AI Knowledge Gaps Detection with RingCentral Integration unveils a crucial approach to enhancing operational efficiency. As organizations grapple with vast data streams, identifying knowledge gaps is imperative for making informed decisions. The integration of AI capabilities with communication tools allows teams to pinpoint these gaps more effectively, facilitating a direct impact on performance dashboards.
This innovative detection process transforms data handling, ensuring that critical insights are not lost in the noise. By systematically analyzing interactions, organizations can discover repetitive concerns and missed opportunities. Thus, collaboration across departments becomes streamlined, driving a culture of continuous improvement and accountability, essential for any thriving organization. Embracing this technology paves the way for more strategic decision-making and a deeper understanding of employee and customer experiences.
Analyze qualitative data. At Scale.
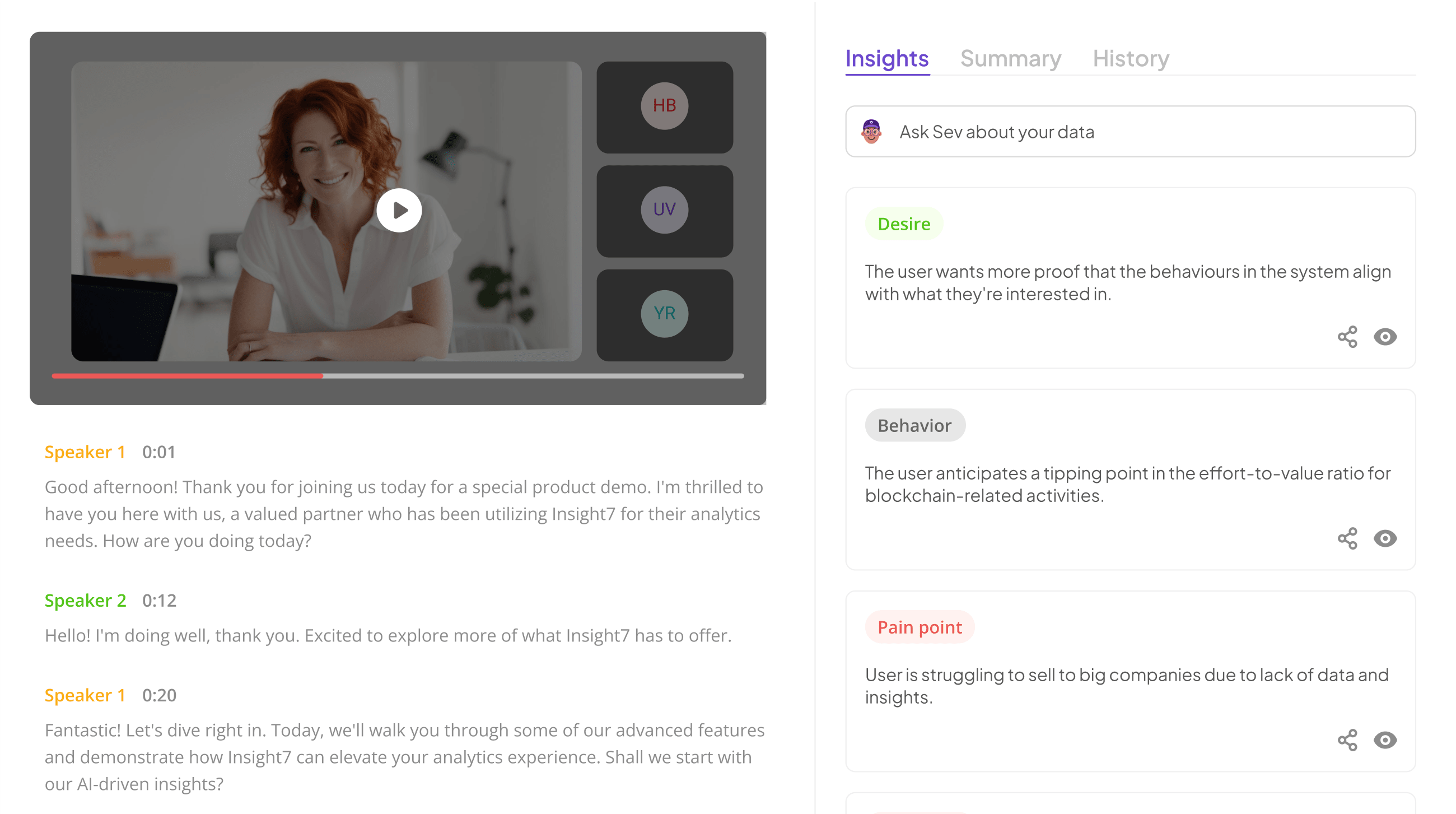
Understanding AI Knowledge Gaps in Performance Dashboards
Understanding AI Knowledge Gaps in performance dashboards is essential for organizations striving to enhance their operational efficiency. AI knowledge gaps can arise during data integration, leading to incomplete insights that hinder decision-making. Identifying these gaps is crucial as it allows organizations to recognize the limitations of their AI systems and make informed adjustments.
Key challenges include inconsistent data quality and varying degrees of knowledge among team members. Users often overlook the need for comprehensive training on how to leverage dashboards effectively. This can lead to misinterpretations of data, skewed analyses, and ultimately, less effective performance outcomes. Addressing these gaps demands a holistic approach that encompasses not only technology but also training and cultural factors within the organization. By prioritizing clear communication and ongoing support for users, companies can significantly improve their dashboard's efficacy and foster a more informed decision-making environment.
Identifying AI Knowledge Gaps in Data Integration
AI knowledge gaps can significantly impede effective data integration within organizations. Identifying these gaps is crucial for optimizing AI performance dashboards, ensuring they are informative and user-friendly. Common challenges include varying data quality, inconsistent data structures, and the misalignment between user expectations and AI capabilities. Addressing these discrepancies helps in creating a more cohesive integration process.
Organizations often overlook the subtle differences in data interpretation and presentation. The nuances in data integration can lead to miscommunication and a lack of actionable insights. Ensuring that the team understands how to recognize and bridge these knowledge gaps is vital. This understanding contributes to better customer interactions and satisfaction, ultimately empowering agents to perform at their best. By focusing on these elements, organizations can foster a productive environment that reduces knowledge gaps and promotes data-driven decision-making.
- Discuss how knowledge gaps can emerge in AI data dashboards.
Knowledge gaps in AI data dashboards can emerge from various factors that hinder the effective utilization of data. One primary cause is incomplete or inaccurate data integration, creating a disconnect between the intended insights and actual performance metrics. Insufficient training for users interfacing with these dashboards can also lead to misinterpretations, resulting in knowledge gaps that diminish the utility of the information presented.
Additionally, the rapid evolution of algorithms can create discrepancies in expected outcomes versus real-time analytics. This situation often arises when relevant data points are not consistently updated or when automated systems lack the adaptability to reflect changes promptly. Organizations must diligently address these gaps, focusing on enhancing data quality and user education to leverage AI more effectively, ultimately achieving more accurate insights and better decision-making in their performance analytics.
- Explore the common challenges faced by organizations in identifying these gaps.
Identifying AI knowledge gaps presents various challenges for organizations attempting to enhance their performance dashboards. One significant issue is the lack of data accuracy, which can hinder the detection of crucial insights. In some cases, organizations may face difficulty in understanding the data sources, leading to misinterpretations of the information presented. This complexity complicates the recognition of the knowledge gaps that exist within their AI frameworks.
Moreover, insufficient training and expertise in utilizing AI tools can exacerbate these challenges. Employees may struggle to interpret data effectively without proper guidance, resulting in a failure to address existing gaps. Additionally, organizational culture can play a pivotal role; if teams are not encouraged to communicate openly about their insights, knowledge gaps may persist unnoticed. Cultivating a transparent environment where data is regularly reviewed can help mitigate these challenges. Implementing robust tools, such as Insight7, can also facilitate the discovery and analysis of these gaps effectively.
Tools for Detecting AI Knowledge Gaps
Detecting AI knowledge gaps is essential for enhancing overall performance dashboards. By understanding where these gaps lie, organizations can evaluate their AI systems better and strategize for improvement. Effective tools play a crucial role in identifying areas where AI performance might falter, thus ensuring customer service remains top-notch and efficient.
Several tools can assist in this endeavor. Insight7 is notable for its comprehensive AI analysis capabilities. Additionally, platforms like IBM Watson and Google AI Platform offer advanced functionalities for assessing data performance. Tableau and Microsoft Power BI also provide visualization tools that can help in pinpointing deficiencies in AI knowledge. Utilizing these tools allows organizations to streamline their processes, ultimately bridging the knowledge gaps and fostering a more robust AI environment. Efficient detection enables teams to focus on delivering quality service rather than being bogged down by metrics that don't reflect true performance.
- Insight7: Highlight its capabilities in comprehensive AI analysis.
Insight7 delivers advanced capabilities for comprehensive AI analysis, serving as a powerful tool for identifying AI knowledge gaps. Through innovative data integration methods, it enables organizations to examine and visualize their performance metrics effectively. This clear visibility into operations allows users to pinpoint discrepancies and inadequacies in their data, ensuring that critical insights are not overlooked.
By employing Insight7, teams can streamline their analysis processes, making it simpler to gather actionable intelligence from vast data sets. The platform utilizes sophisticated algorithms that not only detect gaps but also suggest potential solutions for improvement. This proactive approach transforms raw data into meaningful insights, ultimately enhancing operational efficiency. As organizations continue to evolve, leveraging Insight7's analytical prowess becomes essential to maintain a competitive edge and foster informed decision-making in todayโs rapidly changing landscape.
- Other Tools: Discuss additional solutions:
In addition to comprehensive solutions like Insight7, several other tools can significantly enhance AI knowledge gaps detection. For instance, IBM Watson is well-regarded for its powerful machine learning capabilities, assisting organizations in accurately identifying knowledge deficiencies. Equally impactful is the Google AI Platform, which provides a suite of services designed to facilitate deep learning and data analysis.
Another notable contender is Tableau, renowned for its user-friendly interface that allows teams to visualize vast datasets effectively. Meanwhile, Microsoft Power BI excels in seamless integration with multiple data sources, enabling real-time insights. By utilizing these tools, organizations can better understand their AI knowledge gaps and enhance their performance dashboards. Each solution brings unique strengths, and selecting the right one will depend on specific organizational needs and integration capabilities. The goal is to provide robust support for addressing AI knowledge gaps efficiently and effectively.
- IBM Watson
IBM Watson serves as a pivotal player in the realm of AI knowledge gaps detection, particularly when integrated with performance dashboards. Its advanced algorithms and machine learning capabilities empower organizations to identify discrepancies in data and performance metrics effectively. This technology can reveal critical insights related to knowledge gaps, such as areas where staff may lack necessary training or where documentation is insufficient.
The use of IBM Watson can enhance the integrity and reliability of performance dashboards. By analyzing vast datasets, it can highlight which specific facets of service or product information are missing, thereby paving the way for targeted improvements. With its ability to process natural language, Watson also aids in understanding user queries and concerns, ensuring that knowledge is both comprehensive and accessible. Ultimately, integrating such AI capabilities is crucial for addressing knowledge gaps effectively, thus improving overall operational efficiency.
- Google AI Platform
The Google AI Platform provides robust tools that facilitate the development and deployment of machine learning models. By integrating these advanced capabilities, organizations can enhance their understanding of AI knowledge gaps within their performance dashboards. This integration empowers users to analyze vast datasets effectively, making it easier to identify discrepancies or underperforming aspects.
With intuitive dashboards and analytical features, the Google AI Platform allows teams to visualize their data and uncover insights that might otherwise remain hidden. Users can manipulate data sets, apply machine learning algorithms, and create predictive models that illuminate potential areas of improvement. Moreover, using the platform can streamline collaboration among team members, enabling them to work together seamlessly in managing and rectifying AI knowledge gaps. Ultimately, embracing the Google AI Platform can significantly elevate an organization's ability to comprehend and address its performance analytics.
- Tableau
Tableau serves as a pivotal tool in addressing AI knowledge gaps within performance dashboards. It enables organizations to visualize data seamlessly, helping to identify discrepancies in AI insights effectively. By transforming raw data into interactive visual formats, Tableau allows users to spot underlying trends and anomalies that may contribute to knowledge gaps in AI.
To fully leverage Tableau in this context, organizations should focus on three key aspects. First, understanding the integration process, ensuring that the data pipelines from various sources are coherent. Secondly, users need to utilize Tableau's advanced analytics tools, which support deeper insights into the performance metrics. Lastly, continual monitoring and feedback loops are essential for refining dashboards, allowing teams to adjust strategies and close knowledge gaps over time. By employing Tableau efficiently, organizations can enhance their AI performance dashboards and make data-driven decisions that reflect accurate knowledge assessments.
- Microsoft Power BI
Microsoft Power BI serves as a powerful tool for visualizing and analyzing data, crucial for addressing AI knowledge gaps in performance dashboards. By integrating various data sources, Power BI enables organizations to consolidate information seamlessly. This allows teams to quickly identify trends and discrepancies that may signal knowledge gaps, providing a necessary foundation for informed decision-making.
Through features like interactive dashboards and real-time reporting, users can visualize their data more effectively. This visual clarity enhances understanding and reveals insights that could be overlooked in raw data. Additionally, Power BI supports custom visualizations, allowing organizations to tailor their dashboards to highlight specific AI knowledge gaps, fostering better engagement and usage among stakeholders. In harnessing its capabilities, organizations not only identify gaps but can also formulate targeted strategies for knowledge enhancement, ultimately driving improved performance and operational efficiency.
Extract insights from interviews, calls, surveys and reviews for insights in minutes
Enhancing AI Knowledge Gaps Detection through RingCentral
Enhancing AI knowledge gaps detection is crucial for organizations seeking to optimize performance dashboards. Effective detection can significantly improve the quality and reliability of data-driven decisions. By integrating advanced communication solutions, companies can streamline workflows and enhance data accessibility directly from the source. Such improvements will help identify gaps in knowledge more rapidly and accurately.
An essential step toward achieving this enhancement involves assessing current dashboard capabilities to understand existing knowledge gaps. Once that foundation is established, configuring API integrations facilitates direct data flow, paving the way for the implementation of AI enhancement tools. Progress tracking and performance evaluations are necessary to refine the process continuously. By adopting these steps, organizations can ensure they effectively address any AI knowledge gaps and maintain a competitive edge in their industry.
Steps to Integrate RingCentral for Improved AI Performance Dashboards
To successfully integrate RingCentral for improved AI performance dashboards, start by assessing current dashboard capabilities. Evaluate the existing data sources and determine where knowledge gaps may exist. Understanding the current state will guide subsequent integration steps and enhance ability to identify AI knowledge gaps effectively.
Next, configure the RingCentral API integration. This involves setting up the necessary authentication and ensuring data flows seamlessly between your systems. Following integration, implement AI enhancement tools that can process and analyze the incoming data. These tools can significantly augment the dashboardโs ability to surface insights related to knowledge gaps.
Lastly, continuously monitor and evaluate dashboard performance. Regular assessments will help identify any remaining knowledge gaps and enable further optimization. By following these steps, organizations can create a dynamic environment that actively manages AI knowledge gaps and benefits from real-time insights.
Step 1: Assess Current Dashboard Capabilities
To effectively enhance AI knowledge gaps detection, the first step is to assess current dashboard capabilities. This assessment provides a foundation for understanding how your existing dashboards operate and what can be improved. Start by evaluating the data visualization tools in use, as well as their ability to present AI-generated insights clearly. Effective dashboards not only illustrate performance metrics; they must also highlight areas of knowledge deficiency.
Next, consider the integration method for data sources. Evaluate how well existing dashboards pull data from various platforms. This includes examining whether important data is accessible and presented accurately. Identifying current limitations in dashboard functionalities will shed light on potential knowledge gaps and inform the next steps. By laying this groundwork, organizations can ensure they develop a robust approach towards integrating AI detection tools effectively.
Step 2: Configure RingCentral API Integration
To successfully configure your API integration, begin by exploring the capabilities offered by the platform. This initial exploration enables you to identify essential features needed for effective monitoring of AI Knowledge Gaps in performance dashboards. Familiarizing yourself with the API documentation is crucial, as it provides insights on available endpoints and data structures. Understanding these parameters is vital for seamless integration and streamlining data flow.
Next, proceed with the technical setup. This includes generating an API key and setting permissions that grant your integration the necessary access to the desired data. Ensure you thoroughly test these configurations using a controlled environment to identify any potential issues. By carefully configuring the API, you empower your data dashboards to efficiently detect and address AI Knowledge Gaps, ultimately contributing to enhanced decision-making and performance evaluation. The success of this integration is the foundation for achieving broader strategic insights and fostering continuous improvement.
Step 3: Implement AI Enhancement Tools
Implementing AI enhancement tools marks a critical step in addressing AI knowledge gaps within performance dashboards. This phase focuses on integrating advanced technologies to optimize data analysis and improve user interactions. Key considerations include assessing which tools will align best with your organization's unique needs and capabilities.
Firstly, explore tools specifically designed for knowledge gaps detection. Utilize platforms like Insight7, which excel in analyzing comprehensive data, to identify where improvements are needed. Next, consider additional tools like IBM Watson and Google AI Platform, which can bring further insights into performance metrics. These enhancements not only facilitate better data interpretation but also provide a more holistic view of team performance.
In conclusion, the successful integration of these AI enhancement tools paves the way for long-term benefits, addressing knowledge gaps effectively. By adopting a strategic approach in this step, organizations can remain agile and responsive to ever-evolving performance demands.
Step 4: Monitor and Evaluate Dashboard Performance
Monitoring and evaluating dashboard performance is crucial to ensuring that AI knowledge gaps are identified and addressed effectively. This process involves tracking various performance metrics that reflect the efficacy of your AI-driven dashboard. Regularly reviewing these metrics provides insights into how well the dashboard is meeting organizational goals and highlights areas for improvement.
Begin by analyzing key performance indicators (KPIs) such as user engagement, response times, and accuracy of data interpretation. These measurements can help ascertain whether the dashboard is delivering reliable insights. Additionally, feedback from users is invaluable; it can reveal potential knowledge gaps in the AI algorithms, prompting necessary adjustments. By maintaining a robust monitoring system, organizations create a framework that not only identifies existing gaps but also anticipates future needs, optimizing the overall user experience and enhancing strategic decision-making.
Case Studies: Successful AI Knowledge Gaps Resolution with RingCentral
In examining successful case studies focused on AI Knowledge Gaps, it becomes clear that organizations have leveraged innovative solutions to enhance their performance dashboards. Through strategic integrations, many have effectively bridged the prevalent knowledge gaps that hamper operational efficiency. By emphasizing a systematic approach to identify these gaps, organizations can employ targeted strategies that yield impressive results.
The success of AI integration often hinges on a few key factors. First, thorough assessments of existing dashboard capabilities can reveal areas needing improvement. Secondly, the configuration of API integrations is vital for seamless data flow. Furthermore, the implementation of enhanced AI tools ensures that insights derived from data are actionable and relevant. Finally, consistent monitoring and evaluation help maintain a high standard of data accuracy and relevance, establishing a robust framework for ongoing success. This continual cycle of improvement not only addresses existing gaps but also anticipates future needs, setting a precedent for knowledge management excellence.
- Present case studies where RingCentral integration has successfully resolved AI knowledge gaps in dashboards.
Case studies illustrate the capacity of RingCentral integration to effectively address AI knowledge gaps in dashboards. One prominent example involved a company that struggled to identify patterns in customer queries, leading to inconsistent service levels. By integrating RingCentral's API with their existing dashboard, the organization was able to pull real-time data analytics. This helped identify specific knowledge gaps, allowing the customer service team to enhance their training programs and refine their response strategies.
Another case highlighted the ability of RingCentral integration to enhance agent performance feedback through AI-driven insights. The organization implemented tools to analyze call data and detect knowledge deficiencies among agents. As a result, they tailored their training initiatives to close these gaps, leading to improved agent confidence and elevated customer satisfaction scores. Such integrations demonstrate that proactive measures can significantly mitigate AI knowledge gaps, ultimately driving organizational excellence and customer loyalty.
- Analyze the key factors contributing to the success of these integrations.
Successful integrations of AI Knowledge Gaps detection within performance dashboards hinge on several key factors. Firstly, robust data accuracy plays a vital role. By ensuring that data provided from various sources is accurate and reliable, organizations can more effectively identify gaps that impact performance. Additionally, a user-friendly interface can significantly enhance user engagement, allowing employees to interact seamlessly with the dashboards.
Clear communication and training are equally important, ensuring that staff understands how to interpret the data and leverage insights effectively. A culture of collaboration fosters an environment where employees feel empowered to contribute to knowledge sharing and improve overall performance. Finally, consistent monitoring and iterative improvements of the integration process can lead to sustained success in bridging knowledge gaps, ultimately enhancing performance outcomes. By addressing these factors, organizations can create more effective, data-driven environments that support continuous improvement.
Conclusion: Achieving Optimal AI Knowledge Gaps Management
Effective management of AI knowledge gaps is critical for enhancing performance dashboards. Organizations must recognize that filling these gaps not only boosts data reliability but also increases overall efficiency. By prioritizing the identification and remediation of knowledge gaps, businesses can achieve better decision-making outcomes and enhance team autonomy.
To reach optimal AI knowledge gaps management, leveraging the right tools and methodologies is essential. Integrating platforms can facilitate this process, ensuring that data flows seamlessly while fostering a culture of continual improvement. As organizations adapt and refine their approaches, the benefits of improved knowledge management will become increasingly evident, leading to sustained success.