Predictive Sentiment Analysis in QA Software is revolutionizing the way companies understand customer feedback. Imagine harnessing powerful algorithms to predict customer feelings before they even express them. This technology not only provides insights into user experiences but also enhances the decision-making process within quality assurance teams.
At its core, Predictive Sentiment Analysis analyzes vast amounts of data, including testimonials and recorded interactions, to deliver actionable insights. By identifying patterns in sentiment, businesses can proactively address potential issues, leading to more informed product developments and improved customer satisfaction. As industries evolve, understanding predictive sentiment will determine the leaders in effective quality assurance systems.
Analyze qualitative data. At Scale.
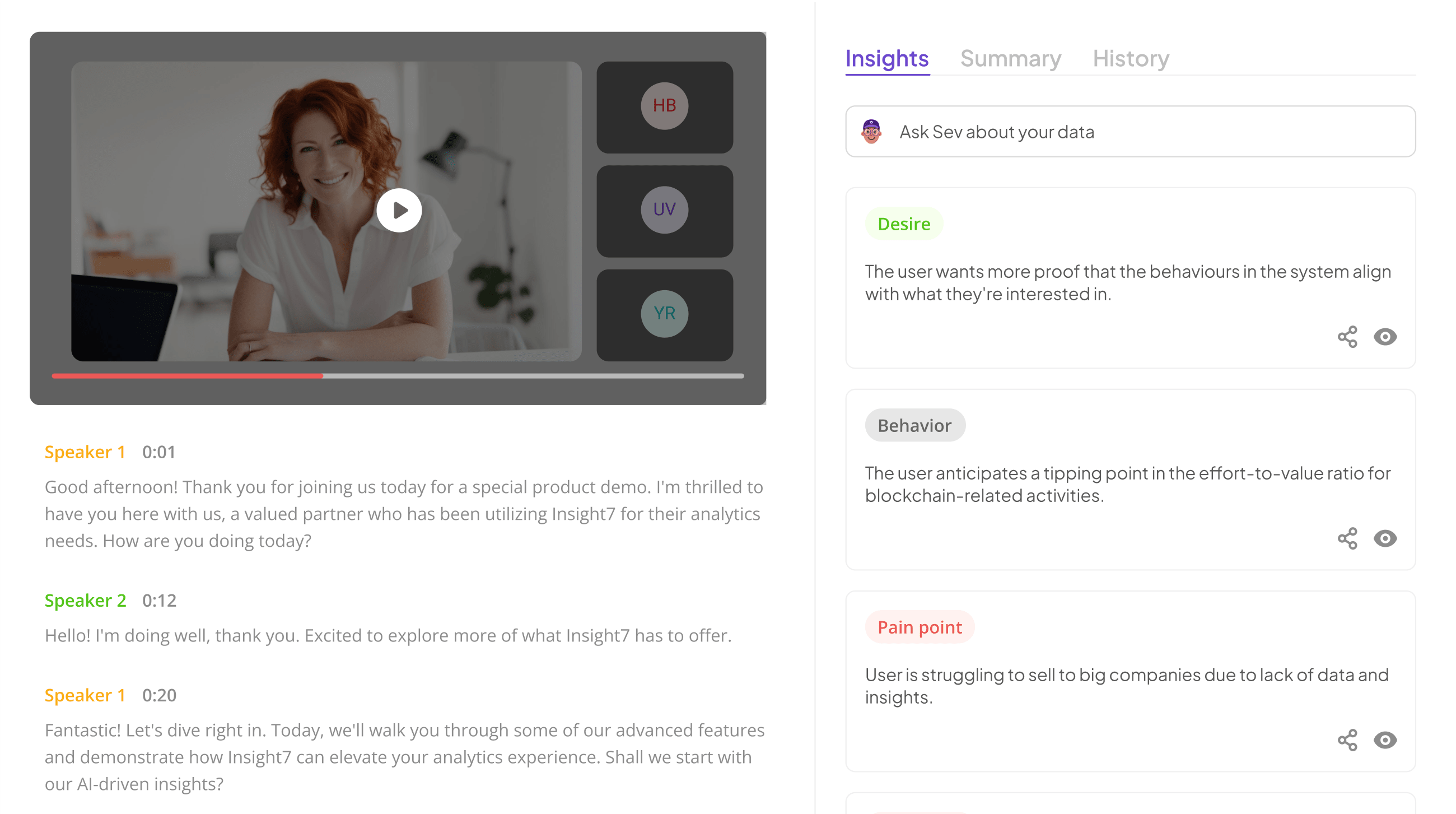
Understanding Predictive Sentiment Analysis in QA Software
Predictive Sentiment Analysis is a method that processes and interprets customer sentiment based on data, often derived from interactions like calls or chats. By utilizing advanced algorithms, it can quantify customer emotions, categorizing feedback as positive, negative, or neutral. This technology not only decodes sentiments but also reveals underlying trends and themes that emerge from large sets of data.
In the realm of quality assurance (QA) software, understanding how Predictive Sentiment Analysis works can significantly enhance evaluations of customer engagements. The process begins with data collection from various channels, such as customer calls or online chats. Subsequently, the software analyzes this data against predetermined criteria, offering insights into the quality of customer interactions. By identifying emotional responses, QA teams can proactively address issues and optimize customer service, making it a crucial tool for any organization aiming to enhance overall customer satisfaction.
What is Predictive Sentiment Analysis?
Predictive Sentiment Analysis refers to a sophisticated method of evaluating customer emotions expressed in various forms of data. This technique utilizes advanced algorithms to analyze sentiments from text, voice, and social media inputs, providing insights into user feelings. By identifying whether sentiments are positive, negative, or neutral, organizations can better understand customer experiences and predict future behaviors.
The technology driving this analysis encompasses natural language processing (NLP) and machine learning, which enable the recognition of nuances in human communication. Specifically, it involves scanning large datasets for patterns that reveal sentiments toward a product or service. These insights can be instrumental in shaping business strategies, improving customer service, and enhancing product development. Ultimately, Predictive Sentiment Analysis transforms raw feedback into actionable insights, offering companies a strategic advantage in understanding and responding to customer needs.
- Definition and core concept
Predictive Sentiment Analysis refers to the process of using data-driven techniques to gauge sentiments expressed in customer interactions. This technology identifies feelings, attitudes, or emotions conveyed in text, enabling businesses to respond proactively to customer needs. By analyzing communication records, such as calls or chat transcripts, organizations can derive actionable insights about their customer bases.
At its core, Predictive Sentiment Analysis operates by employing natural language processing and machine learning algorithms. These tools assess the tone, context, and content of communications, transforming subjective sentiments into quantifiable data. This enables businesses to spot emerging trends, understand customer pain points, and enhance overall experiences. Overall, Predictive Sentiment Analysis serves as an essential tool in quality assurance software, helping businesses stay ahead in an increasingly competitive landscape.
- The technology driving sentiment scoring
Predictive Sentiment Analysis relies on advanced technologies like machine learning and natural language processing. By analyzing vast amounts of customer feedback, these technologies help organizations assess sentiments expressed in comments, reviews, and discussions. This analysis identifies patterns and trends in customer emotions, enabling businesses to understand their audience better.
The first component of this technology is data processing, where raw data is converted into actionable insights. It involves collecting data from multiple sources, such as social media, surveys, and support interactions. The second aspect is sentiment scoring, which assigns values to feedback based on its emotional tone. Machine learning algorithms continuously improve this scoring method, allowing for more accurate predictions over time. By harnessing these technologies, organizations can uncover valuable insights that guide product development and enhance customer satisfaction.
How Predictive Sentiment Analysis Works in QA
Predictive Sentiment Analysis begins with the systematic collection and processing of customer feedback. This involves gathering data from various sources, including surveys, social media, and customer interactions. By employing advanced algorithms, the software parses this data to identify emotional tones, categorizing feedback as positive, negative, or neutral. Such nuanced insights help QA teams to pinpoint issues that require immediate attention or highlight strengths within a product or service.
Once the data is processed, sentiment scoring methods are employed to quantify emotional responses. These scores allow organizations to gauge customer satisfaction effectively. By tracking sentiment trends over time, teams can proactively address potential problems, enhancing the overall customer experience. The application of Predictive Sentiment Analysis thus empowers QA initiatives, transforming feedback into actionable insights and fostering a deeper understanding of client needs. In this way, the technology plays a critical role in shaping responsive, customer-centered QA practices.
- Data collection and processing
Data collection and processing are crucial elements in the realm of Predictive Sentiment Analysis for QA software. The process begins with gathering data from diverse sources, often including customer feedback, product reviews, and social media interactions. This data serves as the foundation for developing insights that guide decision-making. Ensuring the quality and relevance of the collected data is paramount, as it directly influences the accuracy of sentiment analysis outcomes.
Once data is collected, the next step involves processing it to extract meaningful patterns and sentiments. This typically involves employing natural language processing algorithms that categorize sentiments as positive, negative, or neutral. Understanding the nuances in language, such as slang or regional dialects, enhances the effectiveness of sentiment scoring. This comprehensive approach not only enriches the analysis but also reinforces the idea that Predictive Sentiment Analysis can be a powerful tool in improving customer experience and product development.
- Sentiment scoring methods
Sentiment scoring methods play a vital role in Predictive Sentiment Analysis, transforming qualitative feedback into quantitative data. These methods utilize both machine learning and natural language processing technologies to interpret customer emotions and opinions accurately. By analyzing textual data from multiple sources, such as customer reviews, feedback, or transcripts, these methods generate sentiment scores that help businesses understand customer reactions effectively.
Several techniques are employed within sentiment scoring, including keyword analysis, where specific words or phrases are analyzed for their emotional weight. Another approach is context-based sentiment scoring, which considers the surrounding text to ensure accurate interpretation. Additionally, machine learning models can continuously learn from new data, refining their scoring methods over time. This adaptability enhances the accuracy of sentiment analysis, allowing organizations to react proactively to customer needs and concerns, thus driving overall satisfaction and loyalty.
Extract insights from interviews, calls, surveys and reviews for insights in minutes
The Impact of Predictive Sentiment Analysis: Game-Changer or Gimmick?
The notion of Predictive Sentiment Analysis has sparked significant debate in various industries. Many herald it as a transformative tool enabling organizations to gauge customer sentiments more accurately than ever before. This capability could lead to enhanced customer insights and proactive resolution of issues, leveling up customer experience. However, not everyone is convinced. Critics argue that the technology may lack the accuracy needed for critical decision-making, labeling it as a potential gimmick rather than a game-changer.
Additionally, the ethical implications surrounding data privacy raise concerns about its reliability. Organizations must navigate this complex landscape carefully, ensuring they employ best practices. To evaluate its effectiveness, it's essential to observe real-world implementations, which reveal both successes and failures. Learning from these cases can provide valuable insights into whether Predictive Sentiment Analysis truly represents a significant breakthrough in quality assurance software or merely an overhyped trend.
Potential Benefits of Predictive Sentiment Analysis
Predictive Sentiment Analysis offers numerous advantages that can significantly enhance quality assurance processes. First and foremost, it provides enhanced customer insights by analyzing feedback patterns. By understanding customer sentiment in real time, organizations can tailor their products or services to better meet user expectations. This insight helps companies not only understand what their customers enjoy, but also pinpoint areas for improvement.
Additionally, predictive sentiment analysis facilitates proactive problem resolution. Instead of waiting for issues to arise, businesses can anticipate customer concerns and address them before they escalate. This proactive approach leads to higher customer satisfaction and retention rates. By combining these benefits, predictive sentiment analysis emerges as an essential tool for organizations striving to improve product quality and enhance user experience in a competitive market.
- Enhanced customer insight
Enhanced customer insight is pivotal in today's rapidly evolving market, where understanding customer sentiment can significantly influence business strategies. Predictive sentiment analysis serves as a catalyst for this comprehension, allowing companies to tap into the wealth of data generated from customer interactions. By leveraging advanced algorithms, organizations can assess customer emotions and opinions, transforming these insights into actionable strategies.
Several factors contribute to enhanced customer insight through predictive sentiment analysis. First, the ability to process vast amounts of qualitative data from customer feedback helps in identifying trends that may otherwise go unnoticed. Second, real-time analysis allows businesses to respond proactively, improving customer satisfaction. Third, this analysis fosters more meaningful conversations with customers, leading to deeper relationships. Together, these elements create a robust understanding of customer needs and preferences, making predictive sentiment analysis a game-changer in customer engagement.
- Proactive problem resolution
Anticipating and addressing customer issues before they escalate is essential in the realm of Quality Assurance (QA) software. Predictive Sentiment Analysis plays a crucial role in achieving proactive problem resolution. By evaluating customer feedback in real-time, organizations can identify potential grievances or concerns. This allows teams to intervene promptly, enhancing overall customer satisfaction and loyalty.
To effectively harness Predictive Sentiment Analysis for problem resolution, consider the following strategies:
Monitoring Feedback: Continually track customer interactions to detect negative sentiments early. This helps in recognizing patterns that may indicate dissatisfaction.
Customized Solutions: Utilize insights gained from sentiment analysis to tailor responses that meet specific customer needs. Personalized communication can alleviate confusion and foster trust.
Continuous Learning: Analyze resolved issues to enhance future interactions. By understanding the effectiveness of your resolutions, you create a feedback loop that informs ongoing strategy.
Implementing these approaches not only enables teams to resolve issues swiftly but also fortifies customer relationships. By embedding predictive capabilities into your QA process, your organization can significantly reduce customer churn and improve service quality.
Limitations and Challenges
Predictive Sentiment Analysis presents several limitations and challenges that users must navigate to fully harness its potential. One major challenge lies in the accuracy and reliability of sentiment scoring. Automated systems may misinterpret nuances in language, leading to incorrect assessments. Variability in terminology and context can significantly impact the results, causing stakeholders to question the dependability of the analysis.
Furthermore, ethical considerations and data privacy issues must be addressed. The collection of personal data for sentiment analysis raises concerns about consent and security. Users may feel uncomfortable knowing their sentiments are being analyzed without full understanding. These limitations highlight the need for continuous improvement in technology and guidelines for ethical usage, ensuring that the benefits of predictive sentiment scoring are not overshadowed by its pitfalls. As such, organizations must consider both the capabilities and the inherent challenges of integrating this technology in their operations.
- Accuracy and reliability concerns
Predictive Sentiment Analysis can significantly enhance customer experience, but accuracy and reliability concerns pose substantial challenges. Firstly, the algorithms used to analyze sentiment may misinterpret nuanced human emotions, leading to incorrect assessments. Misinterpretations can arise from cultural differences, idiomatic expressions, or sarcasm, resulting in misleading scores that affect decision-making processes.
Additionally, data quality plays a crucial role in the reliability of predictive analysis. If the datasets used for training the models lack diversity or represent biased viewpoints, the final sentiment scoring will reflect those flaws. Proper calibration and continuous monitoring of algorithms are essential to maintain accurate outcomes. Thus, ensuring that the predictive sentiment analysis process is built on high-quality, representative data is vital to minimize risks associated with false conclusions and to enhance the overall effectiveness of QA software.
- Ethical considerations and data privacy
Ethical considerations and data privacy are paramount in predictive sentiment analysis, particularly within QA software applications. As technology evolves, it becomes crucial to assess how data is collected and utilized. With predictive sentiment analysis, vast amounts of personal information may be analyzed to gauge customer sentiment. Such practices raise significant ethical questions regarding consent, data ownership, and privacy.
Two primary concerns emerge in this space. First, the necessity for informed consent is critical. Users should be aware of how their data is being collected and processed. Secondly, data security measures must be robust to protect sensitive information, such as social security numbers and identifiable personal details. Organizations must implement stringent policies to mitigate risks associated with data breaches. Addressing these ethical considerations is essential to building trust and maintaining high standards in utilizing predictive sentiment analysis technology.
Case Studies: Successes and Failures
Case studies examining the effectiveness of predictive sentiment analysis reveal both successes and failures that are invaluable for understanding its true potential. In several successful implementations, organizations report enhanced customer insights, enabling teams to make data-driven decisions that significantly improve product offerings. This proactive approach allows businesses to resolve issues before they escalate and raises overall customer satisfaction.
Conversely, some attempts have not yielded the anticipated results due to challenges such as data integrity and algorithm inaccuracies. Lessons learned from these failures highlight the importance of ensuring reliable data sources and maintaining ethical practices around data privacy. By carefully analyzing both successful and unsuccessful case studies, businesses can formulate better strategies for incorporating predictive sentiment analysis into their QA processes.
- Real-world examples of implementation
Implementing Predictive Sentiment Analysis in real-world settings has yielded significant insights and improvements for various organizations. In one case, a customer service platform used the technology to analyze calls, identifying common issues raised by customers. This led to a series of actionable changes, including updates to website content and enhanced training programs for staff. By effectively utilizing the gathered sentiment data, the platform could better respond to customer needs, fostering a more positive service experience.
In another instance, a marketing team adopted Predictive Sentiment Analysis to drive their advertising strategies. By examining customer feedback, they tailored campaigns to address specific concerns and preferences, resulting in increased engagement and conversion rates. This example highlights the importance of integrating sentiment analysis into decision-making processes. These implementations showcase how organizations can transform raw data into valuable insights, ultimately driving continuous improvement and customer satisfaction.
- Lessons learned from unsuccessful attempts
Unsuccessful attempts to implement Predictive Sentiment Analysis in QA can teach us valuable lessons about its limitations. Firstly, many organizations rushed into adopting sentiment analysis without first understanding their unique needs and context. This often led to the deployment of ineffective tools that failed to capture meaningful insights. Secondly, inaccurate data is a significant hurdle; when the foundations of data quality are shaky, the analysis suffers.
Another common pitfall is neglecting user feedback to shape the implementation process. Ignoring the voices of frontline employees can result in overlooking practical challenges. It's crucial to recognize that integration with existing systems requires careful planning and adaptability. Blinded by technology's allure, some teams underestimated the importance of ongoing evaluations and adjustments. Ultimately, learning from these setbacks fosters a more informed approach to using Predictive Sentiment Analysis, ensuring it evolves into a genuinely valuable asset rather than a superficial gimmick.
Top Tools for Predictive Sentiment Analysis in QA Software
In the realm of QA software, selecting the right tools for predictive sentiment analysis is essential. Various tools excel in processing large volumes of data, offering insights that can drive strategic decisions. These tools utilize advanced algorithms to analyze customer feedback, allowing quality assurance teams to preemptively address potential issues. By integrating such tools, organizations can transform data into actionable insights that enhance customer satisfaction.
Notable options include Insight7, which provides an intuitive platform for analyzing customer conversations and extracting critical sentiment metrics. Other tools in the market offer unique features, such as customizable dashboards and real-time analytics, helping users adapt quickly to changing customer sentiments. Effective use of these tools can ultimately lead to improved product quality and a more engaged customer base, confirming the value of predictive sentiment analysis in today’s competitive landscape.
Insight7
In the realm of QA software, Predictive Sentiment Analysis offers a fresh perspective on understanding customer feedback and enhancing user experiences. This method analyzes textual data, extracting sentiments to provide insights that were hard to obtain through traditional analysis. By employing advanced algorithms, businesses can rapidly interpret customer emotions, allowing them to act on critical feedback promptly.
The benefits of implementing this analysis are manifold. First, companies gain deeper insights into customer satisfaction, enabling proactive engagement strategies and facilitating timely interventions. Second, organizations can identify patterns in feedback that signal underlying issues, fostering an agile response to resolve concerns efficiently. However, it is essential to remain aware of potential drawbacks, such as concerns regarding accuracy and data privacy. Understanding these aspects is crucial for organizations aiming to harness the full potential of Predictive Sentiment Analysis in their QA efforts.
- Overview and key features
The integration of Predictive Sentiment Analysis in QA software marks a transformative shift in how organizations evaluate customer interactions. This technology utilizes advanced algorithms to process data, enabling businesses to gauge emotional responses and satisfaction levels from various communication channels. By leveraging insights derived from customer feedback, companies can identify pain points and desires, facilitating more effective decision-making.
Key features of this software often include user-friendly interfaces that do not require extensive training, making them accessible to all employees. Enhanced reporting capabilities allow teams to convert raw data into actionable insights promptly. Additionally, these tools often support multi-file analysis, enabling businesses to simultaneously process hundreds of customer interactions, thereby streamlining workflows and improving efficiency. Overall, predictive sentiment analysis can drive strategic improvements in product and service offerings, positioning organizations to stay competitive in evolving markets.
Other Notable Tools
In the realm of Predictive Sentiment Analysis, several other notable tools complement existing software and enhance functionality. Tool A stands out for its advanced machine learning algorithms, which provide accurate sentiment scoring based on diverse data inputs. This tool excels in real-time analysis, enabling businesses to react swiftly to customer feedback.
Tool B brings a user-friendly interface, ensuring teams can easily navigate sentiment metrics. Its capability to visualize data helps teams identify trends and insights that inform decision-making. Meanwhile, Tool C integrates seamlessly with existing platforms, simplifying data sharing across departments and boosting collaboration. Finally, Tool D emphasizes data security and ethical handling, addressing growing concerns around privacy and compliance.
These tools demonstrate that while predictive sentiment analysis can be transformative, selecting the right software can significantly impact outcomes. Teams should consider specific needs and capabilities to leverage these tools effectively, maximizing the potential of predictive sentiment analysis in their QA processes.
- Tool A
Tool A is an innovative software solution designed to integrate predictive sentiment analysis into quality assurance processes. This tool leverages advanced algorithms to analyze customer interactions, providing insights into user sentiment with unprecedented accuracy. By processing large volumes of data swiftly, it allows businesses to gauge how customers feel about their products or services in real-time.
One of the standout features of Tool A is its ability to generate actionable insights from user feedback. It not only identifies trends in sentiment but also highlights potential areas for improvement, allowing companies to address issues proactively. Furthermore, its user-friendly interface makes it accessible for teams of varying technical expertise. As organizations increasingly recognize the value of predictive sentiment analysis, Tool A positions itself as a crucial asset in fostering customer satisfaction and driving quality assurance initiatives.
- Tool B
Tool B emerges as a noteworthy contender in the realm of predictive sentiment analysis, particularly when applied within QA software. This tool capitalizes on advanced algorithms to analyze customer feedback while generating insightful sentiment scores. The main advantage lies in its ability to swiftly process complex data, allowing teams to identify trends and make informed decisions. Such capabilities can radically transform how quality assurance teams monitor customer interactions, equipping them with the knowledge to enhance service quality.
Utilizing predictive sentiment analysis, Tool B stands out for its intuitive interface and integration capabilities with existing workflows. The analytics provided aid in predicting customer satisfaction levels and potential pain points. This foresight enables organizations to proactively address concerns, fostering a better customer experience. By harnessing the power of this tool, companies can move beyond reactive measures, positioning themselves as leaders in quality assurance while embracing innovation.
- Tool C
In the realm of predictive sentiment analysis, Tool C stands out by offering specific functionalities tailored to enhance customer insights. This tool utilizes advanced algorithms to sift through extensive data sets, identifying mood indicators and sentiments expressed in user interactions. By analyzing these sentiments, organizations can gain a deeper understanding of customer satisfaction and areas that may require attention.
Another notable feature of Tool C is its ability to provide real-time feedback. This ensures that businesses can proactively address customer concerns before they escalate. Effective sentiment analysis can drive improvements in products and services, ultimately leading to higher customer retention rates. Additionally, with the insights provided by Tool C, teams can make informed decisions that streamline compliance procedures and enhance overall quality assurance efforts.
Overall, Tool C illustrates how predictive sentiment analysis can serve as a valuable asset in modern QA software, allowing for improved operational efficiency and customer engagement.
- Tool D
Tool D brings a unique perspective to the realm of Predictive Sentiment Analysis, potentially revolutionizing how QA software assesses customer interactions. This tool leverages advanced algorithms to process vast amounts of data, providing insights into customer sentiment with unmatched speed and accuracy. Its predictive capabilities enable organizations to anticipate customer needs, enhancing the overall user experience.
One of the standout features of Tool D is its adaptability to various industry contexts, allowing for tailored sentiment scoring methods. This versatility ensures that the scoring aligns closely with specific customer expectations and regulatory standards. Additionally, by integrating real-time feedback analysis, Tool D safeguards against potential issues before they escalate, empowering teams to address concerns proactively rather than reactively. In a rapidly evolving market, such tools exemplify how technology can be a true game-changer in quality assurance.
Conclusion: The Future of Predictive Sentiment Analysis in QA Software
Predictive Sentiment Analysis holds considerable promise for the future of QA software, evolving beyond its initial stages. As organizations increasingly adopt data-driven approaches, this technology can transform feedback into actionable insights, enabling teams to preemptively address customer concerns. By tapping into sentiment scores, businesses can enhance their products and services more effectively, leading to improved customer satisfaction.
However, the long-term success of Predictive Sentiment Analysis depends on continuous refinement of algorithms and data handling practices. Addressing challenges such as accuracy and ethical data usage will be critical for sustaining user trust. As the field matures, the balance between innovation and responsibility will shape the implications of this technology in enhancing quality assurance processes.