Analytical methodology evolution marks a pivotal shift in the way research is conducted, bridging the gap between traditional methods and modern predictive analytics. This transformation has been driven by advancements in technology, enabling researchers to process data more efficiently and extract valuable insights. As the field evolves, understanding the implications of these changes becomes essential for those seeking to stay relevant in an increasingly data-driven landscape.
The emergence of predictive analytics represents a significant departure from conventional research techniques. While traditional methods, grounded in established practices, serve as a foundation, they often struggle with agility and scalability. The advantages of predictive analytics lie in its ability to analyze vast datasets and surface trends that can inform decision-making. This section explores the journey of analytical methodologies, examining how predictive analytics offers unprecedented opportunities for uncovering insights and enhancing research efficacy.
Analyze & Evaluate Calls. At Scale.
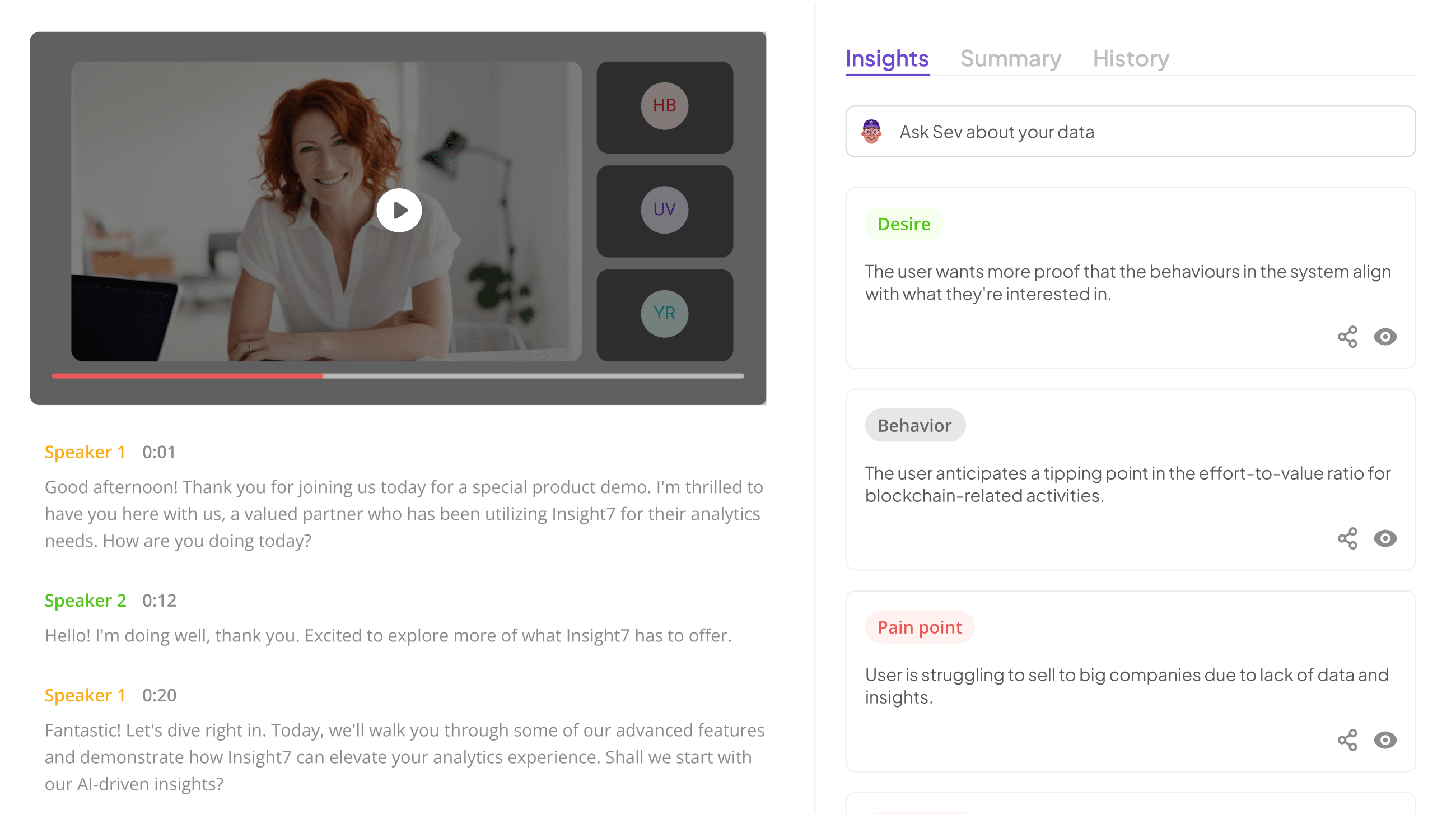
Understanding the Analytical Methodology Evolution: Predictive Analytics
In recent years, the analytical methodology evolution has prominently featured predictive analytics, fundamentally transforming how researchers approach data interpretation. At its core, predictive analytics involves using statistical algorithms and machine learning techniques to identify the likelihood of future outcomes based on historical data. This data-driven approach leverages vast amounts of information to create more accurate insights, enabling businesses to respond proactively to changing market conditions.
The rise of predictive analytics can be attributed to several key drivers, including the significant increase in data availability and advancements in computational power. Organizations increasingly rely on predictive models to streamline decision-making processes and enhance customer experiences. Unlike traditional research methods that often focus on descriptive analytics, predictive analytics empowers organizations to forecast trends, optimize strategies, and drive innovation by harnessing the potential of machine learning and statistical modeling. This evolution marks a pivotal shift in research methodologies, illustrating the growing importance of advanced analytics in todayโs data-centric landscape.
The Rise of Predictive Analytics in Modern Research
Predictive analytics has emerged as a game-changing approach in modern research, marking a significant departure from traditional methodologies. By leveraging complex algorithms and statistical techniques, predictive analytics enables researchers to identify patterns and forecast future outcomes with unprecedented accuracy. The rise of big data and advancements in computing power have further accelerated this evolution, making it easier for industries to embrace predictive models for strategic decision-making.
One of the key drivers of this transformation is the increasing demand for timely and actionable insights. Unlike traditional methods that often rely on historical data and slow, manual analysis, predictive analytics offers real-time data interpretation, allowing organizations to respond swiftly to market changes. With tools that can analyze vast datasets, researchers gain the ability to uncover hidden trends and correlations, leading to more informed decisions and a competitive edge in their respective fields. As businesses recognize the value of these capabilities, the adoption of predictive analytics continues to expand, reshaping how research is conducted across various sectors.
- Definition and core principles of predictive analytics
Predictive analytics is a branch of data analysis that focuses on using historical data to forecast future outcomes. It employs various statistical techniques, machine learning algorithms, and data mining practices to identify patterns and build models that predict future events. By analyzing past behaviors, organizations can uncover insights that inform strategic decision-making. This approach stands out as part of the broader evolution of analytical methodologies, reshaping how research is conducted.
The core principles of predictive analytics include data-driven insights, statistical reasoning, and model validation. Organizations rely on these principles to uncover trends that traditional research methods may miss. Data-driven insights allow stakeholders to make informed choices, while statistical reasoning provides a framework for evaluating potential outcomes. Model validation ensures that predictions are reliable and actionable, thus driving successful strategic initiatives. Embracing predictive analytics can significantly enhance an organization's ability to adapt to changing environments, ultimately leading to improved performance and competitiveness.
- Key drivers of its adoption in recent years
Predictive analytics has witnessed significant adoption in recent years due to various key drivers that have reshaped research methodologies. First, the explosive growth of data in numerous sectors has necessitated advanced analytical methods capable of harnessing this information effectively. Organizations seek to derive actionable insights to remain competitive, prompting a shift towards predictive analytics as a primary research tool.
Secondly, technological advancements have made sophisticated analytical tools more accessible. These tools allow researchers to process and analyze large datasets rapidly, enabling real-time decision-making that traditional research methods often lack. Additionally, the integration of artificial intelligence and machine learning into predictive analytics enhances its capabilities, providing deeper insights and more accurate forecasts. Such developments signify a clear shift in the analytical methodology evolution, where organizations are increasingly prioritizing real-time insights over traditional data collection methods.
Key Tools for Predictive Analytics
In the landscape of predictive analytics, various tools significantly enhance data analysis capabilities. These tools, crucial to the analytical methodology evolution, empower organizations to transform raw data into actionable insights efficiently. Recognizing the key tools is essential for harnessing the full potential of predictive analytics in research.
Firstly, Insight7 offers a comprehensive suite for meaningful insights, making it indispensable in predictive analytics. Tableau stands out for its visual data analysis functions, allowing users to create predictions intuitively. SAS provides advanced analytics and multivariate analysis capabilities, essential for deeper understanding of complex data. IBM SPSS excels in data mining and text analytics, facilitating thorough exploration of trends and patterns. Lastly, RapidMiner focuses on data preparation and predictive modeling, simplifying the analytical process. Each tool serves a unique purpose, yet collectively they drive the evolution of analytical methodologies in research, enabling better decision-making based on data-informed insights.
- Insight7: A leading tool in predictive analytics for enhanced insights.
In the realm of predictive analytics, Insight7 stands out as a leading tool that significantly enhances insights across various sectors. This platform transforms how companies analyze customer data, enabling them to harness insights that were previously elusive with traditional research methods. The evolution of analytical methodology is evident as organizations increasingly rely on tools that can process vast amounts of data quickly and effectively.
Companies using Insight7 can adapt to changing market conditions and customer sentiments with unprecedented speed. By automating the analysis of qualitative data from interviews and feedback, Insight7 ensures that insights are readily available for decision-making. This efficiency empowers organizations to convert raw data into strategic actions, overcoming challenges often observed in conventional approaches. As the demand for responsive and data-informed strategies grows, tools like Insight7 become indispensable, underscoring the critical shift towards predictive analytics in research methodologies.
- Tableau: Visual data analysis for predictions.
Tableau represents a significant advancement in the analytical methodology evolution, particularly in the realm of predictive analytics. By transforming raw data into intuitive visualizations, it empowers researchers and businesses to make informed decisions quickly and effectively. Unlike traditional research methods, which often rely on static data reporting and manual analysis, Tableau allows users to interact dynamically with their data, uncovering trends and patterns that might otherwise remain hidden.
In addition to its user-friendly interface, Tableau enables real-time data analysis, which is essential for developing accurate predictions. Users can create interactive dashboards, filter data, and visualize outcomes with ease, making the analytical process more engaging and efficient. As institutions embrace predictive analytics over traditional methods, Tableau stands out as an invaluable tool, helping teams harness the full potential of their data for insightful decision-making and strategic planning.
- SAS: Advanced analytics and multivariate analysis.
SAS stands out in the realm of advanced analytics due to its robust multivariate analysis capabilities. This powerful tool empowers researchers to dissect complex data sets, revealing underlying patterns and relationships that traditional research methods often overlook. By integrating various statistical techniques, SAS enables users to gain profound insights from data, enhancing the overall analytical methodology evolution.
Multivariate analysis plays a crucial role in understanding how multiple variables interact simultaneously. This approach allows researchers to explore potential influences and predict outcomes with greater accuracy. Moreover, SAS provides visual data representation, making it easier to communicate findings effectively. As the landscape of predictive analytics advances, SAS continues to evolve by integrating new technologies and methodologies. Embracing such advanced tools is essential for researchers who wish to stay at the forefront of data-driven discovery and innovation.
- IBM SPSS: Data mining and text analytics.
Data mining and text analytics are crucial components in understanding and analyzing large datasets effectively. These methods have evolved significantly, adapting to the increasing complexities of data in various fields. By employing sophisticated techniques, analysts can extract valuable insights from unstructured information, enhancing decision-making processes. The platform facilitates this by allowing users to integrate and analyze data from multiple sources seamlessly, streamlining the workflow.
As analytical methodologies evolve, the relevance of traditional research methods is increasingly scrutinized. While conventional approaches have laid a foundation for data analysis, they often lack the flexibility to adapt to new data types and scales. Data mining techniques complement these traditional methods by enabling deeper exploration and revealing patterns that might not be evident. This synergy between old and new methodologies marks a significant step forward in the quest for actionable insights, highlighting the importance of embracing innovation in research practices.
- RapidMiner: Data preparation with predictive modeling.
Data preparation is a critical element in the predictive modeling process, serving as a bridge between raw data and actionable insights. Through intuitive project management features, users can easily import various data sources, whether from transcripts, files, or cloud storage. This flexibility allows teams to unify different datasets, creating a comprehensive foundation for analysis. By organizing and synthesizing this data effectively, researchers can identify patterns and trends that traditional methods might overlook.
The processing capabilities further enhance predictive analytics by simplifying complex queries. For instance, project users can extract specific insights or testimonials relevant to their inquiries effortlessly. This data-driven approach fosters an environment where understanding customer sentiments and behaviors becomes streamlined. In an era where the analytical methodology evolution is paramount, utilizing sophisticated tools for data preparation can significantly elevate the quality of predictions and decision-making processes. Adjusting to these advancements is essential for organizations hoping to remain competitive in their respective fields.
Extract insights from interviews, calls, surveys and reviews for insights in minutes
Comparing Analytical Methodology Evolution: Traditional Research Methods
Traditional research methods have been the cornerstone of information gathering for decades. Their structured approaches, relying on surveys, interviews, and observational studies, provided valuable insights and a solid foundation upon which further research could be built. This methodology emphasizes gathering qualitative and quantitative data through established protocols, ensuring credibility and reliability in findings. However, as analytical methodology evolution has taken place, the limitations of these traditional approaches have become increasingly evident.
The constraints of traditional research methods often impact their effectiveness in a rapidly changing environment. Rigid questions can lead to biased results, while lengthy data collection processes might miss timely insights. Moreover, the inability to swiftly analyze large data sets can hinder researchers from responding to emerging trends. In some instances, areas like consumer behavior and market dynamics demand more adaptive methodologies, revealing a gap where traditional research might not suffice. This has prompted a shift toward more dynamic strategies, such as predictive analytics, seeking to enhance decision-making through more robust insights.
The Foundation of Traditional Research Methods
Traditional research methods have laid the groundwork for understanding and analyzing complex phenomena over decades. These methodologies, such as surveys, interviews, and focus groups, have historically provided valuable insights into consumer behavior and market trends. Even as analytical methodology evolves, these foundational approaches continue to play a crucial role in research, helping organizations gather qualitative and quantitative data.
The significance of traditional research methods lies in their structured frameworks that ensure rigorous data collection and analysis. Researchers often rely on these methods for their ability to produce reliable findings, despite the growing popularity of predictive analytics. While traditional methods have limitations, such as being time-consuming and sometimes unable to capture rapidly changing market dynamics, they remain essential for certain research areas. Their continued relevance highlights the importance of a balanced approach that appreciates both historical methods and innovative analytics.
- Overview of traditional research methodologies
Traditional research methodologies have evolved significantly over time, yet their foundational principles remain relevant. These methodologies include various qualitative and quantitative approaches, such as surveys, focus groups, and statistical analysis. Each method aims to gather information systematically, enabling researchers to draw conclusions based on collected data. Understanding these methods provides insight into their historical significance and their role in shaping contemporary research practices.
Despite their reliability, traditional research methods face limitations, particularly in adaptability and efficiency. For instance, they often require substantial time and resources to collect and analyze data. In sectors that demand rapid decision-making, such as marketing, these traditional approaches may not suffice. As the field progresses, the analytical methodology evolution drives the need for innovative tools like predictive analytics, which offer quicker and more dynamic insights into complex datasets. By integrating both traditional and modern methods, researchers can achieve a comprehensive understanding of their subject matter.
- Historical significance and their continued use
The evolution of analytical methodologies has roots deeply embedded in historical research practices. Traditional research methods have served as the backbone of data collection and analysis, providing foundational insights across various disciplines. Despite the emergence of predictive analytics, these traditional methods retain their significance due to the rigor and structure they bring to research. Their systematic approach allows researchers to collect qualitative and quantitative data effectively and ensures that the findings are grounded in thorough investigations.
Moreover, many researchers continue to utilize these traditional methods alongside modern techniques. They enhance the validity of results and offer a comprehensive understanding of complex issues, enriching the analytical landscape. While predictive analytics offers speed and forecast capabilities, the historical significance of traditional methodologies lies in their ability to establish credibility and trustworthiness. The blend of both approaches represents a harmonious evolution, acknowledging the past while advancing toward future research possibilities.
Limitations and Challenges of Traditional Methods
Traditional research methods have served as foundational approaches for data collection and analysis for many years. However, these methodologies face significant limitations in todayโs fast-paced environment. One key challenge is their inherent rigidity; they often struggle to adapt quickly to changing market dynamics and consumer preferences. Traditional methods, such as surveys and focus groups, require substantial time to gather and analyze data, resulting in delayed insights that can hinder decision-making.
Moreover, the scope of traditional methodologies is often limited. They may not capture emerging trends or complex behaviors since they rely heavily on structured questions and predefined answers. Research areas such as dynamic customer behaviors and real-time market fluctuations reveal these weaknesses. As predictive analytics rises in prominence, it highlights the need for more agile and responsive analytical methodologies, illustrating the growing importance of evolving our research approaches to stay competitive.
- Discuss inherent limitations in scope and adaptability
Traditional research methods, while foundational, face intrinsic limitations concerning their scope and adaptability in comparison to emerging predictive analytics. One significant limitation is their reliance on historical data, which may not always reflect current trends or future possibilities. This static nature can lead to outdated conclusions that are less relevant in rapidly changing environments, such as technology or consumer behavior.
Moreover, traditional methods often lack the flexibility required to adapt to new data or shifting contexts. While they provide valuable insights, they may not effectively address complex research questions that demand agility. On the other hand, predictive analytics offers a dynamic approach, allowing researchers to model various scenarios and make informed predictions based on real-time data analysis. This adaptability is crucial in todayโs data-driven landscape, ensuring that methodologies can evolve alongside new findings and market trends. As the analytical methodology evolution continues, understanding these limitations is essential for developing more robust and responsive research strategies.
- Examples of research areas where traditional methods fall short
Traditional research methods often fall short in dynamic and rapidly changing environments, particularly in areas like market trends, consumer behavior, and technology adoption. One major drawback is their reliance on historical data, which may not accurately predict future patterns. For instance, industries experiencing technological disruption require real-time insights to remain competitive. Traditional approaches can struggle to provide these timely analyses due to their slower data processing and interpretation.
Another significant area where they falter is in the complexity of customer sentiment analysis. Traditional surveys and focus groups may capture surface-level feedback but often miss the nuanced emotions that drive customer decisions. This gap in understanding can lead to misaligned strategies and lost opportunities. As the landscape of research evolves, the shift toward predictive analytics becomes essential for businesses aiming to leverage data effectively and transform insights into actionable strategies.
Conclusion: Bridging the Gap in Analytical Methodology Evolution
The evolution of analytical methodologies signifies a transition from traditional research methods to innovative predictive analytics. This shift is crucial in addressing today's complex challenges, where real-time data insights can enhance decision-making processes. As organizations strive for effective strategies, bridging the gap between these methodologies becomes essential for maximizing research outcomes.
Integrating predictive analytics with traditional approaches offers a comprehensive view of data analysis. The combination allows researchers to harness the strengths of both methods, enhancing accuracy and relevance in findings. By embracing this analytical methodology evolution, businesses can stay ahead of market trends and deliver actionable insights, ultimately driving success in their respective fields.