AI-Powered QA Enhancement marks a significant leap forward in the realm of quality assurance. As businesses increasingly demand reliable outcomes, traditional evaluation methods are no longer sufficient. AI technologies offer innovative ways to analyze data at scale, ensuring that QA processes are streamlined and effective.
Incorporating AI into QA provides tools to evaluate calls against predefined criteria, resulting in actionable insights. Organizations can customize evaluation templates for various scenarios, from compliance checks to customer service assessments. This shift not only enhances the accuracy of evaluations but also empowers teams to focus on strategic improvements, ultimately future-proofing their quality assurance models.
Analyze qualitative data. At Scale.
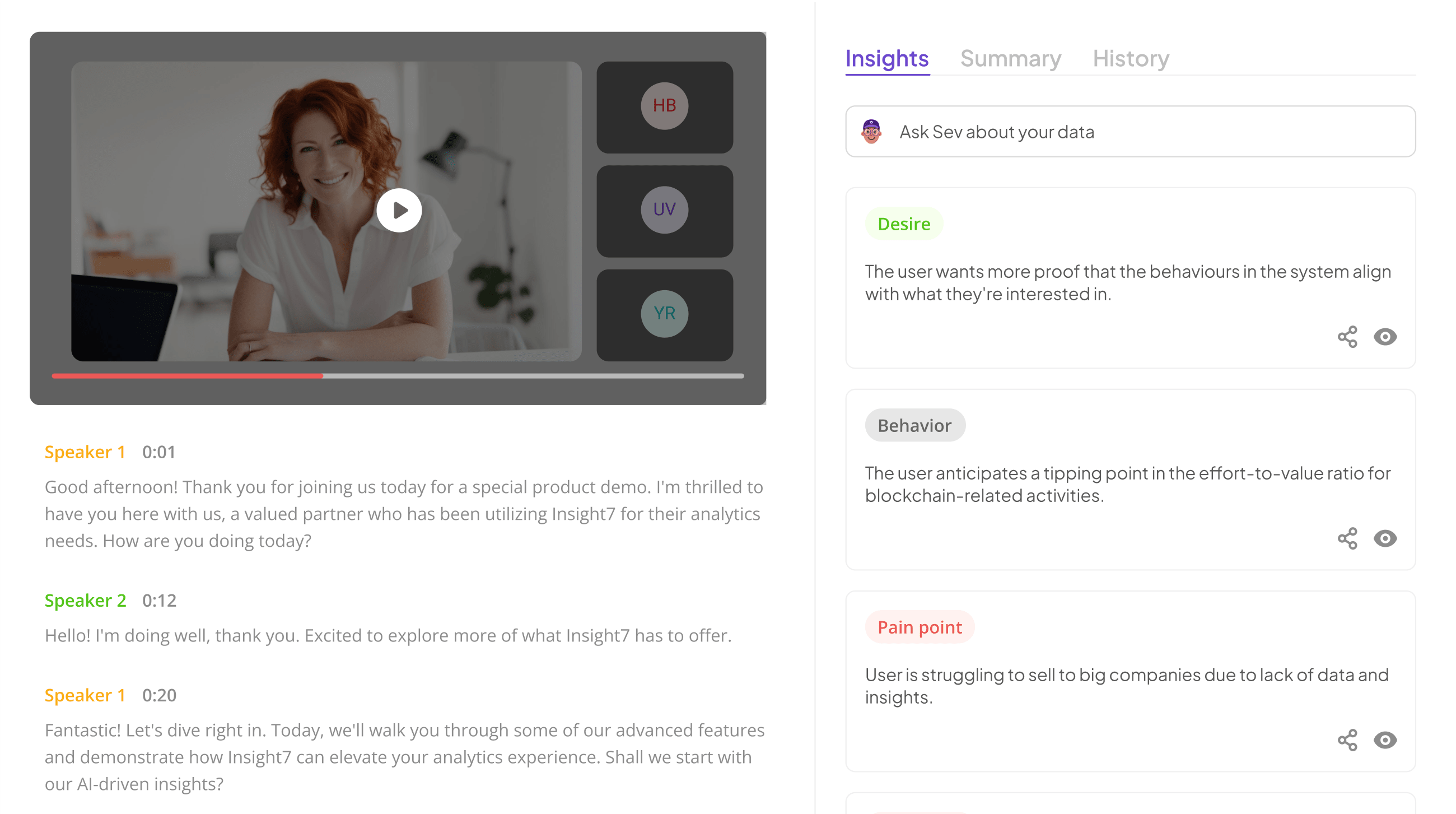
The Evolving Landscape of QA
Quality assurance has undergone significant changes as technology and customer expectations evolve. AI-powered QA enhancement plays a pivotal role in this transformation by enabling organizations to analyze vast amounts of data more effectively. Traditional QA methods are often too slow to keep up with the fast-paced demands of modern businesses. Companies must now rethink their approaches to quality assurance in order to utilize customer insights efficiently and stay competitive.
As businesses generate increasing volumes of data and customer interactions, the need for sophisticated AI evaluation systems becomes essential. These systems can automate processes, improve accuracy, and provide actionable insights from customer conversations. By adapting to these advancements, organizations can ensure that their quality assurance models remain relevant and capable of delivering high standards of service. This evolution signifies not just a change in tools but also a fundamental shift in how organizations prioritize and understand quality assurance.
The Role of AI in Modern Quality Assurance
In today's rapidly changing technological environment, the role of AI in modern quality assurance (QA) cannot be overstated. AI-powered QA enhancement transforms traditional testing methods, introducing efficiency and precision that were previously unattainable. By automating repetitive tasks, machine learning algorithms can detect patterns and anomalies faster and more accurately than human testers. This shift enables QA teams to focus on more complex challenges, ensuring software quality is not just maintained but continuously improved.
Additionally, incorporating AI-driven evaluation systems provides real-time analytics and insights into quality processes. This capability allows organizations to identify areas needing enhancement and adjust strategies accordingly. AI's ability to analyze vast datasets enables predictive insights, which helps preemptively address issues before they escalate. In summary, embracing AI in QA empowers teams to future-proof their processes by creating a robust framework that prioritizes quality and user satisfaction in a competitive marketplace.
AI-Powered QA Enhancement Methods
AI-powered QA enhancement methods fundamentally transform the quality assurance process. By integrating advanced AI-driven evaluation systems, organizations can enhance the accuracy and efficiency of their QA efforts. These innovative methods utilize machine learning algorithms to analyze data and provide real-time insights, ultimately improving decision-making processes.
To effectively embrace these AI-powered QA enhancement methods, organizations should begin by assessing their current QA processes. This evaluation will identify areas for improvement and help determine the most suitable AI tools. Once appropriate tools are selected, implementation and rigorous testing are essential to ensure seamless integration. This structured approach not only enhances QA models but also contributes to more responsive and dynamic quality assurance in the future. Ultimately, AI-driven systems empower teams to deliver superior performance and maintain high standards in their QA practices.
Embracing AI-Driven Evaluation Systems
Adopting AI-Driven Evaluation Systems is an essential step toward enhancing quality assurance practices. These systems harness the power of artificial intelligence to analyze and evaluate performance, making improvements more precise and effective. They allow organizations to set clear performance standards and assess adherence to these metrics, creating a structured environment for continuous enhancement.
The implementation of AI-Powered QA Enhancement can streamline the evaluation process significantly. By effectively capturing data, organizations can identify trends and areas needing attention. For instance, AI tools can analyze call recordings, providing insights into customer interactions and helping teams refine their approach. Moreover, this technology can reduce human error and bias, leading to more reliable evaluations across various scenarios. Through embracing AI-driven solutions, businesses can ensure they not only meet current quality standards but also adapt to evolving demands in the market.
Steps to Integrate AI-Powered QA Enhancement Tools
To successfully integrate AI-powered QA enhancement tools, begin by assessing your current QA processes. This includes a thorough evaluation of existing workflows, techniques, and outcomes. Understanding these elements is crucial for recognizing gaps and areas needing improvement. Gaining insights here allows teams to set clear goals for AI implementation.
Next, choose suitable AI tools that align with your specific requirements. Evaluate features like scalability, ease of use, and compatibility with existing systems. The right tools should enhance your QA processes seamlessly, with intuitive interfaces for all team members.
Finally, implement and test the selected AI systems. Run pilot programs to gauge effectiveness and gather feedback to refine the approach. An iterative testing phase ensures that the tools meet the desired objectives while allowing for necessary adjustments. By following these steps, organizations can effectively harness AI-powered QA enhancement, ensuring models remain robust and future-ready.
- Step 1: Assess Current QA Processes
Begin by evaluating your current QA processes critically. Understanding where your existing practices stand is essential for implementing AI-powered QA enhancement effectively. Examine the steps your team currently takes, identifying bottlenecks and inefficiencies that may hinder performance. This assessment should include gathering insights from team members, assessing existing tools, and reviewing common pain points.
Once you've mapped out your current QA procedures, focus on identifying specific areas for improvement. Look for repetitive tasks or processes that lack consistent evaluation metrics. This step sets a solid foundation for integrating AI-driven evaluation systems. By identifying areas where AI can add the most value, you can ensure a smoother transition toward enhanced QA practices. With a clear understanding of your current landscape, you'll be better equipped to leverage AI technologies effectively to optimize performance and quality.
- Step 2: Choose Suitable AI Tools
Selecting the appropriate AI tools is crucial for effective AI-powered QA enhancement. Begin by identifying your specific QA needs and objectives. Consider the functions you want these tools to perform, such as automation, reporting, or predictive analysis. The right tool can transform your quality assurance processes and streamline evaluation methods. Therefore, thorough research on available tools is essential, focusing on compatibility with your existing systems and user-friendliness.
Next, evaluate potential tools based on their capability to analyze data efficiently and provide actionable insights. Testing these tools on smaller projects can offer a clearer picture of their performance. Additionally, consider factors such as costs, scalability, and the level of support provided by the vendors. By taking a strategic approach in choosing AI tools, you will enhance your QA processes robustly, ensuring long-term viability in an ever-evolving landscape.
- Step 3: Implement and Test AI Systems
Implementing AI systems for Quality Assurance (QA) requires a structured approach that emphasizes testing and optimization. After selecting suitable AI tools, it is essential to launch pilot projects that simulate real-world scenarios. This step will allow you to collect actionable data and evaluate the effectiveness of AI-powered QA enhancement in practice. Testing should include diverse datasets, enabling comprehensive assessments of the systemโs performance.
Monitoring outcomes during the testing phase is crucial. Analyzing the AI's decision-making processes will provide insights into potential areas for improvement. Teams should focus on user feedback, which can reveal how well the AI systems integrate with existing workflows. Finally, iterating on your AI implementation based on test results will ensure the systems remain effective and relevant as quality demands evolve over time. By committing to these steps, organizations can significantly bolster their QA processes.
Extract insights from interviews, calls, surveys and reviews for insights in minutes
Top AI Tools for QA Enhancement
Artificial Intelligence has transformed Quality Assurance (QA) by introducing innovative tools that significantly enhance evaluation processes. These AI-powered QA enhancement tools improve consistency, speed, and accuracy, allowing teams to identify errors and streamline workflows efficiently. With advancements in natural language processing and machine learning, AI-driven solutions are essential for modern QA strategies, providing comprehensive analytics and insights that manual methods often overlook.
Among the leading tools are Insight7, IBM Watson, Testim, Applitools, and Mabl. Insight7 stands out with its robust analytics capabilities, enabling teams to gain actionable insights quickly. IBM Watson offers extensive AI functionalities, making it versatile for varied QA requirements. Testim excels in automating testing scenarios, allowing for rapid deployment. Applitools is renowned for its visual testing features, ensuring pixel-perfect results, while Mabl integrates seamlessly with CI/CD pipelines to enhance workflow efficiency. These tools collectively empower organizations to achieve consistent quality and stay ahead in an increasingly competitive landscape.
insight7
The integration of AI-Powered QA Enhancement in evaluation systems significantly transforms the quality assurance process. Many organizations struggle with managing customer data and insights. Traditional methods often fall short in meeting the demands of scalable analysis. The crucial first step involves assessing current QA processes to pinpoint inefficiencies and areas for improvement.
Organizations then need to select suitable AI tools tailored to their specific needs. This could encompass tools that automate data collection and analysis, ensuring that insights can be generated rapidly and accurately. Finally, implementing and testing these AI systems will establish a solid foundation for future growth. By embracing AI-driven methodologies, companies can streamline collaboration, convert insights into actionable strategies, and ultimately stay ahead in a competitive market. The journey of evolution in QA is ongoing, but AI holds the key to its future.
IBM Watson
With the rapid advancements in artificial intelligence, an innovative tool has emerged that significantly enhances quality assurance processes. This tool is capable of analyzing large datasets to provide insights that were previously difficult to obtain. By utilizing machine learning algorithms, it can identify patterns and pinpoint areas for improvement in quality assurance workflows. This capability allows teams to streamline their evaluations and dramatically improve their overall efficiency.
The AI-Powered QA Enhancement offered by this system allows organizations to make data-driven decisions on their quality assurance methods. Its ability to generate comprehensive reports in a matter of minutes helps quality assurance teams stay agile and responsive. By integrating this powerful AI-driven evaluation system, companies can ensure they remain ahead of the curve in maintaining quality standards while minimizing the risk of human error in their assessments. This intelligent approach signifies a transformative shift towards more effective and efficient quality assurance practices.
Testim
Testim represents a vital component in the journey towards AI-Powered QA Enhancement. It provides a seamless platform for automated testing, making QA processes more efficient and reliable. The introduction of such tools allows teams to implement testing solutions that minimize manual errors and improve overall accuracy. By harnessing AI, Testim enables users to adapt to changing requirements, ensuring that quality standards are not just met but exceeded.
Incorporating Testim into your QA framework transforms the testing experience. Its user-friendly interface combined with advanced analytics offers insights that are actionable. This not only speeds up the testing process but also supports teams in identifying potential challenges early. As businesses look to future-proof their QA models, Testim stands out as an essential ally, integrating effortlessly into existing workflows while enhancing quality assurance with robust, AI-driven evaluations.
Applitools
Utilizing advanced AI techniques allows for a significant enhancement in quality assurance processes. AI-Powered QA Enhancement streamlines evaluation methodologies, transforming traditional QA practices into efficient, data-driven systems. By pragmatically analyzing transcripts against established criteria, organizations can ensure compliance and capture insights that were previously elusive.
The application of AI algorithms simplifies the identification of customer concerns and effectively evaluates performance against predetermined metrics. With fundamental criteria in placeโspanning areas such as problem identification and resolution processesโAI systems can systematically assess interactions and feedback. Such evaluations not only improve operational efficiency but also enhance the overall customer experience. As organizations increasingly rely on AI-driven solutions, they position themselves at the forefront of innovation, ultimately future-proofing their QA models against emerging challenges.
Mabl
Mabl offers an innovative solution for organizations looking to enhance their quality assurance processes. By leveraging AI technology, Mabl streamlines test automation, making it easier for teams to maintain high standards of software quality. This method transforms the way testing is approached, allowing for rapid adjustments and continuous improvement.
The platform's AI-Powered QA Enhancement features are designed to adapt to changing requirements, ensuring that testing is as efficient and effective as possible. With its intelligent system, Mabl allows teams to focus on critical testing needs rather than mundane tasks. As organizations face increasing demands for faster releases, the use of such adaptive tools can be pivotal in achieving long-term success in quality assurance. Embracing this technology empowers teams to stay agile and responsive in a constantly evolving software environment.
Conclusion: Future-Proofing QA with AI-Powered QA Enhancement
In conclusion, the integration of AI-Powered QA Enhancement marks a significant evolution in the quality assurance landscape. By employing these advanced systems, organizations can transform their QA processes, ensuring high standards and timely evaluations. This proactive approach not only safeguards the integrity of products and services but also fosters a culture of continuous improvement in quality assurance.
Looking ahead, embracing AI in QA will be essential in navigating the complexities of future challenges. Companies that adopt AI-Powered QA Enhancement will benefit from more accurate insights and efficient workflows, enabling them to stay competitive. As technology evolves, adapting quality assurance strategies with AI will be crucial for long-term success.