As the landscape of research continues to evolve, the advent of AI-generated data introduces intricate ethical concerns. Introduction to AI Data Ethics in Research necessitates a critical examination of how data is sourced, used, and interpreted in academic contexts. Researchers are tasked with navigating uncharted territories, balancing innovative exploration with responsibility towards participants and society.
AI Data Ethics encompasses pivotal issues like privacy, bias, and accountability. Questions arise about how data is collected and whether it accurately represents diverse populations. Ensuring fair representation and protecting individual rights becomes essential in fostering trust within research communities. Ultimately, establishing ethical standards guides researchers towards integrity and accountability, reinforcing the importance of responsible practices in this digital age.
Analyze qualitative data. At Scale.
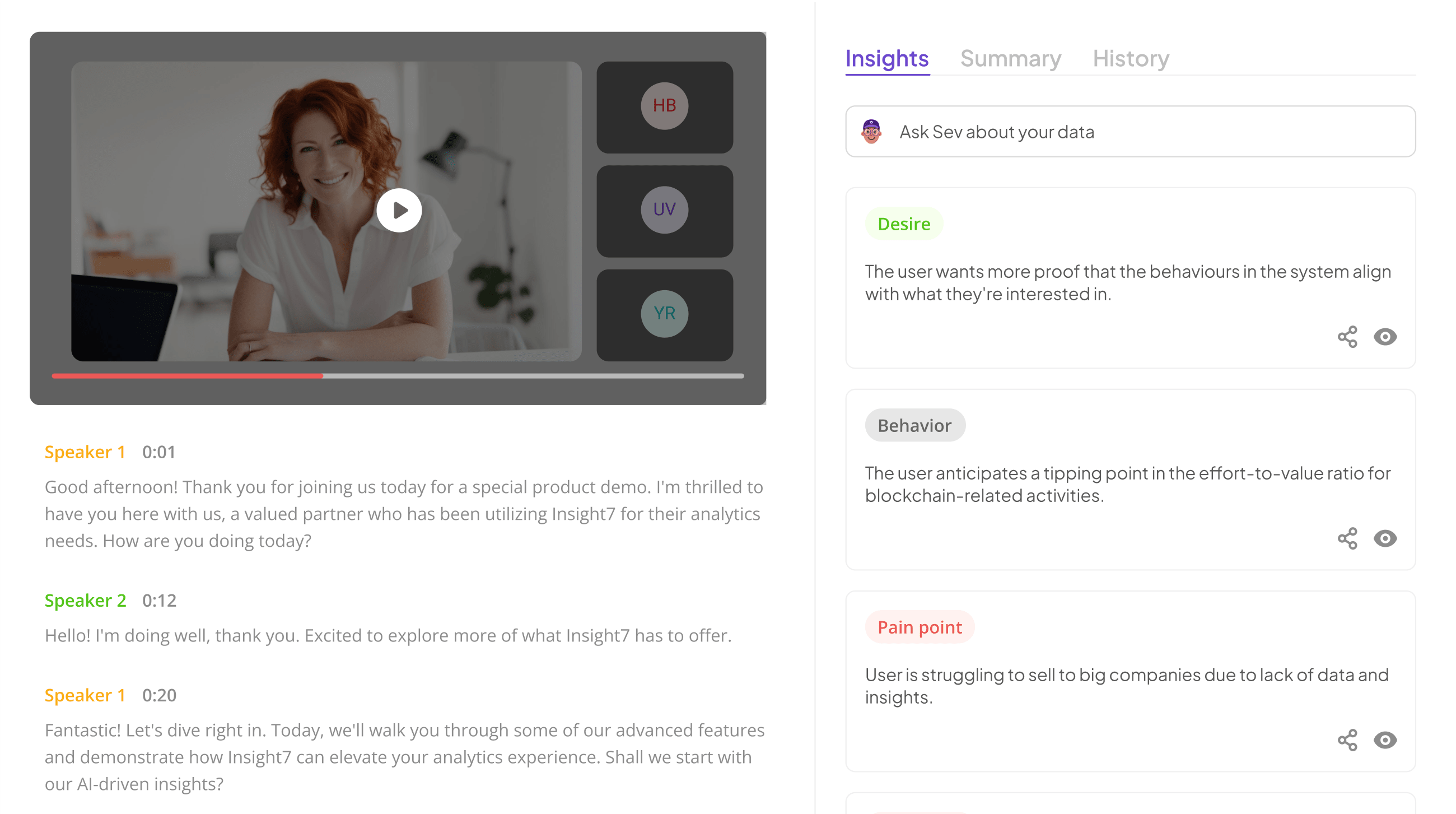
Ethical Concerns Surrounding AI Data Ethics in Research
Ethical concerns surrounding AI Data Ethics in research are vital to ensuring responsible usage of AI-generated information. One significant issue is the potential invasion of privacy. AI systems often require vast amounts of data, which can include sensitive personal information. Researchers must prioritize safeguarding this data to maintain trust and comply with privacy regulations.
Another critical aspect is bias in AI-generated research data. AI can inadvertently perpetuate existing biases present in the training datasets. This leads to outcomes that may unfairly favor certain groups over others, compromising fairness. Therefore, understanding and mitigating these biases is essential for researchers. Through careful scrutiny of data sources and the development of algorithms, researchers can foster an equitable research environment that respects AI Data Ethics. Balancing innovation and ethical responsibility is necessary for the future of research and technology.
Ethical Concerns Surrounding AI Data Ethics in Research
Ethical concerns surrounding AI data ethics in research are increasingly significant as AI-generated data becomes more prevalent. One major concern is the potential for invasion of privacy. Researchers must ensure that data collection methods respect individual rights and comply with regulatory standards. This requires thorough consideration of how data is sourced, stored, and used to prevent unauthorized access or misuse.
Another critical issue is the presence of bias in AI algorithms, which can skew research outcomes. Algorithms trained on biased datasets can inadvertently reinforce existing societal inequalities. Researchers need to actively work towards identifying and mitigating biases in AI-generated data to ensure fairness and equity. Addressing these ethical dilemmas is essential to fostering trust in AI applications and protecting the integrity of research findings. By navigating these concerns, the academic community can promote responsible AI data ethics in research contexts.
Privacy and Security in AI Data Ethics
Privacy and security are pivotal concerns within AI data ethics, particularly when dealing with AI-generated research data. As AI systems increasingly encounter sensitive information, ensuring that this data is handled responsibly becomes paramount. Individuals expect that their personal information, such as social security numbers and birth dates, remains confidential and secure. In an era of heightened data breaches, AI must be designed to prioritize these ethical considerations and maintain user trust.
Moreover, robust security measures are essential to prevent unauthorized access and misuse of data. Organizations must implement strict protocols for data access and processing, ensuring that consent is obtained when using personal information. Transparency in how AI utilizes this data is equally important. Providing clear guidelines on data handling can help mitigate risks and foster confidence among users. In summary, addressing privacy and security in AI data ethics is crucial for protecting individual rights and upholding ethical standards in research practices.
Bias and Fairness in AI Data Ethics
Bias and fairness are significant considerations within AI data ethics, especially in the context of generated research data. AI systems learn from existing data, which can carry inherent biases reflecting societal inequalities. Acknowledging these biases is essential for any ethical framework in AI data ethics. The impact of biased data on research outcomes can lead to discriminatory practices that ultimately shape policy and societal perceptions.
To address bias and promote fairness, one can follow several key steps. First, it is crucial to identify the sources of bias in the data sets being used. Second, implementing diverse data collection methods can help mitigate existing disparities. Third, employing algorithmic fairness assessments during the AI development process will ensure that models produce equitable results. Finally, continuous monitoring and updating of models in response to new data or social changes can enhance fairness and reduce bias. By prioritizing these actions, stakeholders can uphold the integrity and ethical standards necessary in AI data ethics.
Extract insights from interviews, calls, surveys and reviews for insights in minutes
The Role of Transparency and Accountability in AI Data Ethics
Transparency and accountability are essential pillars of AI Data Ethics, particularly in the context of generated research data. Transparency allows stakeholders to understand how data is collected, processed, and utilized. When organizations openly share their methodologies and data sources, it fosters trust and promotes ethical standards. Without transparency, there's a heightened risk of misinformation and unethical practices, which can undermine the credibility of AI-generated research.
Accountability is closely tied to transparency but focuses more on the responsibility organizations have regarding the data they use. Establishing clear guidelines and benchmarks for ethical practices ensure that parties are answerable for their actions. This could involve regular audits, third-party oversight, and clearly defined consequences for lapses in ethical standards. By embracing both transparency and accountability, organizations can promote more robust AI Data Ethics, ultimately leading to better outcomes in research and innovation.
The Importance of Transparency in AI Data Ethics
Transparency plays a crucial role in AI Data Ethics, especially in the realm of research. When data is collected, analyzed, and utilized, it is vital that the processes behind these actions are clear and open. This clarity fosters trust between researchers and the public, ensuring that stakeholders understand how data influences outcomes and decisions. Without transparency, there may be hidden biases or inaccuracies, ultimately affecting the reliability of results and the ethical integrity of research.
Moreover, transparent AI data practices promote accountability among those involved in research. By openly sharing methodologies, data sources, and analytical processes, researchers can be held responsible for their work. This accountability not only strengthens the ethical framework within which AI operates but also empowers consumers and stakeholders to question and verify findings. Ultimately, emphasizing transparency in AI Data Ethics safeguards against misuse and supports a more ethical research environment, reflecting a commitment to high standards of integrity and responsibility.
Ensuring Accountability in AIGenerated Research Data
Ensuring accountability in AI-generated research data is crucial for maintaining ethical standards. To achieve this, organizations must establish clear guidelines and processes that promote integrity in data usage and research outcomes. First, data provenance should be tracked meticulously to verify the origins of the data, ensuring it was collected ethically and complies with applicable regulations. This also helps identify any potential biases that may affect the research findings.
Secondly, implementing robust auditing mechanisms can bolster accountability. Regular reviews of data handling practices will ensure adherence to ethical standards and foster trust among stakeholders. Engaging independent third parties to conduct these audits can enhance transparency. Lastly, fostering a culture of ethical awareness among researchers and developers is essential. Continuous education on AI data ethics will empower them to prioritize accountability in their work, ultimately leading to more reliable and responsible AI-generated research outcomes.
Conclusion on AI Data Ethics in Research
In conclusion, AI Data Ethics plays a critical role in shaping responsible research practices. As the reliance on AI-generated data increases, ethical implications surrounding privacy, bias, and accountability must be thoroughly examined. Researchers and institutions should prioritize ethical standards to ensure that data collection and analysis do not compromise individual rights or perpetuate existing inequalities.
Furthermore, fostering transparency throughout the research process enhances trust and credibility. By openly sharing methodologies and decision-making processes, stakeholders can better evaluate the integrity of AI-generated findings. Ultimately, a commitment to AI Data Ethics not only safeguards participants but also enriches the quality and impact of research outputs.