steps, QA teams can leverage Outlier Detection Tools to enhance their processes significantly.
Tool #2: Automated Audio Analysis
Automated Audio Analysis tools provide a cutting-edge solution for Quality Assurance (QA) teams focused on surfacing outlier calls quickly. These innovative tools enable teams to efficiently transcribe audio recordings, turning spoken conversations into text that can be easily analyzed for anomalies. By utilizing automated audio analysis, QA teams can filter out normal call patterns and pinpoint outliers that may indicate potential quality issues or opportunities for improvement.
One of the key advantages of automated audio analysis is its ability to handle large volumes of calls simultaneously. Instead of manually reviewing recordings, teams can upload multiple audio files for bulk transcription and analysis. This streamlined process not only saves time but also enhances the accuracy of outlier detection. Once transcribed, teams can extract meaningful insights, such as customer pain points, trends, and other critical data, enabling informed decision-making and timely interventions in quality processes. Embracing automated audio analysis empowers QA teams to maintain high standards and continuously improve their service quality.
Examine another top-tier tool that utilizes automated audio analysis to identify anomalies within call data.
Automated audio analysis tools are revolutionizing the way QA teams detect anomalies within call data. These advanced systems leverage artificial intelligence to assess voice interactions, converting raw audio into insightful metrics. By automatically identifying factors such as tone, sentiment, and interruptions, these tools surface potential outlier calls swiftly, allowing teams to respond proactively.
Using these sophisticated Outlier Detection Tools enables QA teams to distill large volumes of call data into manageable insights. Features such as bulk transcription and detailed analytics help ensure that no crucial information slips through the cracks. Moreover, the ability to highlight specific insights and pain points with just a few clicks makes data-driven decision-making easier than ever. With seamless integration and user-friendly interfaces, these automated solutions empower teams to enhance the quality of customer interactions consistently.
Tool #3: Real-Time Call Monitoring Solutions
Real-time call monitoring solutions serve as invaluable assets for QA teams striving to enhance operational efficiency. These tools are designed to identify outlier calls as they occur, thereby enabling teams to respond quickly to any deviations from established quality standards. By analyzing both spoken interactions and key performance indicators in real time, QA teams can instantly recognize calls that warrant further examination.
Typically, real-time monitoring includes features like live scoring and automated feedback, which provide insights into representative customer service agent performance. This approach not only accelerates the detection of problematic interactions but also fosters an environment of continuous improvement. With the implementation of such outlier detection tools, organizations can adapt their training and operational strategies more effectively, ensuring alignment with customer expectations and fostering a culture of excellence in service delivery.
Discover real-time call monitoring systems that assist in the immediate detection of deviations in standard call quality.
Real-time call monitoring systems are essential for Quality Assurance (QA) teams seeking to maintain high standards of call quality. By leveraging advanced technology, these systems facilitate the immediate identification of deviations from expected call performance. This proactive approach empowers QA teams to address issues as they arise, ensuring that customer interactions meet established benchmarks.
Outlier Detection Tools play a vital role in this context. They analyze calls in real time, assessing various metrics like call duration, tone, and specific keywords. When anomalies are detected, alerts are generated, allowing QA teams to take swift action. For instance, if a customer service representative consistently scores lower than their peers, the system flags these interactions for further review. This immediate feedback loop helps identify training needs and areas for process improvement, ultimately leading to a more efficient and effective customer service operation.
By employing these monitoring systems, QA teams can ensure that they do not miss critical insights that can elevate their performance standards and enhance the customer experience significantly.
Steps to Implement Outlier Detection Tools Effectively
To implement Outlier Detection Tools effectively, QA teams should initiate the process with a thorough assessment of their specific needs. This involves identifying the types of anomalies they typically encounter and understanding the context in which these outliers appear. Different tools may cater to varied requirements, so discernment in selecting the right one is crucial.
Next, integration is key to harnessing the full potential of these tools. Teams should establish a clear integration plan, ensuring compatibility with existing systems. Comprehensive training programs are essential to ensure every team member understands how to use the tools effectively and can navigate any challenges. Ultimately, this methodical approach leads to the successful adoption of Outlier Detection Tools, resulting in quicker identification and resolution of anomalies, thereby enhancing overall quality assurance outcomes.
To maximize the benefits of these tools, QA teams must follow specific implementation steps that guide them through integration and usage.
To maximize the benefits of outlier detection tools, QA teams must carefully navigate a series of implementation steps that ensure effective integration and usage. First, teams should assess their specific quality assurance needs to determine the most suitable tools for their goals. Understanding how these tools will fit into existing QA processes helps in selecting the right ones for effective outlier identification.
Next, integrating the chosen tools requires attention to detail and proper training for the team members. During this phase, QA teams should not only familiarize themselves with the functionalities of the tools but also establish workflows that make reporting and analysis straightforward. Finally, consistent usage and feedback loops should be emphasized to refine their practices and gain maximum insights from the outlier detection tools. By following these strategic steps, QA teams can enhance their capabilities to surface outlier calls instantly and improve overall quality outcomes.
Step 1: Assessment of QA Needs
Before implementing Outlier Detection Tools, it's essential to assess the specific needs of the QA team. This first step lays the foundation for selecting tools that best align with quality assurance objectives. Identifying the types of calls that may contain outliers and understanding what constitutes a successful interaction is critical. Creating a detailed set of criteria tailored to your operational context will guide the assessment.
Additionally, documenting expected outcomes can aid in establishing clear benchmarks. Engage team members in discussions to highlight their concerns and priorities regarding call quality. This collaborative effort ensures that the selected outlier detection tools address actual needs instead of hypothetical scenarios. By taking a structured approach to assessment, teams can maximize the efficacy of the chosen tools, leading to improved compliance and overall QA performance.
Outline the initial step of assessing the teams specific needs and choosing the appropriate outlier detection tool accordingly.
Understanding the specific needs of the QA team is the foundation for selecting the right outlier detection tools. Start by engaging team members to gather insights on their unique challenges. This discussion should include current pain points, the types of anomalies they encounter, and their desired outcomes in addressing these issues. By clarifying these requirements, teams can ensure that the selected tools align with their operational goals.
Next, evaluate the available outlier detection tools against the identified requirements. Consider key features such as ease of integration, analysis capabilities, and user-friendliness. It is also beneficial to assess potential scalability to accommodate future needs. Gathering feedback from team members about their preferences can further inform the decision-making process. This tailored approach ensures that the chosen solution effectively meets the team's specific requirements and enhances overall call quality assessment.
Step 2: Integration and Training
To effectively integrate Outlier Detection Tools into your quality assurance processes, a systematic approach is essential. The first step is ensuring that these tools align with your teamโs specific needs. This involves collaborating with stakeholders to assess the current QA environment thoroughly and identifying the key metrics for monitoring. Once the right tool is chosen, the next phase focuses on the integration process, which may require technical adjustments to ensure compatibility with existing systems.
Training is crucial for the successful adoption of these tools. Once integrated, providing comprehensive training sessions will help team members understand the toolโs functionalities and best practices. This can include hands-on demonstrations, alongside creating easy-to-follow user guides that outline common processes and troubleshooting steps. By fostering a learning environment and encouraging regular feedback, QA teams can enhance their ability to surface outlier calls instantly and maintain high-quality standards.
Guide QA teams through the integration process and the necessary training to ensure seamless tool adoption.
Integrating Outlier Detection Tools into QA processes requires a structured approach to ensure successful implementation and usage. First, teams must assess their specific needs to select the right tools tailored to their operational context. Once a suitable tool is identified, the integration process begins, inclusive of technical setup and alignment with existing workflows. Itโs vital at this stage to establish clear objectives about what the tool is expected to achieve in terms of outlier detection capabilities.
Training is a crucial aspect of this integration process. QA teams should engage in comprehensive training sessions to familiarize themselves with the toolโs features, functionalities, and best practices. This may include hands-on workshops, instructional webinars, or interactive tutorials. Regular feedback should also be collected post-training to refine skill sets and address any remaining challenges. By taking these steps, QA teams can achieve seamless adoption of Outlier Detection Tools and enhance the overall effectiveness of their quality assurance efforts.
Conclusion: Enhancing QA Outcomes with Outlier Detection Tools
The strategic application of Outlier Detection Tools empowers QA teams to elevate their operational efficiency and accuracy. By swiftly identifying outlier calls, teams gain immediate insights into potential issues, facilitating timely interventions. This proactive approach helps in maintaining high-quality standards, ultimately enhancing the overall customer experience.
Moreover, embracing these tools allows for a more systematic and data-driven analysis. With a focus on actionable insights, QA teams can transform their processes, making informed decisions that optimize quality outcomes. In a competitive landscape, leveraging Outlier Detection Tools not only streamlines workflows but also ensures teams stay ahead of challenges.
In conclusion, the strategic application of Outlier Detection Tools is paramount for QA teams aiming to enhance efficiency and accuracy in their processes. By identifying outlier calls instantly, these tools provide actionable insights that drive improved quality outcomes.
The strategic application of Outlier Detection Tools is crucial for QA teams seeking to elevate their performance. These tools enable swift identification of outlier calls, allowing QA professionals to focus on significant deviations that might compromise quality. By utilizing these insights, teams can enhance their processes, ensuring that potential issues are caught early, thereby preventing costly errors.
Furthermore, the continuous monitoring capabilities of these tools offer invaluable data that support ongoing training and development. This empowers teams to cultivate a culture of compliance and excellence, ultimately leading to improved outcomes. In today's fast-paced environment, integrating effective outlier detection is not just beneficialโit is essential for sustained success.