In today's fast-paced world, quality assurance (QA) has evolved significantly, merging traditional methods with modern technology. The introduction of Collaborative Intelligence Evaluation offers a new lens through which to assess QA models. This evaluation paradigm seeks to combine human intuition with AI efficiency, aiming to enhance accuracy while maintaining a human touch.
As businesses increasingly rely on both human QA programs and hybrid AI-human models, understanding their respective strengths and weaknesses is crucial. This section explores how Collaborative Intelligence Evaluation provides insights that can inform strategic decisions regarding QA models, ultimately fostering improved collaboration between human experts and advanced AI systems. By analyzing these methodologies, stakeholders can better navigate the complexities of quality assurance.
Analyze & Evaluate Calls. At Scale.
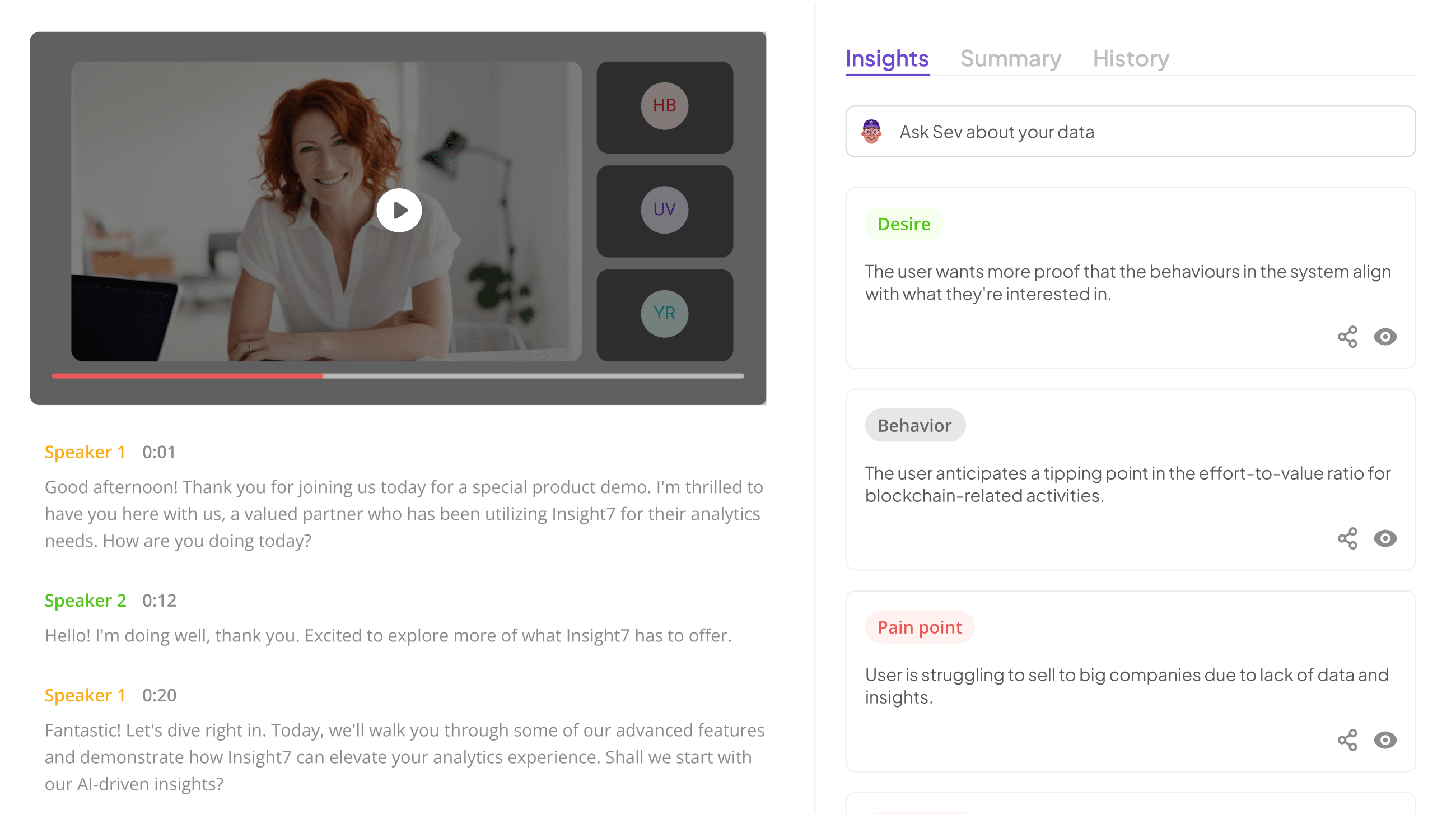
Understanding the Basics of Collaborative Intelligence Evaluation in QA
Collaborative Intelligence Evaluation in QA combines the strengths of human judgment with artificial intelligence capabilities. In this dynamic approach, quality assurance processes benefit from human nuanced understanding while leveraging AI's efficiency and speed. To grasp its essence, we must look at key components involved in this evaluation process.
Firstly, defining clear evaluation criteria is crucial. By establishing benchmarks for quality assessments, both human reviewers and AI systems can align their focus towards meaningful objectives. Secondly, data collection plays a vital role. Reliable data ensures that evaluations are grounded in factual insights rather than assumptions. Finally, employing an iterative feedback loop enhances the system. Continuous learning and adaptation allow both AI and human reviewers to improve their methodologies over time. By understanding these foundational elements, organizations can effectively integrate collaborative intelligence into their quality assurance practices.
Human QA Programs: A Traditional Approach
Human QA programs represent a traditional yet crucial approach in quality assurance, leveraging human evaluators to assess performance and compliance. These programs typically involve a structured evaluation of calls and interactions based on predefined criteria. Human evaluators are adept at nuanced assessments and can provide context-rich insights that machines often overlook.
In a traditional QA setup, evaluators focus on specific qualities, such as customer understanding and resolution effectiveness. By employing qualitative measures, these programs ensure adherence to best practices. However, the process can be resource-intensive and time-consuming, leading to potential inefficiencies in fast-paced environments. Therefore, while human QA programs excel in providing detailed evaluations, integrating technology through hybrid AI models could enhance efficiency and support human judgement. Balancing both methods presents an interesting path forward for optimizing quality assurance practices.
Hybrid AI + Human Models: A Modern Synergy
Hybrid AI + Human Models create an innovative blend of technology and human insight, revolutionizing how organizations approach quality assurance. This synergy allows for more nuanced evaluations, merging the analytical strength of artificial intelligence with the contextual understanding of humans. Such a partnership can dynamically enhance decision-making processes and foster better communication across teams.
Incorporating Hybrid AI + Human Models into quality assurance practices presents several advantages. First, it streamlines data analysis, providing faster insights from customer conversations. Second, it paves the way for a more responsive understanding of customer needs through improved real-time feedback mechanisms. Lastly, this approach enables organizations to transform scattered information into actionable strategies, ultimately enhancing competitiveness. Collaborative Intelligence Evaluation thrives in this environment, as the combination of AI and human intuition leads to richer, more reliable insights, empowering businesses to stay ahead in an increasingly complex marketplace.
Extract insights from interviews, calls, surveys and reviews for insights in minutes
Collaborative Intelligence Evaluation: Comparing Pros & Cons
In the realm of quality assurance, the Collaborative Intelligence Evaluation is pivotal for assessing different models effectively. This evaluation aims to weigh the benefits and downsides of traditional Human QA Programs against the innovative Hybrid AI + Human Models. Each approach presents unique strengths and weaknesses that significantly impact performance, efficiency, and user experience. Understanding these factors is essential for organizations seeking to improve their quality assurance practices.
One critical aspect of this evaluation is recognizing the pros and cons associated with each model. Human QA Programs rely on the expertise and intuition of human evaluators, providing a nuanced understanding of quality standards. However, they may lack scalability and can be prone to bias. Conversely, Hybrid AI + Human Models bring together the speed and consistency of AI with human judgment, offering a balanced approach. Nevertheless, over-reliance on AI can lead to challenges, including reduced human oversight and potential neglect of emotional intelligence in evaluations.
Pros of Human QA Programs in Collaborative Intelligence Evaluation
Human QA programs play a crucial role in Collaborative Intelligence Evaluation, providing numerous advantages that enhance quality assurance efforts. First, human evaluators offer nuanced understanding and context that machines may overlook. Their ability to interpret subtleties in communication fosters more accurate evaluations, especially in complex scenarios where emotionally charged or intricate dialogues are involved.
Moreover, human QA specialists can proactively identify patterns and trends in evaluations, leading to continuous improvement. Their knowledge and insights allow organizations to refine their standards and practices effectively. In cases where compliance and regulatory adherence are vital, the experience of human evaluators can significantly mitigate risks. By integrating human oversight into the evaluation process, organizations ensure that the output of their systems maintains a high standard of quality, which builds trust and confidence among users. This human touch in Collaborative Intelligence Evaluation is essential for creating a robust, reliable, and effective quality assurance program.
Pros of Hybrid AI + Human Models in Collaborative Intelligence Evaluation
Hybrid AI + Human Models in Collaborative Intelligence Evaluation offer unique advantages that enhance the quality and accuracy of assessments. First, these models combine the strengths of both AI's analytical power and human intuition. This synergy allows for more nuanced evaluations that may overlook solely AI-driven approaches. By integrating human oversight, organizations can refine AI outputs, minimizing errors and enhancing overall reliability.
Moreover, hybrid models create opportunities for continual learning. As humans provide feedback on AI assessments, the system adapts and improves over time. This dynamic collaboration prompts a cycle of innovation and learning that enhances decision-making processes. Lastly, fostering a collaborative environment encourages diverse perspectives, which is crucial for accurate insights and effective solutions. This multifaceted approach not only boosts the robustness of evaluations but also builds trust among stakeholders, ensuring a more comprehensive understanding of the evaluated data.
Cons of Human QA Programs and AI Integration Challenges
Human QA programs possess inherent limitations, impacting their effectiveness in quality assurance. One major concern is the potential for human error, which can lead to inconsistent evaluations and skewed results. Additionally, such programs require significant time and resources to be effective, often resulting in slower turnaround times. These challenges hinder the agility needed in fast-paced environments, prompting organizations to explore alternative solutions.
Integrating AI into human QA models presents another layer of complexity. The challenge lies in ensuring effective collaboration between AI systems and human evaluators. Differences in interpretation and analysis can create friction that impairs overall efficacy. Furthermore, adapting current QA workflows to incorporate AI tools frequently necessitates extensive training and can deter personnel from embracing the new technology. Therefore, organizations must navigate these integration challenges to achieve a successful balance between human insights and AI capabilities in their collaborative intelligence evaluation efforts.
Cons of Over-reliance on Hybrid AI Models
Over-reliance on hybrid AI models can lead to significant drawbacks that impact the quality of Collaborative Intelligence Evaluation. One of the main concerns is the potential for reduced human oversight. As AI systems manage more tasks, the role of human evaluators diminishes, which can stifle valuable insights from experienced professionals who understand nuances and context. This dependency may result in a narrow focus, where the AI's analysis overlooks critical elements that only human intuition can capture.
Another critical issue is the risk of entrenching biases within AI algorithms. When these models are constantly in use, they can perpetuate biases present in the training data. Consequently, the insights generated may be skewed, affecting decision-making processes adversely. Organizations must maintain a balance between AI and human involvement to ensure accurate evaluations. Fostering a collaborative environment, where both AI and human experts contribute equally, can mitigate these risks and enhance overall effectiveness in quality assessment.
Conclusion: The Future of Collaborative Intelligence Evaluation in QA
The future of Collaborative Intelligence Evaluation in Quality Assurance promises to enhance assessment accuracy and streamline processes. Both human QA programs and hybrid AI models offer unique benefits that, when combined, could lead to more effective evaluation practices. By recognizing individual strengths, organizations can create tailored strategies that leverage human intuition alongside AI efficiency.
Moreover, as technology evolves, ethical considerations and user trust will become increasingly important. The successful integration of human and AI capabilities will depend on transparency and accountability in evaluation criteria. Ultimately, fostering collaboration between human insight and AI analysis can shape a more robust and reliable quality assurance framework, ensuring high standards and continuous improvement across industries.
Analyze & Evaluate Calls. At Scale.
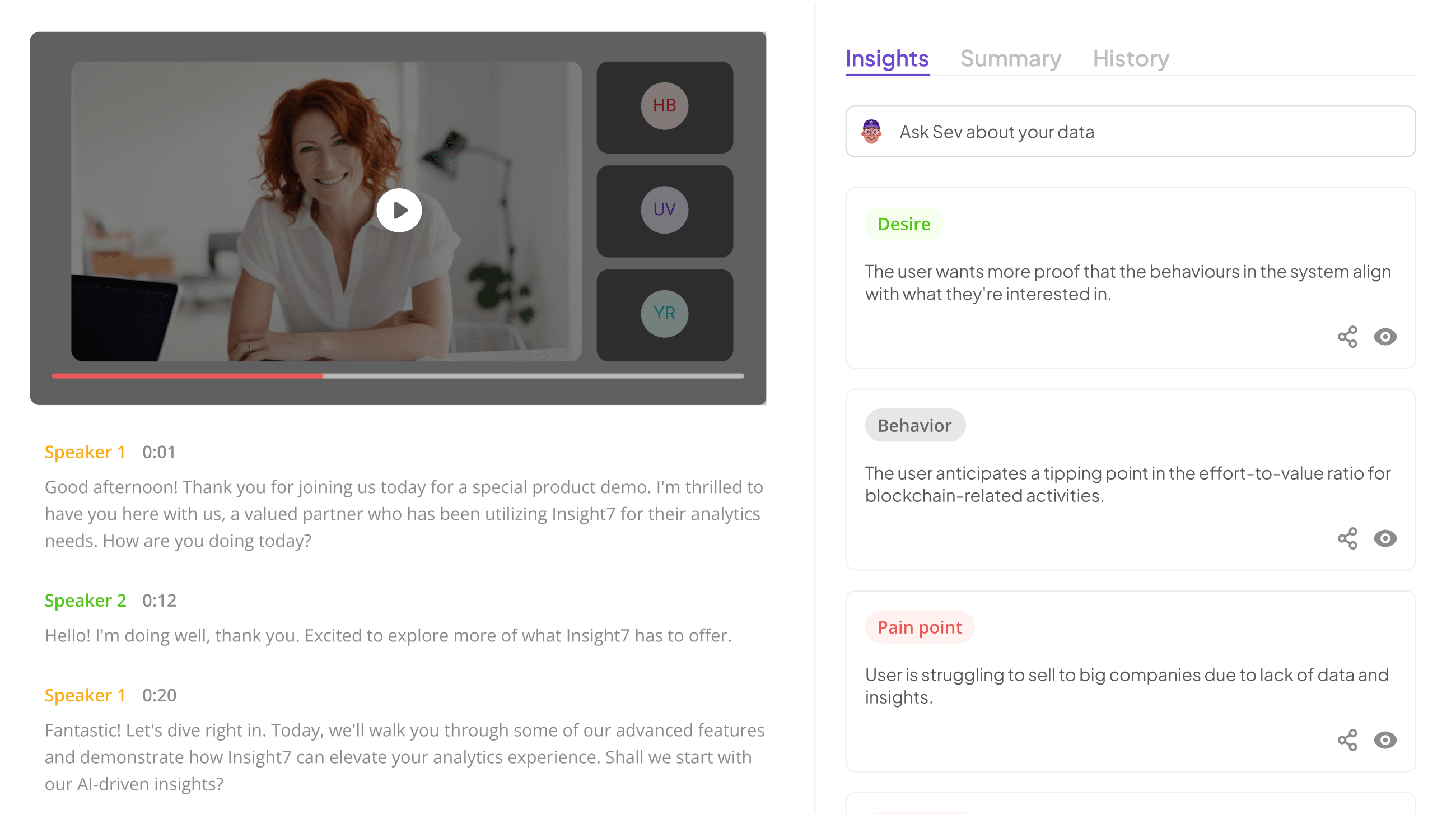