Churn risk forecasting is an essential tool for businesses aiming to maintain a loyal customer base. Understanding which customers are likely to disengage can significantly influence a company’s strategies and future growth. As markets become increasingly competitive, proactive measures driven by accurate forecasting become not just beneficial, but necessary for sustainability.
By identifying patterns and key indicators that signal potential churn, organizations can implement targeted interventions. This section delves into the fundamentals of churn risk forecasting, highlighting its importance in predicting customer behavior and mitigating turnover. Fostering a data-driven approach allows businesses to enhance customer satisfaction and loyalty, ultimately leading to improved financial outcomes.
Analyze qualitative data. At Scale.
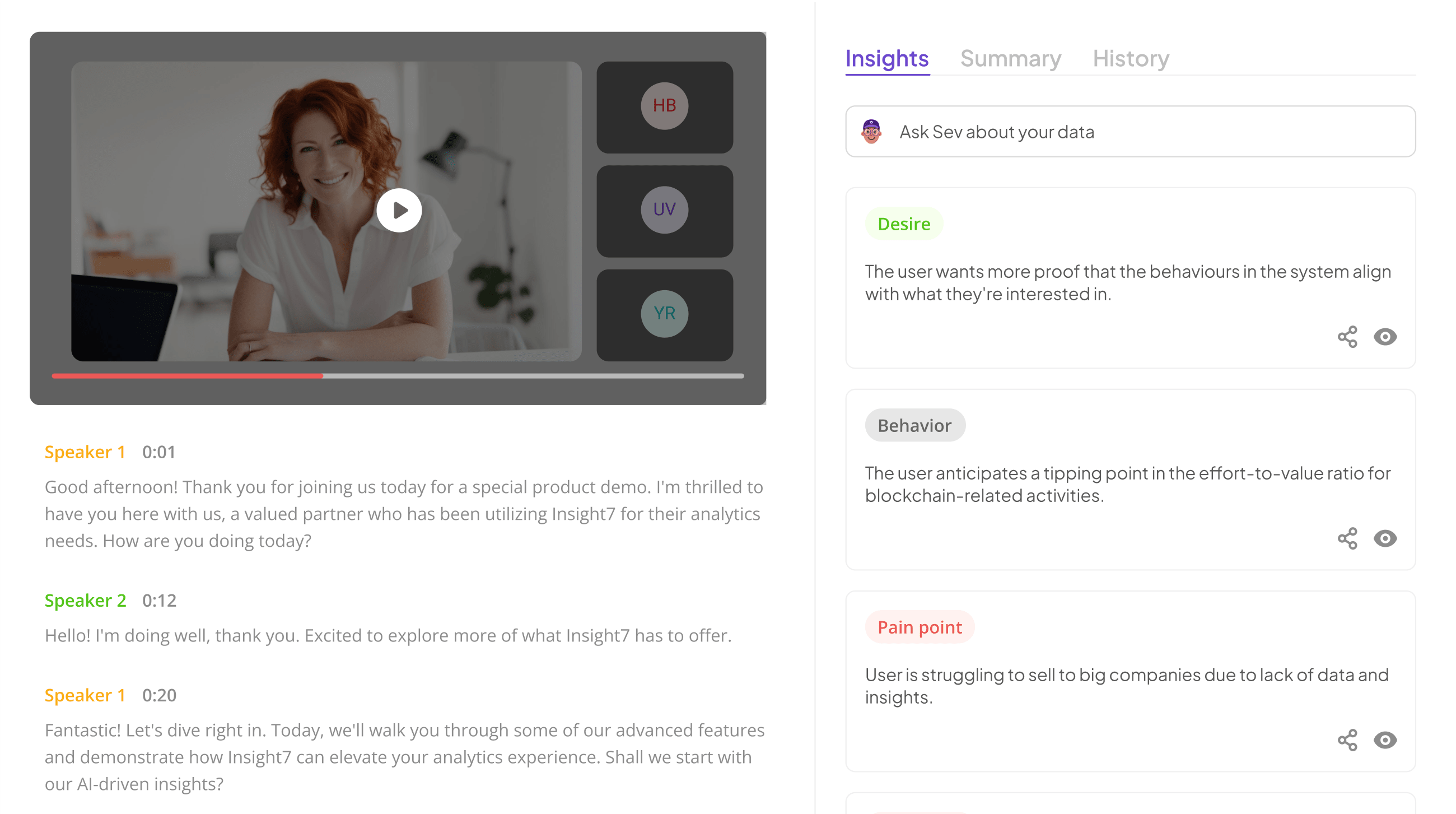
Understanding the Importance of Churn Risk Forecasting
Churn Risk Forecasting is essential for businesses aiming to maintain revenue stability and foster growth in a competitive market. Understanding churn—when customers discontinue their relationship with a business—highlights the potential negative impact on profitability and brand loyalty. By proactively identifying churn risk, companies can implement strategies that not only retain customers but also promote an environment of continual improvement and engagement.
Forecasting churn risk equips businesses with crucial insights into customer behavior, enabling them to address concerns before they escalate into permanent losses. This involves recognizing patterns in customer interactions and feedback, allowing businesses to tailor their services more effectively. Ultimately, embracing churn risk forecasting can lead to improved customer satisfaction, lower turnover rates, and higher overall profitability through informed decision-making and targeted strategies.
The Impact of Churn on Businesses
Churn refers to the loss of customers who discontinue service or product use, significantly affecting businesses. This loss not only leads to direct revenue decline but also impacts brand reputation and future growth potential. The consequences of churn extend beyond simply losing sales; they can disrupt market share and weaken customer trust. Organizations that do not effectively address churn risk may find themselves struggling to keep pace with competitors.
Understanding the broader implications of churn is vital for strategic business planning. Companies investing in churn risk forecasting can better anticipate customer attrition, which allows them to implement targeted retention strategies. By focusing on customer satisfaction and engagement, businesses can mitigate churn’s adverse impact, fostering sustainable growth and stability. Prioritizing churn risk assessment and employing analytical tools to forecast customer behaviors can ultimately lead to stronger financial performance and improved customer relationships.
- Explanation of churn and its negative effects on revenue and growth.
Churn is the rate at which customers disengage from a service, and it can significantly jeopardize a company's revenue and growth. High churn rates indicate dissatisfaction or unmet expectations, leading to lost revenue that could otherwise be reinvested in product development and customer service. Understanding churn is essential, as it serves as a red flag that signals potential vulnerabilities in customer loyalty.
When churn goes unchecked, businesses face not only immediate financial losses but also hindered long-term growth. Each customer departure damages the brand and impacts the overall market share. Moreover, acquiring new customers typically costs more than retaining existing ones. Effective churn risk forecasting can help identify potential churners before they leave, allowing companies to implement retention strategies that enhance both revenue and customer satisfaction. Effective measurement and analysis can lead to robust solutions, reinforcing business stability.
Understanding the Importance of Churn Risk Forecasting
Churn risk forecasting plays a vital role in understanding customer retention and longevity. By accurately predicting churn, businesses can implement targeted strategies to retain at-risk customers. Churn doesn’t just affect revenue; it can hinder overall business growth and impact brand reputation. This makes it essential to address churn effectively.
Forecasting allows organizations to recognize patterns and identify possible churn triggers. Implementing proactive measures based on these predictions enables companies to mitigate losses and enhance customer satisfaction. Additionally, predicting churn empowers teams to customize solutions and improve service delivery. Thus, understanding and implementing churn risk forecasting can significantly impact an organization's long-term success and stability in a competitive landscape.
How Forecasting Helps Mitigate Churn
Forecasting plays a pivotal role in mitigating churn by enabling organizations to identify at-risk customers early on. By implementing churn risk forecasting, businesses can analyze behavioral patterns and customer interactions, allowing them to proactively address concerns before they result in cancellations. This foresight not only preserves the existing customer base but also strengthens the overall relationship between the business and its clientele.
Additionally, accurate forecasting equips teams with the data necessary to tailor interventions effectively. Whether through personalized offers or enhanced support, targeted strategies can be developed based on predicted churn trends. Implementing these proactive measures not only enhances customer retention but also fosters loyalty and drives sustainable growth. Embracing churn risk forecasting allows organizations to not just react but to anticipate and act thoughtfully, maintaining a thriving business environment.
- Overview of the benefits of predicting churn and proactive measures.
Predicting churn effectively offers numerous advantages to businesses aiming to enhance customer retention. By implementing churn risk forecasting, organizations can proactively identify at-risk customers. This foresight not only reduces customer attrition but also directly impacts revenue stability and growth. Understanding which customers may leave allows businesses to tailor their engagement strategies, addressing potential issues before they escalate.
Proactive measures based on these predictions—such as personalized outreach or improved support—can transform customer relationships. When customers feel valued and understood, their loyalty increases. Additionally, businesses can assess the effectiveness of their retention strategies through continuous monitoring of engagement metrics and customer feedback. This approach results in meaningful insights that foster long-term relationships, ultimately leading to stronger brand loyalty and improved financial performance. In summary, churn risk forecasting is a powerful tool for ensuring sustained business success.
Extract insights from interviews, calls, surveys and reviews for insights in minutes
Identifying QA-Backed Indicators for Churn Risk Forecasting
Identifying QA-backed indicators is crucial for effective churn risk forecasting. These indicators provide a structured approach to understanding customer behavior and pinpointing potential risks. By defining what constitutes a QA-backed indicator, businesses can focus on specific metrics that enhance their prediction efforts. Quality Assurance (QA) insights ensure that the data collected is reliable, which can significantly improve the accuracy of churn forecasts.
Several key indicators are essential for assessing churn risk. Customer feedback scores, such as Net Promoter Score (NPS) or Customer Satisfaction Score (CSAT), reveal how customers perceive your service. Analyzing interaction data can uncover patterns in customer behavior that suggest dissatisfaction. Additionally, monitoring service usage frequency and support requests can illuminate potential red flags. Together, these QA-backed indicators inform a proactive strategy to reduce churn, allowing businesses to take targeted actions to retain customers effectively.
Defining QA-Backed Indicators
QA-backed indicators represent crucial metrics that enhance the reliability of churn risk forecasting. These indicators are derived from quality assurance processes, providing insights into customer interactions and experiences. By systematically analyzing data from customer engagements, businesses can pinpoint early warning signs of churn, such as decreased satisfaction or engagement levels.
Implementing QA-backed indicators allows organizations to develop a deeper understanding of their customers' needs and pain points. Commonly utilized metrics in this context include customer feedback scores and resolution times, which directly reflect service quality. By continuously monitoring these indicators, businesses can proactively address issues, tailor their strategies, and ultimately decrease churn rates. Establishing a robust framework around these indicators transforms raw data into actionable insights, solidifying their role in effective churn risk forecasting.
- Explanation of what QA-backed indicators are.
QA-backed indicators are essential tools in churn risk forecasting, particularly when it comes to analyzing interactions between businesses and their customers. They refer to metrics derived from quality assurance processes, which evaluate customer engagements for key performance factors. Essentially, these indicators help in assessing whether the service provided meets set expectations and standards, thus influencing customer satisfaction and retention.
By utilizing QA-backed indicators, businesses can improve the accuracy of churn predictions significantly. For instance, specific metrics could include the frequency of customer inquiries, overall satisfaction scores, and the resolution rate of issues. These elements provide valuable insights into potential churn risks by highlighting patterns and concerns that may lead to customer dissatisfaction. Ultimately, leveraging QA-backed indicators allows businesses to take proactive measures to retain customers and reduce churn.
- How they improve the accuracy of churn predictions.
To improve the accuracy of churn predictions, organizations focus on analyzing various qualitative and quantitative data. This process emphasizes the role of Quality Assurance (QA) in defining accurate indicators that signal potential churn. By collecting data from customer interactions, trends can emerge that highlight specific pain points and dissatisfaction levels. These insights are invaluable in developing a nuanced understanding of customer behavior, which is crucial for effective churn risk forecasting.
Moreover, integrating customer feedback systems allows for real-time analysis, enhancing the forecast's reliability. Regular assessment of customer interactions helps businesses adapt their strategies to meet changing needs. This continuous loop of feedback and analysis ensures that churn predictions remain aligned with actual customer experiences, ultimately fostering improved retention strategies. By making informed decisions based on data, companies can counteract churn before it becomes a significant financial burden.
Key Indicators for Churn Risk Assessment
To effectively assess churn risk, it's essential to identify crucial indicators that signify customer dissatisfaction or disengagement. Key indicators play a vital role in churn risk forecasting by providing insights into behaviors and trends that could lead to customer attrition. This includes analyzing customer interaction data, monitoring feedback metrics, and evaluating satisfaction scores.
Understanding these indicators enables businesses to strategically address potential issues before they escalate, allowing for proactive measures to retain valuable customers. For instance, a sudden increase in customer complaints or a decline in engagement metrics may signal impending churn. By regularly monitoring these indicators, organizations can adapt their strategies and improve customer experiences, thereby reducing the likelihood of churn and fostering long-term loyalty.
Implementing a structured approach to track and analyze these indicators is critical for an effective churn risk forecasting framework. This process not only empowers teams to make data-driven decisions but also enhances overall customer satisfaction and retention.
- Discussion of specific metrics and data points used in QA.
In the discussion surrounding metrics and data points used in QA for churn risk forecasting, specific indicators play a vital role. Firstly, customer feedback forms the foundation of understanding retention risks. High satisfaction scores correlate significantly with lower churn rates, emphasizing the need for regular survey updates. Additionally, analyzing support interaction metrics, such as call resolution times and customer engagement levels, provides insights into potential dissatisfaction triggers.
Moreover, evaluating compliance with defined quality standards can uncover recurring issues impacting customer loyalty. Metrics such as problem identification accuracy and context collection effectiveness are crucial. By utilizing these data points, businesses can proactively identify at-risk customers and tailor interventions to enhance retention. Regularly monitoring these QA-backed indicators will not only aid in predicting churn but can also foster a deeper understanding of customer needs, ultimately improving service delivery and satisfaction.
- Importance of customer feedback and satisfaction scores.
Customer feedback and satisfaction scores play a crucial role in churn risk forecasting. By understanding customer sentiments, businesses can proactively address issues and improve their services. Gathering feedback helps identify areas where customers may be dissatisfied, allowing for timely interventions that can enhance overall experience.
Customer satisfaction scores provide a quantitative measure of how well a business is meeting customer needs. High satisfaction scores often correlate with lower churn rates, signaling that customers are likely to remain loyal. Conversely, negative feedback can highlight potential churn risks that need addressing through targeted strategies, further preventing revenue loss. Thus, consistently monitoring feedback and satisfaction scores is essential for maintaining strong customer relationships and minimizing churn risk.
Steps to Implement Churn Risk Forecasting with QA-Backed Indicators
Implementing Churn Risk Forecasting using QA-backed indicators involves a series of steps that ensure accurate predictions and effective strategies. The first step is to collect and analyze data from various customer interactions. This data serves as the foundation for your forecasting model, so prioritize quality and comprehensiveness. Gathering insights from customer feedback and support interactions is vital, as they reveal key risk factors associated with churn.
Next, focus on creating a predictive model that integrates the gathered data. Utilize statistical techniques and algorithms to identify potential churn patterns. QA-backed indicators will enhance the model's accuracy, allowing for better-targeted interventions. Finally, invest in continuous monitoring and improvement. Regularly revise the model based on new data and insights gleaned from ongoing QA processes. This cycle of refinement helps to maintain the effectiveness of your churn risk forecasting system, ultimately supporting stronger customer retention strategies.
Step 1: Data Collection and Analysis
To initiate the process of churn risk forecasting, the first step involves meticulous data collection and analysis. You begin by gathering data from various customer interactions, such as feedback, call transcripts, and survey responses. This diverse data collection forms the basis for understanding customer behaviors and experiences. Compile this information in an organized manner, focusing on key metrics and pain points that are commonly expressed by your customers.
Next, delve into analyzing the collected data. Use analytical tools to highlight trends and patterns, ensuring you can identify critical insights effectively. By examining these patterns, you can begin to discern which factors significantly contribute to churn risk. This analytical phase is critical, as it sets the groundwork for predictive modeling and establishes a clear understanding of underlying customer issues. With a solid data foundation, you pave the way for more informed strategies aimed at reducing churn and enhancing customer retention.
- Gathering relevant data from customer interactions.
Gathering relevant data from customer interactions is a crucial step in churn risk forecasting. This process begins with effective communication during customer touchpoints, such as sales calls, support tickets, and feedback surveys. Engaging customers in a meaningful way not only provides valuable insights but also helps in understanding their needs and expectations. Each interaction offers a potential data point that can highlight areas of concern, allowing businesses to proactively address customer issues before they escalate.
To effectively gather this data, focus on key areas. First, capturing both qualitative and quantitative feedback during interactions can reveal underlying trends and sentiments. Second, analyzing customer engagement patterns can help identify signs of disengagement that may lead to churn. Lastly, integrating these insights into a centralized system fosters a comprehensive view of customer behavior. By doing so, companies can take informed actions based on the insights gathered, thereby increasing their chances of retaining valued customers.
Step 2: Creating a Predictive Model
Creating a predictive model for churn risk forecasting requires careful integration of quality assurance data. First, it’s vital to collate historical data from customer interactions, focusing on both positive and negative feedback. This allows you to identify trends and patterns that may indicate potential churn. Utilize various metrics like customer satisfaction scores and feedback ratings to form a solid data foundation for the model.
Once you have gathered sufficient data, employ machine learning algorithms to analyze it. These algorithms help predict future churn risks based on identified QA-backed indicators. Regularly refine the model by incorporating new data and insights, ensuring enhanced accuracy over time. By continuously monitoring these predictive outcomes, businesses can proactively address churn issues, strategizing interventions tailored to customer needs. This proactive approach ultimately strengthens customer retention strategies and overall business performance.
- How to use QA data to build an effective churn prediction model.
To build an effective churn prediction model, it is crucial to leverage Quality Assurance (QA) data. This data encompasses customer interactions, feedback, and satisfaction metrics, offering insights into potential churn risks. Begin by meticulously gathering and analyzing these data points to identify patterns that correlate with customer attrition. Understanding these trends allows businesses to make informed, proactive changes to improve customer retention.
Next, create a predictive model using the identified QA indicators. Employ statistical and machine-learning techniques to analyze the data, helping to forecast churn risk accurately. Continuous monitoring is essential, as it enables constant refinement of the model based on ongoing insights from QA data. By integrating these steps, businesses can enhance their churn risk forecasting and develop actionable strategies that lead to improved customer loyalty and reduced turnover.
Step 3: Continuous Monitoring and Improvement
Continuous monitoring and improvement are critical components of effective churn risk forecasting. After implementing your predictive model, ensure to revisit and assess its performance regularly. This ongoing evaluation helps you adapt to changes in customer behavior and business dynamics, ensuring that your forecasts remain reliable and relevant.
To enhance your insights, consider integrating the following strategies:
Regular Data Updates: Continuously refresh your data sources to capture emerging trends and patterns. This dynamic approach allows for real-time adjustments in your prediction models.
Feedback Mechanisms: Implement systems to gather customer feedback consistently. Both qualitative and quantitative insights can illuminate underlying issues contributing to churn.
Process Refinement: Analyze existing processes and adjust based on performance metrics and feedback. This iterative approach fosters a culture of improvement and responsiveness.
By consistently applying these practices, you can effectively minimize churn risk and drive improved customer retention through informed decision-making.
- The importance of refining models based on ongoing QA insights.
Refining churn risk forecasting models based on ongoing quality assurance (QA) insights is crucial for effective customer retention strategies. When businesses regularly analyze QA findings, they can identify shifts in customer sentiment and behavior. This continuous feedback loop enables organizations to adapt and enhance their predictive models, ensuring accuracy in forecasting churn risk.
Moreover, leveraging this iterative process helps in fine-tuning strategies that directly address customer concerns. For instance, QA insights may uncover specific issues that lead to dissatisfaction, allowing teams to implement targeted interventions. By regularly updating these models, companies can not only track changes in customer preferences but also preemptively address potential churn triggers. This proactive approach fosters a culture of responsiveness and agility, ultimately enhancing customer loyalty and driving business growth.
Tools for Effective Churn Risk Forecasting
Effective Churn Risk Forecasting relies on a blend of advanced tools and techniques designed to analyze customer behavior. One of the foundational aspects is selecting appropriate software solutions that can capture detailed customer data, helping businesses identify potential churn patterns. Tools like Zendesk and Salesforce provide valuable insights into customer interactions, allowing teams to monitor satisfaction levels and engagement metrics over time.
In addition to these platforms, employing advanced analytics tools can enhance decision-making processes. Qualtrics, for instance, enables organizations to gather and interpret feedback directly from users, providing critical information that informs retention strategies. Integrating such tools helps create a comprehensive view of customer sentiment, thus facilitating proactive measures to mitigate churn risk. By utilizing these technologies effectively, businesses can better anticipate churn and implement strategies to enhance customer loyalty and satisfaction.
insight7
Understanding churn risk is crucial for businesses aiming to maintain strong customer relationships and revenue streams. Insight7 emphasizes utilizing QA-backed indicators to enhance churn risk forecasting. These indicators not only refine data analysis but also make it possible to predict churn more accurately.
To implement effective churn risk forecasting, follow these essential steps:
Data Collection and Analysis: Start by gathering pertinent data from customer interactions. This includes feedback, satisfaction scores, and behavioral metrics.
Creating a Predictive Model: Use the collected QA data to construct a predictive model. This model will help identify potential churn risks early on.
Continuous Monitoring and Improvement: It is vital to consistently monitor churn indicators and refine your model based on fresh insights. As patterns emerge, you can adjust strategies accordingly.
By understanding and applying these steps, businesses can significantly mitigate churn risk and foster a more stable customer base.
- Overview of insight7 and its capabilities in churn prediction.
Insight7 is a powerful tool designed to support businesses in managing churn risk forecasting effectively. Its user-friendly interface allows anyone in the organization to access and utilize insights derived from customer interactions. This accessibility helps empower team members to analyze trends and identify key pain points within customer experiences. By streamlining the process of data analysis, businesses can better understand their customers' needs and address any issues that may lead to churn.
A unique capability of insight7 is its ability to collate and visualize data from various sources, including customer calls and feedback. The platform automatically extracts significant indicators from these interactions, providing actionable insights for churn risk forecasting. This enables organizations to not only predict potential churn but also implement proactive measures to enhance customer retention, ultimately improving overall business stability and growth.
Additional Tools for Churn Prediction
Incorporating additional tools can significantly enhance churn risk forecasting. These tools offer unique features that help identify patterns in customer behavior, enabling businesses to predict and mitigate churn more effectively. For instance, platforms like Zendesk provide comprehensive customer interaction analytics, allowing you to gauge customer satisfaction over time. Salesforce integrates CRM data seamlessly, providing insights into sales patterns and customer retention metrics. Each tool contributes to a nuanced understanding of churn risk.
Moreover, utilizing tools like Qualtrics facilitates the collection of real-time feedback and surveys, helping businesses stay ahead of potential churn triggers. Birdeye offers reputation management solutions focused on customer reviews, shedding light on sentiments that may signal risk. By integrating these tools into your churn forecasting strategy, you not only enhance data analysis but also cultivate proactive engagement with customers, lowering the overall churn rates.
- Zendesk
Utilizing advanced customer service platforms can significantly bolster churn risk forecasting. These tools allow organizations to gather vital insights from customer interactions, helping to identify potential churn indicators. Notably, platforms like Zendesk provide valuable analytics related to customer satisfaction and engagement. By systematically analyzing these metrics, businesses can pinpoint at-risk customers early and implement tailored interventions to mitigate churn.
Furthermore, data collected through comprehensive support channels enables organizations to understand customer sentiment better. Utilizing QA-backed indicators from Zendesk, such as ticket resolution times and customer feedback ratings, enhances the predictive accuracy of churn risk assessments. This approach not only improves customer experiences but also reinforces overall business stability. Ultimately, employing such tools effectively aids in recognizing patterns and behaviors linked to churn, empowering organizations to make informed decisions.
- Salesforce
Salesforce plays a pivotal role in churn risk forecasting by providing a comprehensive platform for managing customer relationships. By leveraging detailed customer data and insights, businesses can accurately identify trends that indicate potential churn. Salesforce enables organizations to track key indicators, such as customer interactions and feedback, which are essential for recognizing at-risk customers before it’s too late.
The integration of Salesforce within the churn risk forecasting framework facilitates the collection of invaluable QA-backed indicators. These indicators not only streamline data analysis but also enhance the predictive capabilities of businesses. For effective churn management, utilizing Salesforce’s data-driven insights allows decision-makers to implement proactive strategies. By ensuring that customer needs are met and expectations are managed, companies can effectively reduce their churn rates and foster long-term loyalty.
- Qualtrics
Qualtrics plays a vital role in the process of churn risk forecasting by providing robust tools for data collection and analysis. This platform allows businesses to gather insights directly from customer interactions, which can be essential for understanding customer behavior and predicting churn. By utilizing sophisticated surveys and analytics, organizations can create a clearer picture of why customers may consider leaving, thus enabling proactive engagement strategies.
Furthermore, Qualtrics enhances churn risk forecasting by integrating quality assurance indicators into the analytics framework. These indicators serve as critical data points, allowing businesses to assess customer sentiment and satisfaction effectively. By continuously monitoring these metrics, companies can adjust their strategies and responses in real time, fostering a more resilient customer relationship and ultimately reducing churn rates. This symbiotic relationship between data collection and actionable insights is paramount for any organization aiming to retain its customer base and enhance overall satisfaction.
- Birdeye
Birdeye offers a powerful platform for businesses aiming to enhance customer experience and minimize churn risk. By providing insights through customer feedback, companies can gain a comprehensive understanding of factors contributing to dissatisfaction. This proactive approach is key to developing effective churn risk forecasting strategies, allowing businesses to anticipate potential customer departures.
Utilizing QA-backed indicators is essential in this process. For instance, monitoring customer interactions and satisfaction can reveal patterns that indicate a higher likelihood of churn. By systematically analyzing these indicators, businesses can refine their approaches, make informed decisions, and initiate timely interventions. Ultimately, implementing such strategies not only improves customer retention but also fosters long-term loyalty and growth.
Conclusion: Enhancing Business Stability with Churn Risk Forecasting
Incorporating churn risk forecasting into your business strategy significantly contributes to enhancing overall stability. By anticipating customer attrition, businesses can implement targeted strategies that increase retention and boost long-term profitability. This predictive approach not only enables proactive engagement but also aids in refining customer experience, creating a more loyal client base.
Ultimately, effective churn risk forecasting builds a solid foundation for future business growth. The key lies in continuously analyzing customer feedback and QA-backed indicators, allowing organizations to adapt swiftly to changing behaviors. By doing so, businesses can maintain stability in a competitive market and foster lasting relationships that drive success.