Voice Sentiment Analysis in AI-based applications has emerged as a pivotal technology in understanding customer interactions. Imagine a world where businesses can decode not only what customers say but also how they feel about their experiences. This capability allows organizations to respond meaningfully, enhancing customer satisfaction and loyalty.
Voice Sentiment Analysis leverages advanced AI techniques to interpret emotional cues from speech patterns, tone, and pitch. By harnessing this technology, companies can pinpoint customer sentiment in real-time, leading to more informed decision-making. As we delve deeper into this transformative field, we will explore its mechanisms, benefits, and practical applications in crafting effective voice sentiment heatmaps for improved outcomes.
Analyze & Evaluate Calls. At Scale.
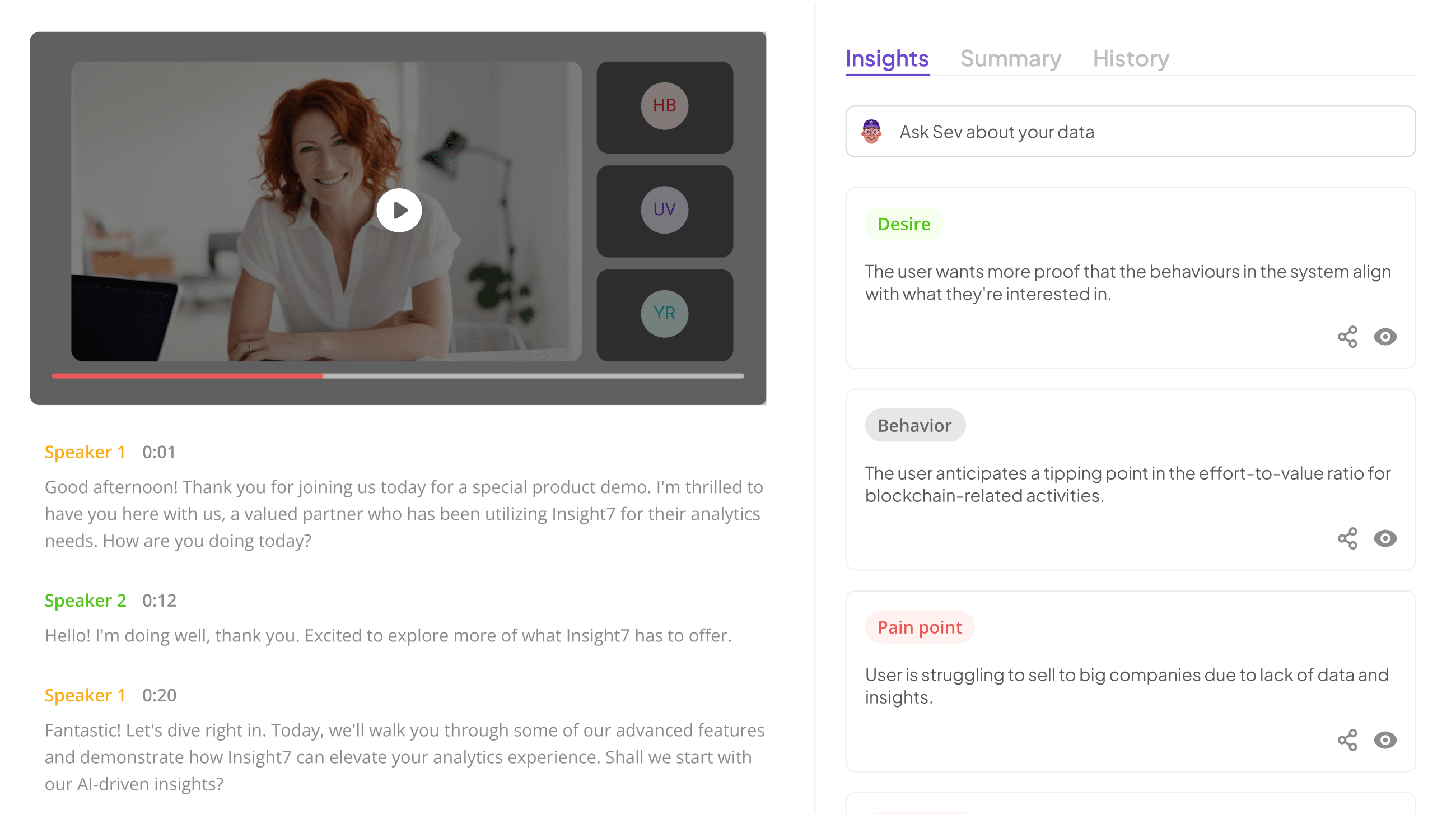
Understanding Voice Sentiment Analysis Technologies
Voice sentiment analysis technologies utilize advanced algorithms to evaluate the emotional tone behind spoken language. Harnessing machine learning and natural language processing, these systems parse vocal intonations, speech patterns, and context to discern sentiment. This approach transcends traditional text analysis by offering a nuanced understanding of human emotions in conversation.
Several factors underpin the effectiveness of voice sentiment analysis technologies. First, they leverage voice features like pitch, volume, and speed, providing key insights into customer mood. Second, these systems facilitate real-time processing, enabling instant feedback that can significantly enhance customer interactions. Finally, their scalability allows businesses to analyze large volumes of data quickly, making them ideal for organizations looking to glean actionable insights from customer interactions. Embracing these technologies can transform how companies engage with their audiences and address customer needs effectively.
How Voice Sentiment Analysis Works
Voice Sentiment Analysis operates by assessing the emotional tone captured in spoken language. It begins with audio recordings of customer interactions, which are then converted into text. This transcription is essential, as it allows the use of linguistic algorithms to analyze sentiment and detect nuances like sarcasm, anger, or positivity.
The analysis involves several key steps: identifying vocal features, such as pitch, tone, and speed; implementing Natural Language Processing (NLP) techniques to interpret the text; and employing machine learning models that classify sentiments appropriately. The combination of these methods enables organizations to recognize customer sentiment in real time, facilitating quicker decision-making. Ultimately, Voice Sentiment Analysis aids businesses in tailoring their communication strategies and improving overall customer experience by turning subjective feedback into actionable insights.
Key Benefits of Using Voice Sentiment Analysis
Voice Sentiment Analysis offers significant advantages for businesses seeking a deeper understanding of customer interactions. By evaluating vocal cues, such as tone and inflection, organizations can gain insights into customer emotions and sentiments. This nuanced understanding helps identify pain points, enabling businesses to address concerns proactively and refine their service offerings.
Moreover, Voice Sentiment Analysis enhances decision-making by providing real-time feedback on customer responses. This data allows companies to create targeted strategies that resonate well with their audience, improving customer satisfaction and loyalty. By employing this analytical approach, organizations not only streamline their operations but also foster a culture of continuous improvement. Ultimately, the integration of Voice Sentiment Analysis into business practices can lead to higher engagement and better overall outcomes.
Tools for Creating Voice Sentiment Heatmaps by Agent Group
When creating voice sentiment heatmaps, you have several tools at your disposal that cater specifically to this analysis. These tools enable organizations to understand the sentiments expressed in customer interactions, which is vital for improving service and product offerings. Each tool varies in functionality, ease of use, and integration capabilities. Properly selecting the right tool for your needs can significantly enhance how you visualize and interpret customer emotions.
Firstly, consider the primary tool known for its capabilities in voice sentiment analysis heatmaps. Its comprehensive features allow users to input large datasets and extract actionable insights effectively. In addition, other secondary tools can complement this primary tool. These might include platforms specializing in data visualization, AI-driven analysis algorithms, and integration features for seamless operations. Each tool you choose plays a crucial role in the overall effectiveness of your sentiment analysis strategy.
Ultimately, selecting the appropriate technologies not only helps visualize sentiments but also provides deeper insights into customer satisfaction and business performance.
insight7: Leader in Voice Sentiment Analysis Heatmaps
In the realm of Voice Sentiment Analysis, understanding customer emotions through their tone and speech patterns is paramount. By embracing these insights, organizations can tailor their interactions more effectively, enhancing overall customer experiences. Heatmaps serve as valuable tools, visualizing emotional trends across various conversations, providing clarity and depth in understanding customer sentiment.
Voice Sentiment Analysis Heatmaps leverage advanced technologies to illustrate where sentiments peak and trough in dialogue. These visual representations are instrumental in identifying emotional triggers, enabling businesses to refine their communication strategies. Additionally, they facilitate real-time feedback loops, empowering teams to make swift adjustments that align with customer expectations. By adopting such innovative methods, businesses can stay ahead of competition and drive customer satisfaction, transforming insights into actionable strategies that resonate with their audiences.
Additional Tools for Voice Sentiment Analysis
To enhance Voice Sentiment Analysis, various additional tools can offer valuable support to your analysis process. These tools provide diverse functionalities that can integrate seamlessly with your existing systems. For instance, incorporating advanced analytical software can help refine your algorithms and improve accuracy. Additionally, real-time data processing tools can elevate your ability to react promptly to customer sentiments, allowing for a more agile approach to customer service.
Consider what each tool brings to the table. Tool one might focus on enhancing speech recognition, ensuring that nuances in customer tone are captured effectively. Tool two could specialize in sentiment tagging, allowing for easy categorization of emotions in conversations. Lastly, tool three may excel in visualization, creating intuitive dashboards where insights can be monitored and analyzed efficiently. Together, these resources optimize your Voice Sentiment Analysis efforts, paving the way for more insightful heatmaps and stronger engagement strategies.
- Tool 1: [Secondary Tool Name]
Tool 1: [Secondary Tool Name]
This tool is a significant player in Voice Sentiment Analysis, enabling businesses to gauge customer emotions through voice interactions. Users can interface with this tool to analyze audio recordings, extracting vital insights about customer sentiments effectively. It operates by converting spoken words into text and analyzing the emotional tone embedded within the speech.
The strength of this tool lies in its real-time processing capabilities. Users can monitor sentiments as they unfold, allowing for timely adjustments in customer engagement strategies. Moreover, its integration with existing customer relationship management systems streamlines data flow, enhancing efficiency. By adopting this tool, organizations can significantly boost their understanding of customer experiences, driving deeper connections and informed decision-making. Thus, it sets the foundation for creating impactful Voice Sentiment Heatmaps, tailored to specific agent groups and scenarios.
- Tool 2: [Secondary Tool Name]
Tool 2: [Secondary Tool Name]
In the realm of voice sentiment analysis, selecting the right tools is crucial for effective performance. Tool 2: [Secondary Tool Name] offers advanced capabilities that enhance the accuracy of sentiment measurement by analyzing vocal tone, pitch, and emotion. This tool is designed to provide insights into customer interactions, making it an essential resource for teams looking to improve service quality.
To maximize the potential of this tool, consider the following aspects:
Integration: Ensure the tool seamlessly integrates with your existing platforms to facilitate smooth operation.
Customization: Look for features that allow you to tailor analysis according to specific client needs and scoring systems.
User-Friendly Interface: A simple, intuitive design helps team members utilize the tool effectively.
By focusing on these elements, you can significantly enhance your voice sentiment analysis process and create impactful heatmaps that drive actionable insights.
- Tool 3: [Secondary Tool Name]
Voice sentiment analysis has become a crucial component in understanding customer interactions and improving service quality. Tool 3: [Secondary Tool Name] offers enhanced functionalities tailored specifically for detailed sentiment analysis. This tool enables organizations to process voice data effectively and derive insights from it, allowing teams to communicate more effectively. Its core capabilities focus on converting voice inputs into sentiment scores, creating a comprehensive overview of customer emotions during interactions.
The primary features include advanced natural language processing capabilities, customizable scoring algorithms, and real-time analysis. By employing this tool, agents can receive immediate feedback on their interactions, facilitating timely improvements. This results in not only better customer relationships but also targeted training programs for agents. The insights gained can significantly impact decision-making processes, ultimately leading to enhanced customer satisfaction and retention. Embracing voice sentiment analysis tools like this unlocks opportunities to refine strategies based on authentic emotional data.
Extract insights from interviews, calls, surveys and reviews for insights in minutes
Steps to Create AI-Based Voice Sentiment Heatmaps
Creating AI-based voice sentiment heatmaps involves a systematic process that can help visualize customer emotions derived from their voice interactions. Begin with data collection and preprocessing. Gather voice recordings and transcriptions, focusing on quality and clarity since these will serve as the foundation for analysis. Clean the data by removing irrelevant content and ensuring consistent formatting, allowing for more accurate sentiment analysis.
Next, implement AI models designed for voice sentiment analysis. Choose the appropriate machine learning algorithms that can analyze emotional cues and vocal tones in the recordings. Once the sentiment data is processed, proceed to visualize the results in heatmaps. This will display emotional intensity across different segments of the conversation, clearly highlighting areas of customer dissatisfaction or satisfaction. Proper visualization enhances understanding and enables informed decision-making to improve customer experiences effectively.
Step 1: Data Collection and Preprocessing
Data Collection and Preprocessing is the foundation for effective voice sentiment analysis. In this initial step, itโs crucial to gather relevant audio data that captures a diverse range of sentiments. Typically, this data might consist of recorded conversations or customer feedback from various sources, such as call centers or online platforms. Ensuring a rich dataset can significantly enhance the model's understanding of different emotional tones, which is essential for accurate sentiment mapping.
Once data is collected, preprocessing comes into play. This involves cleaning and formatting the audio files to ensure consistency. Techniques such as noise reduction, normalization, and segmentation help bring clarity to the recordings. Additionally, converting audio data into text through transcription enables further analysis of spoken words. These meticulous steps in data collection and preprocessing set the stage for the development of AI models, ensuring that voice sentiment analysis yields reliable and insightful heatmaps.
Step 2: Implementing AI Models for Sentiment Analysis
To implement AI models for sentiment analysis effectively, it's vital to understand the foundational elements of Voice Sentiment Analysis. Begin by selecting appropriate machine learning algorithms that can interpret emotional cues from voice recordings. Algorithms like Natural Language Processing (NLP) and Deep Learning are particularly useful, as they enable the system to analyze tone, pitch, and pace of speech.
Next, preprocess the audio data to ensure it is clean and ready for analysis. This may involve noise reduction, normalization, and transcription of verbal inputs into text. Once the data is prepped, train your AI models using labeled datasets that reflect various sentiments. During the training phase, your model should learn to differentiate between positive, negative, and neutral sentiments based on the variations in voice dynamics. Finally, validate your models with testing datasets to ensure their predictive accuracy, enabling you to create insightful heatmaps that visually represent sentiment patterns.
Step 3: Visualizing Results in Heatmaps
In this stage, we focus on visualizing the analysis outcomes through heatmaps. Using heatmaps allows us to transform intricate data into an easily digestible format, highlighting patterns in voice sentiment analysis. By representing sentiment scores visually, stakeholders can quickly identify trends and anomalies across agent groups.
To create effective heatmaps, first, categorize your data according to sentiment intensityโranging from positive to negative. Next, choose appropriate color scales to ensure clarity; for example, use warm tones for negative sentiments and cool tones for positive ones. Finally, overlay these visuals with key metrics from your analysis, such as customer pain points and satisfaction scores, to provide context. This interplay of color and data aids in comprehensive decision-making and helps guide future strategies in voice sentiment analysis.
Conclusion on the Impact of AI-Based Voice Sentiment Analysis
The impact of AI-based voice sentiment analysis is profound and multifaceted. By harnessing advanced technology, organizations can gain insightful perspectives on customer emotions and engagement, leading to better decision-making. Voice sentiment analysis not only helps in identifying trends but also enables agents to refine their interactions based on real-time feedback, thereby enhancing the overall customer experience.
Moreover, the capability to create detailed heatmaps allows for a visual representation of sentiment across different interactions. This fosters a deeper understanding of how various factors influence customer emotions. Ultimately, the integration of voice sentiment analysis can drive significant improvements in service quality, compliance, and customer satisfaction, ensuring that businesses effectively respond to their audiences.