In today's competitive market, understanding customer behavior has never been more crucial. Businesses are inundated with data, yet the challenge lies in converting that data into meaningful insights. This is where Customer Insight Techniques come into play, providing a roadmap for organizations to unlock invaluable information about their customers. By effectively utilizing these techniques, companies can enhance their strategies and outpace their rivals.
Customer Insight Techniques focus on gathering and interpreting customer data to reveal patterns and preferences. These methods allow businesses to better understand their audience, leading to improved product offerings and customer engagement. Successful application of these techniques not only facilitates informed decision-making but also fosters stronger connections with customers, ultimately driving growth and satisfaction.
Generate visualizations from your qualitative data. At Scale.
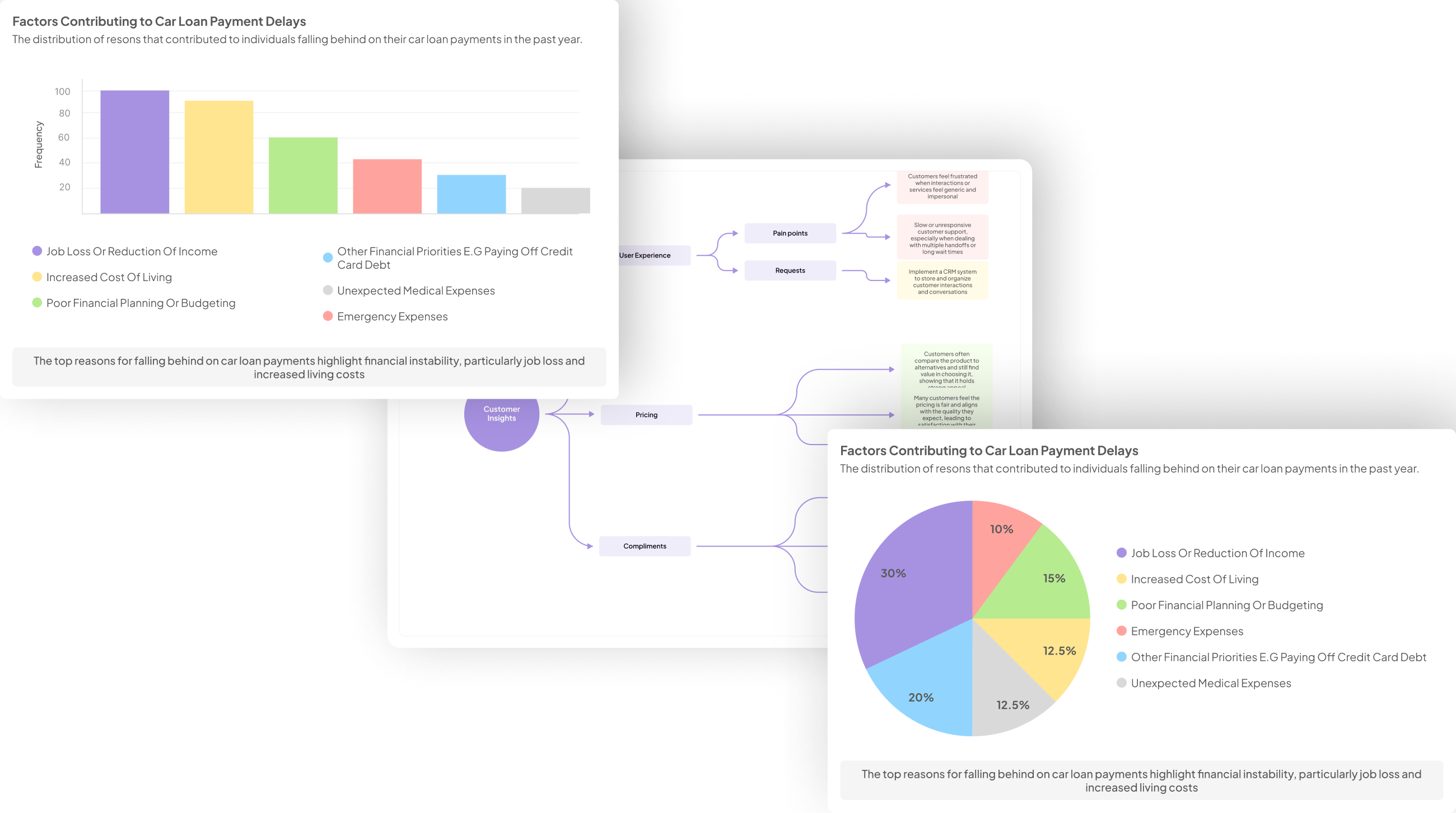
Understanding Customer Insight Techniques in Segmentation
To understand Customer Insight Techniques in segmentation, it's crucial to explore how different methods can reveal valuable customer data. Effective segmentation allows businesses to identify distinct groups within their customer base, tailoring strategies that meet each group's specific needs and preferences. Techniques such as demographic analysis, behavioral segmentation, and psychographic profiling provide rich insights into customer motivations and habits.
Moreover, advanced methods like clustering algorithms and propensity modeling enhance segmentation efforts. These techniques not only categorize customers but also predict future behaviors, helping companies make data-driven decisions. By integrating various Customer Insight Techniques, businesses can create a dynamic approach to reach, engage, and retain their customers effectively. Understanding these techniques ultimately leads to more strategic marketing efforts and improved customer satisfaction, driving overall business success. So, in essence, the power of segmentation lies in its ability to turn customer insights into actionable strategies that foster growth.
Techniques for Identifying Customer Segments
To effectively identify customer segments, businesses can utilize a variety of techniques that deliver valuable insights. One foundational approach is demographic segmentation, where data on age, gender, income, and education can provide a clear picture of distinct groups within your customer base. Another powerful method is psychographic segmentation, which delves into customer lifestyles, values, and interests. Understanding these connections allows you to tailor marketing strategies that resonate deeply with specific segments.
Additionally, behavioral segmentation focuses on customer actions, such as purchase history and brand loyalty, revealing patterns that can be used to effectively target communications. Firms can also apply data analytics tools to process and analyze large data sets, uncovering trends that are not always visible through traditional methods. By employing these customer insight techniques, businesses can make informed decisions that enhance engagement and drive growth. Understanding how to apply these methods leads to a more nuanced and productive approach to reaching your target audience.
Tools for Analyzing Customer Segments
Tools for analyzing customer segments play a crucial role in understanding market dynamics. Various methodologies can dive deep into consumer behavior, helping businesses identify distinct groups within their audience. By utilizing relevant tools, organizations can extract meaningful patterns and insights from large datasets, providing a clearer picture of customer preferences.
Several effective tools can be utilized for segment analysis. First, customer relationship management (CRM) systems allow firms to segment customers based on purchase history and interactions. Second, survey tools enable you to gather detailed feedback from your audience, which can be segmented based on demographics and psychographics. Lastly, data visualization software helps in interpreting complex data, making it easier to identify trends. By using these tools, businesses can gain valuable customer insights, informing strategic decisions and marketing initiatives effectively.
Generate Journey maps, Mind maps, Bar charts and more from your data in Minutes
Applying Customer Insight Techniques in Predictive Analysis
Customer insight techniques play a vital role in predictive analysis, enabling businesses to anticipate customer behaviors effectively. By understanding customer preferences and patterns, companies can refine their strategies to meet evolving demands. This approach requires a blend of data collection, analysis, and the application of insights to forecast future trends accurately.
To apply customer insight techniques in predictive analysis, consider the following steps:
Data Collection: Gather comprehensive data from various customer interactions, such as surveys, purchase histories, and feedback forms.
Data Analysis: Employ analytical tools to filter and interpret the collected data, identifying key behavior patterns and trends.
Predictive Modeling: Develop models that utilize historical data to predict future customer behavior, enhancing the decision-making process.
Continuous Improvement: Regularly update models with new data to ensure accuracy and relevance in predictions.
By following these steps, organizations can effectively harness customer insight techniques to drive growth and maintain a competitive edge in the market.
Steps to Develop Predictive Models
Developing predictive models involves several crucial steps that inform decisions based on customer insight techniques. First, understand your goals. Clearly define what you aim to predict, such as customer behavior, churn rates, or sales forecasts. Identifying the foundation allows you to structure your data collection around the desired outcome.
Next, gather and prepare your data. Large volumes of customer data, including demographics, transaction history, and interaction logs, must be cleaned and organized for analysis. After preparing your dataset, choose the right analytical model tailored to your needs. Various algorithms, such as regression or decision trees, can be employed depending on the complexity of your prediction task. Finally, validate and refine the model. Testing with historical data ensures its reliability in making accurate forecasts. By following these steps, you can leverage predictive models to gain valuable insights and enhance customer satisfaction significantly.
Utilizing Machine Learning for Customer Predictions
Machine learning plays a pivotal role in refining customer predictions by analyzing vast datasets. Utilizing advanced algorithms, businesses can uncover hidden patterns and trends, leading to enhanced decision-making. For instance, these predictive models facilitate an understanding of customer behavior, enabling personalized marketing strategies and tailored product recommendations.
To ensure effective predictions, follow several key principles. First, gather comprehensive customer data, including demographics, purchase history, and feedback. Next, choose the appropriate algorithms, such as regression analysis or classification techniques, based on your specific goals. Finally, continually train and update your models with new data to maintain accuracy. By implementing these strategies, organizations can significantly improve their customer insight techniques, fostering deeper connections with their audience and driving growth. This proactive approach empowers businesses to act on insights and adapt swiftly to evolving customer needs.
Conclusion: Harnessing Customer Insight Techniques for Business Growth
Customer Insight Techniques are essential for unlocking business growth in today's competitive environment. By effectively gathering and analyzing customer data, organizations can deepen their understanding of customer preferences and behaviors. This knowledge enables businesses to tailor their products and services, ultimately driving customer satisfaction and loyalty.
To fully harness these techniques, companies must prioritize the timely application of insights. Streamlining data analysis processes helps transform raw information into actionable strategies. By doing so, organizations can foster a proactive culture focused on customer-centric decision-making, thus ensuring ongoing growth and success in an ever-evolving market landscape.