AI Pattern Detection in interview responses plays a crucial role in enhancing qualitative research by streamlining data analysis. As organizations face the challenge of extracting meaningful insights from countless interviews, utilizing AI can make this process more efficient and reliable. Manual analysis often leads to inconsistencies and biases, causing delays in reporting. By employing AI tools, researchers can uncover patterns, themes, and sentiments quickly, allowing for timely decision-making.
Incorporating AI not only accelerates the analysis but also improves the accuracy of insights derived from open-ended responses. As AI technology evolves, researchers can leverage advanced algorithms to systematically identify trends across various interviews. This capability transforms qualitative research, empowering teams to focus on strategic insights rather than getting bogged down by tedious data work. Embracing AI for pattern detection ultimately leads to richer, more actionable findings in interviews.
Analyze qualitative data. At Scale.
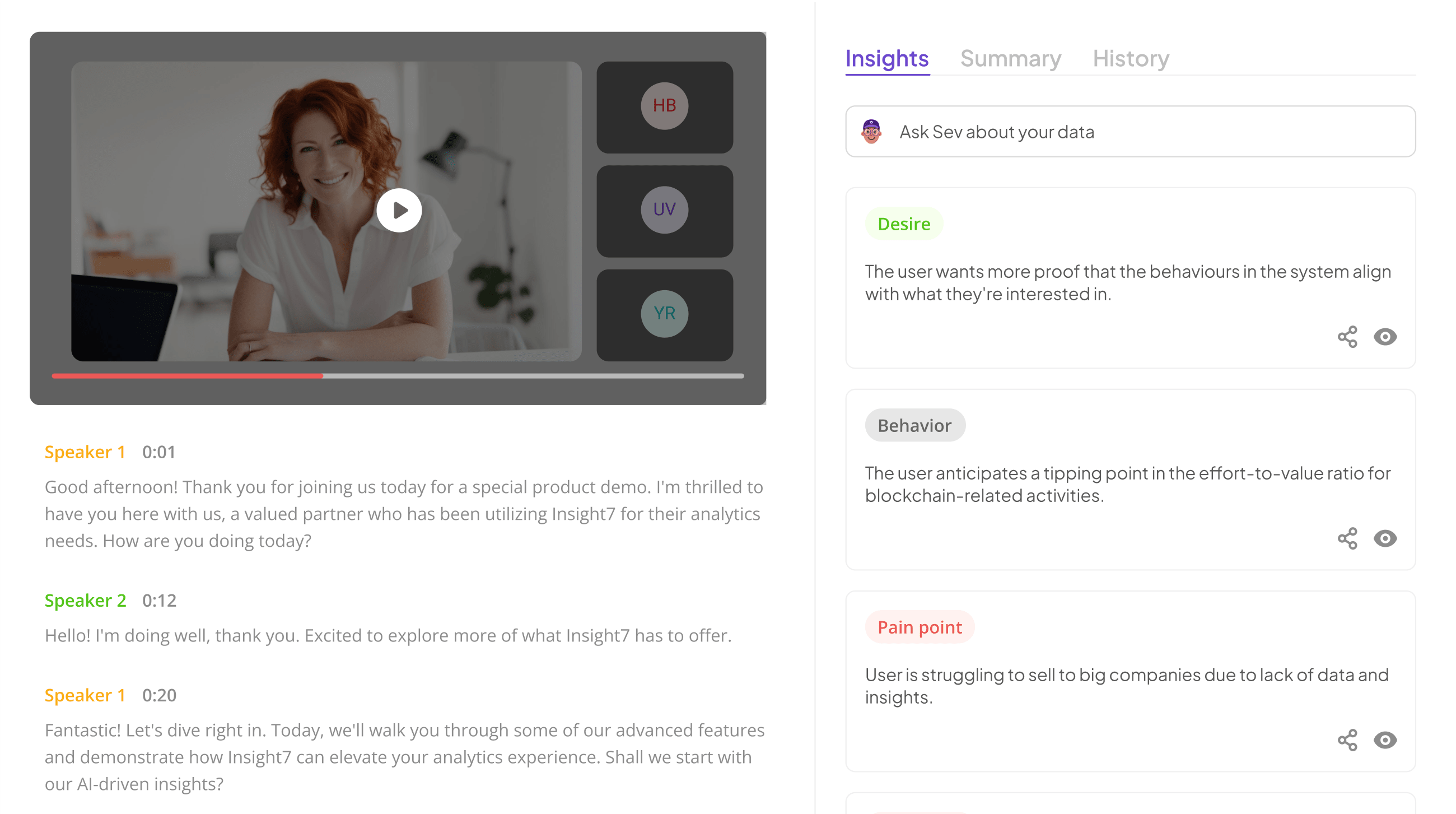
Understanding AI Pattern Detection
AI Pattern Detection is a crucial method for extracting insights from open-ended interview responses. This technique utilizes algorithms to identify recurring themes and trends within text, allowing researchers to grasp underlying sentiments and preferences expressed by participants. By employing AI tools, one can efficiently process large volumes of qualitative data, transforming raw responses into structured, actionable insights.
Understanding how AI Pattern Detection operates involves recognizing its ability to analyze language and context. The technology goes beyond simple keyword searches, using natural language processing to understand nuances in conversations. As a result, it can uncover insights that may not be immediately obvious, guiding decision-making and strategy development in various fields. By harnessing AI Pattern Detection properly, you can enrich the analysis of interview responses, ultimately leading to more informed conclusions and enhanced strategies based on user feedback.
What is AI Pattern Detection?
AI Pattern Detection involves the use of artificial intelligence technologies to identify trends and common themes within large sets of data, particularly in texts such as open-ended interview responses. This technique is essential for transforming qualitative data into quantifiable insights, making it easier to discern underlying narratives and patterns. By processing vast amounts of text, AI can uncover relationships and sentiments that might not be immediately apparent to human analysts.
This approach begins with data collection, where interviews provide valuable insights. Once collected, advanced algorithms apply natural language processing techniques to analyze the content. These algorithms identify recurring phrases, sentiments, and topics, leading to a comprehensive overview of participant perspectives. Understanding AI Pattern Detection not only enhances the analysis but also informs strategic decisions based on the insights gained from interview responses.
Importance of AI Pattern Detection in Interviews
AI pattern detection is crucial for enhancing the analysis of open-ended interview responses. It allows researchers to sift through vast amounts of qualitative data efficiently, identifying recurring themes and insights that manual analysis might overlook. This capability is especially significant as qualitative data can be rich and complex, often embedding valuable information within nuanced expressions.
Implementing AI pattern detection empowers interviewers to draw more accurate conclusions. By utilizing AI, organizations can minimize biases that typically arise during human-led analysis. This fosters a more consistent and reliable understanding of participants’ viewpoints. Most importantly, AI technologies can significantly reduce the time spent on data analysis, enabling quicker turnaround times for reporting insights to stakeholders. By recognizing patterns effectively, organizations can make informed decisions and strategize based on real data, leading to an outcomes-driven approach in their operations.
Extract insights from interviews, calls, surveys
and reviews for insights in minutes
Extract insights from interviews, calls, surveys and reviews for insights in minutes
Extract insights from interviews, calls, surveys and reviews for insights in minutes
Steps to Implement AI Pattern Detection in Open-Ended Responses
Implementing AI pattern detection in open-ended responses requires a structured approach to transform raw data into insightful analysis. First, data preparation and cleaning are critical, as the quality of input data significantly influences AI outcomes. This involves removing inconsistencies, duplicates, and irrelevant information to ensure that only meaningful responses are analyzed.
Next, selecting the appropriate AI model is vital based on the specific goals of the analysis. Different models can be evaluated to determine which aligns best with the pattern detection requirements. The process continues with training and fine-tuning the chosen AI model on relevant datasets. This step often involves iterating on model parameters to enhance accuracy and relevance. Finally, analyzing and interpreting the AI-generated results leads to actionable insights that can guide decision-making and further improve the understanding of open-ended responses. By following these steps, organizations can effectively harness AI pattern detection to streamline their interview analysis processes.
Step 1: Data Preparation and Cleaning
Data preparation and cleaning form the foundation for effective AI pattern detection in open-ended interview responses. This step involves collecting all relevant data, such as transcripts from audio and video interviews, and organizing it into a manageable format. Begin by ensuring that the data is comprehensive and accurately reflects the interview content. Import transcripts into a centralized project, allowing for easier access and manipulation throughout the analysis process.
Once your data is organized, it’s essential to clean it for inconsistencies and errors. This includes removing irrelevant information, correcting transcription mistakes, and standardizing text formats. Consider identifying and tagging key themes or terms, as these will aid in the subsequent AI analysis. Properly prepared and cleaned data boosts the accuracy of AI pattern detection, leading to more reliable insights from your interviews.
Step 2: Choosing the Right AI Model
When it comes to AI pattern detection, selecting the appropriate AI model is a critical step. Each model employs different algorithms and capabilities, which can significantly impact the quality of analysis in open-ended interview responses. First, you need to consider the specific requirements and goals of your analysis. For instance, if your focus is on extracting themes and sentiments, natural language processing models might be the most suitable choice. They are specifically designed to understand human language nuances.
Next, evaluate the scalability and flexibility of the model. Some models are better suited for larger datasets and complex queries, while others may excel in processing smaller samples with more detailed insights. It’s essential to conduct preliminary tests, experimenting with different models to assess their efficacy in handling your specific data set. Ultimately, the right AI model will empower you to uncover valuable patterns and insights effectively, enhancing the overall outcome of your research.
Step 3: Training and Fine-Tuning AI Models
Training and fine-tuning AI models is a crucial part of implementing AI pattern detection in open-ended interview responses. This step involves adapting the chosen AI model to better understand the nuances of the data it will process. In this phase, you customize the model to recognize specific keywords, sentiment, and themes relevant to your research objectives.
First, you should begin with a robust dataset, ideally comprised of previous interview responses. This dataset helps the AI model learn from actual examples. Next, adjust hyperparameters to optimize the model’s performance for your specific task. Finally, conduct iterative testing and validation, refining the model based on its accuracy and insights. This process enhances the model’s ability to effectively discern patterns, ultimately leading to actionable outcomes that drive better decision-making.
Step 4: Analyzing and Interpreting Results
Analyzing and interpreting results from open-ended interview responses is a vital part of AI pattern detection. This process allows researchers to derive meaningful insights from qualitative data, guiding decision-making and strategy development. The first step involves utilizing AI tools to identify and categorize recurring themes within the data. This categorization may include aspects such as challenges, desires, or specific behaviors of participants, enabling deeper understanding.
After organizing the data into themes, researchers should focus on sentiment analysis. This analysis reveals the emotional tone linked to various themes, helping to distinguish between positive and negative sentiments toward specific topics. By extracting quotes and evidence from the transcripts, analysts can provide a narrative that supports their findings. Ultimately, the goal of this step is not only to summarize the data but also to guide actionable insights that can inform future initiatives and improve user experiences.
Tools for AI Pattern Detection
Utilizing advanced tools for AI pattern detection enhances the analysis of open-ended interview responses significantly. These tools facilitate easier data handling and insightful reporting. Familiar options include AI platforms like IBM Watson Natural Language Understanding and Google Cloud Natural Language API, which utilize machine learning algorithms to identify themes and sentiments within qualitative data.
When selecting a tool, consider its capability to process large datasets, integrate with existing technologies, and provide comprehensive insights rapidly. For instance, platforms like MonkeyLearn and Lexalytics offer specific functionalities in text analysis that can streamline the identification of recurring themes. The right tool will not only enhance efficiency but also ensure the accuracy of data interpretations, leading to more actionable insights for decision-making and strategy formulation in research efforts.
insight7
To effectively utilize AI Pattern Detection, it is essential to first understand the nuances of open-ended interview responses. These responses can be rich in qualitative data but often contain complexities that manual analysis may overlook. AI can swiftly sift through vast amounts of text, identifying recurring themes and sentiments that emerge from diverse perspectives. This streamlined process allows researchers to unearth insights that inform decision-making.
The next step is selecting the appropriate AI tools for pattern detection. Various platforms, such as IBM Watson and Google Cloud Natural Language API, offer robust capabilities to analyze textual data. After establishing a solid foundation, researchers should focus on training the AI models adequately to enhance accuracy. Finally, interpreting the results requires a nuanced understanding of the context, ensuring that findings are relevant and actionable. By following these key steps, practitioners can harness AI Pattern Detection to transform raw qualitative insights into meaningful data-driven narratives.
IBM Watson Natural Language Understanding
IBM Watson Natural Language Understanding provides effective tools for analyzing open-ended interview responses. This technology employs advanced natural language processing to extract emotions, sentiment, and themes from unstructured text. As organizations increasingly rely on AI, this kind of pattern detection becomes invaluable for understanding customer perspectives and improving decision-making processes.
The platform automatically categorizes responses, identifying key trends and sentiments that can direct future strategies. By processing large volumes of text efficiently, it reveals insights that would otherwise be overlooked. Beyond traditional analysis, utilizing this technology allows for deeper exploration into what customers feel and think. Consequently, its applications extend across various sectors, aiding organizations in extracting actionable insights from interviews, surveys, and feedback efficiently. Embracing such AI capabilities not only enhances outcomes but also simplifies the analytical process, leading to more informed choices and better engagement with audiences.
Google Cloud Natural Language API
The Google Cloud Natural Language API provides a robust framework for understanding the nuances in open-ended interview responses. This technology excels at extracting meaningful patterns and themes from large volumes of text, enabling organizations to gain critical insights. By utilizing natural language processing, the API analyzes sentiments, entity recognition, and syntax, effectively summarizing the data in an easily digestible format.
When implementing AI pattern detection, the Google Cloud Natural Language API can significantly enhance your ability to interpret qualitative data. It offers capabilities such as sentiment analysis, which helps gauge the emotional tone of responses, and entity extraction, which identifies key concepts and topics within the text. This allows researchers to focus on trends and themes that may not be immediately evident, ultimately fostering a deeper understanding of participant perspectives and experiences. With such powerful tools at your disposal, harnessing AI for pattern detection becomes an invaluable asset in analyzing interview narratives.
MonkeyLearn
MonkeyLearn provides a user-friendly platform for AI pattern detection, particularly in the realm of open-ended interview responses. This tool enables users to effortlessly input and analyze data, such as interview transcripts, making it accessible to both seasoned researchers and newcomers alike. Users can quickly extract insights, summarize content, and identify key themes directly from their interview responses, which helps to streamline the analysis process.
One of the standout features of this platform is its collaborative approach. Users can import files from their personal library or their team members’, fostering a team-oriented environment. Furthermore, the platform offers pre-built analysis kits that accelerate the insight extraction process. These kits are customizable and specifically designed for various research needs, allowing for tailored insights that align with project objectives. In summary, MonkeyLearn is a powerful tool for those looking to enhance their AI pattern detection capabilities in evaluating open-ended interviews.
Lexalytics
In the context of AI pattern detection, a robust tool can significantly enhance your ability to extract meaningful insights from open-ended interview responses. This specific solution focuses on text analysis, enabling you to transcribe and examine conversations efficiently. When you upload audio or video files, the tool transcribes them seamlessly, allowing you to handle multiple files in a single project. This makes it easy to distill information from your qualitative data into actionable insights.
Once transcribed, you gain the ability to analyze individual responses or aggregate data from entire projects. By extracting themes, quotes, and key insights, you can ask targeted questions that drive further investigation. Additionally, this platform ensures data privacy through strict compliance protocols. As you navigate your projects, you will appreciate the user-friendly interface, making it straightforward to access and utilize your data effectively. Overall, this tool streamlines the process of AI pattern detection, enabling richer analysis of open-ended interview content.
Conclusion on AI Pattern Detection for Interviews
AI pattern detection serves as a pivotal tool in analyzing open-ended interview responses. By harnessing advanced algorithms, it reveals underlying trends and common themes within qualitative data. This process enhances understanding among researchers and stakeholders, enabling informed decision-making based on actionable insights.
As organizations continue to conduct interviews, the need for efficient analysis becomes apparent. AI pattern detection not only streamlines the evaluation process but also minimizes biases that can influence traditional methods. Ultimately, embracing this technology empowers teams to extract meaningful insights swiftly, fostering greater alignment with their strategic goals.
Analyze qualitative data. At Scale.
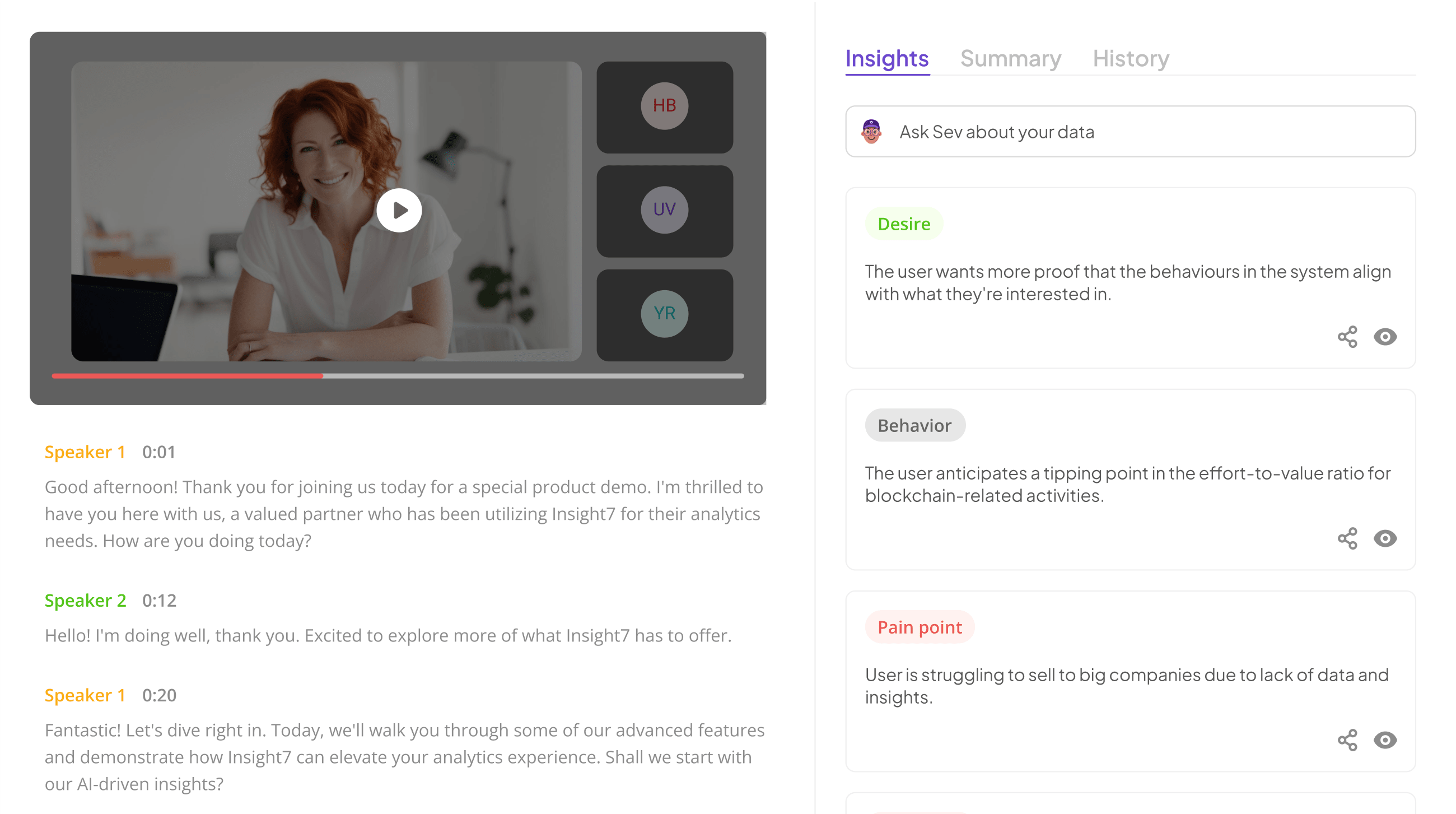