Predictive SLA Optimization begins with the integration of advanced AI forecasting engines, which revolutionize how organizations manage Service Level Agreements. These engines leverage vast amounts of data to anticipate service delivery challenges and performance gaps. By analyzing historical trends and current usage patterns, AI significantly enhances the foresight needed for effective SLA planning. This proactive approach not only mitigates risks but also ensures that commitments to clients are met.
Incorporating AI into SLA management allows businesses to adapt quickly to fluctuating demands. With improved accuracy in predictions, organizations can make informed decisions about resource allocation and operational adjustments. Ultimately, this leads to better service quality and stronger client relationships, highlighting the value of predictive SLA optimization in today’s fast-paced market.
Transcribe & extract insights from interviews. At Scale.
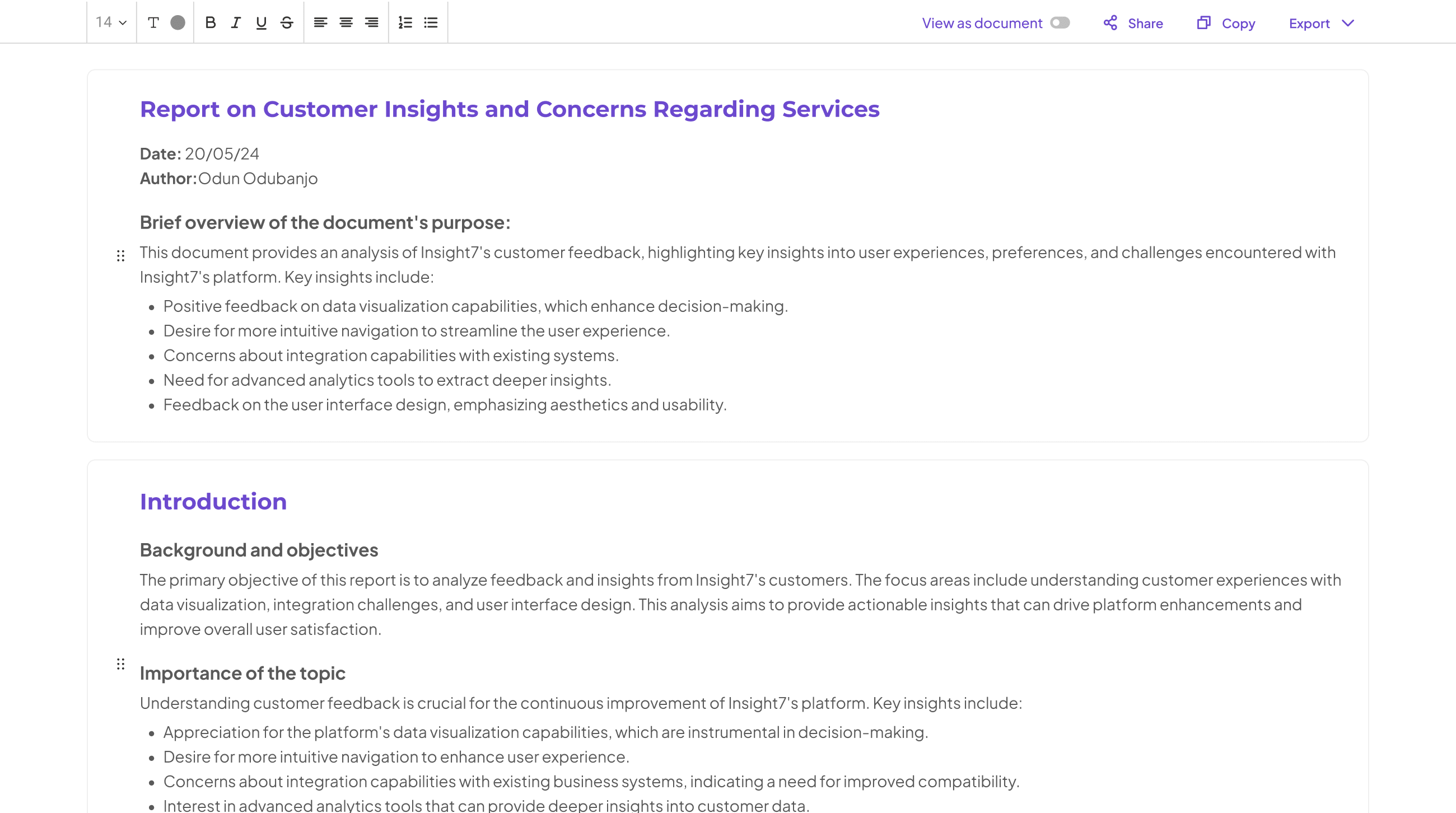
The Role of AI Forecasting Engines in SLA Planning
AI forecasting engines play a vital role in service-level agreement (SLA) planning by offering insights that enhance decision-making. These advanced tools analyze historical data and patterns to predict future performance trends, allowing organizations to optimize their SLAs effectively. By leveraging predictive SLA optimization, businesses can align their offerings with customer expectations and operational capabilities, leading to better satisfaction and adherence to contractual standards.
Furthermore, AI forecasting engines facilitate a proactive approach to SLA management. They enable continuous monitoring and adjustment of service levels based on real-time data. This adaptability minimizes the risks of non-compliance and enhances operational efficiency. Companies can accurately forecast demand fluctuations, allocate resources efficiently, and ensure they meet or exceed service commitments to clients. Ultimately, understanding how AI integrates into SLA planning can significantly impact business relationships and overall service quality.
Understanding Service Level Agreements in Depth
Service Level Agreements (SLAs) are essential contracts that define the expected service quality and performance standards. Understanding SLAs involves recognizing key components such as service scope, performance metrics, and penalties for non-compliance. This knowledge enables businesses to set realistic expectations and measure success accurately.
To enhance SLA planning, organizations can utilize Predictive SLA Optimization, which leverages AI forecasting engines. These engines analyze historical data to predict service outcomes and identify potential bottlenecks. By implementing this predictive approach, businesses can make informed decisions, allocate resources effectively, and minimize risk. Moreover, the collaboration between teams improves, as insights derived from predictive analytics allow for a more cohesive strategy in managing SLAs. Thus, understanding SLAs in depth empowers organizations to achieve better performance and customer satisfaction in an increasingly competitive landscape.
How AI is Transforming SLA Management
Artificial Intelligence is revolutionizing SLA management by enhancing precision and efficiency in performance monitoring. With predictive SLA optimization, organizations can anticipate potential service issues before they arise, thereby improving customer satisfaction. AI forecasting engines analyze historical data and trends to forecast performance metrics and identify potential bottlenecks in service delivery.
This transformation allows for proactive adjustments to be made, ensuring compliance with service agreements. AI’s capabilities streamline data analysis and provide actionable insights that support decision-making processes. By automating task management and communication, AI not only reduces human error but also accelerates response times. As companies transition to these advanced technologies, they are better equipped to meet customer expectations, leading to improved operational effectiveness and strategic advantage in the marketplace.
Predictive SLA Optimization: Enhancing Planning with AI
Predictive SLA Optimization enhances planning by leveraging advanced AI technologies to improve accuracy in service level agreement forecasting. By analyzing historical data and identifying patterns, AI forecasting engines can offer valuable insights into potential future performance and resource allocation. This proactive approach allows organizations to establish realistic goals and respond to client needs more effectively, ultimately enhancing customer satisfaction.
To implement Predictive SLA Optimization, organizations should follow structured steps. First, data collection is essential; this involves gathering relevant historical performance metrics to ensure accuracy. Next, selecting the right model and training it with the collected data helps refine forecasting capabilities. Finally, engaging in forecast analysis leads to informed decision-making, allowing teams to plan strategically. The result is a responsive SLA management process that adapts based on predictive insights, aligning performance with client expectations and streamlining operational efficiency.
Steps to Implement AI Forecasting Engines for SLA Improvement
To effectively implement AI forecasting engines for SLA improvement, organizations should follow a structured approach. The first step is data collection and preprocessing. Collect relevant historical data, ensuring it's clean, organized, and comprehensive. This foundation is crucial for accurate predictive SLA optimization, as quality input data directly influences forecasting accuracy.
Next, model selection and training are essential. Choose appropriate algorithms that align with your specific SLA requirements. Train these models using your preprocessed data, fine-tuning parameters to maximize forecasting performance. This step will help enhance the system's ability to predict future service levels based on historical trends.
Once your models are trained, conduct forecast analysis and decision-making. Evaluate the predictions against your SLA objectives and make necessary adjustments to service strategies and resource allocation. By following these steps, organizations can significantly improve their SLA management and achieve better predictability through AI forecasting engines.
- Step 1: Data Collection and Preprocessing
Data collection and preprocessing serve as foundational steps in enhancing Predictive SLA Optimization through AI forecasting engines. Initially, diverse datasets must be gathered, including historical performance data, customer feedback, and service usage patterns. This rich information is essential for developing accurate forecasting models.
Once collected, preprocessing is crucial to clean and structure the data. This step involves identifying and addressing missing values, filtering out irrelevant information, and standardizing formats to create a cohesive dataset. Successful preprocessing allows the AI system to learn effectively, ensuring that the insights derived from the data are both reliable and actionable.
By meticulously compiling and refining the data, organizations can significantly improve their SLA planning processes. The quality of input data directly impacts the quality of AI predictions, ultimately leading to better decisions and enhanced service delivery.
- Step 2: Model Selection and Training
Model selection plays a crucial role in the process of predictive SLA optimization. During this stage, data scientists assess various algorithms to determine which one will best fit the SLA requirements and forecast patterns related to service delivery. Factors such as the nature of the data, the specific KPIs defined in the SLA, and the historical performance metrics must be taken into account when selecting the model.
After choosing the most suitable model, the next step involves training it on historical SLA data. This training process allows the model to learn from past incidents, ensuring it captures trends and anomalies effectively. Validation of the model against a testing dataset is also essential to confirm its predictive accuracy before deployment. By following these steps, organizations enhance their SLA forecasting capabilities, leading to improved decision-making and resource allocation.
- Step 3: Forecast Analysis and Decision Making
Forecast analysis and decision-making represent critical stages in the journey toward Predictive SLA Optimization. At this juncture, organizations sift through the data collected and interpret predictive insights generated by AI forecasting engines. The outcome is a refined understanding of service demands and potential risks, facilitating informed decision-making.
Effective forecasting not only highlights trends but also recommends actions that align with organizational goals and SLA commitments. Utilizing AI algorithms can enhance this process, allowing teams to simulate various scenarios and their potential impacts on service levels. This ability empowers businesses to address potential service deficiencies proactively while optimizing required resources efficiently. By focusing efforts in these areas, organizations can elevate their SLA planning strategies and foster long-term success.
Key Benefits of AI-Driven SLA Forecasting
AI-driven SLA forecasting transforms how businesses approach service level agreements by enhancing accuracy and efficiency. One of the key benefits of this technology is the ability to perform Predictive SLA Optimization. This method leverages historical data and advanced algorithms to anticipate potential service delivery issues before they arise.
Another critical advantage is improved decision-making capabilities. By analyzing patterns and predicting trends, organizations can proactively adjust their strategies to meet SLA commitments. Enhanced visibility into service performance also allows for timely interventions, ultimately leading to higher customer satisfaction. Moreover, AI can identify resource allocation inefficiencies, ensuring optimal use of staff and technology, which streamlines operations and reduces costs. In a rapidly evolving market, such adaptability is essential for maintaining competitive advantage and ensuring client trust.
Top Tools for Predictive SLA Optimization
In the realm of predictive SLA optimization, various tools stand out for their effectiveness in leveraging AI capabilities. One prominent tool is Insight7, which simplifies the process of analyzing vast amounts of customer data. By facilitating self-service functionalities, it ensures that organizations can efficiently derive actionable insights from their customer conversations. This capability is crucial as traditional methods often fail to keep pace with the growing volume of data generated.
Beyond Insight7, several other advanced AI forecasting tools contribute significantly to SLA planning. IBM Watson Studio excels in enabling robust data analysis and modeling, while Google Cloud AI Platform offers seamless integration with other cloud services for better efficiency. Microsoft Azure Machine Learning provides a user-friendly interface to create and deploy models, enhancing scalability. Finally, Amazon Forecast utilizes machine learning to deliver precise demand forecasts, making it indispensable for effective SLA management. These tools collectively enhance predictive SLA optimization, ensuring that organizations can plan effectively and stay competitive.
Using insight7 for SLA Forecasting
Using insight7 empowers organizations to harness the power of data for SLA forecasting. By leveraging advanced analytics, it transforms raw customer conversations into valuable insights. This process fosters a proactive approach to managing service level agreements, thus enhancing predictive SLA optimization. By identifying patterns and trends in customer interactions, companies can better anticipate needs and allocate resources effectively.
The platform’s self-service capabilities allow businesses to analyze interviews and feedback at scale, ensuring timely access to critical information. This efficiency minimizes the manual efforts traditionally associated with data analysis, enabling teams to focus on strategic decision-making. Additionally, by consolidating insights in one place, collaboration becomes seamless, driving informed actions across the organization. Ultimately, using insight7 streamlines SLA forecasting, paving the way for organizations to stay ahead in a competitive landscape.
Other AI Forecasting Tools for SLA Planning
The utilization of AI forecasting tools for SLA planning has emerged as crucial for businesses aiming to optimize service delivery. Among the prominent tools available, IBM Watson Studio stands out with its advanced machine learning capabilities, allowing users to analyze large datasets efficiently. It caters to a wide range of industries, making it versatile for SLA optimization through predictive analytics.
Another noteworthy tool is the Google Cloud AI Platform, which offers robust tools for data processing, model training, and prediction. Its user-friendly interface and integration with existing data systems simplify the SLA planning process. Microsoft Azure Machine Learning is also a key player, providing end-to-end workflows that help teams create tailored models for monitoring SLA performance.
Lastly, Amazon Forecast leverages deep learning to produce accurate demand forecasts crucial for effective SLA management. These tools highlight how predictive SLA optimization can enhance overall service quality and responsiveness in today's competitive landscape.
- Tool 2: IBM Watson Studio
IBM Watson Studio serves as a powerful tool for predictive SLA optimization, offering a user-friendly interface that democratizes data access across organizations. With its intuitive design, anyone—from analysts to management—can easily create, manage, and analyze data projects without needing extensive expertise. This accessibility fosters collaboration and enables diverse teams to derive actionable insights that lead to improved service level agreements (SLAs).
One of the standout features is its ability to aggregate data from various sources, allowing users to compile extensive project clusters swiftly. This capability not only streamlines the data analysis process but also facilitates the identification of trends and potential pain points in service delivery. Consequently, organizations can make evidence-based decisions that enhance their SLA planning. By utilizing IBM Watson Studio, businesses can effectively harness the power of AI to refine their SLA strategies, ultimately leading to better customer satisfaction and operational efficiency.
- Tool 3: Google Cloud AI Platform
The Google Cloud AI Platform serves as a powerful resource for organizations looking to refine their predictive SLA optimization strategies. This tool simplifies the entire process of integrating AI into SLA management. By providing an intuitive interface, users can easily access functionalities without requiring extensive training or expertise. The platform democratizes the process, enabling all team members to leverage AI capabilities efficiently. This accessibility ensures that valuable insights are extracted to enhance service quality and operational efficiency.
With the cloud platform, businesses can collect large volumes of data, analyze recorded customer interactions, and identify key pain points through AI-driven analytics. The tool consolidates insights from various communication channels, enabling organizations to visualize trends and prioritize customer needs. This comprehensive approach not only streamlines SLA planning but also helps in forecasting potential issues before they arise, reinforcing a proactive rather than reactive strategy in service delivery. Through the use of such tools, companies can significantly elevate their SLA optimization efforts, fostering superior customer experiences.
- Tool 4: Microsoft Azure Machine Learning
Microsoft Azure Machine Learning stands out as an impactful option for optimizing service level agreements (SLAs). By employing its advanced capabilities, organizations can delve into extensive datasets, identifying trends and forecasting demands effectively. This functionality significantly enhances predictive SLA optimization, as businesses can anticipate customer needs with improved accuracy.
The platform offers an array of tools designed for data preprocessing, model training, and analytics. Users can start by integrating their datasets into the system, followed by selecting the most suitable algorithms tailored to their specific needs. As models train on historical data, they generate insights that drive more informed decision-making. Ultimately, the predictive features enhance SLA planning, providing businesses with the ability to adapt swiftly to changing conditions and customer expectations. Through this process, organizations not only streamline their operations but also foster better client relationships by delivering on promises more consistently.
- Tool 5: Amazon Forecast
Amazon Forecast serves as a powerful tool for predictive SLA optimization, integrating advanced machine learning techniques into the forecasting process. This tool allows organizations to accurately predict future service demands by analyzing historical data and identifying trends. By utilizing algorithms that learn from past patterns, it enables businesses to enhance their SLA planning effectively, ensuring they meet their service commitments.
Incorporating Amazon Forecast into SLA management can significantly improve decision-making. The tool provides insights that assist in understanding potential future demands, allowing businesses to allocate resources more efficiently. Moreover, its capability to analyze various data sources means organizations can derive actionable insights that directly align with their SLA objectives. As a result, teams can proactively address potential shortfalls and reinforce their commitment to delivering exceptional service. This proactive approach ultimately leads to improved customer satisfaction and operational reliability.
Conclusion: Embracing AI for Predictive SLA Optimization
The journey towards embracing AI for predictive SLA optimization is both essential and transformative. By integrating advanced forecasting engines, organizations can significantly enhance their SLA planning processes. AI not only streamlines data analysis but also empowers decision-makers to anticipate needs and adjust strategies proactively. This improved foresight is instrumental in maintaining service quality while optimizing resource allocation, ensuring that customer expectations are consistently met.
Moreover, predictive SLA optimization fosters a culture of continuous improvement. Companies are better equipped to adapt to changing demands and market dynamics, leading to enhanced customer satisfaction and loyalty. As organizations continue to explore and implement these AI-driven solutions, the potential to revolutionize SLA management becomes clearer. The future of SLA planning lies in leveraging AI's capabilities to drive efficiency, accuracy, and strategic value.