Predictive SLA Management is transforming how organizations handle service level agreements (SLAs) in the current digital era. By employing AI forecasting, businesses can proactively identify potential violations before they occur, significantly enhancing their operational efficiency. This shift from reactive to proactive management not only mitigates risks but also fosters improved client relationships through reliability and trust.
As AI continues to evolve, harnessing its capabilities for predictive SLA management becomes essential. Understanding data patterns and trends allows organizations to forecast potential issues and plan effectively. Implementing such systems will ensure that service commitments are met consistently, reducing SLA violations and ultimately contributing to better overall service delivery.
Extract insights from Customer & Employee Interviews. At Scale.
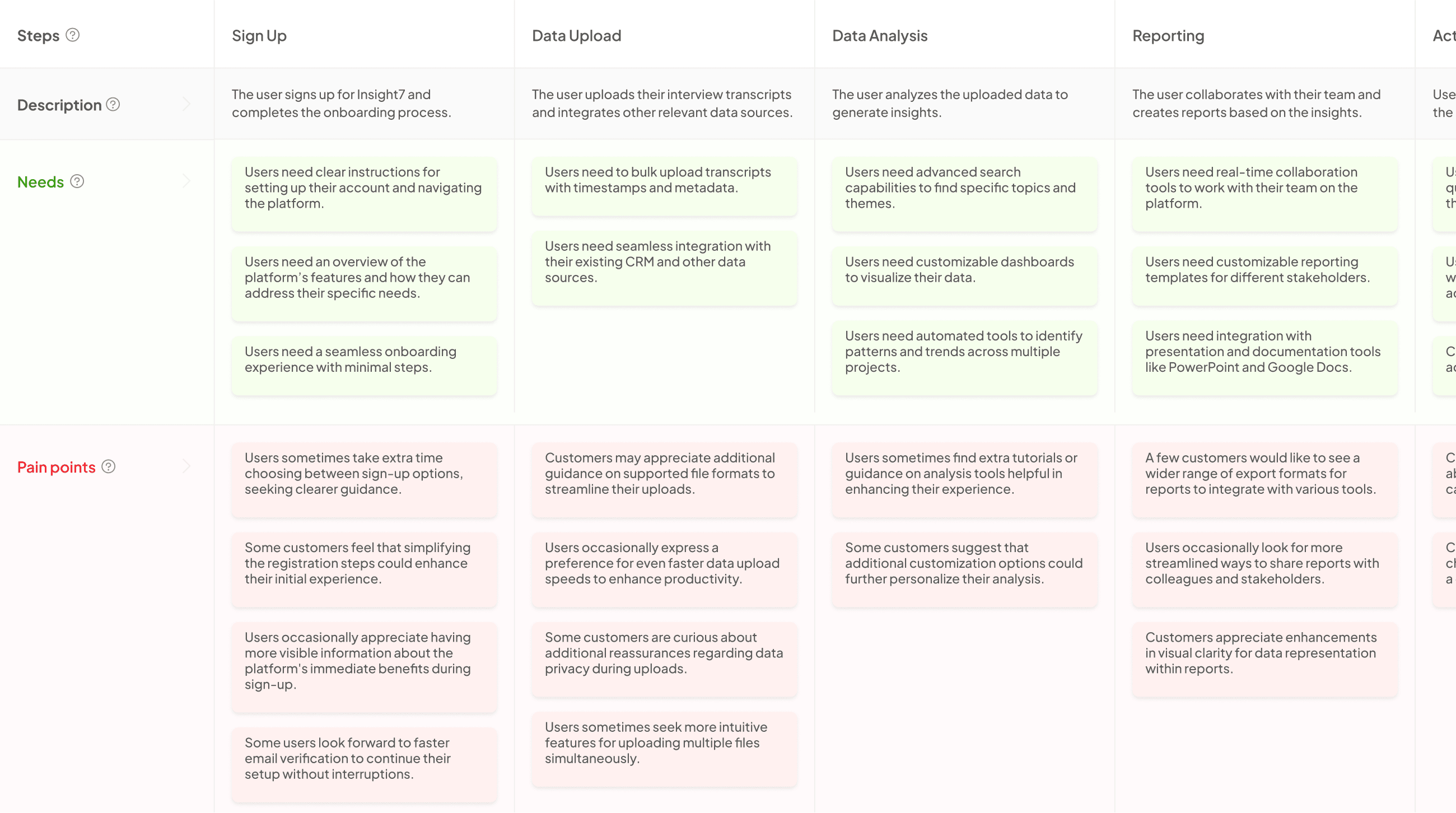
Leveraging Predictive SLA Management for Better Forecasting
Predictive SLA Management plays a pivotal role in improving organizational forecasting capabilities. By harnessing advanced data analysis and machine learning, businesses can anticipate service level agreements (SLA) requirements more accurately. This proactive approach allows organizations to identify potential issues before they escalate into violations, thereby enhancing customer satisfaction.
Implementing Predictive SLA Management involves several critical steps. Firstly, accurate data collection from various sources ensures that relevant metrics are monitored continuously. Real-time analysis helps in recognizing trends and patterns that could affect SLA fulfillment. Next, developing robust AI models for forecasting requires training on historical data, allowing organizations to predict future performance effectively. Finally, continuous improvement through validation and feedback ensures that the predictive models remain aligned with changing conditions, leading to sustained SLA adherence and improved operational efficiency.
Understanding Predictive SLA Management
Predictive SLA Management is a powerful approach that enables organizations to anticipate and mitigate SLA violations. By leveraging AI forecasting, companies can gain insights into potential risks and take proactive measures to ensure service compliance. The foundation of this management style lies in the ability to analyze historical data patterns, which AI technologies can assess effectively.
To implement Predictive SLA Management, several key components should be considered. First, identifying and collecting relevant data is crucial for accurate forecasting. This data forms the basis of AI-driven analysis, allowing for real-time monitoring that highlights fluctuations in service performance. Next, developing robust AI models tailored to specific SLA requirements is essential for forecasting accuracy. Finally, organizations must commit to continuous improvement, ensuring that AI models are regularly updated and refined based on feedback and changing conditions. By following these steps, businesses can better navigate the complexities of service delivery and significantly reduce SLA violations.
- Definition and Importance
Predictive SLA Management is a methodology that focuses on anticipating service level agreement (SLA) violations before they occur. By employing AI forecasting technologies, organizations can proactively identify risks and implement strategies to mitigate potential delays. This approach not only enhances operational efficiency but also significantly improves customer satisfaction by assuring timely service delivery.
The importance of Predictive SLA Management cannot be overstated. With the growing complexity of service delivery chains, businesses face heightened pressure to meet SLAs sustainably. Integrating AI-driven insights facilitates real-time monitoring of key performance indicators, allowing organizations to detect deviations early. Furthermore, the ability to forecast potential violations empowers teams to make informed decisions, ultimately leading to reduced downtime and enhanced service reliability. Overall, adopting this proactive stance is essential for maintaining competitive advantage in today's rapidly evolving market.
- Key Components in AI Forecasting
AI forecasting is driven by several key components that enhance its effectiveness in Predictive SLA Management. First, high-quality data is crucial for making accurate forecasts. Organizations must focus on collecting and analyzing relevant data from diverse sources, including customer interactions and operational metrics. This collected data can unveil patterns and trends, allowing for proactive rather than reactive responses to potential SLA violations.
Another vital component is the development of robust AI models. These models, trained using historical data and industry trends, can predict potential SLA breaches more accurately. Validation processes are equally important to ensure the reliability of these models. Finally, continuous improvement through iterative updates and feedback loops allows organizations to refine their AI forecasting capabilities over time, adapting to changing business environments and customer needs. This holistic approach enables a significant reduction in SLA violations.
Steps to Implement Predictive SLA Management in AI
To implement predictive SLA management effectively, a structured approach is essential. The first step involves data collection and analysis, where businesses must identify relevant data sources and engage in real-time monitoring. By aggregating historical and real-time data, organizations can gain insights into patterns and trends that may affect SLAs. Continuous monitoring ensures that any anomalies are detected early, allowing timely intervention.
Next, model development becomes crucial. AI models should be trained using the identified data, followed by rigorous validation and testing. This process ensures that the models are capable of accurate forecasting and can adapt to a variety of scenarios. Finally, continuous improvement is vital in predictive SLA management. Iterative updates to the models and incorporating feedback are necessary to refine the predictions further. This continuous feedback loop helps organizations remain agile and responsive to changing conditions, ultimately reducing the likelihood of SLA violations.
- Data Collection and Analysis
Data collection begins with identifying relevant sources, which can range from historical transaction data to real-time system alerts. This diverse range of data is crucial for effective predictive SLA management as it provides insights into various operational metrics. Real-time monitoring allows organizations to track SLA adherence continuously, identifying trends that can signal potential violations before they occur.
In conjunction with data collection, analysis plays a vital role in transforming raw data into actionable insights. Advanced analytics techniques, such as predictive modeling, help organizations forecast future SLA performance by analyzing historical patterns. By integrating these insights, businesses can proactively adjust strategies and resources to mitigate the risk of SLA violations, ultimately enhancing customer satisfaction and operational efficiency. Predictive SLA management not only anticipates issues but also fosters a culture of continuous improvement based on data-informed decision-making.
- Identifying Relevant Data Sources
Identifying relevant data sources is crucial for effective predictive SLA management. Businesses can harness diverse types of data to improve their forecasting accuracy and subsequently minimize SLA violations. Start by considering historical performance metrics, customer feedback, and operational logs. Historical data reveals patterns and trends that can inform future behavior. Additionally, customer feedback includes both quantitative ratings and qualitative comments, providing deeper insight into service expectations.
Next, consider the role of real-time data. Integrating live operational data from systems can enhance the forecasting model's responsiveness. This allows organizations to detect potential SLA breaches proactively, enabling timely interventions. Moreover, external factors such as market trends and competitive benchmarks should not be overlooked, as they can significantly impact service delivery. By meticulously selecting and integrating these varied data sources, businesses can refine their predictive models and enhance overall service reliability, ultimately fostering customer satisfaction.
- Real-time Monitoring and Analysis
Real-time monitoring and analysis are essential components in the realm of predictive SLA management. This process enables businesses to track performance metrics as they occur, allowing for immediate adjustments. By analyzing data streams in real-time, organizations can detect anomalies and potential violations before they escalate. This proactive approach not only helps in ensuring compliance with service agreements but also enhances overall operational efficiency.
To effectively utilize real-time monitoring, businesses should focus on several key aspects. First, identifying relevant data sources is crucial; this may include system performance data, customer feedback, and usage patterns. Second, implementing robust analytical tools facilitates deeper insights, enabling teams to visualize trends and pinpoint potential issues swiftly. Lastly, establishing a feedback loop is vital, as ongoing analysis informs necessary adjustments, ensuring that the predictive models remain accurate and effective over time. Embracing these strategies can significantly minimize SLA violations and enhance customer satisfaction.
- Model Development
To effectively implement predictive SLA management, model development plays a crucial role in the overall success of AI forecasting. This involves training AI models specifically designed to analyze historical data and identify patterns that could lead to SLA violations. By utilizing advanced algorithms, organizations can predict potential issues and address them proactively.
The development process consists of several key steps. First, data scientists collect and analyze relevant historical data, ensuring it accurately reflects the operational environment. Next, the model is trained using this data, allowing it to recognize trends and anomalies in service delivery. Validation and testing are essential to confirm the model's accuracy, ensuring that predictions are reliable and actionable. Continuous monitoring and adjustment of the model are vital for adapting to changing circumstances, maintaining effectiveness over time, and ultimately preventing future SLA violations. This iterative process is fundamental in enhancing the organization's ability to meet service commitments.
- Training AI Models for Forecasting
Training AI models for forecasting involves several critical steps that can enhance Predictive SLA Management. Initially, it necessitates rigorous data collection and analysis to ensure the model is grounded in accurate, relevant information. This stage includes identifying essential data sources and leveraging real-time monitoring for comprehensive insights.
Once the data is in place, the next step is model development. This involves crafting algorithms that can identify patterns and forecast potential SLA violations. Each model must be rigorously validated and tested, ensuring it performs reliably across different scenarios.
Additionally, continuous improvement is essential in maintaining the effectiveness of AI models. Iterative updates based on feedback and results from real-world applications allow organizations to refine their forecasting capabilities. In doing so, companies can proactively address potential SLA issues, resulting in minimized violations and enhanced service delivery.
- Validating and Testing Models
Validating and testing models is a crucial aspect of Predictive SLA Management. This step ensures the accuracy and reliability of AI forecasting outputs. By rigorously evaluating the models, organizations can identify any inconsistencies or biases in the predictions. This validation process usually involves comparing the model's forecasts against actual historical data, allowing for recalibration as needed.
To effectively validate and test models, several key techniques can be employed. First, conducting cross-validation helps in understanding how the model performs with different subsets of data. Second, utilizing statistical metrics, such as precision and recall, can provide insights into model effectiveness. Lastly, simulation testing can be valuable for assessing the model's behavior under various scenarios. These approaches not only enhance the modelโs performance but also instill greater confidence in decision-making processes surrounding SLA management.
- Continuous Improvement
Continuous improvement is vital in effectively implementing Predictive SLA Management. This process emphasizes the need for organizations to adapt and refine their forecasting models over time. It begins with iterative model updates, where new data and insights lead to enhanced accuracy in predicting service-level agreements. As trends and conditions change, continuously revisiting forecasting models ensures that they remain aligned with real-world scenarios.
Feedback and adjustment cycles are equally important. Engaging teams in regular assessments of model outcomes can reveal gaps or areas for enhancement. By harnessing customer service data, organizations can assess the effectiveness of their SLA management and make informed adjustments. This thoughtful approach fosters a culture of ongoing improvement, ultimately minimizing SLA violations and enhancing customer satisfaction. Such proactive measures can lead to a more responsive and efficient operational framework, ensuring organizations meet their service commitments effectively.
- Iterative Model Updates
To effectively reduce SLA violations, the iterative model updates play a crucial role. This continuous improvement process involves regularly refining the predictive models based on new data and feedback. By revisiting the model's assumptions and performance outcomes, organizations can better align their forecasts with real-world scenarios, thus enhancing predictive SLA management.
The iterative process can be broken down into several key steps. First, it requires active monitoring of SLA metrics and outcomes to identify trends and deviations. Next, analysts gather feedback from various stakeholders to uncover insights that may influence adjustments. Finally, the model is updated, incorporating this new information to refine forecasts. This cyclical approach not only improves the accuracy of predictions but also fosters a culture of responsiveness and agility. By harnessing iterative model updates, organizations can enhance their AI forecasting capabilities, ultimately leading to decreased SLA violations.
- Feedback and Adjustment Cycles
Feedback and adjustment cycles are essential for refining predictive SLA management strategies continuously. These cycles involve regularly evaluating performance metrics and soliciting feedback from stakeholders. By analyzing real-time data and incorporating user insights, organizations can identify discrepancies between expected and actual service levels. This ongoing assessment allows teams to adjust forecasting models and improve decision-making processes.
The process typically consists of several key steps. First, gather feedback from customer service reports and performance evaluations. Next, analyze this information to pinpoint areas for improvement. Finally, update models based on insights gained, testing them against fresh data to validate their accuracy. This iterative approach ensures that predictive SLA management remains responsive and aligns with organizational goals, ultimately reducing the risk of service level violations. By fostering a culture of continuous improvement, businesses can enhance their operational effectiveness and customer satisfaction.
Generate Journey maps, Mind maps, Bar charts and more from your data in Minutes
Tools and Technologies Enhancing Predictive SLA Management
Various tools and technologies are essential for enhancing predictive SLA management in contemporary business environments. These technologies ensure that organizations can anticipate potential service level agreement violations before they occur. For instance, sophisticated analytics platforms like IBM Watson Analytics provide organizations with real-time insights into their operational data, enabling proactive decision-making.
Another key player in this space is Google Cloud AI, which allows for seamless integration of machine learning models. This can enhance the accuracy of SLA forecasts by analyzing historical data patterns. Additionally, tools like Microsoft Azure AI facilitate comprehensive data analysis, offering user-friendly interfaces that streamline the forecasting process. All these tools contribute to building a robust framework for predictive SLA management, ensuring timely interventions that greatly reduce the risk of SLA violations. By harnessing these technologies, companies can stay ahead of potential pitfalls and optimize their service delivery.
Top Tools in AI Forecasting
In the realm of AI forecasting, several tools stand out for their effectiveness in enhancing predictive SLA management. First up is insight7, which excels in providing data-driven insights through advanced analytics capabilities. Its ability to visualize customer feedback helps organizations understand performance trends, tackling SLA violations proactively.
Next, Google Cloud AI offers a suite of machine learning tools that enable users to build custom models tailored for precise forecasting. Its scalable infrastructure supports real-time analysis, ensuring rapid responses to potential SLA breaches. IBM Watson Analytics follows with powerful predictive modeling features and user-friendly dashboards that simplify complex data interpretation for improved decision-making.
Additionally, Microsoft Azure AI integrates seamlessly with existing systems, allowing businesses to forecast outcomes based on operational data. Lastly, Amazon SageMaker empowers developers to create, train, and deploy machine learning models with ease, specifically focusing on metrics that can predict SLA compliance. These tools collectively contribute to smarter, more effective predictive SLA management, minimizing the risk of service level agreement violations.
- insight7
Predictive SLA Management has emerged as a vital solution for organizations aiming to enhance service delivery and minimize violations. The ability to foresee potential service level breaches allows companies to proactively address issues before they escalate. By analyzing historical data and identifying patterns, organizations can better understand client needs and tailor their services accordingly.
In this context, AI forecasting plays a crucial role in predictive SLA management. It facilitates real-time monitoring, allowing teams to respond swiftly to emerging challenges. Furthermore, effective model development ensures that AI tools are refined for accuracy, adapting to changes in environments and client behaviors. Continuous feedback loops improve the forecasting models, ensuring they remain relevant and effective. Organizations that adopt predictive SLA management benefit from improved operations, satisfied customers, and reduced risks associated with service delivery failures.
- Key Features and Benefits
The integration of Predictive SLA Management within AI forecasting offers significant advantages. First and foremost, it enhances the accuracy of forecasts, enabling organizations to anticipate potential service-level agreement (SLA) violations more effectively. By analyzing historical data patterns and trends, businesses can identify risk areas and take proactive measures to mitigate them. This predictive capability transforms the approach to managing SLAs from reactive to proactive.
Another key benefit is the democratization of insights across the organization. With user-friendly interfaces, team members can independently access analysis tools, gaining instant insights without extensive training. This fosters a culture of data-driven decision-making, where employees can generate reports and understand customer experiences seamlessly. Furthermore, real-time monitoring of performance metrics ensures that any deviations from SLA standards are promptly addressed, resulting in improved service reliability and customer satisfaction. By utilizing Predictive SLA Management, organizations position themselves to not only meet but exceed their SLA commitments.
- Google Cloud AI
Google Cloud AI offers a powerful platform for harnessing machine learning and artificial intelligence, particularly in the realm of Predictive SLA Management. Organizations can utilize its advanced algorithms and tools to analyze vast amounts of data, identifying patterns that could indicate potential SLA violations. This foresight enables teams to proactively address issues before they escalate, ultimately safeguarding service quality and customer satisfaction.
When implementing Google Cloud AI for predictive analysis, it's essential to follow a structured approach. Start with data collection from relevant sources, followed by real-time monitoring to ensure accuracy. Next, the insights gathered are utilized to develop and train AI models tailored to specific scenarios. Finally, the process involves continuous improvement, where regular feedback loops help refine the forecasting models. By integrating Google Cloud AI into operations, businesses can significantly reduce SLA violations and enhance overall service delivery.
- Introduction to Tool
Predictive SLA Management serves as a powerful tool in the landscape of AI forecasting. It aims to anticipate compliance challenges before they develop into violations. By leveraging data-driven insights, it empowers organizations to optimize their service delivery and improve customer satisfaction.
This tool democratizes access to critical information, allowing all team members to engage with the data. Users can easily analyze conversations, identify customer pain points, and summarize insights without needing extensive training. With a user-friendly interface, Predictive SLA Management turns complex data into actionable strategies, driving efficiency and compliance throughout the organization.
- Unique Capabilities
Unique capabilities of AI forecasting can significantly improve Predictive SLA Management. First, AI systems can analyze vast amounts of data rapidly. This allows businesses to identify patterns in service performance that may lead to SLA violations. By examining historical data and current trends, predictive models can forecast potential violations, enabling proactive measures.
Second, AI forecasting can offer real-time insights and alerts. These notifications can inform teams of impending issues, allowing businesses to intervene before breaches occur. This continuous monitoring is crucial for maintaining compliance and satisfaction levels. Additionally, by using machine learning algorithms, predictive models can adapt over time, updating their forecasts based on new data inputs, ensuring accuracy. Lastly, AI-generated reports can provide valuable insights for decision-makers, helping them understand their operational strengths and weaknesses. This enhances planning and resource allocation, ultimately reducing the risk of SLA violations.
- IBM Watson Analytics
IBM Watson Analytics serves as a powerful tool in the realm of Predictive SLA Management, helping organizations anticipate and mitigate potential SLA violations. With its robust data visualization and analysis capabilities, it enables users to uncover insights from extensive datasets. This insight-driven approach can highlight trends, customer feedback, and performance metrics essential for proactive decision-making.
The platform empowers teams to analyze historical data, monitor real-time performance, and predict future SLA-related challenges. By integrating with various data sources, it enhances the ability to view both positive and negative customer sentiments, allowing organizations to address issues swiftly. For instance, businesses can compare SLA performance across different locations or segments, identifying patterns that could lead to potential violations. This level of granularity in data analysis not only enhances operational efficiency but ultimately supports a culture of continuous improvement.
- Overview of Functions
In the realm of predictive SLA management, understanding the functions of AI forecasting becomes essential for effective service level agreements. AI-driven forecasting enhances decision-making by analyzing vast data sets and providing insights into potential SLA violations. The primary function of this approach lies in its ability to identify patterns and trends, which enables organizations to preemptively tackle issues before they escalate.
Furthermore, predictive SLA management incorporates continuous data monitoring and model refinement. This dynamic process ensures that the forecasting models adapt to changes in operational conditions. By leveraging real-time data, companies can respond swiftly to challenges, thereby reducing SLA violations significantly. The integration of these functions allows for a proactive approach, transforming how organizations manage their SLAs and ultimately delivering improved customer satisfaction. Understanding these core functions lays the groundwork for successfully implementing AI forecasting in any operational environment.
- Implementation Examples
In exploring the implementation of Predictive SLA Management, several noteworthy examples can illuminate its efficacy in reducing SLA violations. One example involves utilizing AI-driven tools to analyze customer interactions in real time, thereby identifying patterns that could lead to delays. For instance, when evaluating customer service calls, specific templates can provide insights into compliance and service quality. By adjusting these templates based on predictive data, teams can proactively address issues before they escalate.
Another implementation example entails combining data collection with AI model development to foresee potential service disruptions. Organizations might adopt continuous monitoring practices to gauge performance against established metrics. If anomalies occur, the system triggers alerts, allowing teams to make informed adjustments swiftly. This proactive stance enhances customer satisfaction and bolsters compliance, ultimately minimizing SLA breaches. Through these examples, it becomes clear that integrating Predictive SLA Management into operations significantly strengthens overall service reliability.
- Microsoft Azure AI
Microsoft Azure AI offers valuable features that enhance predictive SLA management, allowing businesses to minimize service level agreement violations. By utilizing advanced machine learning algorithms, organizations can analyze vast amounts of data, enabling proactive decision-making and optimized resource allocation. This greatly aids in forecasting potential SLA breaches before they occur, allowing for timely interventions.
The integration of Azure AI into a predictive SLA management strategy involves several key functionalities. First, it offers dynamic performance dashboards that provide real-time insights into system health and traffic patterns. Next, machine learning models analyze historical data to identify trends and predict future outcomes. Finally, the platform's versatility ensures that businesses can scale their solutions according to demands, offering a robust toolkit for managing SLAs effectively. Overall, Azure AI streamlines forecasting and significantly contributes to reducing the risk of SLA violations.
- Features and Integration
Incorporating Predictive SLA Management requires careful attention to features and integration. Various tools provide distinct features that enhance forecasting capabilities. Utilizing real-time monitoring allows organizations to track service levels closely and make informed decisions proactively. Additionally, integrating AI models with existing IT infrastructure can optimize resource allocation, ensuring timely responses to potential SLA violations.
The integration of predictive analytics enhances collaboration across departments. For instance, seamless data flow between IT and customer service can lead to quicker resolutions, decreasing violation risks. Furthermore, ongoing feedback loops enable continuous improvement of forecasting models, adapting them to evolving business needs. By ensuring these features work harmoniously, organizations can effectively mitigate the risk of SLA violations and bolster overall service quality.
- User Experience Insights
Incorporating user experience insights is crucial for successful predictive SLA management. The effectiveness of AI forecasting to prevent SLA violations begins with understanding user interactions and needs. When teams analyze customer feedback, they can identify pain points and improve service delivery. This proactive approach not only addresses potential disruptions but also enhances overall satisfaction.
To effectively leverage user experience insights, consider the following elements. First, gather comprehensive data through user interviews and feedback loops. This direct input helps in understanding user expectations. Second, implement structured analysis of the collected data to draw actionable insights. Lastly, regularly update teams on these findings to align service offerings with user needs effectively. By fostering a culture of transparency and responsiveness, organizations can significantly reduce SLA violations and enhance client relationships through predictive SLA management.
- Amazon SageMaker
Amazon SageMaker is a powerful tool that enhances predictive SLA management, enabling organizations to manage and optimize their service level agreements effectively. This cloud-based machine learning platform allows users to build, train, and deploy predictive models quickly. By automating the model development process, businesses can focus on interpreting insights rather than getting bogged down in the complexities of data processing.
One of the key advantages of using Amazon SageMaker is its ability to integrate with various data sources and existing workflows. It enables real-time monitoring and analysis of SLA-related data, empowering organizations to predict potential violations before they occur. Furthermore, success stories abound, as companies have successfully reduced SLA violations through the deployment of predictive models developed on SageMaker, demonstrating its practical application and effectiveness in real-world scenarios. Ultimately, this platform supports organizations in creating proactive strategies around SLA management, minimizing risks associated with service delivery.
- How it Supports Predictive SLA
Predictive SLA Management plays a crucial role in shaping how organizations forecast and mitigate service level agreement (SLA) violations. At its core, it leverages advanced data analytics and machine learning to predict and address potential issues before they escalate. By analyzing historical data patterns, organizations can identify trends that lead to SLA breaches. This predictive approach allows teams to proactively manage resources and timelines more effectively.
Additionally, implementing a predictive SLA framework fosters continuous communication across teams. By utilizing real-time monitoring and alerts, organizations can swiftly adjust to any deviations from the agreed service standards. Such adjustments not only enhance efficiency but also significantly improve customer satisfaction. As a result, the overall outcome of adopting this forward-thinking methodology is a more reliable service delivery process, ultimately ensuring that SLAs are consistently met, thus reducing the likelihood of violations.
- Success Stories
In recent applications of predictive SLA management, organizations have witnessed remarkable success in reducing SLA violations. One notable case involved an e-commerce company that implemented AI forecasting tools. By analyzing customer data and order patterns, they predicted potential delivery delays and proactively addressed them. This approach not only streamlined operations but also significantly enhanced customer satisfaction.
Another effective example can be found in the telecommunications sector. A service provider adopted AI-driven analytics to monitor service level metrics in real time. The system enabled them to anticipate spikes in usage and effectively allocate resources, resulting in fewer service interruptions. These success stories highlight the transformative impact of predictive SLA management, showcasing its ability to create more efficient processes and foster better relationships with customers. Ultimately, these advancements pave the way for companies to not only meet, but exceed customer expectations in service delivery.
Conclusion: Achieving Reduced SLA Violations Through Predictive SLA Management
Predictive SLA Management plays a vital role in minimizing service level agreement violations. By forecasting potential risks and performance issues, organizations can proactively address them before they escalate. This approach transforms reactive strategies into proactive ones, fostering an environment where compliance is maintained diligently.
Moreover, integrating predictive analytics enables consistent performance monitoring, ensuring that service delivery aligns with established benchmarks. Ultimately, the success of Predictive SLA Management hinges on leveraging data-driven insights, allowing for timely interventions that reduce SLA violations and enhance overall service quality. This strategic shift not only improves customer satisfaction but also builds long-term trust in service providers.