Understanding buying signal analysis within quality assurance (QA) systems is essential for any researcher aiming to identify key consumer behaviors. By analyzing buying signals, researchers can uncover critical insights that indicate when potential customers are ready to make a purchase. This analysis serves as a bridge between observed customer expressions and actionable marketing strategies, empowering organizations to respond effectively.
QA systems enhance the ability to scrutinize data, providing a structured method to capture and analyze buying signals. Through sophisticated data processing and integration techniques, these systems allow researchers to transform ambiguous customer behavior into clear, actionable insights. Consequently, understanding buying signal analysis is not just about gathering data; it's about interpreting that data to drive more effective research outcomes and business strategies.
Analyze qualitative data. At Scale.
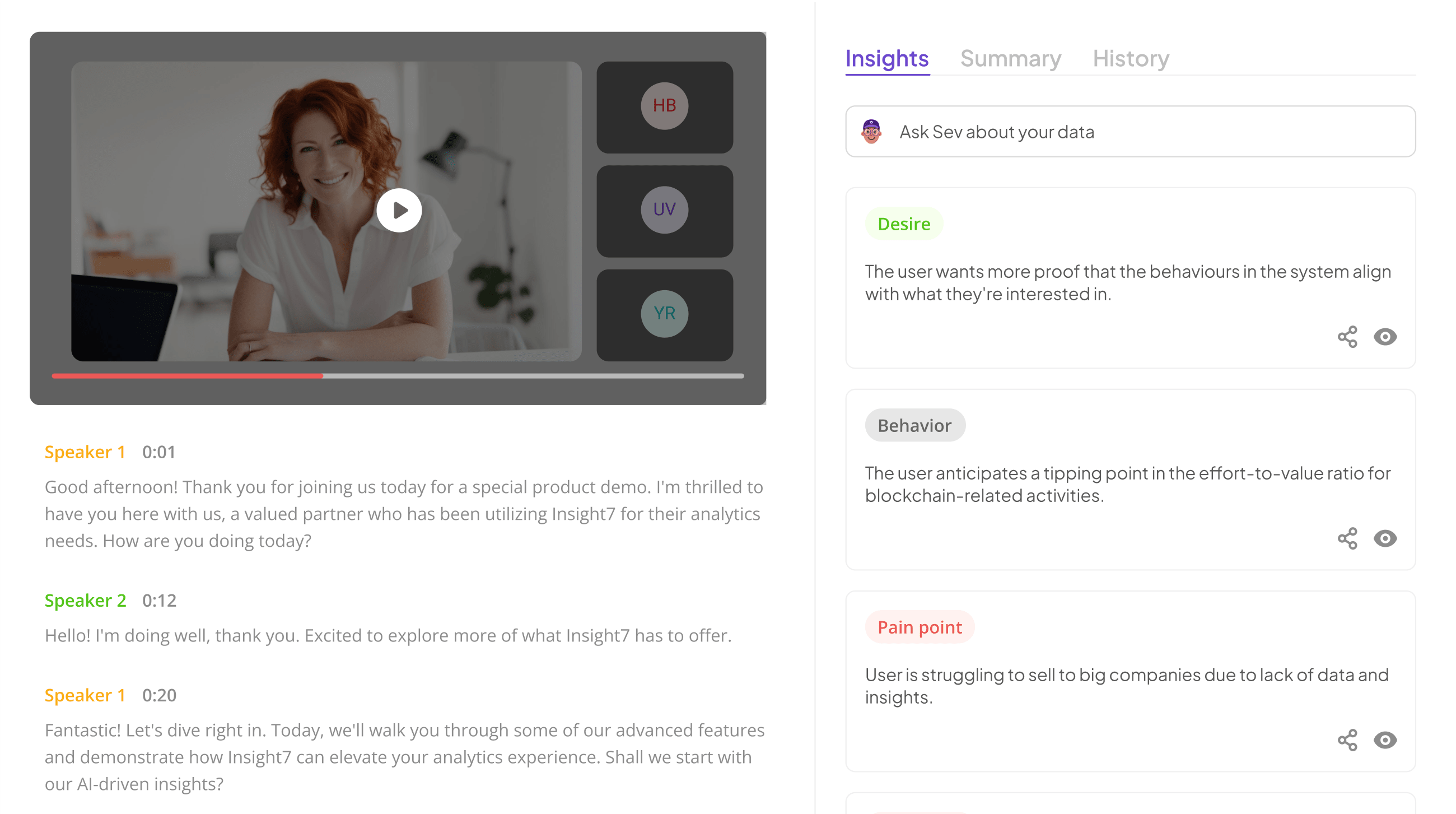
The Role of QA Systems in Buying Signal Analysis
QA systems play a crucial role in buying signal analysis by ensuring that data is accurately captured and interpreted. These systems provide researchers with the tools necessary to evaluate the nuances of customer interactions. By systematically assessing conversations and engagements, QA systems help identify key moments when a customer shows intent to purchase. The ability to analyze transcripts and feedback allows businesses to refine their strategies and tailor their approaches to meet consumer needs effectively.
Implementing effective buying signal analysis within QA systems involves several key steps. First, itโs essential to identify relevant data sources that capture customer interactions, whether from calls, chats, or surveys. Second, configuring data processing techniques enables researchers to filter and analyze the information efficiently. Finally, integrating QA systems with advanced analysis tools enhances the accuracy of signal detection. Together, these steps create a comprehensive framework that empowers businesses to react promptly to buying signals and optimize their offerings accordingly.
Enhancing Data Interpretation through Buying Signal Analysis
Data interpretation is crucial for researchers aiming to uncover buying signals. Enhancing this process through Buying Signal Analysis allows teams to make informed decisions based on tangible insights. By dissecting customer feedback and interactions, researchers can identify key patterns reflecting consumer intent. This analysis transforms raw data into structured visualizations, illuminating both positive and negative feedback responses.
Utilizing strategic tools and methodologies enhances the accuracy of signal identification. Researchers should embrace techniques such as sentiment analysis, utilizing variables like location and context to derive deeper insights. For instance, comparing feedback across different geographical datasets can reveal unique trends and preferences. With Buying Signal Analysis at the forefront, teams can streamline their objectivity, ensuring that insights drive product development and marketing strategies effectively. By focusing on actionable data, organizations can foster a more significant connection between their offerings and customer needs, ultimately enhancing their market position.
Steps to Implementing Effective Buying Signal Analysis in QA Systems
In order to effectively implement buying signal analysis in QA systems, a systematic approach is essential. Begin by identifying relevant data sources. This involves gathering information from customer interactions, sales calls, and market reactions. Ensuring that the right data is captured is critical, as it directly influences the accuracy of buying signal analysis. Next, configure data processing techniques that transform raw data into actionable insights. Establishing clear parameters for analysis helps in honing in on specific buying signals relevant to your products or services.
Integrating QA systems with analysis tools is the final essential step. This integration allows for seamless data flow and enhances the ability to track and evaluate buying signals over time. These systems can also facilitate the automation of report generation, making it easier for teams to respond promptly to identified buying triggers. By following these steps, organizations can harness the power of buying signal analysis to gain a competitive edge in their respective markets.
- Step 1: Identifying Relevant Data Sources
Identifying relevant data sources is foundational in the process of Buying Signal Analysis. Begin by mapping out the types of data that can provide insights about customer behavior and preferences. These include customer feedback, purchase histories, and social media interactions. By gathering data from varied sources, researchers can create a comprehensive view of buyer intent, signaling when potential customers are most likely to make a purchase.
Next, prioritize your data sources based on reliability and relevance. Consider utilizing surveys, focus groups, and analytics tools that track user behavior online. Each of these data sources offers unique insights that can be cross-referenced for accuracy, enhancing the overall analysis process. Establishing a well-rounded data collection strategy will ensure that researchers can effectively identify and act upon buying signals, driving informed decisions that lead to successful sales outcomes.
- Step 2: Configuring Data Processing Techniques
To effectively analyze buying signals, configuring data processing techniques is a critical step. Begin by establishing clear objectives for your data processing setup. Identify what specific buying signals you want to detect, whether they are related to customer inquiries, purchase behaviors, or engagements. This foundational step ensures that the data you collect is aligned with your analysis goals, ultimately enhancing the relevance and accuracy of the insights drawn.
Next, streamline your data processing methods. This involves selecting appropriate tools and automating repetitive tasks to improve efficiency. Set up templates for categorizing data based on various buyer behaviors. Additionally, ensure that the system can cross-reference data from multiple sources for a comprehensive view. This systematic approach not only optimizes your analysis but also solidifies your understanding of potential buying signals in real-time, enabling timely and informed decision-making.
- Step 3: Integrating QA Systems with Analysis Tools
Integrating QA systems with analysis tools is crucial for refining buying signal analysis. By combining quality assurance frameworks with advanced analytical tools, researchers can unearth valuable insights from customer interactions. This synergy enables a real-time assessment of buying signals, revealing the nuances of customer behavior and intent.
To achieve effective integration, first, ensure that your QA systems can seamlessly communicate with chosen analysis tools. This may involve standardizing data formats and ensuring compatibility between platforms. Next, implement continuous feedback loops where analysis outcomes inform and refine QA processes. Lastly, utilize visualization techniques that translate data into actionable insights, making it easier for researchers to identify and respond to buying trigger signals. Following these steps will enhance your ability to track and interpret customer cues, ultimately driving better decision-making in sales and marketing strategies.
Extract insights from interviews, calls, surveys and reviews for insights in minutes
Top Tools for Buying Signal Analysis in QA Systems
In today's competitive market, employing the right tools for buying signal analysis in QA systems is crucial for researchers looking to uncover key purchasing triggers. These tools help streamline the process of gathering and interpreting data, allowing users to derive actionable insights from customer interactions. By leveraging advanced analytics solutions, organizations can effectively identify potential buyers' needs and intentions, ensuring timely engagement and support.
Key tools for effective buying signal analysis include platforms such as Salesforce Einstein Analytics and IBM Watson Discovery. These tools offer powerful data-processing capabilities that transform unstructured information into structured insights. Additionally, Google Cloud Natural Language provides exceptional text analysis features, while NetBase Quid excels in social listening and market intelligence. Integrating these tools into QA systems enhances overall analytical capabilities, empowering teams to make informed decisions and enhance client relationships in a rapidly evolving landscape.
insight7: Leading the Charge in Buying Signal Analysis
Understanding buying signal analysis is paramount for organizations seeking to effectively interpret customer intent and preferences. As the market evolves, the volume of customer interactions is increasing, leading to a wealth of data. However, conventional approaches often fail to keep pace with this growing influx. Insight7 stands at the forefront of this challenge, empowering teams to harness and make sense of these signals.
The first step is to identify specific signals that indicate buying intent. This includes not only direct inquiries about products but also subtle cues during customer interactions. Following this identification, it is essential to utilize advanced data processing techniques to extract actionable insights from the noises of the data. Finally, integrating analytical tools with quality assurance systems ensures that organizations can respond rapidly and effectively to market demands, positioning themselves ahead of their competitors. By adopting these strategies, businesses can transform buying signals into strategic advantages.
Additional Tools Enhancing Buying Signal Detection
Incorporating additional tools can significantly enhance buying signal detection for researchers. Various advanced technologies can amplify the efficiency and accuracy of buying signal analysis, ensuring that key insights do not go unnoticed. Each tool brings unique capabilities to the table, enabling organizations to analyze vast datasets quickly and effectively.
Among the top recommended tools are Salesforce Einstein Analytics, which excels at predictive insights, and IBM Watson Discovery, recognized for its AI-driven analytics. Google Cloud Natural Language offers powerful text analysis, which can help uncover consumer sentiments, while NetBase Quid specializes in social media analytics, providing insights into market trends. Each of these tools allows researchers to capture nuanced buying signals, transforming raw data into actionable strategies that align with business objectives. Adopting a combination of these strategies is crucial for staying ahead in a competitive market.
- Salesforce Einstein Analytics
Salesforce Einstein Analytics stands out as a powerful asset for conducting Buying Signal Analysis. Its capability to analyze vast amounts of data enables researchers to identify critical buying triggers with precision. By harnessing advanced analytics and AI, it provides deep insights into buyer behavior, which is crucial for understanding market dynamics.
To effectively utilize this tool for Buying Signal Analysis, researchers should follow several key steps. First, they need to explore and define their data sources clearly, ensuring that all relevant information feeds into the analytics engine. Next, it is essential to configure data processing techniques that accurately capture trends and patterns from this data. Finally, seamless integration with other QA systems allows for real-time monitoring and analysis, enriching the research approach and elevating strategic decision-making. In doing so, Salesforce Einstein Analytics empowers researchers to surface buying trigger signals with greater confidence and clarity.
- IBM Watson Discovery
IBM Watson Discovery serves as a powerful tool for researchers seeking to enhance buying signal analysis. It utilizes natural language processing and machine learning to sift through vast data volumes, identifying key insights that can influence purchasing decisions. This AI-driven solution transforms unstructured data into structured formats, making it easier for researchers to track buying signals.
The platform excels in extracting relevant information by analyzing customer interactions and feedback. Researchers can utilize the insights gathered to understand preferences and trends. By integrating IBM Watson Discovery into their existing workflows, organizations can streamline their processes, allowing for more timely and accurate decision-making. Ultimately, its ability to uncover latent buying signals empowers businesses to respond effectively to shifting market dynamics.
- Google Cloud Natural Language
Google Cloud Natural Language shines as a robust tool for buying signal analysis. By applying natural language processing (NLP) technologies, this tool transforms raw data from discussions and inquiries into actionable insights. This capability enables researchers to synthesize customer interactions, uncovering the underlying motivations for purchases.
Its features include sentiment analysis, entity recognition, and syntax analysis, which collectively enhance the understanding of customer behavior. Researchers can efficiently sift through vast amounts of text data, such as call transcripts or social media comments, to identify patterns that signify buying intent. Additionally, integrating this tool with QA systems optimizes the analysis process, allowing for real-time insights that inform marketing strategies. As researchers dive deeper into buying signals, the clarity offered by this technology can significantly enhance decision-making and alignment with market demands.
- NetBase Quid
NetBase Quid stands out as a powerful tool in the realm of Buying Signal Analysis, enabling researchers to decode complex consumer behaviors. This platform excels in processing vast volumes of data to identify trends and patterns that signify purchasing intent. By using advanced analytics and comprehensive data visualization, users can gain deeper insights into customer motivations and market dynamics.
Moreover, the ability to analyze multiple data sources concurrently enhances the platform's capability to surface buying triggers. This means researchers can swiftly react to emerging trends and adjust their strategies accordingly. With real-time insights at their disposal, organizations can transition from traditional sales approaches to more consultative engagements with customers. Ultimately, this enhances the overall customer experience by aligning product offerings more closely with what consumers truly desire. By leveraging such insights, businesses can make informed decisions, driving their success across competitive markets.
Conclusion: Leveraging QA Systems for Effective Buying Signal Analysis
In conclusion, QA systems play a vital role in conducting effective buying signal analysis. These systems enable researchers to collect, transcribe, and analyze vast amounts of data seamlessly, unlocking insightful patterns in customer behavior. By utilizing templates tailored to specific evaluation criteria, organizations can assess customer interactions and detect potential buying triggers more accurately.
Furthermore, integrating advanced analysis tools enhances the interpretation of buying signals, allowing for more strategic decision-making. As researchers harness these capabilities, the landscape of buying signal analysis becomes richer, enabling businesses to respond proactively to customer needs and preferences. This methodology ultimately drives more informed marketing strategies and improves overall engagement with potential buyers.