In the rapidly evolving world of data analytics, 2024 promises to revolutionize how businesses leverage insights. The future analytics toolbox will empower organizations with advanced descriptive, diagnostic, predictive, and prescriptive tools designed to optimize decision-making processes. This transformative approach will not only enhance operational efficiency but also foster innovation, putting data at the heart of every strategy.
As organizations embrace this future analytics toolbox, they will gain a deeper understanding of consumer behavior and market trends. With the integration of sophisticated technologies, insights from descriptive and diagnostic tools will drive actionable strategies, enabling predictive analytics to forecast outcomes effectively. Ultimately, prescriptive tools will provide tailored recommendations, helping businesses navigate complexities with confidence and precision.
Analyze qualitative data. At Scale.
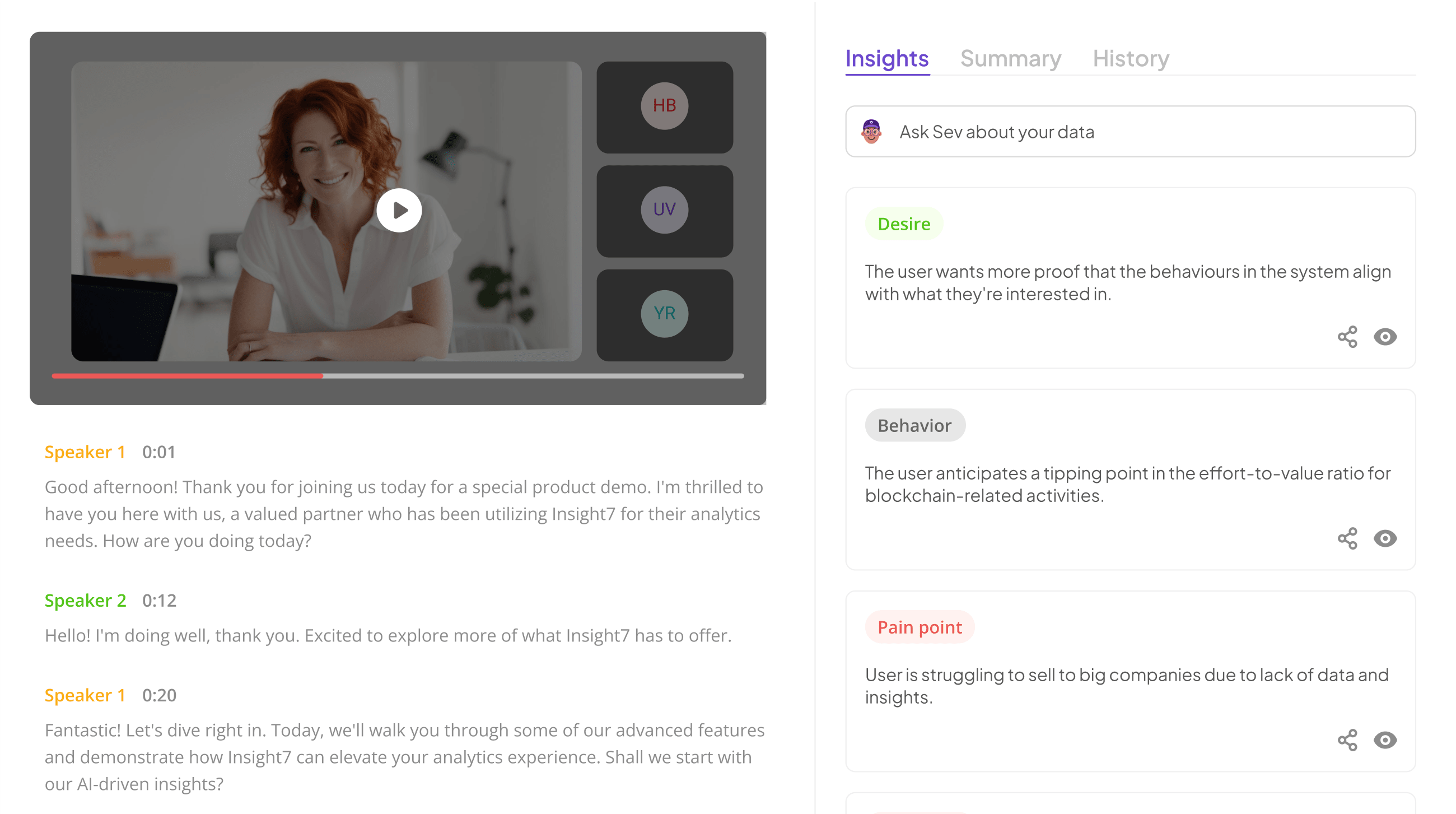
Unveiling the Future Analytics Toolbox: Descriptive and Diagnostic Tools
The Future Analytics Toolbox brings forth a transformation in how businesses engage with data. Descriptive tools play a crucial role in this evolution, offering straightforward insights and visualizations. This section will explore the multifaceted benefits of using these tools to understand past performance, identify trends, and make informed decisions. By synthesizing data, organizations can uncover what has happened, which is fundamental for strategic planning.
On the other hand, diagnostic tools dig deeper into data to answer the critical "why" behind observed trends. By employing advanced analytics, companies can determine the root causes of specific outcomes. This analytical approach allows organizations to refine their strategies and improve operational efficiency. With the Future Analytics Toolbox, combining both descriptive and diagnostic tools empowers businesses to not only reflect on their past but also anticipate future challenges and opportunities dynamically.
Exploring Descriptive Tools in 2024
In 2024, descriptive tools will play a crucial role in refining data visualization and analysis within the Future Analytics Toolbox. These tools serve as the foundation for understanding historical data, which more effectively informs decision-making processes. By transforming complex datasets into easily interpreted visual formats, descriptive tools provide insights that help organizations track performance and identify trends.
For instance, tools like Tableau and Power BI facilitate the creation of interactive dashboards that allow users to explore data dynamically. Users can segment and filter data to extract meaningful insights, uncovering patterns and nuances otherwise hidden in raw datasets. As organizations seek to enhance data literacy and empower stakeholders, exploring these descriptive tools will become essential for achieving strategic goals, optimizing processes, and ultimately driving growth. The combination of powerful analytics with user-friendly interfaces will redefine how businesses utilize data to inform their strategies.
- Insight7
Understanding the role of Insight7 in the Future Analytics Toolbox is crucial for businesses aiming to stay competitive. This innovative platform streamlines the process of data analysis, making it easy for organizations to derive actionable customer insights from vast amounts of conversation data. Traditional methods often fall short in todayโs fast-paced environment, but Insight7 empowers users to extract meaningful patterns from customer interactions quickly and effectively.
Furthermore, businesses can overcome common challenges, such as slow data processing and inefficient collaboration. By consolidating insights into a unified platform, Insight7 not only accelerates decision-making but also enhances strategic planning. As we navigate the complexities of data in 2024, tools like Insight7 will be essential in equipping organizations with the insights they need to thrive in a competitive landscape. Embracing such technology will enable firms to proactively address customer needs and streamline their business strategies effectively.
- Tableau
Tableau stands out as a key component in the Future Analytics Toolbox for 2024, revolutionizing the way data is analyzed and visualized. This powerful tool allows users to create interactive and shareable dashboards that depict trends, patterns, and insights derived from diverse data sources. By using Tableau, businesses can transform complex datasets into visually engaging formats that enhance understanding and drive decision-making.
One of Tableau's remarkable features is its ability to connect to multiple data sources, providing a comprehensive view of various metrics and key performance indicators. Users can employ advanced visualization techniques to uncover insights that are otherwise hidden within raw data. Additionally, Tableau's analytics capabilities accommodate descriptive, diagnostic, predictive, and even prescriptive analytics, making it an all-encompassing tool for organizations aiming to leverage data effectively. With Tableau, enterprises can navigate their data landscape efficiently and make informed decisions for future growth and success.
- Power BI
Power BI plays an essential role in the Future Analytics Toolbox for 2024. Its capabilities enable users to analyze vast datasets, providing insights that drive decision-making. Through intuitive data visualization and real-time reporting, Power BI aids organizations in identifying trends and patterns, allowing for timely actions based on these findings. This platform transforms raw data into meaningful visualizations, making complex data sets easier to interpret and share.
Understanding customer pain points is crucial. With Power BI, users can track key performance indicators across various metrics. This tool combines descriptive analytics with interactive dashboards, empowering businesses to grasp their performance metrics quickly. As organizations adapt to an increasingly data-driven environment, the integration of Power BI into their analytics strategy will prove vital for harnessing actionable insights within the Future Analytics Toolbox.
Navigating Diagnostic Tools with the Future Analytics Toolbox
Navigating diagnostic tools with the Future Analytics Toolbox is an essential step toward intelligent data interpretation. These tools allow businesses to analyze their historical data and identify patterns that inform future decisions. With platforms like Looker, SAP Analytics Cloud, and RapidMiner, users can easily sift through vast amounts of information to discover insights that were previously hidden.
By integrating these diagnostic tools within the Future Analytics Toolbox, organizations can enhance their problem-solving capabilities significantly. For instance, Looker enables real-time data visualization, allowing for quick insights into operational efficiencies. Similarly, SAP Analytics Cloud consolidates data from multiple sources, facilitating comprehensive analysis. Lastly, RapidMiner offers advanced predictive analytics techniques to analyze complex datasets. As businesses prepare for 2024, effectively utilizing these diagnostic tools will empower them to make well-informed, strategic decisions while navigating the evolving analytics landscape.
- Looker
Looker stands out as a notable player in the evolving landscape of analytics tools for 2024. It effectively bridges descriptive analytics and actionable insights, making it an integral component of the Future Analytics Toolbox. By offering a user-friendly interface and powerful data visualization features, Looker helps organizations unlock patterns hidden within their data. This capability enables businesses to make informed decisions based on empirical evidence rather than intuition.
In the realm of diagnostic analysis, Looker excels by providing interactive dashboards that facilitate exploration and comprehension of complex data sets. With its API-driven architecture, it allows for seamless integration with other platforms, enhancing collaborative efforts across teams. As organizations increasingly rely on data-driven strategies, incorporating Looker into their analytics toolkit can lead to significant improvements in operational efficiency and strategic planning. Embracing tools like Looker ensures that entities remain competitive in an ever-changing digital environment.
- SAP Analytics Cloud
SAP Analytics Cloud offers a comprehensive platform for organizations seeking an effective, future-proof analytics solution. With its blend of connectivity, visualization, and intelligent insights, it plays a vital role in the Future Analytics Toolbox of 2024. Users can seamlessly integrate data from various sources, making it easier to gather critical insights that drive informed decision-making.
Beyond simple data visualization, SAP Analytics Cloud supports advanced analytics capabilities. It facilitates both descriptive and diagnostic analysis, empowering users to identify trends and explore root causes. With features such as predictive forecasting and planning, organizations can proactively navigate market changes, ultimately optimizing their strategy. As businesses increasingly rely on data-driven insights, harnessing the full potential of tools like SAP Analytics Cloud becomes essential for navigating the complexities of the modern business landscape.
- RapidMiner
RapidMiner stands as a key player in the Future Analytics Toolbox, especially for businesses seeking to enhance decision-making through data insights. This platform offers a comprehensive suite of tools aimed at extracting valuable information from complex datasets. Users can easily set up projects, allowing them to compile and analyze various data sources efficiently. This simplicity is critical, as organizations strive to make data-driven decisions swiftly in todayโs fast-paced environment.
Additionally, RapidMiner provides powerful data visualization capabilities, helping users uncover meaningful patterns and trends from their analyses. By transforming raw data into visually engaging formats, stakeholders can grasp concepts quickly and communicate findings effectively. This focus on user experience makes RapidMiner an essential tool that aligns well with the descriptive and diagnostic analytics needed in 2024, positioning it as a pivotal resource in the journey toward informed business strategies.
Extract insights from interviews, calls, surveys
and reviews for insights in minutes
Bridging to Predictive and Prescriptive Tools in the Future Analytics Toolbox
Extract insights from interviews, calls, surveys and reviews for insights in minutes
Extract insights from interviews, calls, surveys and reviews for insights in minutes
Leveraging Predictive Tools for Tomorrow
The Future Analytics Toolbox offers a robust suite of predictive tools to anticipate and shape the market landscape. These tools analyze historical data to forecast future trends, enabling businesses to make informed decisions. Organizations can tap into advanced analytics platforms that utilize machine learning techniques to identify patterns and insights. By harnessing these predictive capabilities, companies can proactively address challenges while optimizing strategies.
Utilizing predictive tools not only enhances decision-making but also encourages collaboration across teams. With easy-to-use interfaces and accessible features, employees at all levels can engage with data effectively. For instance, platforms like IBM Watson Analytics and SAS Advanced Analytics allow users to visualize data trends, translating complex analytics into actionable insights. Embracing these technologies will undoubtedly position businesses for success in 2024 and beyond, ensuring they are prepared to meet the evolving demands of their industry.
- IBM Watson Analytics
In the ever-evolving landscape of data analytics, a robust analytical tool is essential for organizations aiming to leverage insights effectively. IBM Watson Analytics stands out as a leading option within the Future Analytics Toolbox, offering a comprehensive suite of features designed for various analytical needs. This platform empowers users to transform raw data into actionable insights through descriptive, diagnostic, predictive, and prescriptive analytics seamlessly integrated into one interface.
With its advanced natural language processing capabilities, users can easily interrogate datasets and visualize results intuitively. Whether you're analyzing customer feedback or examining product performance, the tool simplifies complex queries, making data-driven decisions more accessible. By facilitating the exploration of patterns and trends across datasets, it enables organizations to remain competitive and informed in their strategic planning. As 2024 approaches, the importance of harnessing such powerful analytical tools will be paramount for businesses striving to thrive in a data-centric marketplace.
- SAS Advanced Analytics
SAS Advanced Analytics plays a crucial role in the Future Analytics Toolbox, enabling organizations to harness the power of data for informed decision-making. With a robust suite of analytical capabilities, this tool supports both predictive and prescriptive analytics, allowing users to foresee trends and optimize outcomes. By utilizing advanced statistical methods, it empowers analysts to uncover hidden insights that lead to better strategic planning and execution.
To understand the impact of SAS Advanced Analytics, itโs essential to consider its key features. First, data mining and predictive modeling help identify relationships and forecast future scenarios. Second, machine learning techniques enhance the ability to analyze large datasets efficiently. Lastly, integration with various data sources provides a comprehensive view of organizational performance. These functionalities not only improve data interpretation but also facilitate timely interventions, making SAS an indispensable component of any forward-thinking analytics strategy in 2024.
Implementing Prescriptive Tools in the Future Analytics Toolbox
In the Future Analytics Toolbox, implementing prescriptive tools is crucial for driving effective decision-making. These tools go beyond merely analyzing data; they provide actionable recommendations based on predictive insights. By integrating models like Oracle Crystal Ball and Gurobi Optimization, organizations can easily identify optimal strategies while minimizing risks.
The implementation process begins with data integration and the establishment of clear goals. This ensures that tools align with specific organizational needs. Following this, organizations should focus on utilizing advanced algorithms to analyze potential outcomes and recommend the best courses of action. Continuous monitoring and adjustment of these models are essential for improving accuracy and relevance, ultimately leading to better business outcomes in 2024 and beyond.
- Oracle Crystal Ball
Oracle Crystal Ball stands out as a pivotal tool within the Future Analytics Toolbox for 2024. It empowers users to make informed predictions based on quantitative modeling and simulation techniques. By integrating risk analysis and forecasting into decision-making, it enables organizations to anticipate future outcomes more accurately. This capability is crucial in an increasingly unpredictable business environment where data-driven insights are essential for success.
The platform uses Monte Carlo simulations to assess risks and uncertainties, which enhances understanding of potential fluctuations in project forecasts. Explicitly visualizing various scenarios helps decision-makers understand the implications of their choices. In this context, organizations aiming for strategic advantages can utilize Oracle Crystal Ball to refine their predictive and prescriptive methodologies, ultimately fostering a more resilient approach to planning and investment. The ability to visualize future possibilities makes this tool indispensable for any analytics-driven strategy in 2024.
- Gurobi Optimization
Gurobi Optimization plays a crucial role in the Future Analytics Toolbox by enabling businesses to make informed decisions through mathematical optimization. As organizations strive to enhance their operational efficiency, Gurobi provides a powerful platform that specializes in solving complex optimization problems across numerous sectors, including finance, manufacturing, and logistics. By using advanced algorithms, it helps companies identify the best strategies to maximize profits, minimize costs, and allocate resources effectively.
In 2024, businesses will increasingly rely on these optimization tools to gain insights and drive actionable outcomes. The importance of integrating Gurobi within the Future Analytics Toolbox cannot be overstated. It allows for more precise forecasting and decision-making, transforming raw data into valuable insights. As companies navigate an ever-evolving landscape, Gurobi Optimization stands out as an essential tool for fostering innovation and ensuring sustainable growth. Embracing these technologies will undoubtedly shape the future of business analytics.
Conclusion: The Integrated Future Analytics Toolbox for 2024 and Beyond
As we look ahead to 2024 and beyond, the Integrated Future Analytics Toolbox emerges as a vital resource for businesses. By combining descriptive, diagnostic, predictive, and prescriptive tools, companies will gain unprecedented access to insights that drive strategic decision-making. This toolbox not only democratizes data access but also empowers users at all levels to extract valuable information without requiring extensive expertise.
The implementation of these integrated tools will facilitate a deeper understanding of market dynamics, customer behaviors, and operational efficiencies. Organizations can effectively leverage data insights to navigate challenges and seize opportunities. Embracing this Future Analytics Toolbox represents a commitment to fostering a culture of data-driven decision-making, ultimately leading towards sustained growth and competitive advantage.