Data-Enhanced Research represents a transformative approach that melds the rigor of traditional research with the innovative capabilities of data science. As researchers delve deeper into complex problems, the intersection of these two fields offers novel insights that were previously unattainable. Data-Enhanced Research allows for a more comprehensive exploration of subjects, providing both qualitative richness and quantitative accuracy.
Employing techniques such as predictive analytics and data visualization can significantly enhance the effectiveness of conventional methods. By integrating data science into surveys and interviews, researchers can uncover patterns that illuminate underlying trends and behaviors. This synergy not only elevates the quality of findings but also enriches the overall research experience, making it more relevant and applicable in a rapidly-evolving world.
Analyze qualitative data. At Scale.
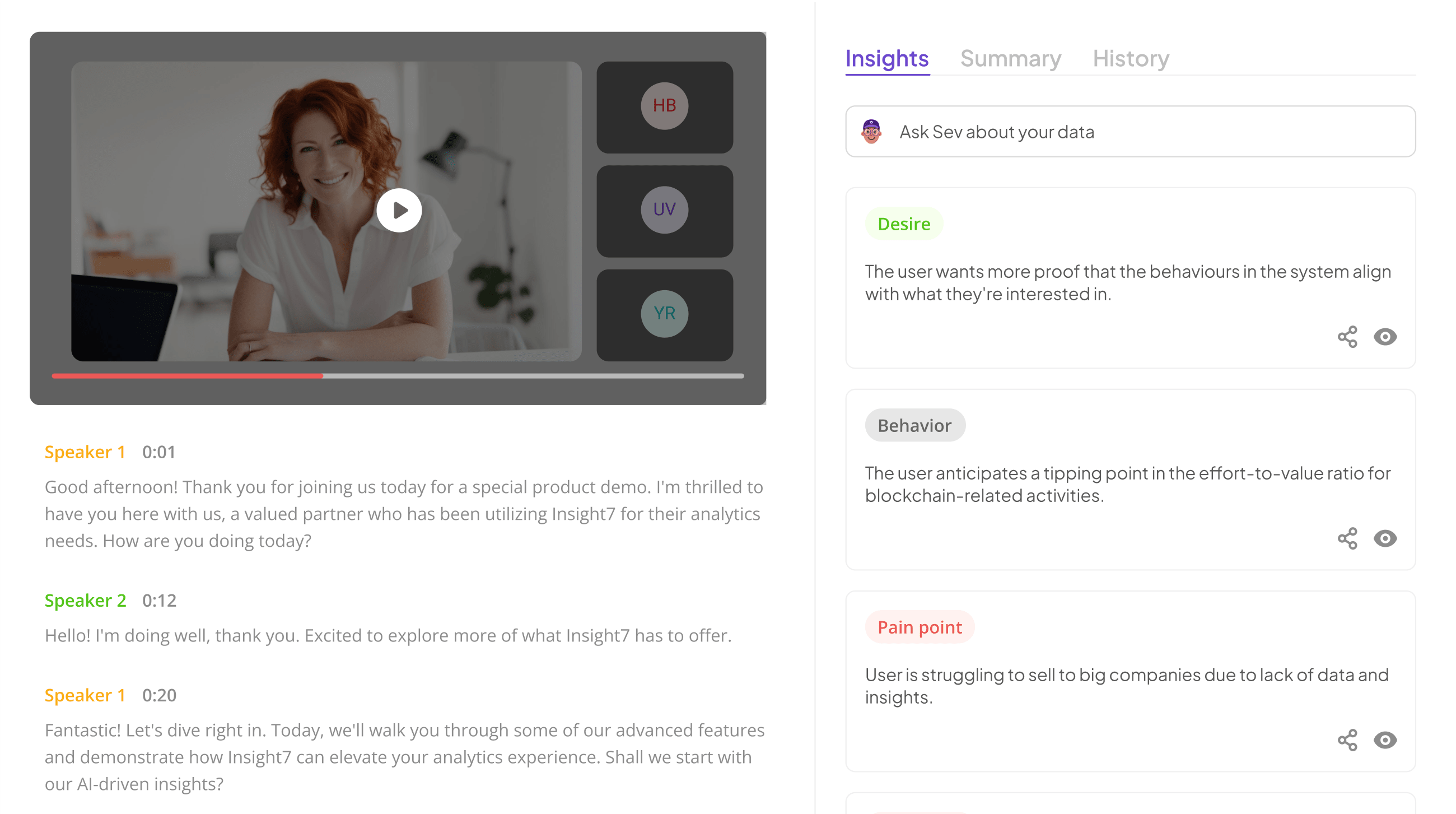
The Role of Data-Enhanced Research in Modern Studies
Data-enhanced research has transformed modern studies by integrating the strengths of data science with traditional research methods. This approach allows researchers to gather rich insights by combining qualitative data from interviews or surveys with quantitative data from analytics. The result is a more comprehensive understanding of research questions, enabling scholars to make informed decisions based on multiple perspectives.
Moreover, data-enhanced research enhances the reliability of traditional techniques. When researchers employ data analytics to interpret the findings from field studies, they can identify trends and patterns that may not be immediately apparent. This synergy between data-driven insights and traditional methodologies fosters a more robust research framework that can adapt to the demands of an ever-evolving academic landscape. By leveraging advanced technologies and analytical techniques, researchers can not only streamline their processes but also elevate the quality of their findings.
Integrating Data Science Techniques
Integrating data science techniques within traditional research methods significantly enhances the research process. Combining qualitative insights with quantitative data allows researchers to develop a more comprehensive understanding of their subject matter. Using advanced analytics, researchers can draw connections that may have otherwise gone unnoticed, ensuring that their findings are robust and actionable.
One effective technique is applying machine learning models to identify patterns within large datasets. This predictive analysis offers valuable forecasts that can guide research directions and decision-making. Moreover, integrating data visualization tools helps communicate complex findings clearly, making insights accessible to both researchers and stakeholders. Ultimately, these methods transform traditional research, providing it with a data-enhanced backbone that strengthens conclusions and boosts overall credibility. By leveraging these innovative approaches, researchers can navigate the complexities of modern inquiries with more precision and confidence.
- Combining qualitative and quantitative approaches
Combining qualitative and quantitative approaches in research provides a holistic view of complex issues. Data-enhanced research marries detailed narratives with numerical data, creating a comprehensive understanding. Qualitative methods offer insights into human experiences, while quantitative analysis provides measurable evidence to support these findings. This blend allows researchers to validate theories or challenge assumptions effectively.
The integration of these approaches involves several key steps. First, researchers should clearly define their questions to understand what data is needed. Next, they can gather qualitative data through interviews or focus groups, alongside quantitative data from surveys or experiments. Analyzing both sets of data enables pattern identification and richer insights. Finally, conclusions drawn from this dual analysis can drive actionable recommendations. This synergistic approach not only enhances the depth of research but also guarantees more reliable and trustworthy conclusions.
- Leveraging machine learning for predictive analysis
Machine learning has emerged as a transformative tool for predictive analysis in research. By effectively analyzing large datasets, researchers can uncover patterns and trends that were previously hidden. This capability significantly enhances the decision-making process, allowing for more informed hypotheses and conclusions.
To fully harness the power of machine learning, researchers can follow a few key steps. First, it is essential to identify specific research objectives. Next, selecting the right algorithms tailored to the nature of the data can optimize outcomes. Subsequently, data collection should prioritize quality and relevance, ensuring results are both reliable and beneficial. Finally, integrating the insights obtained from machine learning with traditional research frameworks enriches the entire study, allowing for more comprehensive conclusions.
In this way, the marriage of machine learning and conventional research methods ushers in a new era of data-enhanced research, dramatically improving predictive capabilities and guiding future studies.
Data-Enriched Traditional Research Methods
Data-Enhanced Research integrates traditional research methods with robust data analytics, creating a powerful hybrid approach. This evolution enables researchers to extract deeper insights from qualitative data, such as surveys and interviews. Data-driven surveys enhance accuracy and reveal patterns that might otherwise remain hidden, allowing researchers to make more informed decisions.
Moreover, leveraging data analytics in field studies substantially enhances the research process. By analyzing historical data alongside real-time responses, researchers can adapt their strategies promptly. This not only streamlines research but also facilitates collaboration among teams by consolidating insights in one central location. As a result, organizations can translate findings into actionable business strategies more efficiently, ultimately leading to a competitive edge in their respective markets. Embracing data-enhanced research fosters an environment where traditional methodologies can thrive amidst contemporary challenges.
- Advantages of data-driven surveys and interviews
Data-driven surveys and interviews offer a myriad of advantages that significantly enhance research quality. To begin with, implementing data-driven methods allows for real-time data collection, enabling researchers to adapt their approach as they uncover insights. This flexibility fosters a deeper understanding of participant responses, ensuring that the gathered information is not only relevant but also actionable. As a result, research teams can make informed decisions swiftly, which is crucial for todayโs fast-paced environment.
Another benefit lies in data convergence. Modern tools integrate qualitative feedback from interviews with quantitative metrics from surveys, providing a comprehensive view of the target audience. This synthesis enables researchers to identify patterns and trends that traditional methods might overlook. Additionally, data storage and analysis are streamlined, eliminating inefficiencies. Ultimately, data-enhanced research empowers organizations to not only capture but also effectively utilize customer insights, driving strategic initiatives forward.
- Enhancing field studies with data analytics
Field studies serve as essential pillars in research, offering valuable insights derived from real-world observations. However, the integration of data analytics significantly enhances these studies, transforming raw findings into actionable intelligence. By employing data-enhanced research methods, researchers can uncover patterns and trends that may not be immediately apparent, leading to more informed conclusions and recommendations.
Incorporating analytical tools allows for a deeper exploration of the collected data. For instance, researchers can employ visualization techniques to identify correlations between variables and discern the underlying factors affecting their observations. Moreover, data analytics facilitates the management of large volumes of information, such as customer feedback or survey responses, enabling researchers to derive comprehensive insights efficiently. Ultimately, merging traditional field study approaches with advanced data analytics not only enriches the research process but also ensures greater accuracy in the interpretations drawn from complex datasets.
Extract insights from interviews, calls, surveys and reviews for insights in minutes
Tools to Facilitate Data-Enhanced Research
In the realm of data-enhanced research, various tools facilitate the integration of data science and traditional research methods. These tools enable researchers to effectively collect, analyze, and visualize data, ensuring their findings are both reliable and insightful. For instance, platforms such as RapidMiner and Tableau offer robust features for data manipulation and visualization, while IBM SPSS provides comprehensive statistical analysis capabilities.
A successful data-enhanced research experience begins with clearly defined objectives. Researchers must choose appropriate tools that align with these objectives, ensuring that their data collection processes are thorough. Once data is gathered, practitioners can analyze it through traditional frameworks, enabling a holistic understanding of their findings. This integration ultimately enhances the quality and depth of research outcomes, paving the way for more informed conclusions.
Top Tools for Data and Research Integration
In the quest for robust data-enhanced research, integrating various tools can streamline and elevate the process significantly. Insightful platforms like Tableau and RapidMiner stand out as essential allies, offering intuitive interfaces that help researchers visualize and analyze data effortlessly. Tools such as IBM SPSS and Qualtrics provide advanced statistical capabilities and facilitate comprehensive surveys, making them indispensable for gathering critical information. These tools not only improve data accuracy but also enhance research credibility through rigorous analysis.
To effectively utilize these tools, itโs crucial to align them with clearly defined research objectives. Start by selecting a tool that meets your analytical requirements, followed by systematic data collection and analysis. Finally, interpreting the results through established traditional frameworks ensures that findings are comprehensible and actionable. By harnessing these top tools, researchers can transform static data into meaningful insights, truly bridging the gap between data science and traditional research methodologies.
- insight7
Data-Enhanced Research is transforming how we approach studies by merging traditional research methods with advanced data analysis techniques. By integrating qualitative insights from interviews and quantitative data from surveys, researchers can achieve a comprehensive understanding of their subject matter. This synergy not only enriches the data collected but also enhances the decision-making process, allowing for timely actions based on real-time insights from various customer interactions.
Moreover, the utilization of data analytics in traditional research methods streamlines the entire process. For instance, data-driven surveys can identify trends while qualitative data from customer interviews provides context. This combination allows researchers to interpret findings with greater clarity and effectively strategize future actions. By incorporating these modern techniques, organizations can stay ahead of the competition, ensuring they not only meet but exceed their research goals. As the demand for actionable insights grows, the transition to Data-Enhanced Research will only become more critical.
- RapidMiner
RapidMiner serves as a powerful tool for integrating data science into traditional research methodologies. Its intuitive interface allows researchers to effortlessly upload various data sources, such as transcripts from interviews or survey results, facilitating quick data analysis. This immediacy of access enables researchers to identify patterns and trends in their data, amplifying the insights drawn from qualitative work. By visualizing historical queries, users can extract meaningful quotes and testimonials that further enhance their findings.
Utilizing RapidMiner makes data-enhanced research both efficient and effective. Projects can be customized, allowing researchers to group collected data and apply analytical frameworks to answer specific questions. With options to analyze data in real-time, researchers can adapt their strategies and insights promptly. This synergy of data science and traditional methods elevates research quality, making it more robust and relevant in addressing contemporary issues.
- Tableau
Tableau is a powerful tool that plays a pivotal role in data-enhanced research. It allows researchers to visualize complex data sets effectively, enabling easier interpretation and analysis. With its user-friendly interface, Tableau helps transform raw data into interactive dashboards that reveal insightful patterns. Researchers can quickly identify trends and anomalies within their data, providing a clearer understanding of their findings.
To maximize the benefits of Tableau in research, consider the following steps. First, start with clear research objectives, which will guide your data visualization process. Next, explore various data sources and integrate them into Tableau to create comprehensive visualizations. Once your data is in the system, use Tableauโs vast array of visualization options to present your findings compellingly. Finally, share your dashboards with stakeholders for feedback, ensuring that your research outcomes are well understood and actionable. By utilizing Tableau, researchers can elevate their work, making it more impactful and accessible through data-illustrated insights.
- IBM SPSS
IBM SPSS serves as a pivotal tool in the realm of Data-Enhanced Research. It allows researchers to analyze large sets of data efficiently, integrating both traditional statistical methods and advanced analytical techniques. By enabling easy data visualization and manipulation, it transforms raw information into meaningful insights, enhancing the research experience.
The functionality of this tool supports various research methodologies, including surveys and field studies. Users can refine their analyses, moving beyond simple data review to uncover patterns and correlations within their datasets. The combination of statistical rigor with contemporary data science practices fosters a deeper understanding of research questions, ultimately leading to more informed decision-making and strategy development. Such integration ensures that traditional research methods are not only preserved but are enriched through data science innovations. The synergy is creating new standards for how research is conducted and interpreted in a data-driven world.
- Qualtrics
In the realm of data-enhanced research, the application of robust survey tools can dramatically transform how researchers gather and analyze data. By providing intuitive interfaces and comprehensive analytics, these tools enable researchers to design surveys that yield meaningful insights. This can streamline the integration of traditional research methods with modern data science techniques, fostering a synergy that enhances both qualitative and quantitative research approaches.
Furthermore, the effectiveness of data-enhanced research lies in its ability to create dynamic reports filled with actionable insights. Researchers can utilize real-time analytics to visualize trends, track engagement, and adapt their methodologies accordingly. This responsiveness not only enriches the research experience but also ensures alignment with evolving market demands and user needs. By marrying traditional methods with innovative tools, researchers can produce findings that are not only relevant but also impactful.
Implementation Steps for Using Data Science Tools
Successful implementation steps for using data science tools can significantly enhance your research outcomes. The journey begins by defining clear research objectives. This clarity not only guides the selection process for appropriate tools but also establishes the focus for your data-enhanced research.
Once your objectives are set, itโs essential to choose the right tools tailored to your specific needs. Tools like Tableau or RapidMiner can efficiently handle data visualization and predictive analytics. Next, systematically collect and analyze data, ensuring that it aligns with traditional research methods. Moreover, interpreting results through established frameworks will help bridge the divide between data science and traditional approaches, providing a coherent narrative for your findings. By following these steps, you can seamlessly integrate data-enhanced research into your practices, yielding richer insights and more impactful outcomes.
- Step 1: Define research objectives
Defining research objectives is a fundamental step in the process of Data-Enhanced Research. This stage acts as a blueprint for guiding your inquiries and determining your data needs. Research objectives clarify what you hope to achieve and establish a framework for evaluating success. Clear objectives streamline the data collection process, ensuring that you target relevant information, whether qualitative or quantitative.
To effectively define your research objectives, consider several key aspects. First, identify the research question you want to answer; it should be concise and focused. Next, determine who will benefit from the research and why the data is significant. Lastly, outline how the findings will be analyzed and applied, integrating both traditional research methods and data science techniques. By addressing these elements, you set a solid foundation to engage more deeply with the complexities of your study, combining empirical data with established research frameworks.
- Step 2: Choose appropriate tools
Choosing the right tools is crucial for effective data-enhanced research. The selected tools must align with your research objectives, whether you're gathering qualitative insights or quantitative data. Begin by assessing the specific requirements of your study, such as the type of data you need to collect and the analysis you plan to conduct. This initial evaluation sets the stage for selecting the most suitable tools from the vast array available.
Next, consider how these tools can integrate seamlessly into traditional research methods. Tools like Tableau can provide data visualization that enhances your field studies, while platforms like Qualtrics offer powerful survey solutions tailored to your needs. Familiarity with these tools is essential, especially when presenting findings to stakeholders or using them for compliance evaluations. By strategically selecting tools that combine data science and traditional techniques, you elevate the rigor and reliability of your research outcomes.
- Step 3: Collect and analyze data
Collecting and analyzing data is a critical step in Data-Enhanced Research, blending the strengths of data science with traditional research methods. First, itโs essential to gather data systematically using various approaches, such as surveys, experiments, or existing datasets. Clear objectives guide this process, ensuring that the right type of information is collected to address specific research questions.
Once the data is gathered, analysis becomes vital. This involves employing both statistical techniques and modern data science tools to uncover patterns and insights. Combining qualitative feedback with quantitative metrics adds depth to the analysis, transforming raw information into actionable findings. Understanding these methods not only enhances research effectiveness but also promotes data-driven decision-making, leading to more informed conclusions. Exploring advanced analytics, such as sentiment analysis and predictive modeling, further augments traditional approaches, emphasizing the value of integrating data science into research practices.
- Step 4: Interpret results through traditional frameworks
In Step 4, interpreting results through traditional frameworks involves integrating insights gained from data-enhanced research with established research methodologies. Traditional frameworks provide context and pathways for understanding complex data. While data science offers rich, quantitative analyses, traditional methods enable researchers to add qualitative depth, enhancing overall interpretative power.
To effectively interpret results, consider the following:
Contextualization: Always relate findings to theoretical frameworks or existing literature. This gives depth to your conclusions and makes them more relatable to traditional research audiences.
Framework Alignment: Choose a traditional framework that complements the type of data collected. For instance, if you conducted interviews, thematic analysis may be appropriate to highlight key insights.
Triangulation: Employ multiple methods to validate findings. This strengthens the reliability of your conclusions and aligns with best practices in traditional research.
By interpreting your data in these ways, you create a comprehensive narrative that bridges data science and traditional research methodologies, thereby enriching the academic discussion.
Conclusion: The Future of Data-Enhanced Research in Academia
The future of data-enhanced research in academia promises unprecedented opportunities for innovation and collaboration. As data science continues to evolve, traditional research methods will increasingly adopt data-driven techniques. This synergy will empower researchers to extract more profound insights from their studies, combining qualitative narratives with quantitative evidence.
Moreover, the integration of advanced data analytics into traditional academic settings will foster interdisciplinary collaboration. Researchers will have the capability to address complex questions with greater precision and depth. This progressive shift positions academia to lead in understanding critical societal issues, marking a transformative era in scholarly inquiry and knowledge creation.