Text Insight Extraction serves as a pivotal aspect of Data Text Analytics, enabling organizations to uncover valuable information from dense volumes of unstructured text. By transforming conversations, feedback, and other textual data into actionable insights, businesses can make informed decisions. The process begins with gathering data, followed by sophisticated analysis techniques that sift through textual nuances, revealing patterns and trends.
Understanding the mechanics of Text Insight Extraction is crucial for any organization engaged in data-driven decision-making. This practice not only enhances customer understanding but also informs strategic planning and product development. In an era where information is abundant, mastering these extraction techniques allows businesses to stay competitive and adaptive in a rapidly evolving landscape.
Generate visualizations from your qualitative data. At Scale.
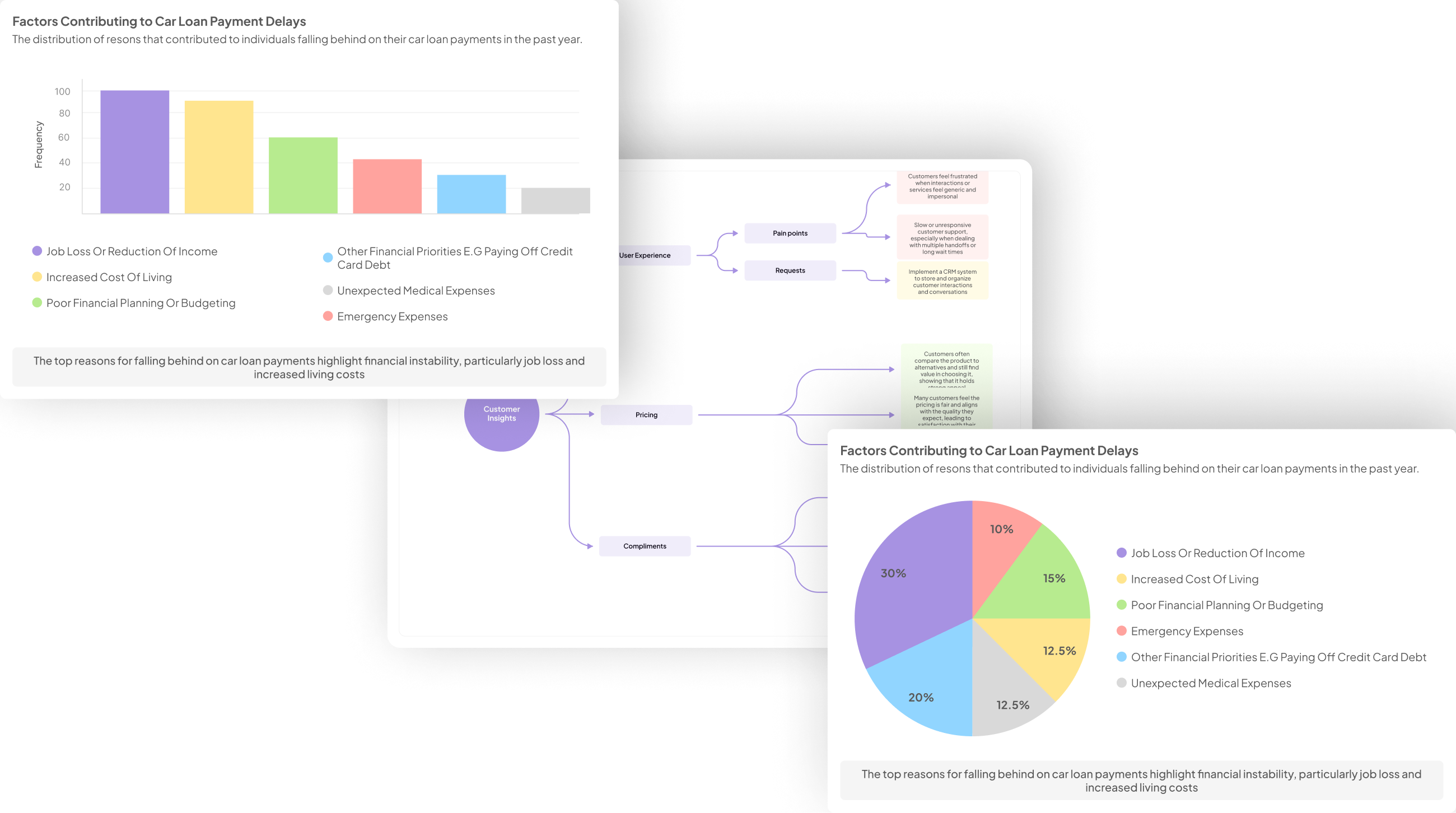
Understanding Text Insight Extraction
Effective Text Insight Extraction involves transforming raw textual data into meaningful insights that guide decision-making. This process begins with meticulous data collection, where relevant text is amassed from multiple sources. Once collected, the text undergoes preprocessing, which ensures uniformity by removing noise, such as irrelevant information and formatting inconsistencies.
Following preprocessing, advanced techniques like Natural Language Processing (NLP) come into play. NLP enables the extraction of keywords, themes, and sentiments embedded within the text. This allows organizations to identify patterns, customer feedback, and market trends efficiently. Furthermore, insights garnered through this process can inform strategic planning, product development, and customer engagement strategies. Utilizing Text Insight Extraction empowers businesses to transform vast amounts of unstructured data into actionable intelligence, enhancing overall operational effectiveness and responsiveness to market needs.
The Role of Natural Language Processing (NLP)
Natural Language Processing (NLP) plays a pivotal role in transforming raw text into actionable insights. By analyzing human language, NLP allows systems to recognize patterns and sentiments within vast amounts of data, enabling businesses to derive meaningful conclusions. This process is crucial for any organization looking to achieve Text Insight Extraction effectively.
When applied in data text analytics, NLP encompasses various techniques that facilitate understanding human interactions, such as customer interviews or feedback. These techniques include tokenization, sentiment analysis, and named entity recognition, each serving to refine and extract valuable information from textual data. By streamlining this analytical process, organizations can convert scattered insights into coherent strategies, ultimately enhancing decision-making and fostering collaboration across teams.
The Importance of Contextual Analysis
Contextual analysis plays a crucial role in Text Insight Extraction, enhancing the comprehension of language and its meaning in varied situations. Understanding the context surrounding data is essential for accurate interpretation and decision-making. When analyzing customer interactions, the nuances in tone, intent, and content can significantly alter the insights derived from the text.
Additionally, context helps in uncovering deeper meanings and implications behind words. Factors such as cultural references, industry jargon, and user behavior can influence how messages are perceived. By integrating contextual analysis into text analytics, organizations can ensure that their insights are not only accurate but also relevant. This approach not only leads to better customer engagement but also fosters a culture of informed decision-making, ultimately driving business success. Investing in contextual analysis is, thus, a vital strategy for leveraging data effectively in today's dynamic market.
Generate Journey maps, Mind maps, Bar charts and more from your data in Minutes
Mechanisms of Text Insight Extraction
In the journey of extracting valuable insights from textual data, several mechanisms come into play. First, data collection and preprocessing set the foundation for effective text insight extraction. This step involves gathering relevant information from various sources, such as customer interviews and feedback. After collection, the data undergoes preprocessing, which includes cleaning, normalizing, and organizing it for analysis.
Next, the focus shifts to text analysis and extraction. This phase utilizes algorithms, often underpinned by natural language processing, to identify patterns, sentiments, and key themes within the text. The extracted insights can inform business strategies, enhance collaboration, and streamline decision-making processes. By employing advanced tools and technologies, organizations can significantly improve their capabilities for text insight extraction. These processes collectively empower businesses to act promptly on customer signals, gaining a competitive edge in today's rapidly evolving market.
Step-by-Step Process of Data Text Analytics
To effectively navigate the step-by-step process of data text analytics, one must first focus on data collection and preprocessing. This foundational step involves gathering textual data from various sources, ensuring a rich and diverse dataset. Once collected, the data undergoes preprocessing, which includes cleaning, removing noise, and formatting to make it suitable for analysis. Effective preprocessing is crucial for accurate text insight extraction, as it enhances the quality of the input data.
Following preprocessing, the next phase is text analysis and extraction. Here, advanced techniques such as natural language processing (NLP) come into play, allowing the system to analyze and derive meaningful insights. Analysts can identify patterns, sentiments, and themes within the text, resulting in valuable extracted insights. With these two structured phasesโdata collection and text analysisโtext insight extraction becomes a powerful tool in uncovering actionable knowledge from vast amounts of textual data.
Data Collection and Preprocessing
Data collection and preprocessing are critical stages in text insight extraction. Initially, data collection involves gathering relevant text from various sources, such as transcripts, web pages, and social media. This diverse data pool is essential for uncovering valuable insights. Once data is collected, it undergoes preprocessing to prepare it for analysis. Preprocessing includes cleaning the text, removing unnecessary characters, and normalizing the data.
During this phase, several processes are usually executed. First, tokenization splits textual data into manageable segments like words or sentences. Second, stemming and lemmatization help in reducing words to their base forms, streamlining the analysis effort. Third, removing stop words eliminates common words that may not contribute to the overall meaning. By systematically executing these steps, you can significantly enhance the quality of data, setting a strong foundation for effective text analysis and insight extraction.
Text Analysis and Extraction
Text Analysis and Extraction involves the systematic examination of textual data to derive meaningful insights. It plays a crucial role in understanding customer feedback and market trends. By converting unstructured text into structured information, businesses can identify sentiments, key themes, and actionable items. This process enhances decision-making and drives strategic initiatives.
In essence, Text Insight Extraction unfolds in multiple stages. Initially, data collection occurs, where relevant textual data is gathered from sources like surveys or social media. Next, the analysis phase begins, employing advanced techniques such as Natural Language Processing (NLP) to interpret the text. Ultimately, insights are extracted, summarized, and presented in a format easily digestible for stakeholders. This method allows organizations to make informed decisions, ensuring they remain ahead of their competition while addressing customer needs effectively.
Tools and Technologies Enhancing Text Insight Extraction
Various tools and technologies are crucial for enhancing Text Insight Extraction, enabling businesses to transform raw text into valuable insights. These tools utilize advanced algorithms and machine learning to analyze large datasets, making it easier to identify patterns and trends. For instance, Natural Language Processing (NLP) techniques allow users to derive meaning from customer interactions, resulting in actionable feedback that drives decision-making.
Several key categories of tools significantly improve this process. First, transcription software automates the capture of spoken dialogues, converting conversations into text for further analysis. Second, data visualization platforms offer intuitive interfaces for examining insights, allowing users to see trends at a glance. Third, sentiment analysis tools assess the emotional tone behind user feedback, helping businesses understand customer perceptions more deeply. By integrating these technologies, organizations can streamline their Text Insight Extraction efforts, ensuring they obtain critical insights that inform strategy and improve user experience.
Conclusion: Harnessing the Power of Text Insight Extraction
Text Insight Extraction serves as a vital tool in transforming unstructured data into actionable insights. By effectively analyzing vast volumes of text, organizations can uncover valuable patterns and trends. This process enables decision-makers to identify pain points, customer desires, and behavioral shifts, creating a clearer understanding of their audience.
Moreover, the democratization of this technology means that anyone within a business can utilize it without requiring specialized training. As more individuals engage with Text Insight Extraction, their collective insights contribute to a deeper knowledge base, ultimately driving innovative solutions and improving customer experiences. Adopting this approach can profoundly influence strategic planning and operational efficiency.