AI QA Metrics serve as a pivotal tool in enhancing call center operations and ensuring quality assurance. In an industry where customer interactions primarily dictate satisfaction, these metrics provide the necessary framework to evaluate agent performance effectively. By focusing on specific criteria such as compliance and customer engagement, organizations can systematically assess calls, pinpoint weaknesses, and celebrate strengths.
The implementation of AI QA Metrics allows for a more nuanced analysis of data gathered from customer interactions. This approach not only streamlines quality assessments but also empowers call centers to fine-tune their strategies based on real-time insights. As technology continues to evolve, understanding and adopting these metrics will be crucial for maintaining competitive advantage in an increasingly demanding marketplace.
Analyze qualitative data. At Scale.
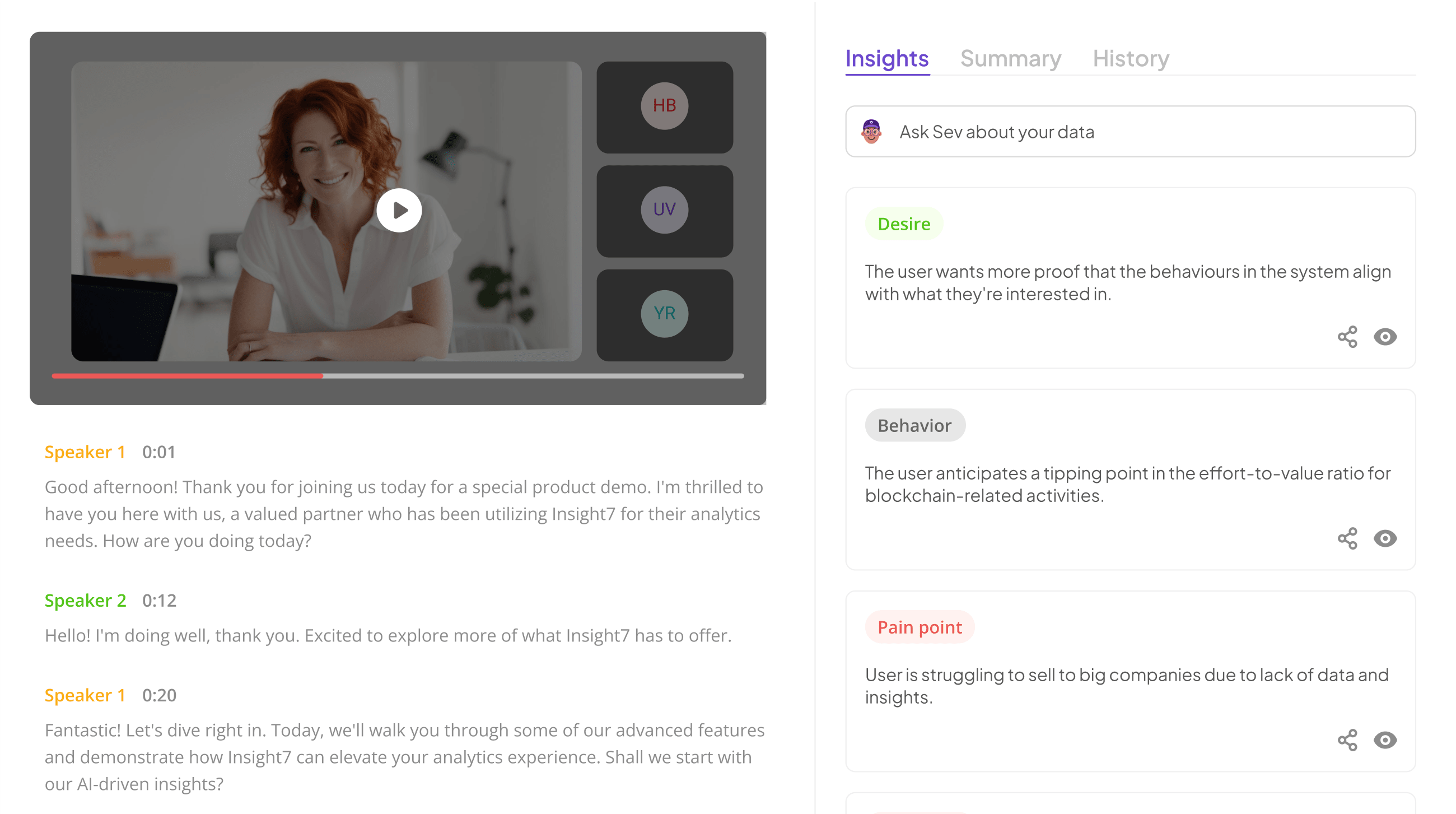
Key Components of AI QA Metrics Development
When developing AI QA Metrics, it is essential to understand the core components that play a pivotal role in measuring call center performance. First, identifying quality indicators is key. These indicators help in evaluating how well interactions align with company standards. Common metrics could include problem identification, customer engagement, and overall satisfaction scores, which form the foundation of quality assurance.
Next, it is important to align the AI capabilities with the specific needs of the call center. This involves customizing evaluation templates that reflect the unique goals of the organization. By leveraging AI technology, organizations can automate the assessment of calls against established criteria, ensuring consistency and reliability. Overall, these foundational elements create a robust structure for AI QA Metrics, enhancing both efficiency and effectiveness in call center operations.
Identifying Quality Indicators for AI QA Metrics
Understanding how to identify quality indicators for AI QA metrics is crucial in the development of effective scoring systems for call centers. Quality indicators serve as measurable benchmarks that ensure AI systems can accurately assess customer interactions. Establishing these indicators begins with defining key aspects, such as compliance, customer satisfaction, and agent performance. By doing so, organizations can tailor AI QA metrics that directly align with their goals.
To create robust quality indicators, consider the following steps:
Define Objectives: Clearly outline the primary goals of the QA process. This could include compliance adherence or customer experience enhancement.
Identify Key Metrics: Determine specific metrics that will reflect performance, such as call resolution rates, average handle time, or customer feedback scores.
Establish Scoring Criteria: Create a detailed framework for scoring based on the identified metrics. This should include weightings for each metric to reflect its importance.
Continuous Review and Improvement: Regularly analyze the effectiveness of the chosen indicators and make necessary adjustments based on emerging insights and business needs.
By focusing on these steps, call centers can implement AI QA metrics that truly enhance service quality and operational efficiency.
Mapping AI Capabilities to Call Center Needs
Understanding how to map AI capabilities to call center needs is vital for enhancing customer service quality. AI QA metrics can streamline evaluation processes, allowing for efficient oversight and training of customer service representatives. By automating call assessments, organizations can save time and focus on actionable insights rather than manual evaluations.
First, identify the specific call center needs based on existing pain points. These might include improving training effectiveness, analyzing customer interactions, or enhancing overall adherence to protocols. Once these needs are established, AI's capabilities can be matched accordingly. For instance, AI technologies can analyze calls for compliance to set parameters, prioritizing efficiency and accuracy. This approach not only improves internal processes but also helps organizations understand customer inquiries and optimize training based on real-world data collected from interactions. Such systematic mapping positions call centers to harness the full potential of AI, ultimately leading to heightened performance and customer satisfaction.
Implementing AI-based QA Scoring in Call Centers
Implementing AI-based QA scoring in call centers is a transformative step that enhances operational efficiency. The first phase involves setting up advanced AI systems tailored to analyze call quality. By integrating tools like NICE Nexidia or Genesys Cloud, organizations can automate the evaluation of customer interactions, dramatically reducing the time spent on manual grading. Once your AI infrastructure is in place, focus on training the models to recognize critical performance indicators, ensuring they align with your desired outcomes.
Next, it's essential to regularly evaluate the performance of AI QA metrics. This involves analyzing the effectiveness of the implemented scoring methods, as well as gathering feedback from team members. Such assessments will not only confirm the accuracy of the metrics but also facilitate ongoing improvements to training processes. By embracing AI QA metrics, call centers can cultivate a culture of compliance and continuous learning, positioning themselves for sustained success in an increasingly competitive landscape.
Extract insights from interviews, calls, surveys and reviews for insights in minutes
Step-by-step Guide to Integrating AI QA Metrics
Integrating AI QA metrics into your call center requires a systematic approach to ensure effectiveness and reliability. Begin by setting up your AI systems using tools like insight7, NICE Nexidia, or Zendesk. Each of these platforms has unique features that can serve your specific quality assurance needs. Once you have established your tools, the next phase involves determining the criteria against which calls will be evaluated.
The training of AI models is crucial as it directly impacts the accuracy of scoring. Consider the quality indicators such as problem identification and resolution effectiveness. Monitor these metrics continuously to refine the AI's performance. By following this step-by-step guide, you can seamlessly integrate AI QA metrics into your operations, enhancing the overall quality of service and customer satisfaction in your call center.
Step 1: Setting Up AI Systems with insight7 and Other Tools
In this initial stage of developing AI QA metrics, setting up your AI systems is crucial for success. Begin by selecting tools that align with your call center's unique needs, such as those specializing in customer interaction analysis. Each tool should facilitate easy integration to streamline the process of monitoring call quality, whether itโs deriving insights from transcripts or identifying patterns in customer feedback.
Several essential tools can optimize this setup. First, consider platforms that offer extensive data visualization capabilities, allowing you to assess call performances readily. Second, choose solutions with robust analytic features to extract key insights about customer experiences, such as pain points and satisfaction levels. By effectively harnessing these tools, your team can create a strong foundation that enhances the overall QA scoring process in your call center, ensuring continuous improvement and superior customer service.
- insight7
In the journey of developing AI QA metrics for call centers, understanding and implementing key elements is essential. One major aspect to explore is identifying quality indicators that can inform the creation of effective AI QA metrics. These indicators should reflect critical areas of customer interaction, including response time, issue resolution rates, and customer satisfaction scores. By pinpointing these aspects, organizations can enhance their scoring systems, leading to improved customer service outcomes.
Next, successful integration of AI QA metrics relies on aligning these measures with specific call center needs. This involves mapping AI capabilities to the unique challenges faced in customer service environments. By ensuring that AI tools directly address these challenges, organizations can foster a more proactive approach to customer interaction. As a result, AI QA metrics become a valuable resource for driving business outcomes, improving service quality, and establishing a competitive edge in the marketplace.
- NICE Nexidia
Integrating AI QA Metrics can effectively enhance the quality assurance processes in call centers. A leading solution in this field offers an intuitive platform that enables users to easily upload call recordings for analysis. By transcribing these audio files, the system allows users to uncover critical insights from customer interactions. This foundational step is crucial as it sets the stage for further evaluation of call performance.
The platform also provides various templates for users to navigate specific metrics, such as identifying pain points or assessing customer sentiment. It is designed to handle bulk analyses, making it suitable for high-volume operations. With features that present key insights and important excerpts from conversations, users can quickly visualize performance metrics. This streamlined approach not only saves time but also empowers organizations to make data-driven decisions that improve overall service quality.
- Cogito
Cogito plays a pivotal role in the development of AI QA Metrics, especially in enhancing call center performance. At its core, Cogito leverages advanced machine learning to provide real-time feedback on customer interactions. By analyzing conversations, it identifies various quality indicators, such as empathy, responsiveness, and the effectiveness of communication strategies. This feedback empowers customer service representatives to engage more effectively with customers and encourages a proactive approach in addressing their needs.
Integrating Cogito into the call center workflow enhances overall customer experience. With AI QA Metrics derived from Cogito, teams can better understand customer sentiment and adjust their responses accordingly. The insights gained enable continuous learning and adaptation, helping teams maintain high service standards. In essence, Cogito not only transforms raw customer interactions into actionable insights but also fosters a culture of improvement, ensuring that call centers are prepared to meet evolving customer expectations effectively.
- Zendesk
In the realm of AI-based call center QA scoring metrics, platforms like Zendesk play a pivotal role. This tool simplifies the process of gathering and analyzing customer interactions, enabling organizations to derive valuable AI QA metrics. By integrating with AI technology, Zendesk allows for automated analysis of call quality, ensuring that customer service representatives adhere to established frameworks during interactions.
Key features such as conversation tracking and reporting capabilities facilitate the continuous evaluation of service quality. By measuring agent performance against predefined metrics, businesses can identify strengths and areas for improvement. Ultimately, Zendesk empowers companies to optimize call center operations, enhance customer experiences, and implement data-driven decisions that lead to superior service outcomes. Investing in such tools is instrumental in refining the quality assurance process, which is critical for ongoing success in the competitive landscape of customer service.
- Genesys Cloud
In the context of developing AI-based call center quality assurance (QA) scoring metrics, the significance of a robust platform like Genesys Cloud becomes clear. This platform efficiently facilitates the integration of various tools and data sources to enhance customer conversations. It seamlessly captures calls and transforms them into meaningful transcripts for analysis, helping teams transcribe, assess, and gain insights from interactions with customers.
With the capability to operate advanced dashboards and analytics tools, users can easily identify trends and patterns in customer interactions. Through this platform, organizations have the potential to elevate their customer service experience by employing AI QA metrics. These insights enable institutions to shift their approach from merely handling calls to delivering personalized consultations, leading to improved satisfaction levels.
The effective use of this technology supports ongoing training and development, fostering a culture of continuous improvement. The insights gained can ultimately refine the quality indicators, paving the way for a more effective call center operation.
Step 2: Training AI Models for Accurate Scoring
Training AI models is a critical step to ensure accurate scoring within the call center environment. By utilizing historical call data, these models can learn the intricacies of customer interactions. This process involves feeding the AI system recordings and transcripts of calls, enabling it to identify patterns related to successful customer service engagements. Proper training ensures the AI can differentiate between various levels of service quality and pinpoint areas for improvement.
To enhance the effectiveness of AI QA metrics, several key actions must be taken: first, clearly define scoring criteria based on business goals; second, utilize diverse datasets to train the models, ensuring they encompass various scenarios; third, continuously validate and refine the models using updated data to maintain accuracy. Adopting these practices ensures that the AI system evolves alongside changing customer needs and preferences, ultimately fostering a culture of excellence in call center performance.
Evaluating the Impact of AI QA Metrics
The evaluation of AI QA metrics is crucial for understanding their effectiveness in enhancing call center performance. By implementing these metrics, organizations can track compliance and identify areas for improvement. This evaluation often involves analyzing call data against predefined criteria, which allows for a structured approach to quality assessment. It helps determine whether the AI is accurately capturing the nuances of customer interactions.
To measure the impact of AI QA metrics, several key factors should be considered. First, assess the accuracy of the AI's scoring compared to human evaluations. This comparison can reveal whether the AI is providing consistent and reliable insights. Second, monitor improvements in customer satisfaction and operational efficiency over time. Noting these changes can provide clarity on the overall effectiveness of AI metrics. Finally, gather feedback from agents to understand their experience and whether the metrics enhance their performance.
Conclusion on Enhancing Call Centers with AI QA Metrics
To significantly enhance call centers, AI QA metrics provide a transformative approach to evaluating performance. By automating quality assurance processes, organizations can streamline their review systems and gain insights into customer service interactions. Advanced AI tools can analyze numerous calls efficiently, offering precise scoring based on established parameters, which ultimately improves agent training and compliance.
Moreover, AI QA metrics facilitate the identification of common customer inquiries and trends, aligning training efforts with actual service needs. As organizations adopt these metrics, they not only enhance operational efficiency but also foster a deeper understanding of customer expectations. This evolution in call center management can lead to improved service quality and a more satisfied customer base.