In the rapidly evolving world of call centers, Predictive Scheduling Optimization emerges as a game-changer in workforce management. This innovative approach uses historical call data to forecast demand and optimize agent schedules, ensuring that the right number of agents are available at peak times. With increasing call volumes and varied customer demands, organizations must harness predictive analytics to enhance service levels while minimizing operational costs.
Understanding the principles behind predictive scheduling is critical for call centers aiming to improve efficiency. By analyzing past call patterns, companies can identify trends that inform staffing decisions, allowing for more dynamic and responsive scheduling practices. This not only improves customer satisfaction but also empowers agents, reducing burnout and turnover. Embracing such intelligent scheduling methods is vital for staying competitive in todayโs fast-paced environment.
Analyze & Evaluate Calls. At Scale.
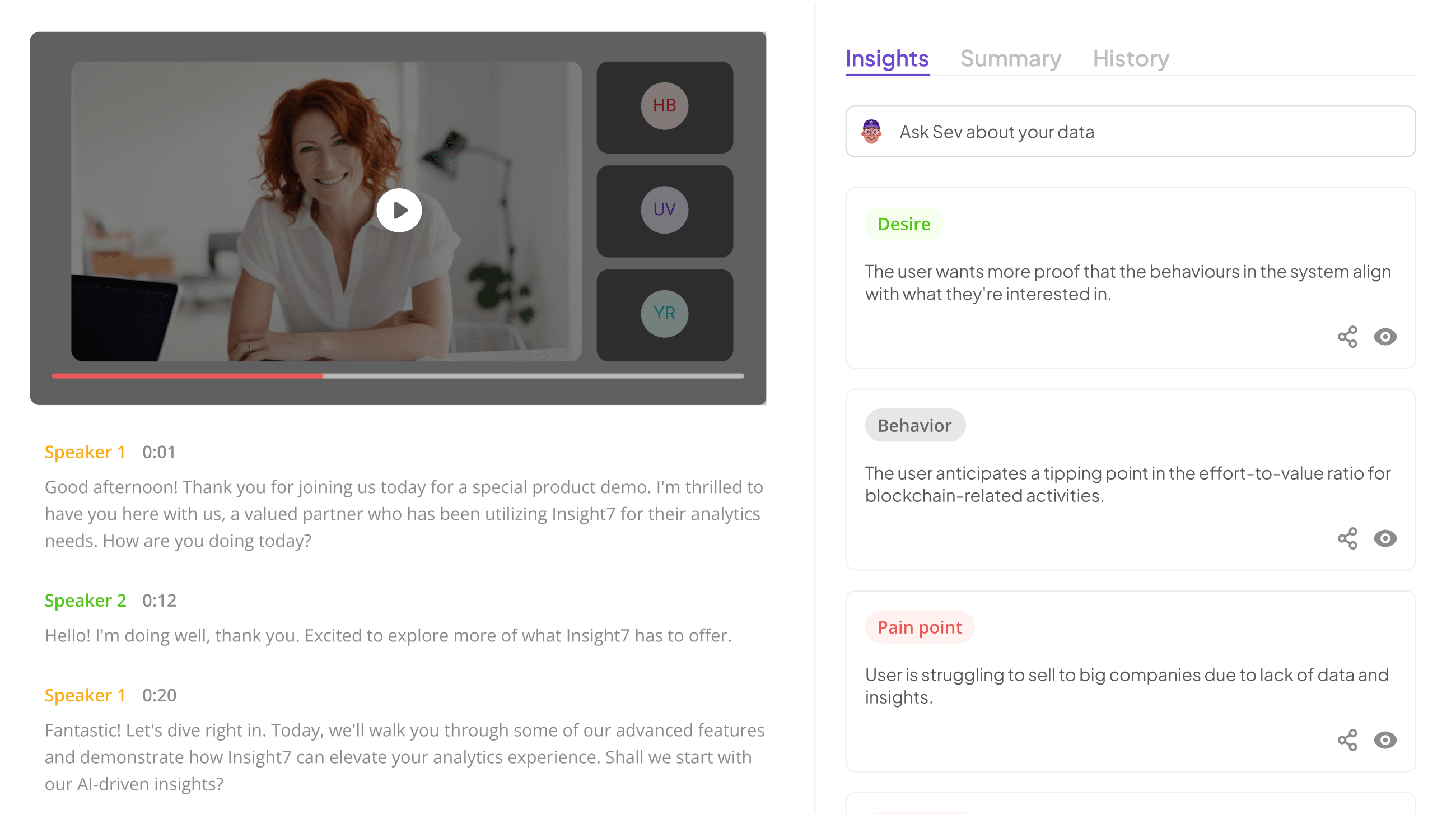
Understanding Predictive Scheduling Techniques
Understanding Predictive Scheduling Techniques involves recognizing how historical call data can significantly influence the efficiency of agent scheduling. By analyzing past call volumes, businesses can identify patterns and trends that inform better staffing decisions. For instance, knowing peak call times can enable organizations to allocate resources efficiently, minimizing wait times for customers and optimizing agent availability.
To implement Predictive Scheduling Optimization effectively, it is crucial to focus on several key aspects. First, selecting relevant features from the historical data is fundamental for accurate model predictions. These features could include call volume, call types, and time of day. Second, accurate modeling directly impacts the efficiency of agent scheduling. A well-trained model not only anticipates demand but also adapts to shifts over time, leading to improved operational performance. By grasping these techniques, organizations can leverage their historical call data for strategic scheduling benefits.
Importance of Historical Call Data Analysis
Historical call data analysis plays a pivotal role in developing effective predictive scheduling optimization. By examining past call data, organizations can gain insights into customer behavior patterns. Recognizing trends, such as peak call times and frequently asked questions, helps tailor agent schedules to meet demand efficiently. This strategic alignment leads to reduced wait times and improved customer satisfaction.
Moreover, analyzing historical call data assists in identifying areas needing staff training. Understanding common customer inquiries allows management to customize training sessions, ensuring that agents are well-prepared for the challenges they face. Additionally, consistent monitoring of performance metrics provides valuable feedback on how effectively the training translates into service quality. Ultimately, historical call data analysis lays a foundation for informed decision-making in scheduling, enhancing overall operational effectiveness.
- How historical data influences agent scheduling
Historical data plays a vital role in enhancing agent scheduling, particularly in predictive scheduling optimization. By analyzing past call data, organizations can identify patterns that inform call volume fluctuations. These insights help determine when to allocate more or fewer agents, ensuring that staffing levels match anticipated demand.
Data from different time periods sheds light on peak hours and seasonal variations. For instance, if past trends show increased call volume during holidays, scheduling can be adjusted to meet this demand proactively. Additionally, understanding historical data allows for the discovery of performance patterns among agents, which can guide training and development opportunities. Effective utilization of these insights not only improves scheduling efficiency but also enhances customer satisfaction by ensuring timely responses to inquiries. By harnessing the power of historical data, organizations can create a robust framework for optimizing agent schedules and addressing future demands effectively.
- Identifying patterns and trends in call volumes
Understanding call volumes can significantly improve agent scheduling efficiency. By examining historical data, organizations can identify consistent patterns and trends in call behavior. For instance, peak call times often occur during specific hours or days. This insight allows teams to allocate resources effectively, ensuring sufficient staffing during high-demand periods, leading to better customer service and reduced wait times.
Moreover, recognizing variations in call volumes can inform predictive scheduling optimization. Analytical techniques can pinpoint seasonal fluctuations or promotional events that might lead to increased call activity. By employing advanced analytics, organizations can develop models that adapt to these trends, ultimately enhancing scheduling accuracy. This strategy not only improves operational efficiency but also aids in workforce management by balancing staff availability with anticipated call volumes. Adapting to these patterns ensures agents are strategically positioned to meet customer needs when demand is at its highest.
Key Elements in Predictive Model Development
In developing a predictive model for scheduling, several key elements play a vital role in ensuring its effectiveness. First, selection of relevant data features is crucial, as it directly influences the modelโs outcome. Historical data, such as peak call times and customer interactions, allows developers to create models grounded in real-world behavior. This foundational step serves to identify patterns that inform optimal scheduling practices tailored to workforce availability and call demand.
Secondly, the accuracy of the modeling process significantly impacts scheduling efficiency. Implementing appropriate algorithmsโlike machine learning techniquesโenhances predictive scheduling optimization. Testing and refining these models ensures robust predictions, translating to better resource allocation and improved service delivery. Achieving a thorough understanding of these elements enables organizations to utilize predictive models that systematically enhance the efficiency of their scheduling processes, ultimately benefiting both agents and customers.
- Selection of relevant data features
The selection of relevant data features is crucial in developing effective predictive models for agent scheduling. In the context of Predictive Scheduling Optimization, identifying the right variables helps uncover insights into call trends and agent performance. Effective feature selection begins with a thorough examination of historical call data, focusing on aspects such as call volume, peak hours, and average handling time.
Additionally, it involves determining which features directly influence scheduling efficiency. Factors like customer wait times, agent availability, and call types can greatly affect outcomes. Once relevant features are established, data scientists can apply machine learning algorithms to refine their predictive models. By systematically selecting features that correlate with successful scheduling outcomes, organizations can significantly enhance their operational efficiency and responsiveness to changing demand patterns.
- Impact of accurate modeling on scheduling efficiency
Accurate modeling significantly influences scheduling efficiency in call centers. When predictive scheduling optimization is established, relying on historical call data transforms how agents are assigned to shifts. A precisely developed model predicts call volumes, enabling organizations to allocate resources where they are most needed. As a result, agents can be scheduled to match anticipated demand, reducing wait times and enhancing customer satisfaction.
Effective predictive modeling involves several crucial steps. First, leveraging robust historical data allows for identifying patterns in call flow. Next, the model must integrate variables such as peak periods and agent availability to refine accuracy. Relying on data-driven insights promotes timely adjustments, allowing organizations to enhance workforce management continually. Ultimately, when these models are implemented successfully, they foster an agile scheduling approach that balances efficiency and employee satisfaction. By recognizing the impact of accurate modeling, organizations can achieve not only operational effectiveness but also long-term growth in service quality.
Extract insights from interviews, calls, surveys and reviews for insights in minutes
Steps for Building Predictive Scheduling Optimization Models
Building Predictive Scheduling Optimization models is essential for maximizing call center efficiency. The first step involves collecting and preparing historical call data. Gathering accurate data can include sourcing information from CRM systems or call logs. Once collected, the next crucial aspect is cleaning and structuring the data. This ensures consistency, which significantly impacts model accuracy.
The second stage entails model selection and training. Here, you need to decide between various predictive models like time series analysis or machine learning algorithms. The selected model is then trained on the structured data to recognize patterns that help forecast future call volumes. Lastly, thorough model evaluation and tuning are vital for optimizing performance. Assess the modelโs predictions against actual call data, making adjustments as necessary to enhance accuracy. Following these steps will lead to an efficient predictive scheduling system tailored to call center needs.
Step 1: Data Collection and Preparation
To initiate Predictive Scheduling Optimization for call centers, the first step is crucial: data collection and preparation. Begin by gathering historical call data from various sources such as call logs, CRM systems, and customer feedback databases. This data will provide valuable insights into customer interactions and call volumes over time, essential for predicting future needs.
Once you've gathered the necessary data, itโs important to clean and structure it properly. This involves identifying and removing duplicates, correcting inaccuracies, and organizing the information for analysis. By preparing your data meticulously, you can identify patterns and trends that inform your scheduling strategies. A well-structured dataset will enhance the accuracy of your predictive models, ensuring they effectively anticipate staffing needs and improve operational efficiency. Remember, the success of predictive scheduling largely depends on the quality of the data you start with, making this initial step vital.
- Methods for gathering historical call data
Gathering historical call data is crucial for implementing successful predictive scheduling optimization in call centers. First, it is essential to collect data from various sources, such as customer interactions, call logs, and performance metrics. This comprehensive collection allows for an accurate reflection of call center activities and trends over time. By centralizing this information, you can ensure that the data is structured effectively for analysis.
Next, the cleaning and structuring of data play a vital role in its usability. Remove duplicates, correct inaccuracies, and standardize formats to enhance data quality. Once clean, relevant data features should be chosen based on your objectivesโwhether itโs to analyze peak call times or agent performance. This organized foundation enables more insightful analysis, ultimately guiding your predictive agent scheduling efforts toward greater efficiency and improved customer experience.
- Cleaning and structuring data for analysis
Cleaning and structuring data for analysis forms a pivotal part of implementing predictive scheduling optimization in call centers. Initially, raw historical call data requires thorough examination to identify inconsistencies such as missing values or duplicate entries. This stage is crucial, as any inaccuracies in this data can skew predictions, leading to inefficient scheduling outcomes. Streamlining data involves standardizing formats and categorizing attributes like call times, durations, and customer feedback efficiently.
Next, structuring the data enhances its usability for analysis. This involves organizing the data into manageable formats, using techniques such as normalization or aggregation. By building a well-defined dataset, users can effortlessly extract meaningful insights that inform agent scheduling decisions. Ultimately, the goal is to ensure that the data serves as a reliable foundation upon which effective predictive models can be constructed, driving efficiency in call center operations.
Step 2: Model Selection and Training
Model selection and training are crucial steps in developing a robust predictive scheduling optimization system. Begin by exploring various predictive models, including time series analysis and machine learning algorithms. It is essential to select a model that aligns with the complexity of your historical call data. Each model has unique strengths that may influence scheduling efficiency depending on the patterns recognized within the data.
Once a suitable model is selected, the next phase involves training it with meticulously prepared historical data. Training requires feeding the model with examples, allowing it to learn and adjust based on patterns and trends. Pay attention to data quality during this stage, as clean and well-structured data significantly enhance the model's performance. The ultimate goal is to create a predictive agent scheduling system that effectively optimizes workforce management, ensuring you meet customer demands while maximizing agent productivity.
- Choosing the right predictive model (e.g., time series analysis, machine learning algorithms)
Selecting an appropriate predictive model is crucial for effective agent scheduling using historical call data. Time series analysis and machine learning algorithms offer distinct advantages depending on the specific needs of your organization. Time series analysis focuses on identifying trends and seasonal patterns in call volumes, providing a structured approach to predict future demands. This model excels when historical data exhibits consistent patterns over time, ensuring a reliable scheduling framework.
On the other hand, machine learning algorithms offer greater flexibility and adaptability. They can process vast datasets and uncover complex relationships that traditional methods might overlook. These models are especially beneficial when call data is highly variable or when numerous factors influence call patterns. By analyzing historical data through these lenses, you can develop a robust Predictive Scheduling Optimization strategy tailored to your operational requirements. Ultimately, the right choice will depend on your data structure and business goals, making it essential to assess both methods carefully.
- Training the model using the processed data
Training the model using processed data is a critical step in developing effective predictive scheduling optimization for call centers. First, it involves selecting the right predictive algorithms that best fit the unique characteristics of your historical call data. This could range from time series analysis to advanced machine learning techniques, designed to identify and leverage patterns in call volume and agent performance.
Once the relevant models are chosen, the training process begins. This phase focuses on feeding the cleaned and structured data into the model, allowing it to learn relationships between various features such as time of day, call type, and agent skills. By iterating through numerous data cycles, the model enhances its predictive accuracy, helping to streamline scheduling decisions. Continuous evaluation and refinement of the model will further improve prediction outcomes, ultimately leading to optimized staffing levels and enhanced operational efficiency.
Step 3: Model Evaluation and Tuning
Evaluating and tuning your predictive scheduling model is essential for achieving optimal performance. This step ensures that your model accurately reflects historical call data and adapts to current trends. Begin by assessing the model's accuracy through various metrics, such as precision, recall, and F1 score, to determine how well it predicts call volumes and agent needs.
Next, fine-tune the model by adjusting parameters or employing different algorithms to enhance Predictive Scheduling Optimization. Experiment with multiple iterations of the model, closely monitoring its performance across different datasets. Furthermore, consider cross-validation techniques to validate the model against unseen data, ensuring it can generalize well. Through systematic evaluation and iterative adjustments, you can significantly improve the predictive capabilities of your scheduling system. Ultimately, this tailored approach leads to enhanced efficiency and effective staffing strategies in response to historical call patterns.
- Techniques for assessing model accuracy and performance
Model evaluation is a critical step in predictive scheduling optimization, ensuring that the predictions made are both accurate and reliable. One essential technique is the use of cross-validation, which involves partitioning the data into subsets. This technique helps in evaluating how well the model performs on unseen data, thereby simulating real-world scenarios. Another important approach is to track metrics such as accuracy, precision, recall, and F1-score, which provide a comprehensive understanding of model performance.
Additionally, visualizations can be helpful in assessing model performance. Tools like confusion matrices can highlight where the model excels and where it falters. Monitoring prediction intervals also aids in understanding uncertainty, allowing adjustments for agent scheduling when necessary. Ultimately, implementing these techniques will enhance predictive scheduling optimization, making it not only effective but also adaptable to changing call patterns.
- Fine-tuning the model for better predictions
Effective fine-tuning of the predictive model is essential for enhancing the accuracy of scheduling predictions. To achieve Predictive Scheduling Optimization, data scientists must closely examine their model's performance through various metrics. This involves initially assessing the model's accuracy and making necessary adjustments, ensuring that it responds accurately to historical data trends. A systematic evaluation process allows the identification of specific areas needing improvement, whether it's accuracy, recall, or precision.
Moreover, incorporating feedback loops can vastly improve performance further. Continuously updating the model with new call data helps it adapt to dynamic patterns, ensuring optimal scheduling choices. Techniques such as hyperparameter tuning can refine how the model interprets data, adjusting settings for improved predictions. By maintaining a cycle of evaluation and adjustment, organizations can ensure their predictive models are accurately aligned with real-world scenarios, thereby enabling more efficient agent scheduling and enhanced call center performance.
Top Tools for Implementing Predictive Scheduling Optimization
Implementing predictive scheduling optimization requires utilizing powerful tools that enhance efficiency and improve accuracy. The first tool worth considering is ZoomShift, which offers robust features tailored specifically for managing call center schedules. This platform integrates seamlessly with existing systems, allowing for easy tracking of employee availability while providing insights into peak call times.
Another effective option is Deputy, known for its comprehensive scheduling capabilities. It not only enhances planning efficiency but also offers analytics to gauge performance against goals. Additionally, 7shifts provides advanced forecasting tools designed for workforce management and helps predict when the highest call volumes are likely to occur. Finally, Sling ensures real-time scheduling updates with alerts for both agents and management.
Choosing the right mix of these tools can empower call centers to implement predictive scheduling optimization effectively. Ultimately, leveraging these solutions will help streamline operations, reduce wait times, and enhance overall customer satisfaction.
insight7
Understanding how to effectively implement Predictive Scheduling Optimization begins with recognizing its significance. When organizations utilize historical call data, they can uncover patterns that inform agent scheduling. This process minimizes wait times, improves service levels, and enhances customer satisfaction. It is crucial, therefore, to focus on the historical dataโs intricacies, revealing trends that directly impact staffing requirements.
To implement Predictive Scheduling Optimization successfully, organizations should follow several key steps. First, data collection and preparation are essential for ensuring that the historical call data is clean and structured, making it ready for analysis. Next, model selection is fundamental; organizations must choose appropriate predictive models like time series analysis or specific machine learning approaches. Finally, model evaluation and tuning help maintain accuracy in predictions and scheduling efficiency. These steps, when executed strategically, substantially enhance operational effectiveness in call centers while significantly improving overall customer engagement.
- Key features and benefits for call centers
Predictive Scheduling Optimization offers call centers numerous features and benefits that enhance overall operational efficiency. Firstly, it enables accurate forecasting of call volumes by analyzing historical data. This precision allows managers to allocate agents strategically during peak and off-peak times. Secondly, the efficiency gained from predictive scheduling reduces instances of both understaffing and overstaffing, ensuring that customer needs are met without incurring unnecessary labor costs.
Furthermore, empowered by such optimization, employees gain better work-life balance due to more predictable schedules. This predictability enhances job satisfaction, leading to lower turnover rates. Additionally, the availability of insights into agent performance helps supervisors conduct targeted training sessions. This ensures consistent quality in customer interactions, elevating the service level across the board. Through these interconnected advantages, call centers can significantly improve productivity and customer satisfaction, solidifying their competitive edge in the marketplace.
Additional Tools
When considering additional tools for Predictive Scheduling Optimization, various options can significantly enhance your scheduling capabilities. Each of these tools offers unique features designed to streamline agent management and improve overall efficiency. These tools can work in tandem with the historical call data youโve already analyzed, providing deeper insights and facilitating better decision-making.
ZoomShift simplifies the scheduling process with its user-friendly interface. It allows for real-time updates, ensuring that shifts are effectively communicated and adjusted as needed.
Deputy stands out for its powerful analytics. Not only does it assist with scheduling, but it also helps in workforce management, analyzing data to provide actionable insights.
7shifts focuses on forecasting, equipping managers with tools to predict future staffing needs based on historical data trends.
Sling ensures agents receive real-time updates and alerts about their schedules, fostering clear communication and adaptability.
By incorporating these tools, organizations can enhance their predictive scheduling strategies and ultimately drive operational success.
- ZoomShift: Features and integration options
The integration of ZoomShift into your predictive scheduling framework offers a host of features catered specifically for call centers. Its dashboard allows users to customize schedules based on historical call data, enabling smarter staffing decisions. Users can leverage automated scheduling options, ensuring that agents are available when call volumes peak. Additionally, performance analytics give insights into agent productivity, supporting better resource allocation.
Integrating ZoomShift with existing systems enhances the capacity for predictive scheduling optimization. It supports API connections, allowing for seamless data flow between platforms. Users can also benefit from mobile access, enabling managers to make real-time adjustments, keeping operations agile. With its focus on user experience, ZoomShift ensures that organizations can easily adapt to fluctuating call demands while maintaining high service levels. Thus, it positions itself as a crucial tool for any center aiming for operational excellence.
- Deputy: Scheduling capabilities and analytics
In the realm of predictive scheduling optimization, effective scheduling capabilities and analytics play a crucial role in enhancing operational efficiency within workplaces. Advanced scheduling platforms can analyze historical call data, offering insights that help organizations forecast call volumes accurately. By leveraging these insights, managers can allocate staff efficiently, reducing downtime and ensuring better service levels.
Understanding workforce analytics further empowers organizations to adapt to fluctuating demands. For example, shift patterns based on historical data can pinpoint peak call times, enabling proactive scheduling adjustments. This capability not only boosts employee productivity but also improves customer satisfaction, as agents can be present during critical periods. Strong analytics integrated into scheduling systems foster a more agile workforce, allowing companies to respond to real-time needs while optimizing overall operations.
- 7shifts: Forecasting tools for workforce management
Forecasting tools play a critical role in effective workforce management, particularly in call centers. By utilizing advanced predictive scheduling optimization techniques, organizations can streamline their agent scheduling processes. These tools analyze historical call data to identify recurring patterns and trends, allowing for more informed scheduling decisions. As a result, businesses can better align agent availability with expected call volumes.
The use of these tools can lead to significant improvements in operational efficiency. For example, by accurately forecasting busy periods, managers can ensure that there are enough agents on duty to handle incoming calls. This not only enhances customer satisfaction but also optimizes resource allocation. The ability to anticipate staffing needs decreases costs while maximizing service quality. In essence, implementing robust forecasting tools can fundamentally transform how call centers approach workforce management, leading to sustainable success in this competitive landscape.
- Sling: Real-time scheduling updates and alerts
Sling provides essential real-time scheduling updates and alerts that enhance operational efficiency in call centers. By utilizing historical call data, it enables teams to respond quickly to changing conditions and maintain optimal staffing levels. The timely notifications allow managers to adjust schedules based on predicted call volumes, ensuring that agents are matched with demand effectively.
Additionally, real-time updates foster improved agent management. With predictive scheduling optimization, agents receive immediate alerts for any changes in their schedules, allowing them to adapt quickly. This leads to reduced downtime and higher customer satisfaction, as the right resources are always available to address incoming calls. Embracing such technologies not only streamlines operations but also empowers employees to perform their best, enhancing both productivity and morale in the workplace.
Conclusion: Embracing Predictive Scheduling Optimization for Enhanced Efficiency
Predictive Scheduling Optimization offers a compelling pathway to enhancing operational efficiency in call centers. By harnessing historical call data, organizations can forecast call volumes more accurately and allocate resources accordingly. This proactive approach not only reduces wait times for customers but also improves agent productivity by aligning their schedules with anticipated demand.
As call centers embrace this optimization, they can expect significant improvements in service delivery and business outcomes. By continuously analyzing patterns in call data, teams can adapt to changing conditions and ensure their operations remain agile. In summary, Predictive Scheduling Optimization serves as a key strategy for achieving operational excellence and meeting customer expectations effectively.