AI-Driven Qualitative Analysis offers a transformative approach to understanding complex qualitative data. Traditional qualitative analysis often relies on time-consuming manual processes that may overlook subtle insights. By integrating AI into this realm, researchers can quickly and accurately transcribe conversations, uncover themes, and analyze emotional tones. This not only enhances efficiency but also enriches the depth of insights garnered from data.
In this evolving landscape, AI tools facilitate the identification of patterns and sentiments, enabling more nuanced interpretations. The application of AI allows researchers to explore vast datasets, revealing unique opinions and trends that might otherwise remain hidden. As a result, AI-driven methods are reshaping qualitative analysis, making it more systematic, scalable, and impactful.
Analyze qualitative data. At Scale.
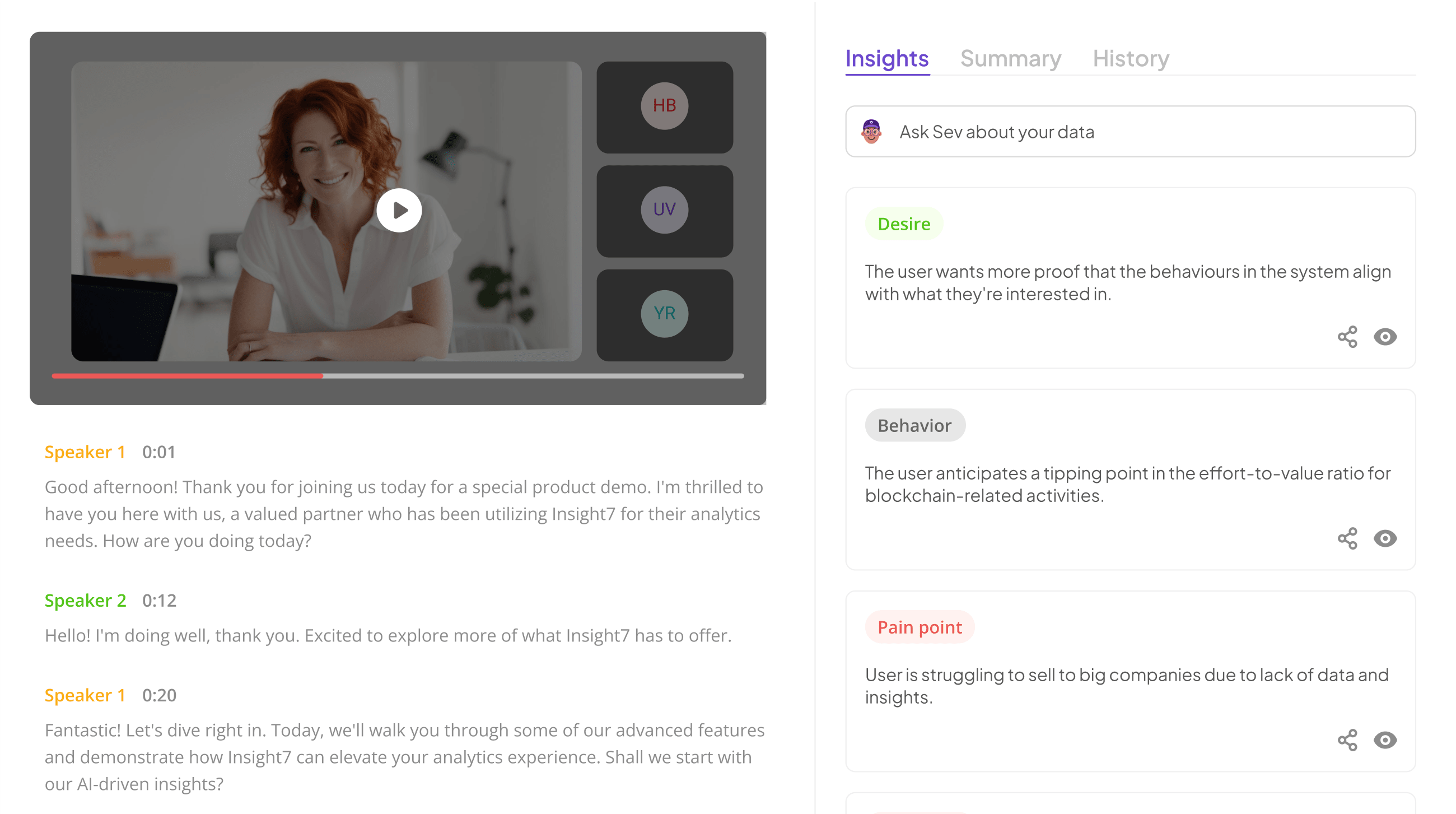
Transcription: Transforming Speech to Text with AI-Driven Qualitative Analysis
Transcription processes have historically been labor-intensive, often requiring skilled individuals to convert spoken language into written text. Today, AI-driven tools are revolutionizing this practice by seamlessly transforming speech into text, enabling researchers to analyze qualitative data with unprecedented speed and efficiency. This transformation is critical during discussions where insight extraction is vital, and AI simplifies this by managing multiple audio files at once.
AI-driven qualitative analysis enhances the ability to sift through large volumes of data. Transcription assists in organizing these insights, allowing researchers to visualize responses and identify common themes more easily. By implementing algorithms that summarize conversations or extract specific insights, researchers can focus on interpreting data rather than spending excessive time on the manual transcription process. Thus, the role of AI in transcription not only expedites data management but also enriches qualitative research outcomes, paving the way for deeper analysis and understanding.
How AI Transcription Tools Work
AI transcription tools convert spoken language into text, enabling significant advancements in qualitative research. The process begins with audio input, where the software uses automatic speech recognition algorithms to differentiate voices, words, and phrases. It analyzes sound waves and transcribes them into written text, effectively creating a digital document from natural conversations.
Once transcription is complete, the real power lies in analysis. Researchers can easily sift through the generated text to extract meaningful insights. Tools often offer features like keyword extraction, sentiment analysis, and summarization, streamlining the process of AI-driven qualitative analysis. These functionalities allow researchers to identify themes, patterns, and notable quotes, transforming raw data into actionable insights. In summary, AI transcription tools not only accelerate the transcription process but also enhance the overall analysis of qualitative data, fostering a deeper understanding of complex information.
Benefits of AI-Driven Transcription in Qualitative Research
AI-driven transcription is revolutionizing qualitative research by enhancing efficiency and accuracy. This technology streamlines the process of converting spoken dialogue into text, allowing researchers to compile large volumes of data quickly. As a result, professionals can focus on analysis rather than spending countless hours transcribing recordings manually.
Additionally, AI-driven transcription services also improve the quality of qualitative data. The tools often include features like speaker differentiation and context capture, which enhance the transcripts' reliability. This leads to more insightful analyses, ultimately enriching the quality of findings. By offering detailed and easily accessible outputs, researchers can identify themes, sentiments, and patterns more effectively, paving the way for deeper understanding in qualitative studies. Embracing AI in transcription is not just about technological advancement; it is about unlocking new dimensions of insights in qualitative research.
Extract insights from interviews, calls, surveys and reviews for insights in minutes
AI-Driven Qualitative Analysis in Data Interpretation
AI-Driven Qualitative Analysis in Data Interpretation enables researchers to extract deeper insights from qualitative data. By harnessing machine learning algorithms and natural language processing, data interpreters can uncover patterns that human analysis might overlook. This integration enhances the ability to analyze vast datasets, delivering timely and accurate results to inform decision-making processes.
This approach focuses on two primary elements: pattern recognition and sentiment analysis. Firstly, pattern recognition allows researchers to identify recurring themes or trends within large volumes of interview transcripts, open-ended survey responses, or social media comments. Secondly, sentiment analysis evaluates emotional tones, categorizing sentiments as positive, negative, or neutral. Together, these elements provide a comprehensive understanding of the data, allowing researchers to act strategically based on nuanced insights. As AI technologies continue to evolve, the potential for AI-Driven Qualitative Analysis in Data Interpretation becomes increasingly vital for informed decision-making in various fields.
Leveraging AI for Pattern Recognition
AI-Driven Qualitative Analysis enables researchers to discover patterns within extensive qualitative data efficiently. By employing advanced algorithms, AI systems can identify recurring themes and trends that might go unnoticed through manual analysis. This capability not only saves time but also enhances the accuracy of insights drawn from qualitative data. Researchers benefit significantly from this technology, allowing them to focus more on strategic thinking rather than getting bogged down in tedious data processing.
One primary advantage of using AI for pattern recognition is its ability to analyze large datasets in real-time. This feature allows for rapid comparisons across different data subsets, such as assessing feedback from various locations or demographic segments. Additionally, AI tools can generate visualizations that make complex data more digestible. As researchers increasingly rely on AI to facilitate qualitative analysis, they unlock deeper insights, fostering more informed decision-making and innovative solutions in their fields.
Sentiment Analysis: Understanding Emotional Tones
Sentiment analysis serves as a vital tool for interpreting emotional tones embedded in qualitative data. By leveraging AI-driven qualitative analysis, researchers can identify and categorize emotional responses from vast amounts of text. This process helps to illuminate the underlying sentiments expressed by respondents, revealing both positive and negative reactions. Understanding these emotional tones is essential for organizations to make informed decisions based on customer feedback.
AI-driven sentiment analysis employs algorithms to analyze linguistic elements, such as word choice and sentence structure. This not only streamlines the analysis process but also enhances accuracy. By detecting subtle shifts in language, the technology provides insight into consumer attitudes, facilitating targeted improvements in products and services. As organizations increasingly recognize the importance of emotional intelligence in data analysis, sentiment analysis will play a crucial role in shaping strategies and enhancing customer experiences.
Conclusion: The Future of AI-Driven Qualitative Analysis
As we look ahead, the future of AI-Driven Qualitative Analysis promises to revolutionize how researchers interact with qualitative data. This technology will not only streamline transcription processes but also enhance the ability to derive actionable insights from vast datasets. By utilizing advanced algorithms, researchers can quickly identify patterns, themes, and sentiments, enabling them to make informed decisions faster than ever before.
Moreover, as AI tools become more sophisticated, they will continue to reduce human error and bias in qualitative analysis. This evolution will make qualitative research more accessible, allowing diverse voices and perspectives to be included in the analysis. Overall, embracing AI-Driven Qualitative Analysis will empower researchers to uncover deeper insights, shaping the future of research methodologies.