Emotion Detection is a vital component of sentiment analysis, providing deeper insights into how individuals feel about a given subject. By understanding the spectrum of emotions expressed in textual data, businesses can better gauge customer satisfaction, preferences, and pain points. In today's data-driven world, extracting these emotional cues allows organizations to fine-tune their strategies and enhance customer experiences.
The growing need for Emotion Detection stems from its potential applications across various domains, including marketing, customer service, and product development. As companies strive to create personalized engagements, accurate emotion detection can guide decision-making processes and drive meaningful connections with customers. Ultimately, integrating this technology into data analysis not only enriches understanding but also fosters a more empathetic approach to business interactions.
Analyze qualitative data. At Scale.
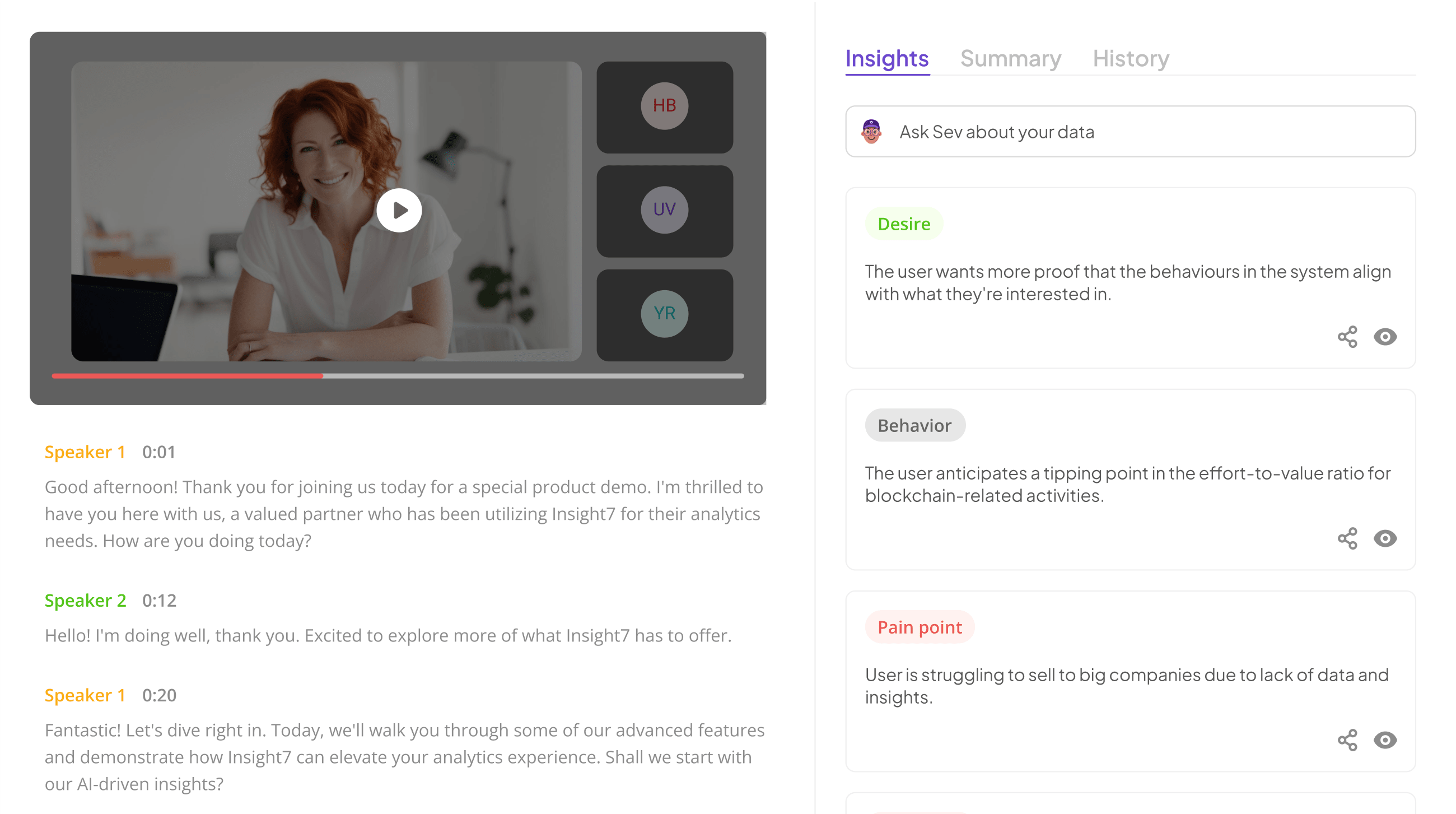
Understanding Emotion Detection: The Heart of Sentiment Analysis
Emotion Detection serves as a crucial element in the realm of sentiment analysis, enabling us to understand and interpret human emotions expressed through data. By identifying various emotional cues within textual information, analysts can gain deeper insights into customer feedback, social media sentiments, and product reviews. This capability not only helps to gauge public opinion but also enables businesses to tailor their strategies effectively.
The process of Emotion Detection involves utilizing advanced algorithms and artificial intelligence techniques to analyze language patterns, word usage, and contextual nuances. By categorizing emotions such as joy, anger, or sadness, organizations are better equipped to respond to customer needs and refine their offerings. Ultimately, understanding Emotion Detection empowers businesses to build stronger connections with their clients by turning raw data into actionable insights, bridging the gap between consumer perceptions and company responses.
What is Emotion Detection?
Emotion Detection refers to the process of identifying and interpreting emotions from various data inputs, particularly textual content. It plays a crucial role in sentiment analysis by enabling machines to understand human emotions, which can be especially useful in fields such as marketing, customer service, and mental health support. By analyzing words, phrases, and context, systems can determine whether the sentiment expressed is positive, negative, or neutral.
The effectiveness of Emotion Detection relies on advanced algorithms and artificial intelligence, which can sift through large volumes of data to extract insights. This technology not only enhances the ability to gauge customer feedback but also helps businesses tailor their communication strategies. By understanding emotional responses, organizations can foster deeper connections with their audience and improve overall engagement. As Emotion Detection continues to evolve, its capacity to transform data into meaningful insights grows, paving the way for more effective interactions in various domains.
The Role of Emotion Detection in Data Analysis
Emotion detection plays a pivotal role in data analysis, significantly enhancing our understanding of user sentiments. By identifying emotional nuances within data, we gain insights that transcend basic sentiment readings. For instance, distinguishing between joy and frustration in customer feedback can drive targeted improvements in products and services.
Understanding emotion detection allows analysts to not only measure positive or negative sentiments but also explore the depths of emotional responses. This depth enables businesses to tailor their strategies effectively, enhancing customer satisfaction and loyalty. Moreover, emotion detection empowers teams to spot patterns across various datasets, enabling quick responses to emerging trends. By incorporating sophisticated techniques, organizations can refine their products while fostering a deeper connection with their audience. As we advance into an era dominated by data, the capacity to gauge emotions will remain essential for informed decision-making and strategic innovation.
Extract insights from interviews, calls, surveys and reviews for insights in minutes
Tools and Techniques for Emotion Detection in Sentiment Analysis
Emotion detection serves as a pivotal aspect of sentiment analysis, enabling the extraction of nuanced emotional insights from textual data. Various tools and techniques have emerged, empowering analysts to conduct in-depth emotional analyses efficiently. A versatile mix of machine learning algorithms and natural language processing engines form the backbone of these tools, transforming unstructured text into actionable insights.
To effectively harness emotion detection, analysts can deploy several key tools. For instance, IBM Watson Natural Language Understanding offers powerful sentiment analysis capabilities, while Google Cloud Natural Language efficiently assesses emotional tone. Microsoft Azure Text Analytics and TextRazor also provide accessible platforms for emotion detection, enhancing workflow efficiencies. Techniques such as lexical analysis, topic modeling, and sentiment classification are essential for structuring the data, allowing analysts to derive meaningful narratives from customer feedback. By utilizing these tools and techniques, businesses can effectively understand and respond to emotional dynamics in their data.
Top Tools for Emotion Detection
In the realm of emotion detection, a variety of sophisticated tools exist that can unveil the underlying sentiments in data. These tools are essential for businesses seeking to analyze customer feedback effectively. They enable organizations to understand emotions like joy, anger, and sadness, providing insights that can influence product development and marketing strategies.
Some of the top tools for emotion detection include well-known platforms like IBM Watson Natural Language Understanding and Google Cloud Natural Language. These platforms utilize advanced algorithms to interpret emotions from textual data, streamlining the analysis process. Moreover, Microsoft Azure Text Analytics and TextRazor offer similar capabilities, ensuring accuracy and depth in data interpretation. By leveraging these tools, businesses can gain a nuanced understanding of customer opinions and sentiments, empowering them to make informed decisions and enhance overall customer experience.
- insight7
Understanding the intricacies of Emotion Detection is crucial for any effective sentiment analysis strategy. This involves identifying emotions expressed in text, which can be as straightforward as categorizing sentiments into positive, negative, or neutral. However, it goes beyond basic classification; it seeks to uncover deeper emotional undercurrents, such as joy, anger, or sadness embedded within data. This complexity is vital in helping businesses decode customer feedback, enhancing their overall service and product offerings.
Incorporating advanced techniques for Emotion Detection can transform raw data into meaningful insights. By analyzing customer reviews, social media posts, and other textual interactions, organizations can grasp their audience's feelings. Such understanding allows for timely responses, informed decision-making, and the potential to build lasting relationships. In the end, utilizing emotion detection methodologies effectively not only fosters customer satisfaction but also positions companies at a competitive advantage in today's fast-paced market.
- IBM Watson Natural Language Understanding
Emotion Detection in sentiment analysis utilizes advanced algorithms to help understand feelings expressed in text. In this context, one prominent tool provides in-depth insights into user experiences. This tool analyzes vast amounts of data to identify emotional cues, enabling businesses to gauge public sentiment effectively. Through its powerful natural language processing abilities, it deciphers nuances in language, recognizing varying emotional states.
Furthermore, it utilizes contextual understanding to improve the accuracy of emotion detection, which is vital in tailoring responses to customer feedback. By employing this technology, organizations can drive better engagement, adapt marketing strategies, and enhance overall customer satisfaction. The automation of these processes not only saves time but also provides valuable insights into consumer behavior, leading to more informed decision-making.
- Google Cloud Natural Language
The power of emotion detection in analyzing data is remarkable, especially with advanced tools that streamline this process. One notable option offers a suite of features that can evaluate sentiment in text, identifying positive, negative, or neutral emotions. Users can analyze large volumes of text, facilitating a deeper understanding of customer feelings towards products and services. This functionality is crucial for marketers, allowing them to tailor strategies according to consumer sentiment.
Additionally, this tool excels in entity analysis, pinpointing significant terms and their accompanying sentiments. For businesses, this means uncovering insights into customer preferences and pain points, ultimately aiding in decision-making. The intuitive interface makes it easier to visualize data trends over time. Using emotion detection technology enhances customer engagement by aligning business actions with sentiment insights, fostering stronger connections between brands and consumers.
- Microsoft Azure Text Analytics
Microsoft Azure Text Analytics offers powerful capabilities for Emotion Detection, enabling users to derive sentiment insights from large datasets. This tool employs machine learning algorithms to analyze text and recognize emotional nuances embedded within user-generated content, such as reviews or social media posts. With its intuitive interface, it allows businesses to identify positive and negative sentiments, which play a critical role in understanding customer feedback.
Emotion Detection through Azureโs platform enhances data-driven decision-making by providing real-time insights. Organizations can utilize these insights to tailor their strategies, improving product offerings and customer interactions. By systematically analyzing emotions expressed in customer communications, businesses can foster a deeper connection with their audience. Additionally, Azureโs analytic capabilities support custom queries that help it recognize patterns across various datasets, further facilitating comprehensive sentiment evaluations. This integration of advanced technology empowers companies to transform emotional insights into actionable strategies, ultimately enriching customer experiences.
- TextRazor
TextRazor is a powerful tool that plays a vital role in effectively implementing emotion detection within sentiment analysis. By utilizing advanced natural language processing techniques, this tool can disentangle layers of meaning in text, helping organizations to assess sentiments accurately. Understanding emotions from data is crucial for deriving insights about user experiences and improving engagement strategies.
This tool's capabilities extend to providing detailed analyses of text data, which can reveal varying emotional tones. By examining keyword associations and contexts, it enables businesses to identify positive, negative, or neutral sentiments in customer feedback. Ultimately, by incorporating such tools into their analysis, companies can transform raw data into actionable insights. The integration of emotion detection into sentiment analysis is not just a technological solution but a strategic advantage in connecting with audiences on a deeper level.
Techniques for Implementing Emotion Detection
Implementing emotion detection involves several techniques that can enhance the analysis of sentiments captured in various datasets. Firstly, natural language processing (NLP) algorithms, which decode and interpret textual data, play a crucial role in identifying emotional cues. By analyzing the context and syntactic structures, these algorithms can classify emotions expressed in a text, ranging from joy to anger and everything in between.
Secondly, machine learning models can be trained to recognize patterns indicative of specific emotions. This involves feeding the models vast amounts of labeled data and allowing them to learn the nuances of emotional expression. Additionally, sentiment lexicons and pre-built emotion classification frameworks can complement these models by providing a reference point for emotion identification. Overall, these techniques empower analysts to extract meaningful emotional insights, driving significant business decisions and enhancing customer interactions.
Conclusion: The Future of Emotion Detection in Data Analysis
The future of emotion detection in data analysis promises exciting advancements that will enhance our understanding of human sentiments. As technology evolves, we can expect increased accuracy in interpreting emotions, leading to more effective decision-making. This progress will empower organizations to analyze consumer feedback, tailor marketing strategies, and improve customer experiences significantly.
Moreover, integrating emotion detection with emerging tools like AI will streamline the process of extracting valuable insights from vast datasets. As we move forward, harnessing this technology could redefine how businesses connect with their audiences, fostering deeper relationships rooted in understanding and empathy. The potential of emotion detection is immense, offering organizations a key to unlock new opportunities in data-driven analysis.