Categorical Data Analysis is an essential element in understanding patterns within qualitative data. As we delve into this topic, it becomes clear that this analytical approach unlocks valuable insights, guiding decisions across various fields. Whether youโre examining customer feedback or market trends, recognizing and interpreting categorical data is crucial for informed decision-making.
This section will introduce the foundational concepts of Categorical Data Analysis. We will explore how to classify data into distinct categories, the significance of these categories in research, and the implications they hold for businesses. By mastering this analytical framework, you can approach data with a clearer perspective, enabling more effective strategies and outcomes.
Generate visualizations from your qualitative data. At Scale.
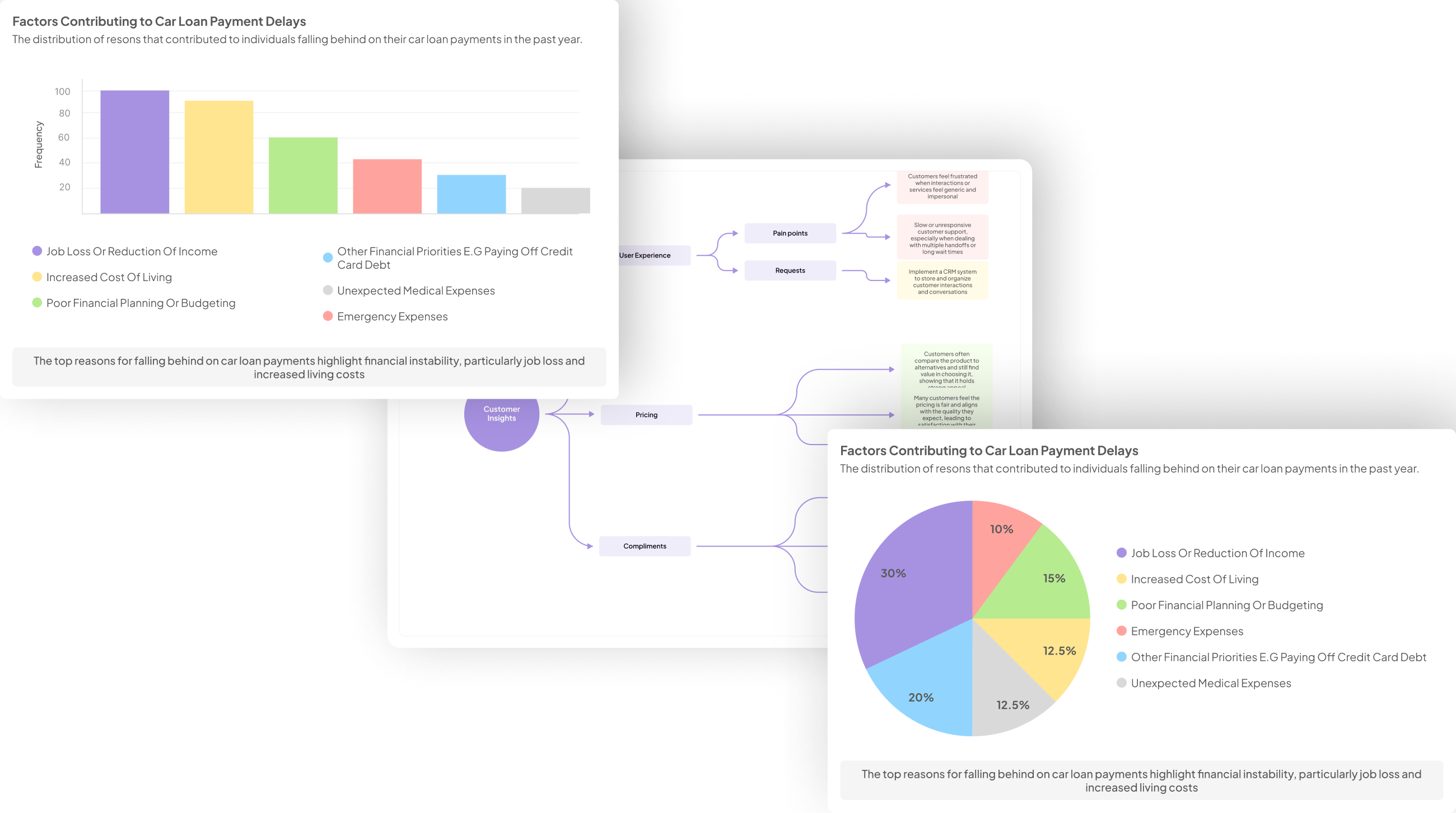
Understanding Categorical Data Analysis
Understanding categorical data analysis is crucial for deciphering how different groups interact within a dataset. This type of analysis focuses on data divided into categories, showcasing the relationships and patterns within those groups. For instance, itโs essential to understand how customer feedback might vary across different demographics or product types, as this can significantly influence decision-making.
Categorical data analysis provides valuable insights in various fields, including market research and social sciences. The key aspects of this analysis include types of categorical data, such as nominal and ordinal data, and their significance in drawing actionable conclusions. Understanding these classifications helps researchers identify trends and capture vital information, which ultimately supports informed strategies and targeted actions. By mastering categorical data analysis, individuals can leverage these insights to enhance their research outcomes.
Types of Categorical Data
Categorical data can be classified into different types, each serving unique analytical purposes. The two primary types are nominal and ordinal data. Nominal data represents distinct categories without any order, such as colors or brand names. In contrast, ordinal data not only categorizes but also ranks items based on their characteristics, like satisfaction levels from "very unsatisfied" to "very satisfied."
Understanding these types is crucial for effective categorical data analysis. Utilizing the correct type determines the appropriate analytical methods, influencing insights derived from the data. For instance, visualizing nominal data may involve pie charts, while ordinal data may suit bar graphs. By distinguishing between these categories, researchers can better interpret results and make informed decisions that drive business strategies and product improvements. Recognizing the structure of your categorical data lays the foundation for successful analysis and valuable insights.
Importance of Categorical Data in Research
Categorical data plays a crucial role in research due to its ability to simplify complex information. By categorizing data, researchers can effectively analyze patterns and trends, enabling clearer insights. This type of data allows for meaningful comparisons across different groups, which is essential for drawing conclusions and making informed decisions.
Understanding the importance of categorical data in research helps in enhancing data analysis techniques. It improves the accuracy of statistical methods employed, ensuring that research findings are robust and reliable. Moreover, categorical data supports the identification of relationships between variables, which can lead to new hypotheses and innovative solutions. Overall, comprehending the significance of categorical data analysis is fundamental for researchers seeking to extract valuable insights from their data.
Generate Journey maps, Mind maps, Bar charts and more from your data in Minutes
Steps in Categorical Data Analysis
The process of Categorical Data Analysis begins with careful data collection and preparation, setting the stage for meaningful insights. First, itโs crucial to gather data that accurately represents your variables of interest. This might involve surveys, interviews, or observational data tailored to capture categorical responses. Once collected, the data needs to be organized, cleaned, and coded appropriately. Clear categorization ensures that your analysis yields reliable results.
Next, descriptive statistics play a vital role in understanding the data. This involves summarizing the categorical variables through frequency distributions or cross-tabulations. By exploring these statistics, you can identify patterns and relationships that may exist within the data. Visualizations, such as bar charts or pie charts, can also enhance comprehension, making it easier to communicate findings effectively. By following these steps, Categorical Data Analysis can reveal valuable insights, driving informed decision-making and strategic action.
Step 1: Data Collection and Preparation
Data collection and preparation form the backbone of any effective categorical data analysis. This phase involves gathering relevant data from various sources, such as surveys, interviews, or existing databases. It is essential to ensure that the data collected is accurate, reliable, and relevant to the questions you aim to answer.
Once youโve gathered the data, the next step is preparation. This process includes cleaning the data, addressing any missing information, and ensuring that it is formatted consistently. Organizing the data into meaningful categories will make the subsequent analysis easier and more insightful. Both these steps are critical because they directly influence the quality of insights you can generate from the analysis. A well-executed data collection and preparation phase lays a strong foundation for revealing patterns and trends in your categorical data. Understanding these nuances is key to conducting effective research.
Step 2: Descriptive Statistics for Categorical Data
Descriptive statistics play a crucial role in Categorical Data Analysis by summarizing and interpreting qualitative data. This step involves methods tailored to capture various attributes of categorical variables, such as frequency counts and proportions. By identifying how many responses fall into specific categories, researchers can unveil patterns that are vital for informed decision-making.
In this process, several key techniques emerge. First, tabulation helps visualize data through frequency tables, allowing for easy comparisons between categories. Second, graphical representations, including bar charts and pie charts, enhance understanding by illustrating the distribution of data. Finally, calculating measures such as mode can provide insights into the most common response. Through these methods, the analysis not only highlights trends but also lays the foundation for further statistical testing.
Conclusion: The Value of Categorical Data Analysis
Categorical Data Analysis plays a vital role in converting diverse data into actionable insights. By analyzing frequency distributions and relationships among categories, businesses can uncover significant patterns that inform decision-making. This process enhances understanding of customer behavior, enabling organizations to tailor their strategies effectively.
The value of Categorical Data Analysis extends beyond mere data examination; it shapes how businesses engage with their audience and improve offerings accordingly. Organizations that embrace this analytical approach are better equipped to navigate market challenges and drive innovation, ultimately leading to more successful outcomes.