AI-Driven Conversation Classification is transforming the way businesses understand and engage with their customers. In an era where vast amounts of customer data are generated daily, traditional analysis methods often fall short. Businesses are seeking efficient ways to extract actionable insights from customer conversations. AI technology steps in as a powerful solution to automate this classification process, enabling organizations to rapidly decipher the nuances of customer feedback and inquiries.
By employing sophisticated algorithms, AI can categorize interactions seamlessly, allowing teams to identify patterns and prioritize responses. This not only enhances the efficiency of customer service but also fosters a deeper understanding of customer needs. Organizations that harness AI-Driven Conversation Classification will be better equipped to adapt their strategies, ensuring they remain competitive in a fast-paced market.
Analyze qualitative data. At Scale.
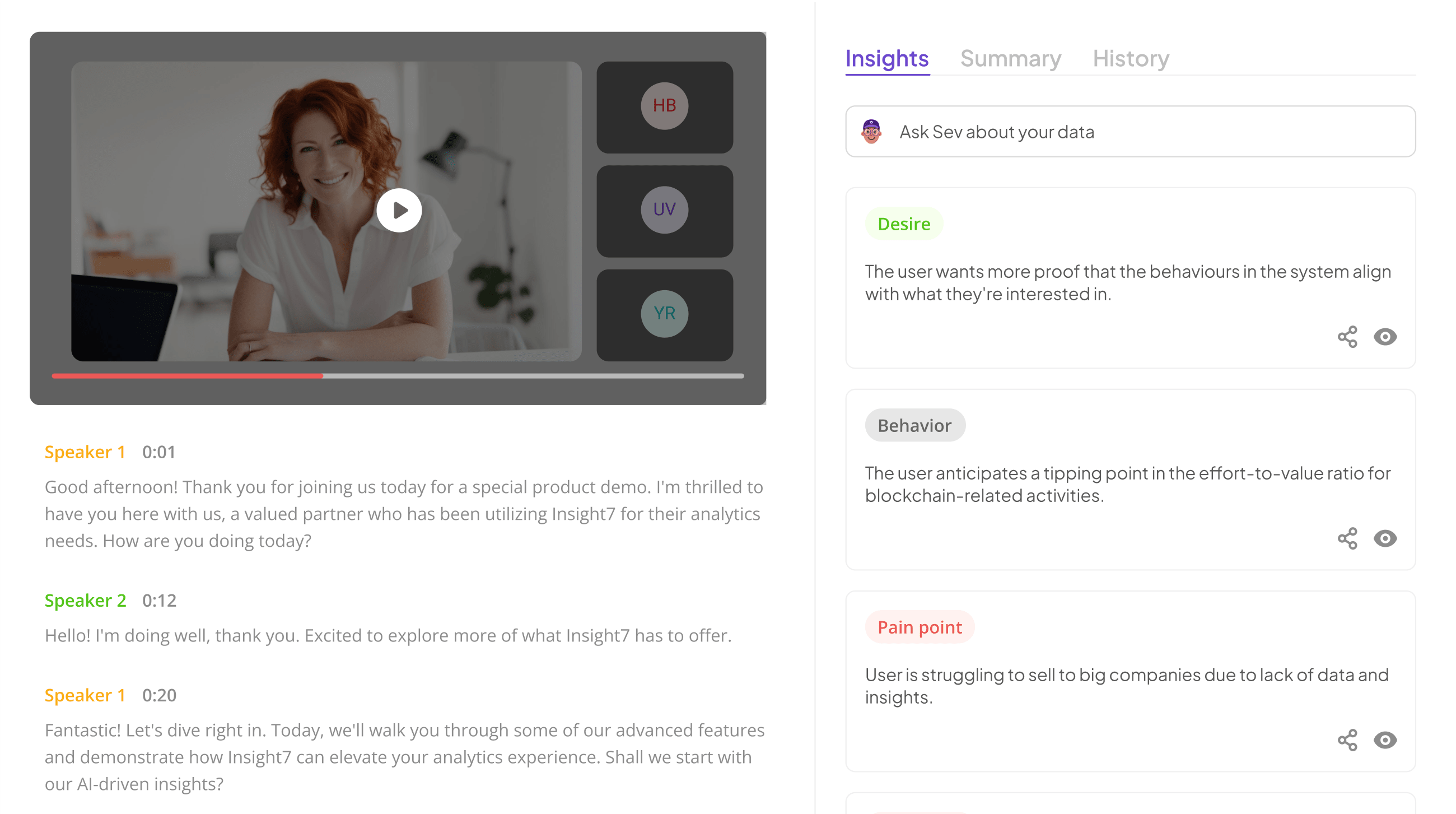
Understanding AI-Driven Conversation Classification
AI-Driven Conversation Classification offers powerful insights into customer interactions during interviews. By utilizing advanced algorithms, businesses can analyze conversations to efficiently categorize various types of discussions, including feedback, complaints, and inquiries. This classification process allows organizations to identify patterns and trends, which helps in improving service and addressing customer needs effectively.
The technology works by analyzing spoken words and transcribing them into actionable data. It can recognize individual speakers, gauge sentiment, and summarize key points within the conversation, ensuring that no valuable information is overlooked. Through AI-driven classification, companies can generate reports that highlight performance metrics and engagement levels across their customer interactions. This capability not only enhances organizational efficiency but also leads to a more tailored customer experience, resulting in increased satisfaction and loyalty.
The Role of AI in Customer Interviews
AI-Driven Conversation Classification plays a pivotal role in refining the customer interview process. By leveraging machine learning algorithms, businesses can now analyze customer conversations at scale and gain invaluable insights. This classification system not only categorizes interactions but also identifies key themes, enhancing the overall understanding of customer sentiment and needs. With AI's ability to process vast amounts of data quickly, companies can respond more effectively to customer inquiries and adapt their strategies accordingly.
Moreover, AI aids in identifying patterns that would be difficult to discern manually. It streamlines the conversation analysis, making it much more efficient and less time-consuming. This efficiency transforms customer interviews from a daunting task into a manageable process, allowing teams to focus on developing actionable insights. Ultimately, AI-driven solutions empower organizations to stay ahead in an increasingly competitive landscape by ensuring that customer needs are promptly addressed.
- Defining Customer Interviews
Customer interviews serve as a vital touchpoint in understanding user perspectives and gathering actionable insights. These interviews are structured conversations where businesses ask customers about their experiences and needs, enabling a deeper understanding of their expectations. By focusing on key areas such as customer pain points and product usability, companies can effectively navigate the landscape of consumer feedback.
AI-Driven Conversation Classification enhances this process by automating the analysis of conversations, saving time and improving accuracy. With comprehensive data collected through customer interviews, AI systems can classify conversation types, highlighting themes and trends that might be overlooked in traditional analysis. Furthermore, this classification helps teams identify the most pressing issues and opportunities for improvement, ultimately leading to better business strategies. By utilizing AI, businesses can transform raw customer interactions into valuable insights that inform future decisions.
- How AI Enhances Interview Processes
AI-Driven Conversation Classification plays a transformative role in enhancing the efficiency of interview processes. By employing advanced algorithms, AI can swiftly analyze conversations and provide real-time insights. This not only saves valuable time but also allows for a more objective evaluation of each interaction. Additionally, AI can identify various conversation types, such as engagement or discovery, streamlining the process for interviewers.
Furthermore, AI enhances interview processes by offering a detailed performance overview of team members. Each representative can receive personalized feedback based on their conversation metrics, enabling continuous improvement. The ability to generate scorecards and reports quickly also aids compliance officers in maintaining oversight. With AI automating these aspects, interviewers can focus on refining strategies and delivering better experiences for customers. In essence, AI makes the interview process more insightful and efficient, ultimately leading to higher-quality interactions.
Steps to Implementing AI-Driven Conversation Classification
Implementing AI-Driven Conversation Classification involves a systematic approach that enhances customer interviews by categorizing conversations effectively. The first step is data collection. Gather diverse and representative audio recordings of customer interviews to ensure the model can learn from various conversation types. This initial dataset is crucial as it lays the foundation for training the AI system.
The second step is training the machine learning model. Using the collected data, apply algorithms that allow the AI to identify and learn patterns in conversation types. This phase may take time, so patience is essential. Finally, deploy the classification model in real-time. This implementation allows the system to classify conversations on the fly, providing timely feedback and insights.
By following these steps, businesses can effectively harness AI-driven conversation classification to improve their customer interview processes and gain deeper insights into customer needs and preferences.
- Step 1: Data Collection
Data collection serves as the foundation for AI-driven conversation classification in customer interviews. Start by gathering a diverse range of interview transcripts, ensuring they represent various conversation types and customer sentiments. The breadth of data is crucial, as it enables AI algorithms to learn from multiple perspectives and interaction styles. A comprehensive dataset aids in identifying trends and classifications effectively.
Next, consider the context and content of each conversation. Group the data into categories such as feedback, inquiries, and complaints. This organization aids in training machine learning models more efficiently. Ensure that the collection process aligns with ethical guidelines, maintaining customer privacy throughout. By implementing these steps, you enhance the quality of insights extracted from the data, facilitating accurate AI-driven analysis and classification in future conversations.
- Step 2: Machine Learning Model Training
Machine learning model training is a crucial step in the process of AI-driven conversation classification. At this stage, the goal is to equip the algorithm with the necessary capabilities to comprehend and categorize diverse conversation types from customer interviews. First, data prepared from previous analyses must be split into training and validation sets. The training set helps the model learn patterns associated with specific conversation types, while the validation set allows for testing its accuracy and effectiveness.
Secondly, the training process involves feeding the model numerous labeled examples. These examples serve as references, enabling the machine learning system to identify key features and attributes that define various conversation categories. As the model trains, it fine-tunes its parameters to optimize classification results. Ultimately, a well-trained model will distinguish between different conversation types with enhanced precision, benefiting businesses seeking to analyze customer insights effectively.
- Step 3: Real-Time Classification Deployment
In Step 3: Real-Time Classification Deployment, the focus shifts to integrating AI-Driven Conversation Classification into customer interactions in a seamless manner. This phase emphasizes the necessity of immediate feedback during customer interviews. By utilizing AI, businesses can process and analyze conversations as they happen, swiftly categorizing them into various types, such as inquiries, complaints, or feedback.
To implement real-time classification effectively, several key actions are involved. First, ensure that the AI system is fully integrated with your communication tools to capture live interactions. Second, the system should be capable of adapting classifications dynamically based on evolving conversation contexts. Third, provide training for team members, enabling them to understand and act on the real-time insights derived from AI analysis. This deployment not only enhances customer engagement but also empowers organizations to refine their strategies instantly, aligning their services more closely with customer needs.
Extract insights from interviews, calls, surveys and reviews for insights in minutes
Key Tools for AI-Driven Conversation Classification
In the realm of AI-Driven Conversation Classification, several key tools can significantly enhance the analysis of customer interviews. These tools utilize advanced algorithms to sift through vast amounts of conversation data and extract meaningful insights. They facilitate a more efficient process than traditional methods, making it easier to identify conversation types and themes effectively.
Among these tools, Insight7 stands out with its self-service platform designed to analyze data at scale. It allows users to manage and streamline customer interactions seamlessly. Other notable tools include MonkeyLearn, which offers customizable text analysis, IBM Watson providing robust natural language processing capabilities, Salesforce Einstein for integrated customer insights, and Google Cloud Natural Language that excels in sentiment analysis. Each tool contributes uniquely to the classification of conversations, enhancing decision-making through actionable insights derived from customer feedback.
insight7
AI-Driven Conversation Classification plays a crucial role in refining how businesses understand customer interviews. This process involves employing advanced algorithms to sift through vast amounts of conversational data efficiently. By analyzing discussions in real-time, businesses can swiftly identify themes, sentiments, and actionable insights that enhance decision-making.
Understanding various conversation types is vital for tailoring customer engagement strategies. For instance, some conversations may indicate customer needs or pain points, while others may reveal potential upsell opportunities. Successfully categorizing these interactions enables companies to prioritize responses and align their efforts with customer expectations. As businesses increasingly rely on automated tools, they can stay ahead by swiftly transforming insights into meaningful actions. By adopting AI-driven solutions, organizations can foster better collaboration, ensuring that critical insights are easily accessible and actionable across teams.
- Overview of Insight7 Capabilities
Insight7 Capabilities provide robust tools designed to enhance customer interview processes through AI-Driven Conversation Classification. This capability allows users to categorize conversations accurately, separating them into distinct types such as feedback, inquiries, and testimonials. By leveraging natural language processing (NLP) and machine learning algorithms, users can automate the classification process, saving time and improving analysis accuracy.
Moreover, Insight7 fosters a seamless experience by integrating with various data sources, making it convenient to collect and analyze customer interactions. Real-time classification enables businesses to act quickly on insights, transforming conversations into actionable strategies. This functionality not only streamlines workflow but also offers deeper insights into customer sentiments and trends. Ultimately, Insight7โs capabilities empower organizations to elevate their understanding of customer needs and drive better decision-making.
- How Insight7 Stands Out
In the realm of AI-driven conversation classification, identifying the unique capabilities of various platforms is essential. What sets one solution apart is its ability to empower users to analyze vast amounts of data quickly and efficiently. For instance, offering a self-service platform ensures that businesses have full control over their customer insights without the cumbersome process of manual analysis. This flexibility allows companies to react promptly to insights, leading to better decision-making.
Moreover, the ability to seamlessly integrate conversation data from multiple sources enhances collaboration. It eliminates the disorganization typically associated with scattered insights. As a result, organizations can focus on actionable strategies derived from these classified conversations. By prioritizing user experience, this platform not only accelerates analysis but also positions businesses to stay ahead of their competitors in understanding customer needs. Ultimately, the capability to classify conversations effectively turns valuable insights into real business actions.
Other Notable Tools
Various solutions in the market provide additional capabilities for AI-Driven Conversation Classification, each catering to different user needs and preferences. For instance, MonkeyLearn offers an intuitive interface for text analysis, making it convenient for teams unfamiliar with complex algorithms. It excels in providing customizable machine learning models, which helps organizations classify conversation types efficiently.
IBM Watson, on the other hand, leverages advanced natural language processing to analyze customer interactions deeply. It can recognize sentiments, intentions, and trends within conversations, adding a valuable layer of insight that assists in strategic decision-making. Salesforce Einstein integrates seamlessly into existing CRM systems, allowing businesses to automate insights generation within their customer relationship platforms. Finally, Google Cloud Natural Language focuses on extracting nuanced details from conversations, enabling teams to assess overall customer sentiment and engagement effortlessly. Each of these tools plays a pivotal role, enhancing how organizations can harness AI for better understanding of customer interactions.
- MonkeyLearn
MonkeyLearn serves as an essential tool in the realm of AI-driven conversation classification, transforming the way businesses interpret customer interviews. By employing natural language processing, it streamlines the classification of conversation types, ensuring that key insights do not get lost in dialogue. Utilizing its capabilities, organizations can effortlessly categorize discussions into distinct types, thereby enhancing their understanding of customer sentiments and needs.
This platform allows users to customize and train models according to specific business requirements. The ease of use coupled with powerful algorithms empowers businesses to make data-driven decisions. Moreover, its visual interface ensures that even those with limited technical expertise can navigate through the complexities of conversation analysis. As businesses adapt to increasing volumes of interview data, AI-driven conversation classification becomes indispensable in extracting actionable insights. This allows companies to refine their strategies and foster deeper customer relationships.
- IBM Watson
AI-Driven Conversation Classification employs advanced technology to categorize and analyze customer interactions effectively. Utilizing sophisticated algorithms, the system can dissect conversations into meaningful segments, such as engagement and discovery. This classification aids businesses in understanding customer needs and improving the overall interview process.
In a competitive environment, accuracy becomes paramount. High precision allows organizations to generate actionable insights swiftly, allowing for timely interventions and enhancements. The process typically involves training a machine learning model with relevant data, which can subsequently classify new conversations with remarkable efficiency. This not only streamlines the feedback process but also enables compliance officers to deliver concise reports to clients. As the landscape of customer interactions evolves, AI-driven solutions provide an innovative approach to understanding and improving engagement in customer interviews.
- Salesforce Einstein
Salesforce Einstein plays a pivotal role in AI-Driven Conversation Classification, streamlining how organizations classify and analyze customer interviews. This tool utilizes advanced natural language processing to identify conversation types efficiently. By analyzing speech patterns and keywords, it enhances the understanding of customer sentiments and needs, providing valuable insights into customer interactions.
With the ability to learn from past conversations, Salesforce Einstein dynamically improves its classification accuracy over time. This ensures that organizations can adapt to evolving customer expectations, refining their engagement strategies accordingly. By automating the classification process, companies can save time and allocate resources more effectively. Ultimately, the integration of such AI technologies empowers businesses to transform raw data from customer interviews into actionable insights, driving improved customer relationships and business outcomes.
- Google Cloud Natural Language
AI-Driven Conversation Classification significantly benefits from advanced tools designed to analyze and interpret language effectively. Google Cloud Natural Language stands out as a powerful solution in this space. Its comprehensive text analysis capabilities allow businesses to extract valuable insights from customer interactions. By understanding sentiment, entity recognition, and syntax, companies can gain a deep understanding of the conversations taking place during customer interviews.
When integrating this tool, organizations can enhance the quality of their customer insights. The AI analyzes raw transcription from conversations, enabling businesses to classify them based on themes and emotional tone. This classification helps refine strategies for customer interaction, transforming traditional methods into a more consultative approach, where understanding the customerโs needs becomes paramount. By employing AI-driven features within this platform, businesses can facilitate real-time feedback and support continuous improvement in their sales and support practices.
Conclusion: The Future of AI-Driven Conversation Classification in Customer Interactions
As organizations look towards the future, AI-Driven Conversation Classification will play a vital role in shaping customer interactions. By automating the classification of conversation types, businesses can enhance their understanding of customer needs and preferences. This technology not only streamlines the analysis process but also enables companies to respond quickly to insights, making informed decisions that can lead to competitive advantages.
In an increasingly data-driven world, the effectiveness of customer interactions hinges on the ability to accurately interpret vast amounts of conversational data. Future advancements in AI will allow for more precise classifications, fostering richer customer relationships and promoting targeted engagement strategies. As businesses embrace AI-driven solutions, they will unlock new opportunities for growth and innovation, setting a new standard for customer experience.