Predictive Staffing Optimization serves as a transformative approach for organizations aiming to enhance workforce efficiency. Imagine a world where your staffing levels are perfectly aligned with demand patterns, leading to increased customer satisfaction and reduced operational costs. This model uses data-driven insights to anticipate workforce needs, allowing for a more agile response to fluctuating requirements.
Understanding the fundamentals of predictive staffing is vital for businesses today. By integrating predictive analytics into staffing strategies, organizations can identify trends, optimize resource allocation, and enhance overall performance. This section will explore the importance of predictive staffing optimization and how it can be effectively implemented in various industries.
Analyze qualitative data. At Scale.
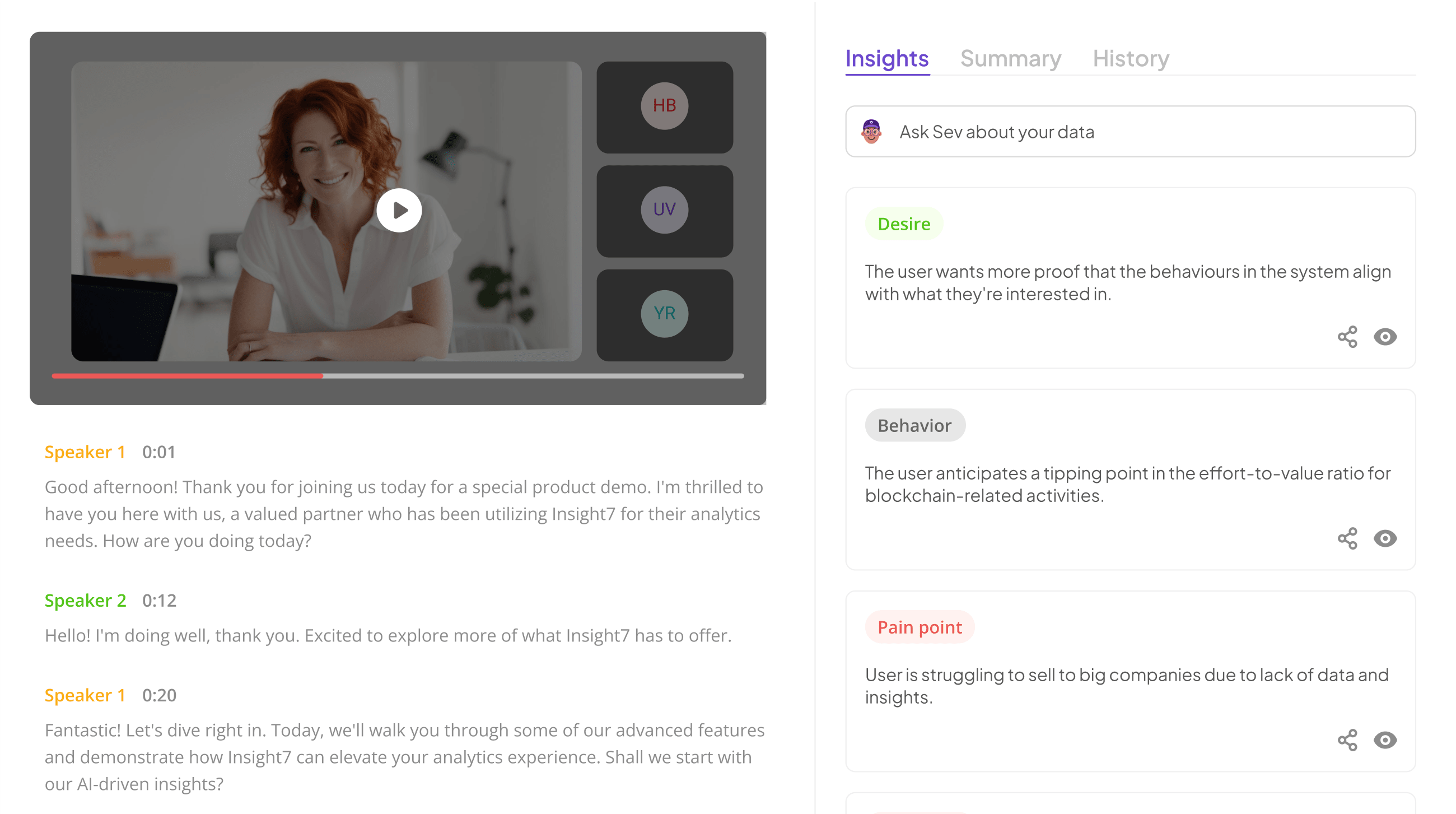
Understanding Predictive Staffing Optimization
Predictive Staffing Optimization serves as a vital approach for businesses aiming to streamline workforce management. By analyzing past performance and future trends, organizations can make informed staffing decisions, reducing overstaffing and understaffing scenarios. This method leverages statistical models and data analytics to identify the right number of employees needed for specific tasks, based on forecasted demand.
Understanding Predictive Staffing Optimization involves recognizing its key components, including data collection, forecasting, and optimization strategies. Effective data collection should focus on key performance indicators and employee metrics that uniquely impact service delivery. Forecasting techniques, such as time series analysis and regression models, help signal future staffing needs. Finally, continuous refinement of staffing strategies ensures that the workforce aligns closely with operational demands, fostering efficiency and productivity across all levels of the organization.
What is Predictive Staffing Optimization?
Predictive Staffing Optimization is a strategic approach that enables organizations to proactively align workforce needs with business demands. It involves analyzing historical data and future projections to ensure the right number of staff is available at the right time, ultimately enhancing productivity and service delivery. By using predictive analytics, companies can anticipate fluctuations in workload, allowing for efficient resource allocation.
Key components of Predictive Staffing Optimization include data collection, forecasting techniques, and continuous monitoring. Organizations begin by gathering relevant data on past staffing levels, customer demand, and operational efficiency. Next, they utilize various forecasting methods to predict future staffing requirements. Additionally, ongoing evaluation ensures that staffing strategies remain effective and adaptable to changing business conditions. Embracing Predictive Staffing Optimization ultimately leads to improved operational efficiency and enhanced employee satisfaction.
- Definition and Importance
Predictive staffing optimization is a strategic approach aimed at aligning workforce needs with anticipated demand. This method not only enhances operational efficiency, but also significantly minimizes costs associated with overstaffing or understaffing. Understanding this concept is crucial for organizations striving to make data-driven staffing decisions in an ever-evolving environment.
The importance of predictive staffing optimization cannot be overstated. By integrating robust forecasting techniques, businesses can identify trends and predict future staffing requirements, ensuring they have the right number of employees at the right time. This proactive approach enables organizations to respond swiftly to fluctuations in demand, thereby improving service quality and customer satisfaction. Additionally, effective optimization fosters a positive work environment by balancing employee workloads, ultimately driving employee engagement and retention.
- Key Components
Key components play a pivotal role in crafting an effective predictive staffing optimization model. To get started, one must focus on three essential elements: data integrity, forecasting accuracy, and stakeholder collaboration. Maintaining high data quality ensures that the staffing model is built on reliable information, which fosters trust in the outputs generated.
Next, accuracy in forecasting is crucial. By employing various forecasting techniques, organizations can anticipate staffing needs effectively. This, in turn, facilitates informed decision-making. Lastly, involving stakeholdersโfrom team leaders to human resourcesโensures that diverse perspectives shape the staffing strategy. Engaged stakeholders can also provide valuable insights into changing operational needs, making the model more adaptable. Collectively, these components work in synergy to enhance the predictive staffing optimization process, ensuring that organizations can efficiently allocate resources and manage workforce demands.
The Role of Forecasting in Staffing
Forecasting serves as the backbone of predictive staffing optimization, enabling organizations to understand future staffing needs accurately. By analyzing historical data and trends, companies can anticipate fluctuations in demand, ensuring that the right number of employees are available when needed. This proactive approach mitigates the risk of overstaffing or understaffing, which can lead to inefficiencies and increased costs.
There are various forecasting techniques that organizations can employ to enhance their staffing processes. Quantitative methods, such as time series analysis, allow for data-driven predictions based on past performance. Meanwhile, qualitative methods, including expert judgment, help incorporate industry insights and trends. Integrating these forecasting techniques into a staffing optimization model not only facilitates better workforce management but also aligns staffing strategies with business objectives, ultimately promoting efficiency and productivity.
- Why Forecasting is Critical
Forecasting plays a pivotal role in the context of Predictive Staffing Optimization, enabling organizations to anticipate workforce demands accurately. By analyzing historical data and identifying trends, businesses can forecast future staffing needs, ensuring that they are adequately prepared to meet customer expectations. This understanding not only enhances operational efficiency but also reduces costs associated with overstaffing or understaffing.
Moreover, effective forecasting allows companies to align their staffing strategies with projected market demands. By employing forecasting techniques, organizations can fine-tune their recruitment efforts, skill development, and workforce allocation. This proactive approach minimizes disruptions and fosters a more agile response to changing circumstances. Ultimately, the significance of forecasting in staffing optimization cannot be overstated, as it is the cornerstone of informed decision-making in today's dynamic work environment.
- Common Forecasting Techniques Used
In the journey towards effective predictive staffing optimization, utilizing reliable forecasting techniques is crucial. Each method serves to enhance decision-making, ensuring staffing levels align with anticipated business needs. Common forecasting techniques often include time series analysis, which identifies patterns based on historical data trends over time. This method is particularly useful when analyzing staffing requirements for regular cycles, like seasonal fluctuations.
Another technique involves regression analysis, which examines relationships between variables, enabling organizations to make informed staffing predictions based on influencing factors. Qualitative methods, such as expert opinions and market research, also play a key role by capturing insights that quantitative data might overlook. Ultimately, a combination of these techniques can create a robust forecasting approach, driving more precise staffing optimization strategies that effectively cater to fluctuating demands. By understanding and applying these techniques, organizations can lay a strong foundation for their forecasting efforts and achieve greater operational efficiency.
Extract insights from interviews, calls, surveys and reviews for insights in minutes
Building a Forecast-Linked Staffing Optimization Model
Building a forecast-linked staffing optimization model begins with a firm understanding of data. Collecting relevant data ensures that you capture essential metrics, which can influence staffing decisions effectively. Utilize tools that not only gather data but also analyze it for trends and patterns that could impact staffing needs.
The next crucial step is developing forecast models tailored to your organization's unique requirements. Choose a forecasting model that aligns with your objectives, whether it be time series analysis or regression modeling. Validate your chosen model by testing it against historical data to ensure accuracy. Once your model is in place, integrate the insights from your forecasts into your staffing strategies. Implement ongoing monitoring and adjustments to refine your approach as circumstances change. This iterative process of predictive staffing optimization will enable your organization to adapt swiftly and efficiently to fluctuating demands while ensuring the right talent is in place when needed.
Step 1: Collecting and Analyzing Data
To effectively build a forecast-linked staffing optimization model, the first step involves collecting and analyzing data. The objective here is to gather comprehensive data that encapsulates various factors affecting staffing needs. Begin by identifying relevant data sources such as historical staffing records, seasonal trends, and external market conditions. This stage sets the foundation for predictive staffing optimization, allowing organizations to understand patterns and trends within their workforce.
Once the data is collected, the next task is meticulous analysis. Utilize tools that can help visualize and interpret this data, transforming raw information into actionable insights. Look for patterns, common themes, and outliers that may exist in employee performance, turnover rates, and customer feedback. By comprehensively analyzing this data, organizations can make informed decisions on staffing requirements, ensuring they align with forecasted needs. This level of detailed analysis is crucial for developing effective future staffing strategies.
- Identifying Relevant Data Sources
Identifying relevant data sources is crucial for creating an effective predictive staffing optimization model. The first step involves assessing both internal and external data reservoirs. Internal sources like historical staffing data, employee performance metrics, and operational logs provide insights into previous patterns and resource allocation. External sources, such as market trends, competitor analysis, and economic indicators, can shape understanding of broader demands impacting staffing requirements.
Once potential data sources are identified, prioritize them based on their relevance and reliability. Carefully evaluate the data collection methods to ensure the accuracy and completeness of the information gathered. Engaging with stakeholders for input on data points can further enhance the model's effectiveness. By integrating various data streams, organizations can cultivate a robust predictive staffing optimization model, which leads to informed decision-making and enhanced operational efficiency. Adopting a comprehensive approach ensures that the staffing model aligns with organizational goals and adapts to evolving needs.
- Tools for Data Collection and Analysis
Data collection and analysis are foundational to building an effective predictive staffing optimization model. Understanding how to gather and interpret relevant data significantly impacts staffing decisions. By utilizing various tools, organizations can streamline the collection processes, ensuring they acquire accurate data that informs their staffing strategies.
Several key tools play a vital role in this endeavor. First, project management platforms allow users to organize and analyze data efficiently, centralizing information for easy access. Second, transcription software can convert audio data into written form, aiding in quick analysis of customer interactions. Finally, data visualization tools can help stakeholders identify trends and patterns, making it easier to draw actionable insights. By combining these resources, businesses can construct a robust framework for predictive staffing optimization, positioning themselves for future success.
Step 2: Developing Forecast Models
Developing effective forecast models is essential for predictive staffing optimization. This process begins by selecting the right forecasting model that aligns with your organizationโs specific needs and objectives. The choice of model can significantly influence the accuracy of workforce projections, thereby impacting staffing decisions.
Once the model is chosen, validating and testing it is crucial. Conducting tests on historical data helps ensure that the model performs reliably when applied to real-world scenarios. By measuring forecast accuracy and adjusting parameters as needed, organizations can refine their approach to staffing. This iterative process fosters a more precise understanding of future staffing requirements, enabling organizations to allocate resources effectively while minimizing operational disruptions. As you proceed through this vital step, remember that strong forecasting only strengthens the foundation of your staffing optimization model.
- Selecting the Right Forecasting Model
Choosing the right forecasting model is a crucial step in predictive staffing optimization. The model you select should align with your specific business needs and the type of data you've collected. Understanding the underlying patterns in your historical data will help you identify which model will provide the most accurate predictions. Common forecasting models include time series analysis, regression models, and machine learning algorithms, each with its strengths and weaknesses.
In addition to understanding the types of models, it's essential to validate and test your chosen method thoroughly. A well-validated model will enhance your ability to predict staffing needs accurately, enabling better resource allocation. By selecting a robust forecasting model, you set the foundation for an effective staffing optimization strategy that can adapt to changing business conditions and demand forecasts. Your choice can significantly influence your organization's agility, efficiency, and overall success in managing workforce planning.
- Validating and Testing the Forecast Model
Validating and testing the forecast model is crucial for ensuring the accuracy and reliability of your staffing optimization approach. First, itโs essential to assess the modelโs predictive performance by comparing your forecasts against actual outcomes. This comparison helps identify any discrepancies, allowing you to fine-tune the model for better precision. Analyzing the errors in predictionsโwhether systematic or randomโwill also provide insights into any patterns that need addressing.
Next, consider conducting sensitivity analyses. Testing how variations in input data impact forecasting results can reveal potential vulnerabilities in the model. Additionally, employing backtesting, where historical data is used to validate the forecast, can enhance confidence in your staffing predictions. By rigorously validating and testing the model, you can create a robust framework for predictive staffing optimization that continually adjusts to new data and changing circumstances, ensuring your staffing strategies remain effective.
Step 3: Implementing the Staffing Optimization Model
To effectively implement the staffing optimization model, begin by integrating predictive staffing optimization into your existing frameworks. This involves aligning forecasting outcomes with your staffing strategies to ensure resource allocation matches anticipated demand. Conduct a thorough analysis of forecast data, identifying gaps and opportunities that can inform staffing decisions. Engaging stakeholders during this phase can enhance buy-in, ensuring that the model is tailored to organizational needs.
Next, establish a continuous monitoring system to evaluate the modelโs performance regularly. This system should track key metrics, such as staffing levels, employee productivity, and customer satisfaction. By analyzing these elements, you'll be able to adjust the staffing optimization model dynamically, adapting to changes in business needs or market conditions. Emphasizing flexibility is crucial for continuous improvement in staffing practices and for achieving operational excellence.
- Integrating the Forecast with Staffing Strategies
Integrating the forecast with staffing strategies is essential for achieving efficient workforce management. By aligning staffing needs with forecasted demand, organizations can ensure they have the right number of employees in place at the right times. This proactive approach helps in avoiding overstaffing and understaffing scenarios, which can be costly and disruptive.
Two primary strategies can enhance this integration process. First, organizations should continually analyze historical performance data to inform future staffing requirements. This analysis helps identify trends and peak periods. Second, it is crucial to foster communication between forecasting and HR teams. These teams must collaborate closely, sharing insights to create a coherent staffing strategy that adapts to changing market conditions. Emphasizing such alignment maximizes the advantages of predictive staffing optimization and builds a resilient workforce strategy.
- Monitoring and Adjusting the Model
Monitoring and adjusting the model is crucial in ensuring that your predictive staffing optimization remains effective and aligned with operational needs. Once the staffing optimization model is implemented, the focus should shift toward continuous monitoring of its performance. This involves regularly analyzing output metrics, comparing them to actual staffing needs, and identifying discrepancies. By actively engaging with these metrics, organizations can uncover patterns and insights that may require adjustments in the model.
Adjustments can be made based on several factors, including shifts in workforce demand, changes in market conditions, or unexpected fluctuations in business activity. Keeping the model updated involves iterative processes, such as refining the forecasting techniques, integrating new data sources, and reassessing staffing strategies. This adaptive approach not only enhances the accuracy of the predictive staffing optimization but also enables organizations to respond proactively to changing circumstances. Through diligent monitoring and timely adjustments, businesses can optimize their staffing resources, leading to improved efficiency and overall performance.
Conclusion: The Future of Predictive Staffing Optimization
The future of predictive staffing optimization lies in its ability to create more responsive and agile workforce strategies. As the demand for skilled labor continues to evolve, organizations must adopt data-driven models that align staffing needs with real-time market conditions. By refining forecasting techniques and enhancing data analysis, businesses can anticipate staffing requirements and make informed decisions.
Implementing predictive staffing optimization not only boosts efficiency but also improves employee satisfaction through better alignment of skills with tasks. Looking ahead, the fusion of artificial intelligence and predictive analytics will further transform staffing practices, leading to a more dynamic approach that meets both organizational goals and employee expectations.