Real-Time Sentiment Analysis has emerged as a powerful tool in today’s data-driven world. By capturing the emotions behind customer interactions, businesses can adapt their strategies almost instantaneously. Imagine a customer expressing frustration on social media; with real-time sentiment tracking, that feedback can prompt immediate action, fostering loyalty and trust.
Understanding real-time sentiment analysis is essential for businesses aiming to succeed in a competitive market. It involves collecting live data from multiple sources and processing this information to gain actionable insights. As companies generate more customer signals than ever, methods that facilitate rapid analysis can make the difference between leading the market and falling behind.
Analyze & Evaluate Calls. At Scale.
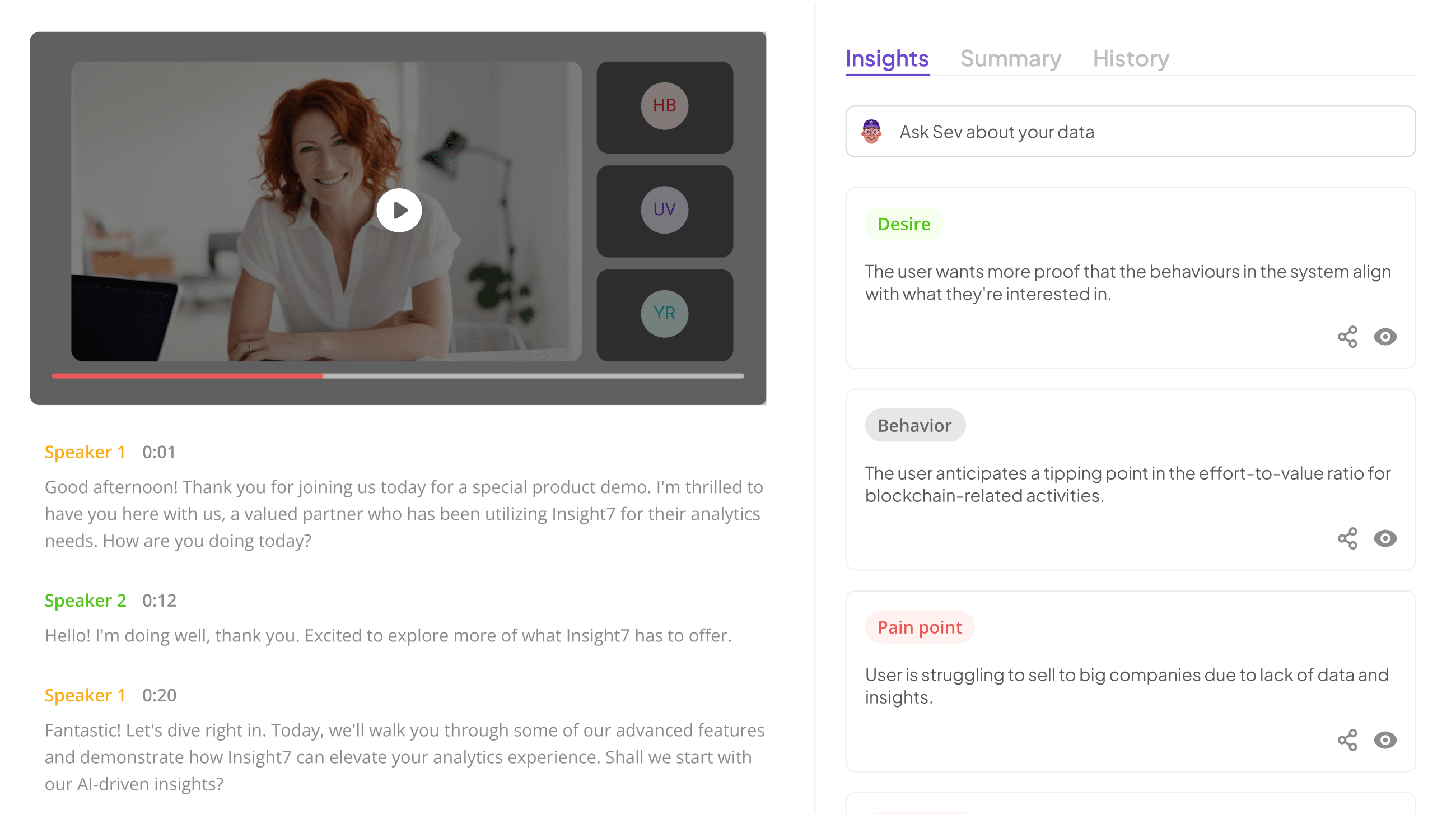
Real-Time Sentiment Analysis: Capturing Emotions Instantly
Real-time sentiment analysis plays a vital role in understanding customer emotions as they emerge. By employing advanced algorithms and machine learning techniques, businesses can capture these feelings instantly from various data sources. This capability allows organizations to respond dynamically to customer needs, ultimately leading to improved satisfaction and loyalty.
To effectively harness real-time sentiment analysis, several key elements should be considered. First, it’s essential to gather data from diverse sources such as social media platforms, customer reviews, and survey responses. Next, the data must be processed efficiently to extract meaningful insights. Utilizing the right tools ensures accuracy and speed in analyzing emotional tones. The insights gained can inform strategic decisions, enabling businesses to engage with their customers more effectively and anticipate their preferences.
By mastering real-time sentiment analysis, companies not only stay ahead of competitors but also foster stronger connections with their clientele.
Step 1: Gathering Data for Real-Time Sentiment Analysis
To initiate the process of building your real-time sentiment tracker, gathering data is a crucial first step. This involves sourcing various types of information that reflect public opinions and emotions about particular topics or products. Leveraging social media platforms, online reviews, and feedback forms can provide a wealth of insights. Each of these data streams contributes unique perspectives, thus offering a more comprehensive view of sentiment trends over time.
Additionally, consider employing web scraping tools to aggregate content from news articles, blogs, and forums. This real-time sentiment analysis allows you to capture spontaneous reactions and sentiments as they evolve. By tapping into diverse data sources, you ensure your analysis is rich and nuanced, enabling informed decision-making processes based on genuine public sentiment. This varied approach not only enhances the depth of your insights but also increases the accuracy of your sentiment tracker as it identifies patterns and shifts in emotional responses.
Break down the sources and methods for collecting data, emphasizing the importance of diverse and live data streams.
To effectively build a real-time sentiment tracker, it is essential to break down diverse sources and methods for collecting data. Various platforms, such as social media, product reviews, and customer feedback channels, serve as vital data streams. Each source contributes distinct perspectives that enrich the sentiment analysis process. Real-time data from platforms like Twitter provides immediate feedback on public opinions, while structured surveys capture deeper insights into customer sentiments.
The importance of integrating these diverse data sources cannot be overstated. Live data streams ensure that the sentiment tracker remains responsive to current events and evolving public moods. Using a combination of qualitative and quantitative data enables a more nuanced understanding of consumer emotions. Together, these methods create a comprehensive ecosystem that enhances the effectiveness of real-time sentiment analysis. By recognizing the strengths of each source, organizations can craft tailored strategies that align with shifting consumer perspectives.
Step 2: Processing Data for Instant Insights
In processing data for instant insights, it’s crucial to focus on organizing and cleaning your data effectively. Start by removing any irrelevant or redundant information, as clutter can lead to distorted results in real-time sentiment analysis. Properly formatted data not only enhances accuracy but also streamlines the analysis process, enabling quicker access to actionable insights.
Next, categorize the data into relevant segments to facilitate deeper analysis. This could involve grouping by sentiment type, such as positive, negative, or neutral responses. By employing this strategy, you can swiftly identify trends and patterns, making it easier to act on the insights derived from your analysis. Continually evaluating your data management practices ensures that the insights you generate remain relevant and helpful. Adopting these practices will allow your organization to respond promptly to customer sentiments, driving meaningful improvements in your offerings.
Discuss the crucial steps in cleaning and organizing the data to ensure accurate sentiment analysis.
Cleaning and organizing the data is essential for achieving accurate real-time sentiment analysis. Initially, data collection from diverse sources is crucial, including social media, surveys, and customer feedback. Next, the raw data often contains inconsistencies, which require thorough cleansing. This involves removing irrelevant information, correcting errors, and standardizing formats to ensure uniformity across datasets.
After cleansing, data organization plays a vital role in facilitating analysis. Structuring the data thoughtfully, perhaps categorizing by sentiment type—positive, negative, or neutral—enables more efficient processing. Additionally, integrating metadata, such as timestamps or user demographics, can enhance contextual understanding. Lastly, consistent updates to the dataset are necessary for maintaining its relevance, as sentiment dynamics can shift quickly. By following these steps, organizations can enhance their capacity for real-time sentiment analysis and derive more valuable insights.
Extract insights from interviews, calls, surveys and reviews for insights in minutes
Implementing Real-Time Sentiment Analysis Tools
Implementing Real-Time Sentiment Analysis Tools involves selecting and integrating software capable of evaluating emotional responses. The first step in implementing these tools is to identify the specific requirements of your organization. Understanding your unique needs will help you choose the most suitable tools for your sentiment analysis efforts.
Next, consider the best tools available for real-time sentiment analysis. Options like IBM Watson Natural Language Understanding and Google Cloud Natural Language offer robust features. Microsoft Text Analytics API is another reliable option, while Aylien and Lexalytics provide user-friendly interfaces for analyzing customer feedback. Evaluating these tools based on factors such as ease of integration and scalability will ensure effective implementation.
Incorporating these advanced tools enables real-time monitoring of customer sentiment, allowing organizations to respond quickly to shifts in public perception. This proactive approach not only enhances customer engagement but also drives better business decisions, thereby staying ahead of competitive pressures.
Best Tools for Real-Time Sentiment Analysis
To effectively conduct Real-Time Sentiment Analysis, selecting the right tools is essential. Several platforms are tailored for this purpose, allowing businesses to gather and interpret emotional data swiftly. Among the top options is IBM Watson Natural Language Understanding, renowned for its robust natural language processing capabilities. It helps organizations understand emotions, sentiments, and intent in customer feedback and social media interactions.
Following closely is Google Cloud Natural Language, which provides powerful text analysis and scoring mechanisms. Microsoft Text Analytics API also stands out for its user-friendliness and integration capabilities, making data processing efficient. Other notable tools include Aylien, known for its advanced machine learning algorithms, and Lexalytics, which excels in sentiment extraction from unstructured text. With these tools, businesses can gain immediate insights, ensuring they remain competitive and responsive to customer needs.
Highlight and discuss the best available tools, starting with insight7, followed by others like:
The landscape of Real-Time Sentiment Analysis is evolving rapidly, with various tools available to optimize the analysis process. Starting with a powerful, self-service platform, the first tool allows users to effortlessly analyze customer interactions and extract actionable insights at scale. This capability is crucial for businesses seeking to improve customer engagement and refine their strategies based on real-time feedback.
Following this, several other tools enhance sentiment analysis efforts. For instance, IBM Watson Natural Language Understanding offers advanced machine learning algorithms for deeper text interpretation. Google Cloud Natural Language provides robust capabilities for sentiment detection and entity recognition, while Microsoft Text Analytics API simplifies the analysis of textual data through its easy-to-use interface. Additionally, Aylien and Lexalytics deliver features that further enhance sentiment tracking by integrating multilingual support and advanced semantic analysis. These tools collectively empower businesses to make informed decisions based on the sentiments expressed by their customers in real time.
- IBM Watson Natural Language Understanding
When discussing advanced analytics, the focus should be on Real-Time Sentiment Analysis as it captures emotions effectively. One prominent tool utilized for this purpose is an innovative technology that excels in understanding and interpreting user sentiment from textual data. Its capabilities include sentiment classification, emotion analysis, and intent extraction, which are pivotal in monitoring reactions to various topics in real time.
By integrating this technology into your sentiment tracker, you can automate the analysis process, providing immediate insights into public perception. This tool processes large volumes of text data instantly, allowing businesses to respond quickly to customer feedback or market shifts. As a result, companies can make informed decisions and adapt their strategies based on the sentiments expressed by their audience. Emphasizing precision and speed, this approach enhances the ability to stay ahead in a competitive landscape.
- Google Cloud Natural Language
To harness the power of Real-Time Sentiment Analysis, exploring advanced tools is essential. One notable option stands out for its robust capabilities: Google Cloud Natural Language. This tool excels at syntactic analysis, entity recognition, and sentiment detection, providing a comprehensive understanding of text data in real-time.
This platform processes unstructured data efficiently, offering instant insights into customer emotions. It can analyze text from various sources, such as social media posts, reviews, and customer surveys, thus aptly capturing sentiments as they emerge. Upon integrating this tool into your systems, you can expect to unravel nuanced emotional responses, equipping your business to adapt swiftly to customer needs. By leveraging such technologies, companies can innovate their strategies, ensuring they remain aligned with evolving market sentiments and customer expectations. This adaptability is what drives success in today's fast-paced environment.
- Microsoft Text Analytics API
The Microsoft Text Analytics API provides robust capabilities for Real-Time Sentiment Analysis, allowing businesses to interpret emotions and opinions instantly. With its advanced algorithms, it can analyze textual data from a variety of sources, transforming raw content into actionable insights. This API excels in identifying emotional tones, categorizing sentiments as positive, negative, or neutral, which is essential for understanding customer feedback and market trends.
To effectively utilize the API, there are a few key features to explore. First, it offers language detection, ensuring that the analysis is accurate regardless of the input language. Second, the feature for key phrase extraction allows businesses to grasp essential topics discussed in the data. Finally, integration options enable seamless use in different applications, making it easier to implement Real-Time Sentiment Analysis in various workflows. By harnessing these capabilities, organizations can make informed decisions based on the sentiments expressed by their customers.
- Aylien
A vital component in the realm of Real-Time Sentiment Analysis is the advanced tool designed to interpret and analyze large volumes of textual data swiftly. This tool integrates natural language processing (NLP), allowing businesses to dissect sentiments expressed in customer feedback, social media posts, and other interactive platforms seamlessly. By capturing emotional tones in real-time, businesses can make informed decisions promptly, ensuring they stay ahead of market trends and customer needs.
To effectively utilize this tool, consider the following steps:
Integrate Data Sources: Combine data from various platforms, such as social media and surveys, to create a comprehensive sentiment profile.
Set Up the Analysis: Ensure the data is appropriately tagged and organized, allowing the tool to process sentiments effectively.
Monitor and Adapt: With real-time capabilities, continually assess the sentiments and adapt strategies based on insights to maintain customer engagement and satisfaction.
These steps will enhance your Real-Time Sentiment Analysis capabilities, leading to more timely and actionable insights that drive business success.
- Lexalytics
In the realm of real-time sentiment analysis, powerful tools play a crucial role in transforming raw data into actionable insights. These tools utilize advanced algorithms to process and interpret language, allowing businesses to understand customer sentiments instantly. One particularly effective solution offers an intuitive interface that enables users of all skill levels to access valuable insights without technical expertise. This democratization of data empowers teams to quickly generate reports and analyze customer experiences without significant training.
The platform facilitates the organization of multiple data sources, providing an easy way to visualize conversations, extract key themes, and identify pain points and desires. By aggregating insights into manageable "cards," users can capture the voice of the customer efficiently. This capability is vital for organizations aiming to enhance their real-time sentiment analysis capabilities and respond to customer feedback proactively. By leveraging these insights, businesses can make informed decisions that positively impact their overall strategy.
Step-by-Step: Building Your Own AI Model for Sentiment Analysis
Creating an AI model for sentiment analysis involves a series of steps that enable you to capture emotions instantaneously. Start by gathering relevant data from various sources, such as social media, customer reviews, and surveys. This diverse mix provides a solid foundation for understanding public sentiment. It's important to ensure your data stream is live to keep your analysis current and relevant.
Next, focus on processing the data. This entails cleaning and organizing the information to enhance the accuracy of your analysis. Techniques like tokenization and removing stop words will help streamline your dataset. Once your data is clean, you can train your AI model using machine learning algorithms. Experiment with different models to find the one that best captures and interprets emotions. By following these steps, you will be on your way to developing a robust system for real-time sentiment analysis, tailored to your specific needs.
Guide the reader through the process of creating a custom AI model with detailed steps for training it effectively.
To create a custom AI model for real-time sentiment analysis, start by defining your goals clearly. Identify the specific types of sentiments you want to track, such as positive, negative, or neutral. Next, gather diverse datasets that reflect various emotional expressions. This can be achieved through social media posts, customer reviews, or online forums. Ensure that the data is current and diverse to capture a wide range of sentiments.
Once you have your data, move on to preprocessing it. This step involves cleaning and organizing the text data to eliminate any noise. Techniques such as tokenization, removing stop words, and stemming can optimize the model’s understanding. After preprocessing, you can select a machine learning framework. Train your model using algorithms like logistic regression or recurrent neural networks, and evaluate its performance using metrics such as accuracy or F1 score. This systematic approach will pave the way for effective real-time sentiment analysis in your projects.
Conclusion: Leveraging Real-Time Sentiment Analysis for Business Success
In today’s fast-paced business world, incorporating real-time sentiment analysis can significantly enhance decision-making processes. By understanding customer emotions as they occur, businesses can swiftly adapt their strategies, ensuring they stay ahead of the competition. These insights transform raw data into actionable strategies, influencing product development and marketing efforts.
Additionally, businesses that effectively implement real-time sentiment analysis often experience improved customer engagement. By promptly responding to customer feedback, companies foster loyalty and build positive relationships. Ultimately, real-time sentiment analysis serves as a powerful tool for achieving sustainable business success by informing both operational and strategic initiatives.