Churn Prevention Analytics is essential for any contact center aiming to enhance customer retention. By analyzing customer interactions, centers can identify patterns that indicate potential churn and take proactive steps to address them. Effective analytics not only help in understanding why customers leave but also provide insights into improving service delivery and customer experience.
Understanding the nuances of customer behavior through churn prevention analytics allows contact centers to tailor their strategies. This targeted approach ensures that resources are utilized efficiently, enhancing customer satisfaction and loyalty. As we delve deeper into this document, we will explore how to build an effective churn prevention engine using Contact Center Business Intelligence (BI), focusing on key metrics, data integration, and actionable insights.
Analyze qualitative data. At Scale.
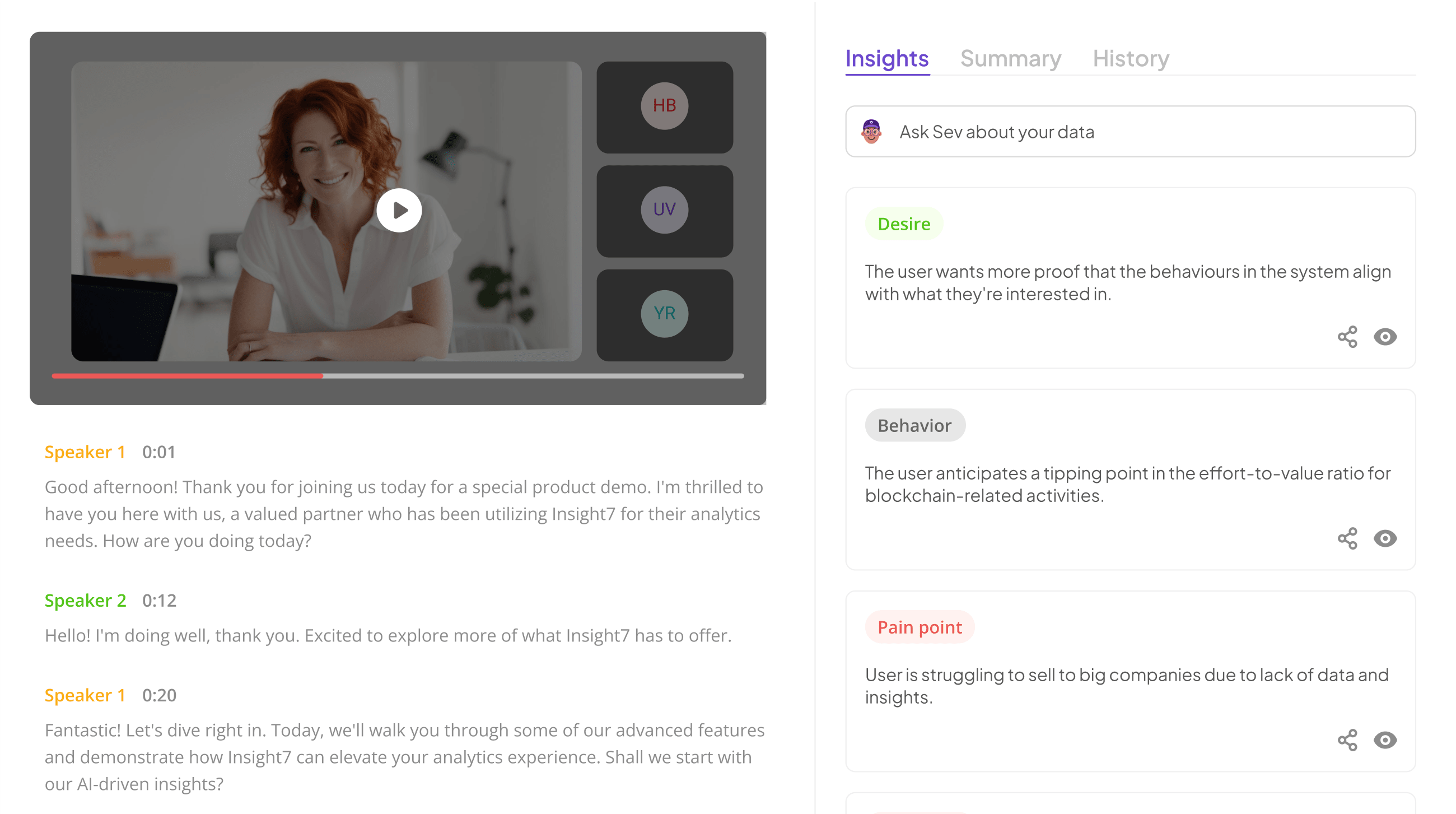
Understanding the Basics of Churn Prevention Analytics
Churn Prevention Analytics focuses on understanding customer behavior to reduce attrition rates. At its core, this discipline involves analyzing data from various customer interactions and identifying factors that contribute to churn. By utilizing advanced analytics, organizations can uncover patterns in customer feedback, service usage, and even agent interactions. This insight serves as a foundation for proactive measures aimed at retaining customers.
To effectively implement churn prevention strategies, itโs essential to understand and collect relevant metrics. Metrics such as customer satisfaction scores, Net Promoter Score (NPS), and service response times are pivotal. Additionally, monitoring customer interactions helps highlight at-risk customers, allowing organizations to address concerns before they lead to churn. Ultimately, mastering Churn Prevention Analytics requires a blend of data-driven insights and proactive engagement to ensure customer loyalty and satisfaction.
The Role of Contact Center BI in Churn Prevention Analytics
Contact Center Business Intelligence (BI) plays a pivotal role in Churn Prevention Analytics by transforming raw data into actionable insights. This process begins with the collection of customer interactions, which include calls, chats, and emails. By analyzing these interactions, businesses can identify recurring issues that contribute to customer dissatisfaction, enabling proactive measures to enhance the customer experience and reduce churn rates.
Moreover, advanced analytics can highlight patterns in customer behavior, helping contact centers understand when and why customers might consider leaving. This insight facilitates targeted interventions, such as personalized offers or improved service training for representatives. By utilizing BI tools effectively, organizations can not only predict churn but also implement strategies that foster customer loyalty, ultimately leading to sustainable growth. Such data-driven approaches integrate well with other operational processes, creating a comprehensive churn prevention engine.
Key Metrics for Churn Prevention Analytics
Key metrics play a vital role in churn prevention analytics, providing insights that can significantly reduce customer attrition. Understanding these metrics allows businesses to identify trends and behaviors that contribute to customer churn. For instance, monitoring customer interaction frequency can reveal whether customers are becoming disengaged, which is a red flag for potential churn.
Additionally, assessing customer satisfaction scores can help gauge how positively customers perceive your service. High satisfaction often correlates with lower churn rates. Metrics such as net promoter score (NPS) and churn rate itself are crucial for evaluating the effectiveness of retention strategies. Keeping a close eye on these metrics allows businesses to make data-driven decisions, enhancing customer experiences and ultimately leading to increased revenue. By focusing on key metrics, organizations can build a robust churn prevention analytics framework that effectively addresses customer needs and fosters loyalty.
Extract insights from interviews, calls, surveys and reviews for insights in minutes
Building a Churn Prevention Engine with Contact Center BI
Developing a Churn Prevention Engine with Contact Center BI requires a strategic approach to data utilization. By focusing on churn prevention analytics, organizations can identify key customer behaviors predictive of churn. This involves collecting and integrating data from various touchpoints in the customer journey, ensuring a comprehensive understanding of customer interactions and preferences.
The next step is data analysis and insights, where patterns and trends emerge. By employing tools such as dashboards, decision-makers can visualize data effectively, making it easier to address potential issues. Predictive modeling plays a crucial role in this process. By employing machine learning algorithms, organizations can forecast customer behavior, allowing them to implement proactive strategies tailored to mitigate churn effectively. This holistic approach fosters a proactive environment where businesses can enhance customer satisfaction and retention.
Step-by-Step Guide to Developing a Churn Prevention Engine
Developing a churn prevention engine requires a systematic, yet adaptable approach to ensure effectiveness. First, start with data collection and integration. This involves gathering diverse data from multiple sources, such as customer interactions and transaction history, and ensuring that this data is centralized within your contact center BI system. Having reliable data is the foundation of churn prevention analytics, enabling you to assess customer behaviors accurately.
Next, focus on data analysis and insights. Utilize robust analytical tools to process the collected data, identifying trends and areas of concern. Automate the reporting process for periodic insights, thus making it easy to track performance metrics. Implementing predictive models follows, where machine learning algorithms can forecast potential churn. By continuously refining these models based on real-time data insights, organizations can proactively engage at-risk customers with tailored retention strategies. This step-by-step approach lays a solid groundwork for an effective churn prevention engine.
Step 1: Data Collection and Integration
To commence building a churn prevention engine, the first step involves meticulous data collection and integration. Gathering comprehensive customer data from various sourcesโlike call transcripts, interaction logs, and feedbackโis essential. This diverse data pool enables a holistic view of customer behaviors and pain points. Itโs crucial to ensure that the data collected is accurate and relevant to effectively inform churn prevention analytics.
Next, integrating this data into a unified system allows for streamlined analysis. Utilize tools that can compile and organize these data sets, creating a central repository for insights. By following this approach, you can identify trends and patterns over time, leading to a better understanding of customer needs and challenges. When executed properly, this foundational step will set the stage for successful predictive modeling and, ultimately, a more effective churn prevention strategy.
Step 2: Data Analysis and Insights
In this stage of building your churn prevention engine, the focus shifts to data analysis and insights. Here, churn prevention analytics plays a pivotal role in understanding customer behaviors and identifying potential churn signals. Begin by organizing data collected from various interactions, such as call transcripts and support requests, into manageable projects. This structured approach allows for deeper analysis, helping to pinpoint key pain points consistently mentioned across customer engagements.
Next, it's essential to distill these conversations into actionable insights. By examining patterns within the data, such as recurring themes and customer feedback, you can generate reports that highlight critical issues. A visual dashboard can further enhance understanding, mapping the customer journey from onboarding to potential advocacy. This comprehensive analysis enables teams to recognize at-risk customers, ensuring timely intervention that could prevent churn and foster stronger customer relationships. Each insight informs strategic decisions that can transform customer support and drive retention.
Step 3: Implementing Predictive Models
Implementing predictive models is critical for building an effective churn prevention engine. This step involves utilizing historical data to forecast customer behavior and identify potential churn risks. By integrating churn prevention analytics into your contact center systems, you can proactively address customer retention issues.
Start by selecting appropriate algorithms that suit your dataโs characteristics. These may include logistic regression, decision trees, or machine learning techniques tailored for customer data. Next, train your chosen model using clean, relevant data to ensure accuracy. Regularly evaluate its performance, adjusting as new data becomes available. This dynamic approach helps in refining the predictive model over time, ultimately making customer interventions more effective. By anticipating customer needs, you can enhance satisfaction and loyalty, leading to a more stable customer base.
Top Tools for Churn Prevention Analytics
To effectively tackle churn prevention analytics, utilizing the right tools is crucial for gaining actionable insights. First on the list is Salesforce Service Cloud, known for its powerful data integration capabilities. This tool helps track customer interactions, making it easier to identify patterns that signal potential churn. Next, Zendesk Explore offers robust reporting capabilities that allow businesses to visualize customer engagement trends and address issues proactively.
Power BI and Tableau further enhance data analysis by providing advanced visualization options, enabling teams to derive deep insights from complex data sets. These intuitive interfaces allow for real-time monitoring and analysis, making it easier to spot trends quickly. Each of these tools contributes significantly to building a robust churn prevention engine, ensuring that companies are well-equipped to understand and mitigate customer attrition proactively. By leveraging these top tools, organizations can enhance their churn prevention analytics effectively.
insight7
To truly understand churn prevention analytics, it's essential to grasp how valuable insights can stem from effective data collection. Data collection and integration serve as the foundation of any churn prevention engine. By ensuring all relevant customer interactions are documented, organizations can construct a comprehensive view of customer behaviors and preferences. This full-picture approach not only supports immediate decision-making but also aids in anticipating future customer needs.
Next, data analysis becomes pivotal. Analyzing this collected data enables businesses to uncover trends linked to customer churn. By employing an adaptable analytics framework, companies can translate raw data into actionable insights. For instance, recognizing patterns in customer inquiries allows teams to proactively address potential issues. Thus, a cycle of continuous improvement and engagement is established, which is critical for an effective churn prevention strategy. With these insights, businesses can craft tailored interventions that significantly enhance customer retention rates.
Salesforce Service Cloud
Salesforce Service Cloud is a powerful platform designed to streamline customer support and enhance engagement, making it instrumental in churn prevention analytics. By leveraging its robust data capabilities, organizations can effectively track customer interactions and identify at-risk accounts. This platform provides insights that enable teams to engage proactively with customers, fostering loyalty and satisfaction.
To effectively utilize Salesforce Service Cloud for churn prevention analytics, organizations should focus on three key elements. First, integrating customer data across all touchpoints ensures a comprehensive view of customer behavior. Next, leveraging automation features can help in managing customer inquiries swiftly, minimizing dissatisfaction. Finally, employing detailed analytics tools provided within the platform can uncover patterns leading to churn, allowing businesses to intervene before it occurs. With these strategies, Salesforce Service Cloud becomes an essential tool for building a robust churn prevention engine.
Zendesk Explore
Zendesk Explore plays a crucial role in the realm of Churn Prevention Analytics. This powerful tool enables businesses to understand customer behaviors and trends through comprehensive data visualization. By simplifying access to insights, Zendesk Explore allows users to deep dive into their contact center operations without requiring advanced technical skills. It democratizes data, ensuring everyone in the organization can contribute to churn prevention efforts.
One of its key features is the ability to analyze customer interactions. Users can explore call transcripts and identify patterns in customer feedback, revealing pain points and areas of satisfaction. This analysis not only aids in understanding current customer sentiments but also helps develop proactive strategies to prevent churn. Ultimately, Zendesk Explore empowers businesses to transform raw data into actionable insights, creating a more resilient customer experience and reducing churn rates.
Power BI
Power BI plays a crucial role in effectively implementing churn prevention analytics within contact centers. This robust tool enables businesses to visualize and analyze data, helping identify patterns and trends in customer behavior. With an intuitive interface, users can easily access reports and dashboards without requiring specialized training, democratizing data insights across the organization.
One of the standout features of Power BI is its ability to integrate diverse data sources. This integration empowers companies to create comprehensive views of customer interactions, making it simpler to pinpoint potential churn risks. By analyzing call transcripts and customer feedback, businesses can extract valuable insights related to customer satisfaction and engagement. Ultimately, utilizing Power BI for churn prevention analytics not only enhances decision-making but also drives better customer experiences, leading to improved retention rates and business success.
Tableau
Tableau serves as a powerful tool for Churn Prevention Analytics, enabling users to create dynamic visualizations from complex data sets. Its user-friendly interface allows even non-technical team members to access and interpret customer insights easily. Through intuitive dashboards, businesses can identify patterns related to customer behavior and potential churn signals.
When utilizing Tableau for churn analysis, focus on three main aspects. First, data visualization helps in pinpointing trends and issues within customer interactions. By visualizing call data and customer feedback, organizations can quickly identify areas needing improvement. Second, Tableau's robust analytical capabilities allow for the correlation of different data sources, revealing deeper insights into customer engagement. Finally, the ability to share dashboards promotes collaboration across teams, ensuring everyone accesses important churn-related metrics. By harnessing these features, companies can enhance their strategy to retain customers and minimize churn.
Conclusion: The Future of Churn Prevention Analytics in Contact Centers
The potential of Churn Prevention Analytics in contact centers is promising as technological advancements continue to reshape the industry. As organizations increasingly focus on customer retention, the integration of data analytics becomes crucial. By harnessing insights from customer interactions, businesses can gain a deeper understanding of dissatisfaction signals and proactively address them.
In the future, utilizing advanced predictive models will enhance the accuracy of identifying at-risk customers. Continuous improvements in data processing and machine learning will allow teams to interpret patterns effectively. Consequently, contact centers will be better equipped to implement targeted strategies, ensuring customer loyalty and satisfaction remain at the forefront of their operations.