Customer Analytics Insights serve as a crucial compass for businesses navigating the intricate landscape of consumer behavior. By harnessing the power of machine learning, organizations can derive actionable insights that inform marketing strategies, product development, and overall customer engagement. This section will introduce the synergy between customer analytics and machine learning, illustrating how data-driven decisions can enhance customer experiences and drive business growth.
As we delve deeper, it's important to understand the foundational concepts that underpin this relationship. Customer Analytics Insights not only reveal trends and preferences but also enable predictive modeling, allowing businesses to anticipate customer needs effectively. By combining these insights with machine learning techniques, businesses can unlock new opportunities, ultimately fostering stronger relationships with their customers while driving successful outcomes.
Generate visualizations from your qualitative data. At Scale.
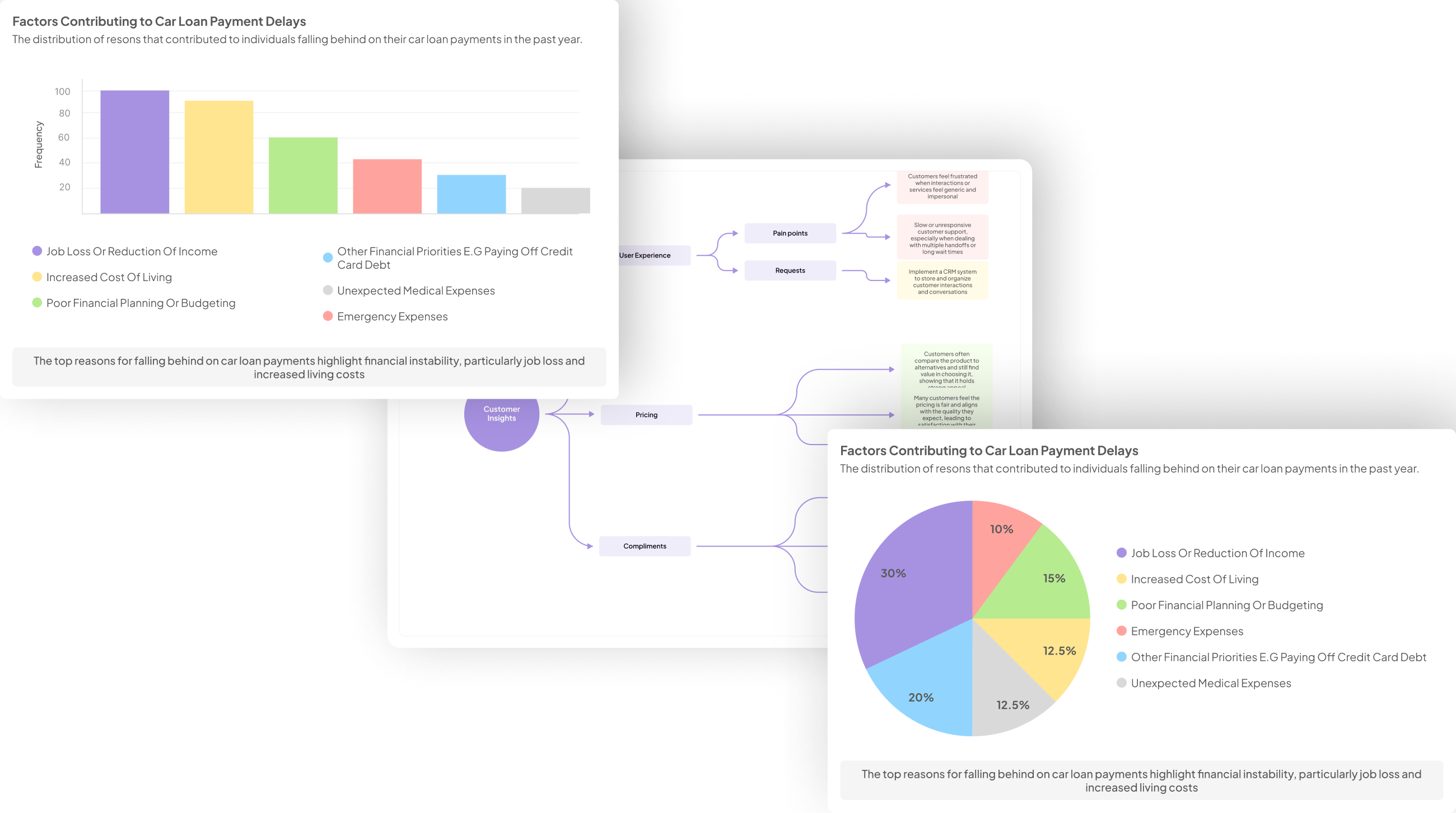
Understanding Machine Learning and Customer Analytics Insights
Machine learning has transformed how businesses gather and interpret Customer Analytics Insights. This innovative approach enables organizations to process vast amounts of customer data efficiently, revealing patterns and trends that might not be immediately visible. By harnessing algorithms and computational power, businesses can personalize experiences, predict customer behavior, and make informed decisions that drive growth.
Understanding the dynamics of machine learning and its application in customer analytics is crucial for businesses looking to stay competitive. Key components, such as data preparation, model training, and results analysis, play a significant role in ensuring accurate insights. As companies increasingly generate data, adopting a proactive stance toward insights becomes pivotal. In this evolving landscape, businesses that effectively implement machine learning will better serve their customers, enhancing satisfaction and loyalty. Ultimately, machine learning offers a powerful lens through which to understand Customer Analytics Insights, paving the way for strategic innovation.
The Role of Machine Learning in Customer Analytics Insights
Machine learning plays a crucial role in extracting valuable customer analytics insights that drive informed business decisions. It enables companies to analyze vast amounts of data quickly, uncovering patterns and trends that are often invisible through traditional methods. By automating data analysis, machine learning enhances the efficiency of gaining insights from customer interactions, whether from interviews, survey responses, or online behavior.
There are several key areas where machine learning significantly enhances customer analytics insights. First, it allows businesses to segment their customer base effectively, identifying distinct groups with unique behaviors. Second, predictive analytics helps forecast customer preferences and behaviors, allowing for more personalized marketing strategies. Third, machine learning algorithms continuously improve over time, adapting to new data and trends, ensuring that organizations remain agile and competitive. Harnessing these capabilities not only provides a deeper understanding of customer needs but also facilitates timely decision-making that can lead to business growth.
Key Benefits of Leveraging Machine Learning for Customer Insights
Machine learning offers transformative advantages for extracting customer analytics insights that are often difficult to achieve through traditional methods. By utilizing advanced algorithms, businesses can delve into vast datasets, uncovering patterns and trends that inform decision-making processes. This capability allows organizations to respond more rapidly and effectively to customer needs, enhancing overall satisfaction and loyalty.
Key benefits include improved accuracy in predictions regarding customer behavior, which aids in refining marketing strategies. Additionally, machine learning automates data analysis, reducing the workload on teams and allowing them to focus on strategic initiatives. Furthermore, these insights can lead to personalized customer experiences, driving engagement and retention. In summary, harnessing machine learning in customer analytics not only streamlines the insight-gathering process but also empowers businesses to adapt dynamically to changing consumer demands.
Generate Journey maps, Mind maps, Bar charts and more from your data in Minutes
Implementing Machine Learning for Customer Analytics Insights: Step-by-Step Guide
Implementing Machine Learning for Customer Analytics Insights involves a systematic approach to ensure effective utilization of data. The initial step is to define clear objectives that align with business goals. This sets a strong foundation for meaningful insights that can drive strategic decisions. Following this, data collection and preparation are crucial. Quality data is the lifeblood of machine learning, so it's imperative to gather diverse and relevant datasets to feed into the models.
Next, selecting an appropriate machine learning model is essential. Various algorithms serve different purposes, and understanding each one's strengths will enhance analytical outcomes. Once you've chosen a model, the training phase begins. Analyzing the results helps refine the model for accuracy. Finally, deploying the model allows businesses to generate actionable Customer Analytics Insights, enabling ongoing optimization and informed decision-making. This step-by-step guide empowers organizations to harness machine learning effectively for customer understanding and engagement.
Step 1: Define Customer Analytics Objectives
In order to effectively harness Customer Analytics Insights, the first step is to define clear objectives. Without well-defined goals, any analytics effort can lead to confusion and wasted resources. Begin by identifying the specific questions you want to answer, such as understanding customer behavior or improving customer retention. This step ensures that the insights generated will be directly aligned with your business needs and capable of driving actionable outcomes.
Next, consider who your target audience is. Understanding their preferences and pain points is crucial. This background knowledge enables you to tailor your analytics objectives accordingly, which can help in prioritizing what data to collect. By establishing a framework that specifies the metrics to measure success, such as customer satisfaction rates or conversion rates, you can track progress effectively. This structured approach allows organizations to use Customer Analytics Insights to refine their strategies and improve customer relationships significantly.
Step 2: Collect and Prepare Data for Machine Learning
To successfully harness machine learning for customer analytics, it is crucial to collect and prepare data meticulously. This step sets the foundation for deriving accurate Customer Analytics Insights. Begin by identifying relevant data sources, which may include customer databases, transaction histories, and feedback forms. Ensure data variety, as diverse datasets enrich the analysis process.
Next, data cleaning is essential. Remove duplicates, correct inconsistencies, and handle missing values to achieve data integrity. Once cleaned, transforming data into an appropriate format is necessary for machine learning algorithms. This includes normalizing numerical values and encoding categorical data, which enhances the model's ability to learn effectively. Pay close attention to feature selection, as choosing relevant variables significantly impacts the quality of your customer insights. Ultimately, a well-prepared dataset not only improves model performance but also enhances the accuracy of the insights derived, providing a clearer understanding of customer behaviors and preferences.
Step 3: Select the Appropriate Machine Learning Model
Selecting the appropriate machine learning model is crucial for deriving meaningful Customer Analytics Insights. The success of your analysis hinges on choosing a model that aligns with both the nature of your data and the specific objectives you've defined. Start by categorizing your problem—whether it is predictive analytics, classification, or clustering. Each of these areas benefits from different types of algorithms, such as regression models for predictions and decision trees for classifications.
Once you've identified the type of problem you are addressing, evaluate various model options. Consider their complexity, interpretability, and performance. Basic models can offer quick insights but may lack depth, while more complex algorithms can offer higher accuracy but require more extensive data. It’s essential to experiment with multiple models and use cross-validation techniques to gauge their effectiveness. Ultimately, your goal is to select a model that not only provides accurate insights but also allows for actionable recommendations in your customer analytics strategy.
Step 4: Train the Model and Analyze Results
Training the model is a pivotal step in developing Customer Analytics Insights. This process transforms raw data into actionable knowledge by teaching the model how to recognize patterns. By providing it with historical data, the model learns to predict customer behaviors and preferences with greater accuracy. Furthermore, implementing various algorithms allows practitioners to discover which ones yield the best results for the specific dataset involved.
After training is complete, analyzing the results becomes critical. This phase involves evaluating the model's predictions against actual outcomes to measure accuracy. Metrics such as precision, recall, and F1 score provide insights into the model's performance. An essential part of this evaluation is understanding how these insights can inform future marketing strategies and product developments. By continuously refining the model based on feedback and data analysis, businesses can achieve deeper Customer Analytics Insights, leading to more tailored offerings and enhanced customer satisfaction.
Step 5: Deploy Model and Generate Customer Analytics Insights
Deploying your machine learning model marks a significant transition from analysis to action. In this step, the focus shifts towards generating Customer Analytics Insights that can inform strategic decisions. Upon deployment, the model will begin processing new customer data in real-time, allowing for prompt updates on customer behavior and preferences. Effective deployment ensures that stakeholders can access insights effortlessly, facilitating quicker response times to evolving market dynamics.
To maximize the value of Customer Analytics Insights, consider the following strategies:
Integrate with Existing Systems: Ensure the model seamlessly connects with your CRM or data management systems. This allows for real-time data flow and immediate insight generation.
Conduct Regular Monitoring: Continuously track the model's performance and the accuracy of insights being generated. Adjustments may be needed based on changes in customer behavior or market conditions.
Visualize Insights: Utilize dashboards to present insights in a user-friendly manner. This enhances accessibility for various teams and aids in decision-making processes.
Solicit Feedback: Gather input from users on the utility of the insights. This helps refine the model and address any concerns regarding data understanding.
By implementing these strategies, businesses can leverage their model effectively, driving informed decisions and ultimately enhancing customer satisfaction.
Conclusion: Transforming Customer Analytics Insights with Machine Learning
Machine learning stands at the forefront of transforming customer analytics insights, enabling businesses to harness vast amounts of data effectively. By utilizing advanced algorithms, organizations can uncover meaningful patterns and trends within customer behavior, leading to more informed decision-making. This process not only enhances traditional analytics but also fosters a deeper understanding of customer needs and preferences.
The integration of machine learning into customer analytics provides a dynamic approach to interpreting insights. As businesses continually adapt to evolving market demands, machine learning equips them with the tools to stay ahead. Embracing this technology will ultimately empower companies to cultivate tailored strategies that enhance customer experience and drive growth.